⚠️ 以下所有内容总结都来自于 大语言模型的能力,如有错误,仅供参考,谨慎使用
🔴 请注意:千万不要用于严肃的学术场景,只能用于论文阅读前的初筛!
💗 如果您觉得我们的项目对您有帮助 ChatPaperFree ,还请您给我们一些鼓励!⭐️ HuggingFace免费体验
2025-04-30 更新
Generative Adversarial Network based Voice Conversion: Techniques, Challenges, and Recent Advancements
Authors:Sandipan Dhar, Nanda Dulal Jana, Swagatam Das
Voice conversion (VC) stands as a crucial research area in speech synthesis, enabling the transformation of a speaker’s vocal characteristics to resemble another while preserving the linguistic content. This technology has broad applications, including automated movie dubbing, speech-to-singing conversion, and assistive devices for pathological speech rehabilitation. With the increasing demand for high-quality and natural-sounding synthetic voices, researchers have developed a wide range of VC techniques. Among these, generative adversarial network (GAN)-based approaches have drawn considerable attention for their powerful feature-mapping capabilities and potential to produce highly realistic speech. Despite notable advancements, challenges such as ensuring training stability, maintaining linguistic consistency, and achieving perceptual naturalness continue to hinder progress in GAN-based VC systems. This systematic review presents a comprehensive analysis of the voice conversion landscape, highlighting key techniques, key challenges, and the transformative impact of GANs in the field. The survey categorizes existing methods, examines technical obstacles, and critically evaluates recent developments in GAN-based VC. By consolidating and synthesizing research findings scattered across the literature, this review provides a structured understanding of the strengths and limitations of different approaches. The significance of this survey lies in its ability to guide future research by identifying existing gaps, proposing potential directions, and offering insights for building more robust and efficient VC systems. Overall, this work serves as an essential resource for researchers, developers, and practitioners aiming to advance the state-of-the-art (SOTA) in voice conversion technology.
语音转换(VC)是语音合成中的一个重要研究领域,它能够使一个发言人的语音特征转变为类似其他人的语音特征,同时保留语言内容。这项技术在许多领域都有广泛的应用,包括电影自动配音、语音转歌唱转换以及病理性语音康复的辅助设备。随着对高质量和自然声音合成语音的日益需求,研究人员已经开发出了多种VC技术。在这些技术中,基于生成对抗网络(GAN)的方法由于其强大的特征映射能力和产生高度逼真的语音的潜力而引起了极大的关注。尽管取得了显著的进展,但确保训练稳定性、保持语言一致性和实现感知自然性的挑战仍然阻碍着基于GAN的VC系统的进步。这篇系统评价对语音转换领域进行了全面的分析,强调了关键技术、主要挑战以及GAN在该领域的变革性影响。该调查对现有方法进行了分类,研究了技术障碍,并对基于GAN的VC的最新发展进行了批判性评价。通过整合和合成散见于文献中的研究成果,本评价提供了对不同方法优缺点结构化的理解。本调查的的重要性在于,通过识别现有差距、提出潜在方向以及为构建更稳健和高效的VC系统提供见解,从而指导未来的研究。总体而言,对于旨在推动语音转换技术最新研究状态的研究人员、开发人员和实践者来说,这项工作是一项必不可少的资源。
论文及项目相关链接
PDF 19 pages, 12 figures, 1 table
Summary
文本综述详细介绍了语音转换领域的关键技术、挑战以及GAN在该领域的变革性影响。通过对现有方法进行分类、探讨技术障碍以及对基于GAN的VC最新发展进行批判性评价,该综述为研究者、开发者和实践者提供了关于构建更稳健、高效的语音转换系统的见解和方向。
Key Takeaways
- 语音转换(VC)是语音合成领域的重要研究方向,能够改变说话人的语音特征,使其类似于另一个人同时保留语言内容。
- VC技术具有广泛的应用,包括自动电影配音、语音到唱歌的转换以及病理性语音康复的辅助设备。
- 随着对高质量、自然听起来合成语音的需求增加,研究者已经开发了许多VC技术。
- 基于生成对抗网络(GAN)的方法已经吸引了人们的广泛关注,因其强大的特征映射能力和产生高度现实语音的潜力。
- GAN-based VC系统面临的挑战包括确保训练稳定性、保持语言一致性和实现感知自然性。
- 文本综述对VC领域进行了综合分析,强调了关键技术、挑战以及GAN在该领域的变革性影响。
点此查看论文截图
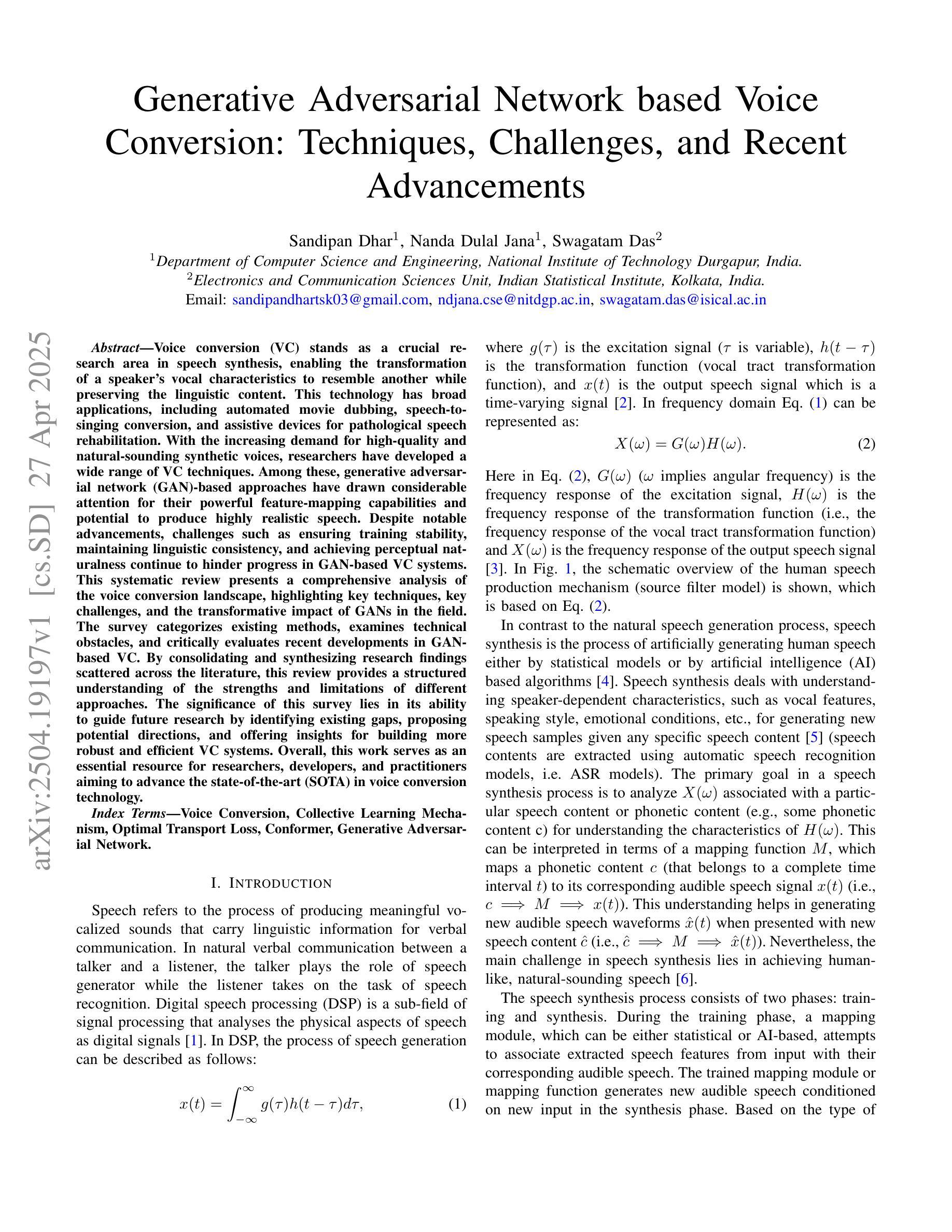
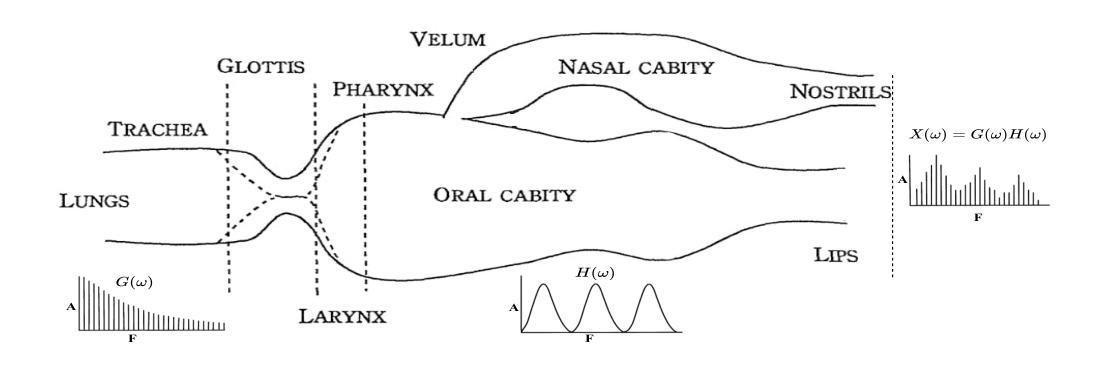
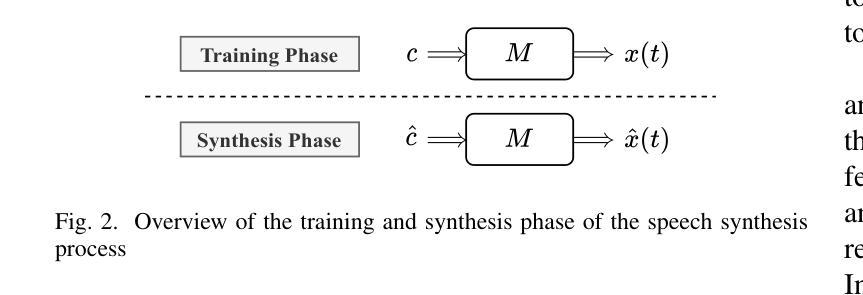
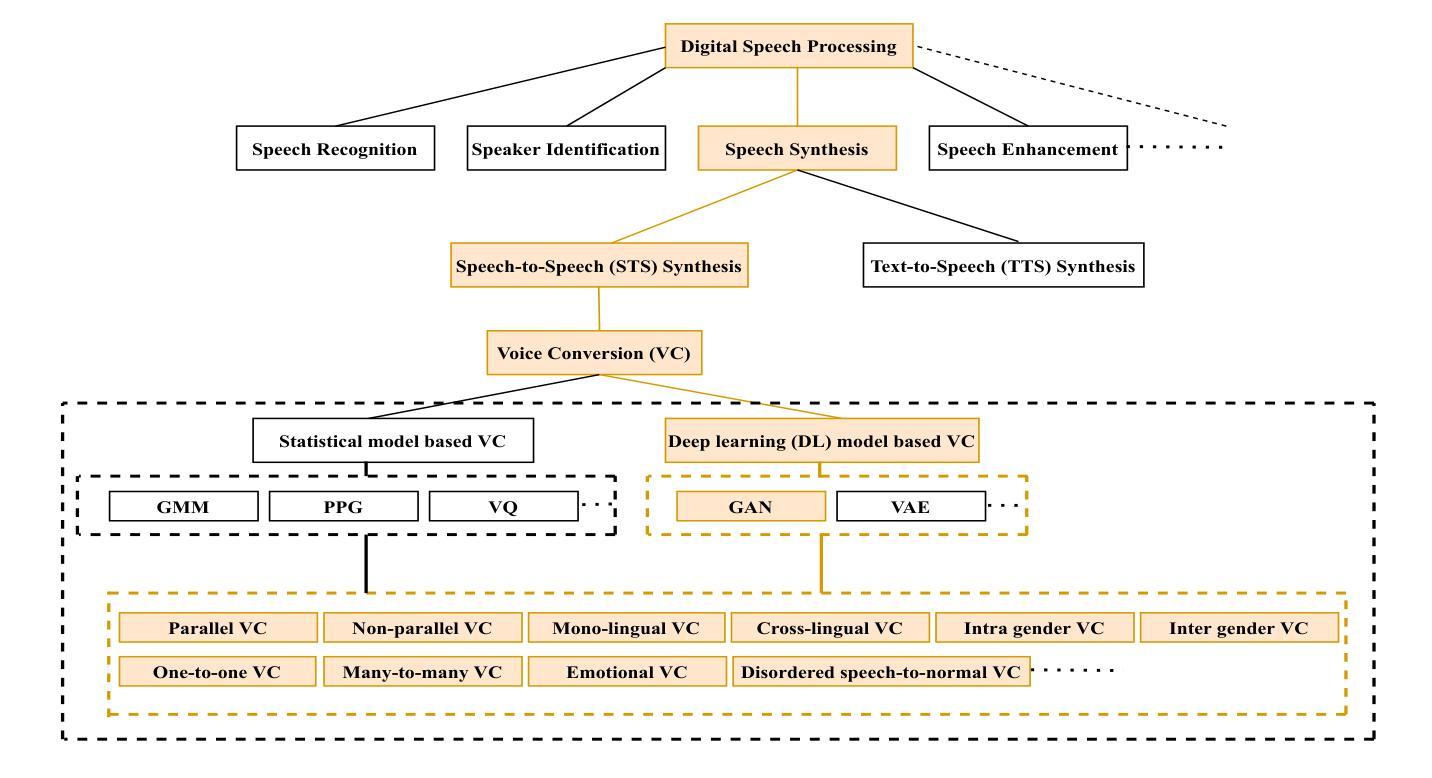
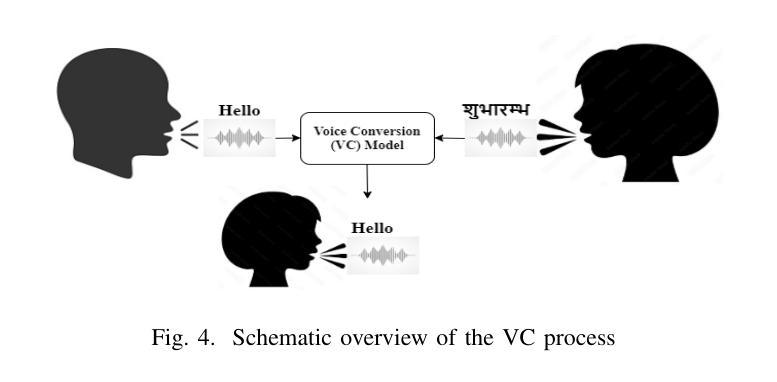
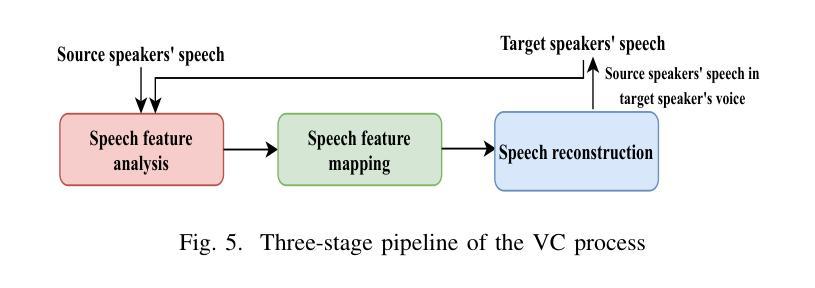
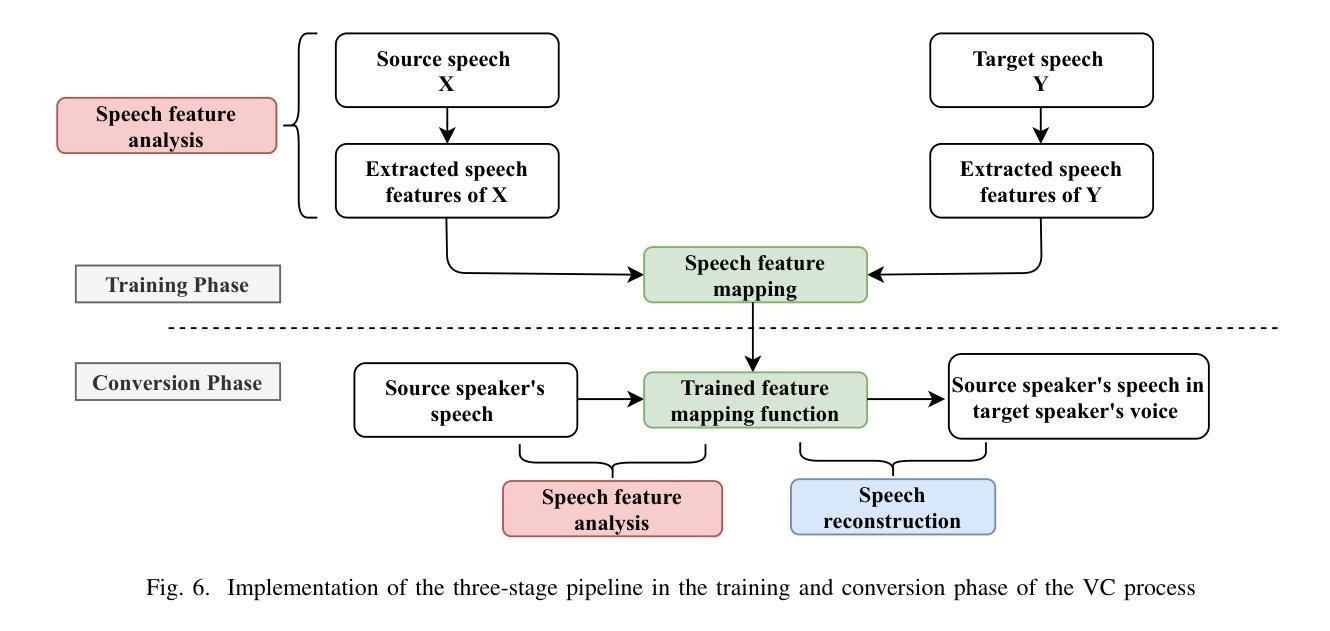
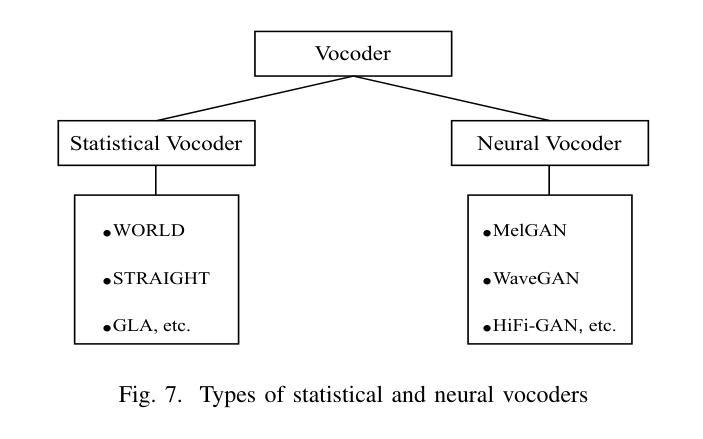
Muyan-TTS: A Trainable Text-to-Speech Model Optimized for Podcast Scenarios with a $50K Budget
Authors:Xin Li, Kaikai Jia, Hao Sun, Jun Dai, Ziyang Jiang
Recent advancements in text-to-speech (TTS) models have been driven by the integration of large language models (LLMs), enhancing semantic comprehension and improving speech naturalness. However, existing LLM-based TTS models often lack open-source training code and efficient inference acceleration frameworks, limiting their accessibility and adaptability. Additionally, there is no publicly available TTS model specifically optimized for podcast scenarios, which are in high demand for voice interaction applications. To address these limitations, we introduce Muyan-TTS, an open-source trainable TTS model designed for podcast applications within a $50,000 budget. Our model is pre-trained on over 100,000 hours of podcast audio data, enabling zero-shot TTS synthesis with high-quality voice generation. Furthermore, Muyan-TTS supports speaker adaptation with dozens of minutes of target speech, making it highly customizable for individual voices. In addition to open-sourcing the model, we provide a comprehensive data collection and processing pipeline, a full training procedure, and an optimized inference framework that accelerates LLM-based TTS synthesis. Our code and models are available at https://github.com/MYZY-AI/Muyan-TTS.
近年来,文本到语音(TTS)模型的进步得益于大型语言模型(LLM)的集成,这提高了语义理解和语音自然度。然而,基于LLM的现有TTS模型通常缺乏开源训练代码和高效的推理加速框架,这限制了它们的可访问性和适应性。此外,没有针对广播情景进行优化的公开可用的TTS模型,而语音交互应用程序对广播情景的需求很高。为了解决这些限制,我们推出了Muyan-TTS,这是一个开源的可训练TTS模型,旨在在5万美元的预算内用于广播应用程序。我们的模型在超过10万小时的广播音频数据上进行预训练,能够实现零样本TTS合成和高质量语音生成。此外,Muyan-TTS支持使用几十分钟的目标语音进行演讲者适应,使其能够轻松定制个人声音。除了开源模型外,我们还提供了全面的数据收集和处理流程、完整的训练过程以及优化的推理框架,以加速基于LLM的TTS合成。我们的代码和模型可在https://github.com/MYZY-AI/Muyan-TTS找到。
论文及项目相关链接
Summary
Muyan-TTS是一个针对播客应用场景的开源可训练文本转语音(TTS)模型,该模型基于大型语言模型(LLM),拥有高语音质量和高度可定制性。它解决了现有TTS模型缺乏开源训练代码和高效推理加速框架的问题,并具有低成本、高质量的语音生成能力。模型预训练在超过百万小时的播客音频数据上进行,支持零样本合成和说话人自适应。更多信息可通过访问GitHub仓库了解。
Key Takeaways
- Muyan-TTS是一个针对播客场景的开源TTS模型,解决了现有模型在开放源代码和推理效率方面的不足。
- Muyan-TTS是基于大型语言模型的先进架构构建,具有高质量的语音生成能力。
- 该模型经过百万小时播客音频数据的预训练,可以实现零样本合成(zero-shot TTS synthesis)。
- Muyan-TTS支持说话人自适应,允许通过几十分钟的语音数据定制个性化声音。
- 模型提供了完整的数据收集和处理流程、训练过程以及优化的推理框架。
- 模型和代码在GitHub上公开可用,便于开发者访问和使用。
点此查看论文截图
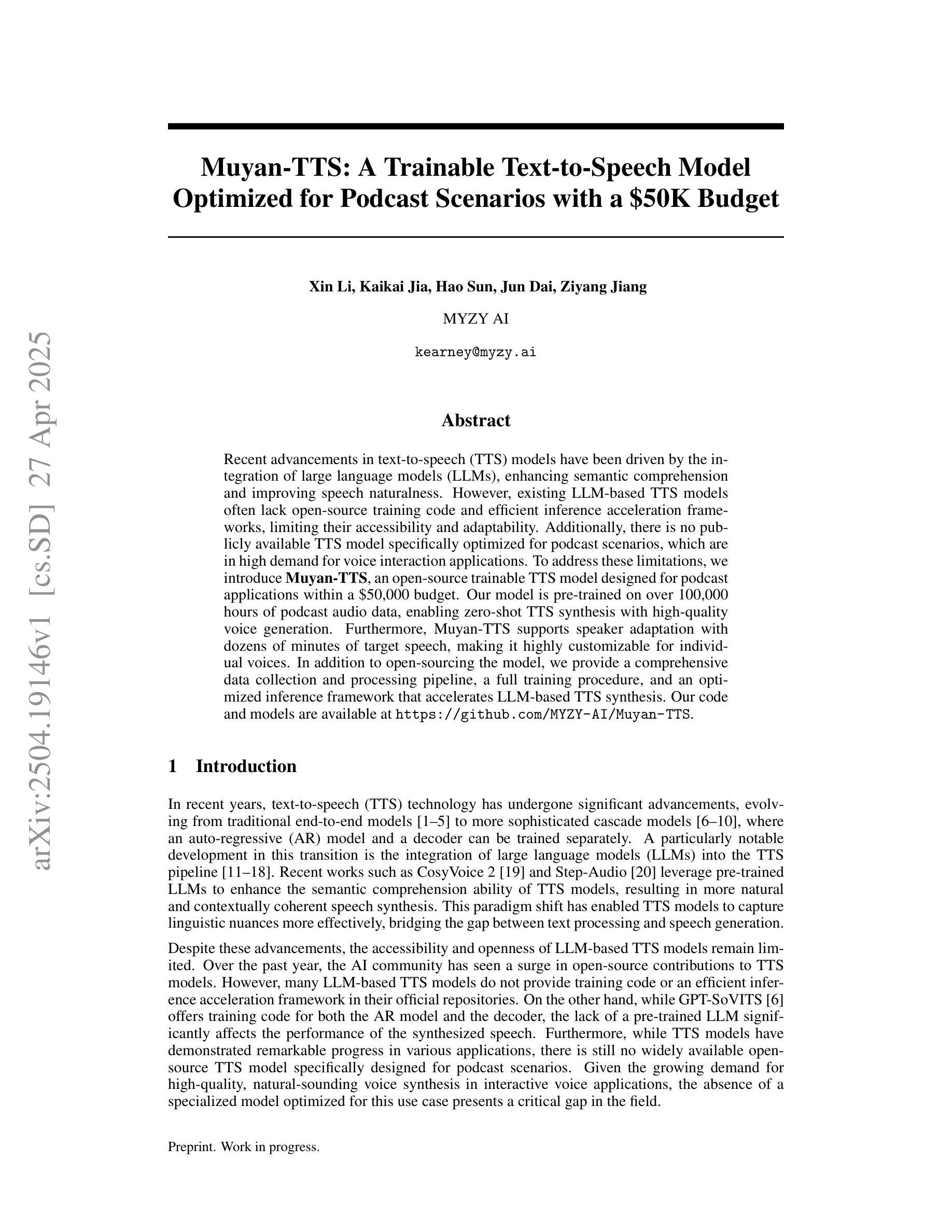
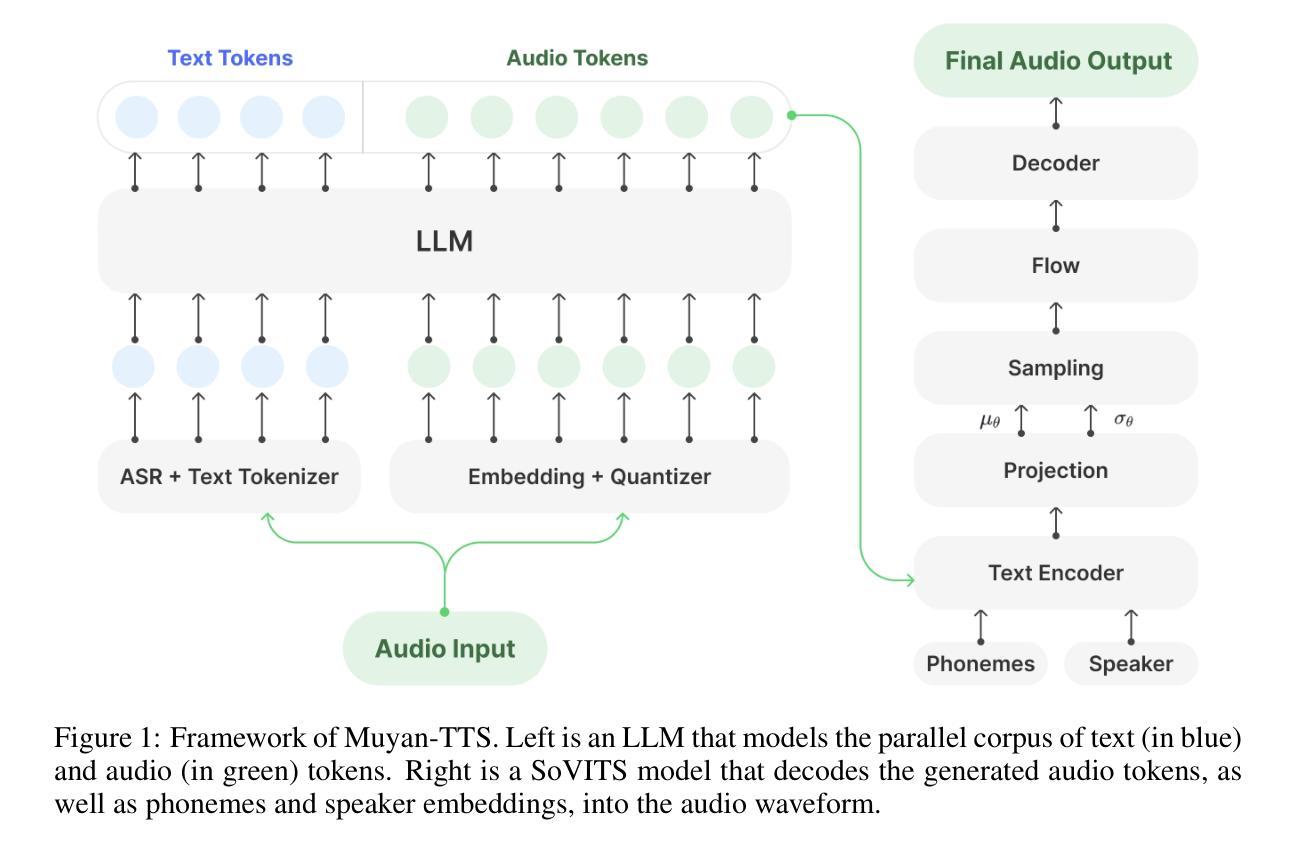
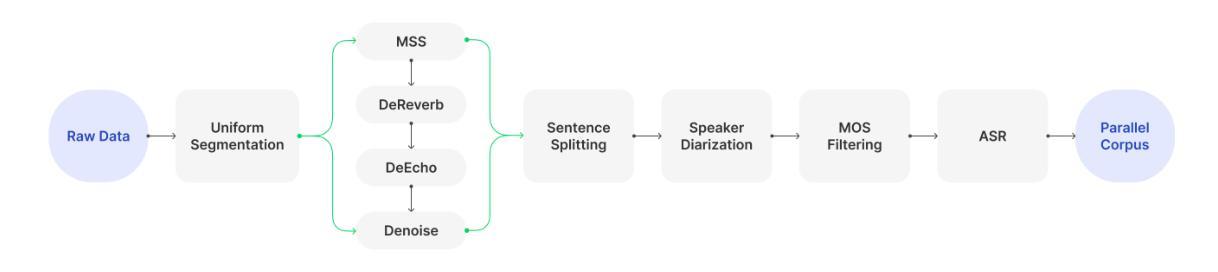

MLAAD: The Multi-Language Audio Anti-Spoofing Dataset
Authors:Nicolas M. Müller, Piotr Kawa, Wei Herng Choong, Edresson Casanova, Eren Gölge, Thorsten Müller, Piotr Syga, Philip Sperl, Konstantin Böttinger
Text-to-Speech (TTS) technology offers notable benefits, such as providing a voice for individuals with speech impairments, but it also facilitates the creation of audio deepfakes and spoofing attacks. AI-based detection methods can help mitigate these risks; however, the performance of such models is inherently dependent on the quality and diversity of their training data. Presently, the available datasets are heavily skewed towards English and Chinese audio, which limits the global applicability of these anti-spoofing systems. To address this limitation, this paper presents the Multi-Language Audio Anti-Spoofing Dataset (MLAAD), created using 91 TTS models, comprising 42 different architectures, to generate 420.7 hours of synthetic voice in 38 different languages. We train and evaluate three state-of-the-art deepfake detection models with MLAAD and observe that it demonstrates superior performance over comparable datasets like InTheWild and Fake-Or-Real when used as a training resource. Moreover, compared to the renowned ASVspoof 2019 dataset, MLAAD proves to be a complementary resource. In tests across eight datasets, MLAAD and ASVspoof 2019 alternately outperformed each other, each excelling on four datasets. By publishing MLAAD and making a trained model accessible via an interactive webserver, we aim to democratize anti-spoofing technology, making it accessible beyond the realm of specialists, and contributing to global efforts against audio spoofing and deepfakes.
文本转语音(TTS)技术提供了显著的益处,例如为言语障碍者提供声音,但它也促进了音频深度伪造和欺骗攻击的产生。基于人工智能的检测方法可以有助于减轻这些风险;然而,此类模型的性能本质上取决于其训练数据的质量和多样性。目前,可用的数据集严重偏向于英语和中文音频,这限制了这些防欺骗系统的全球适用性。为了解决这一局限性,本文提出了多语言音频防欺骗数据集(MLAAD),使用91个TTS模型创建,包含42种不同架构,生成38种不同语言的420.7小时合成语音。我们使用MLAAD训练并评估了三种最先进的深度伪造检测模型,并观察到,当用作训练资源时,它在InTheWild和Fake-Or-Real等可比数据集上表现出卓越的性能。此外,与著名的ASVspoof 2019数据集相比,MLAAD证明是一种补充资源。在八个数据集的测试中,MLAAD和ASVspoof 2019交替表现出优势,各自在四个数据集上表现出色。我们发布MLAAD,并通过交互式网站提供经过训练的模型,旨在使防欺骗技术民主化,使其超越专家领域变得可访问,并为全球反对音频欺骗和深度伪造的努力做出贡献。
论文及项目相关链接
PDF IJCNN 2024
Summary
文本转语音(TTS)技术虽有助于解决语言障碍等问题,但也带来了音频深度伪造和欺骗性攻击的风险。人工智能检测方法是缓解这些风险的有效手段,但模型性能受限于训练数据的质量和多样性。现有的数据集主要偏向英语和中文音频,限制了反欺骗系统的全球适用性。为解决此问题,本文提出多语言音频反欺骗数据集(MLAAD),利用91种TTS模型生成包含多种语言和架构的420.7小时合成语音。使用MLAAD训练并评估先进深度伪造检测模型,显示其在类似InTheWild和Fake-Or-Real数据集上的优越性能。此外,与著名的ASVspoof 2019数据集相比,MLAAD是一个互补资源。通过发布MLAAD并通过交互式网站提供训练模型,我们旨在普及反欺骗技术,使其不仅限于专家使用,并为全球打击音频欺骗和深度伪造做出贡献。
Key Takeaways
- TTS技术虽有益,但也存在音频深度伪造和欺骗性攻击的风险。
- AI检测方法是缓解TTS风险的关键,但模型性能受训练数据限制。
- 现有数据集主要偏向英语和中文,限制了反欺骗系统的全球应用。
- MLAAD数据集利用多种语言和TTS模型生成合成语音,以扩展反欺骗技术的适用范围。
- MLAAD在多个数据集上的性能优于其他类似数据集,如InTheWild和Fake-Or-Real。
- MLAAD与ASVspoof 2019数据集相互补充,可在不同数据集上交替表现出优越性能。
点此查看论文截图
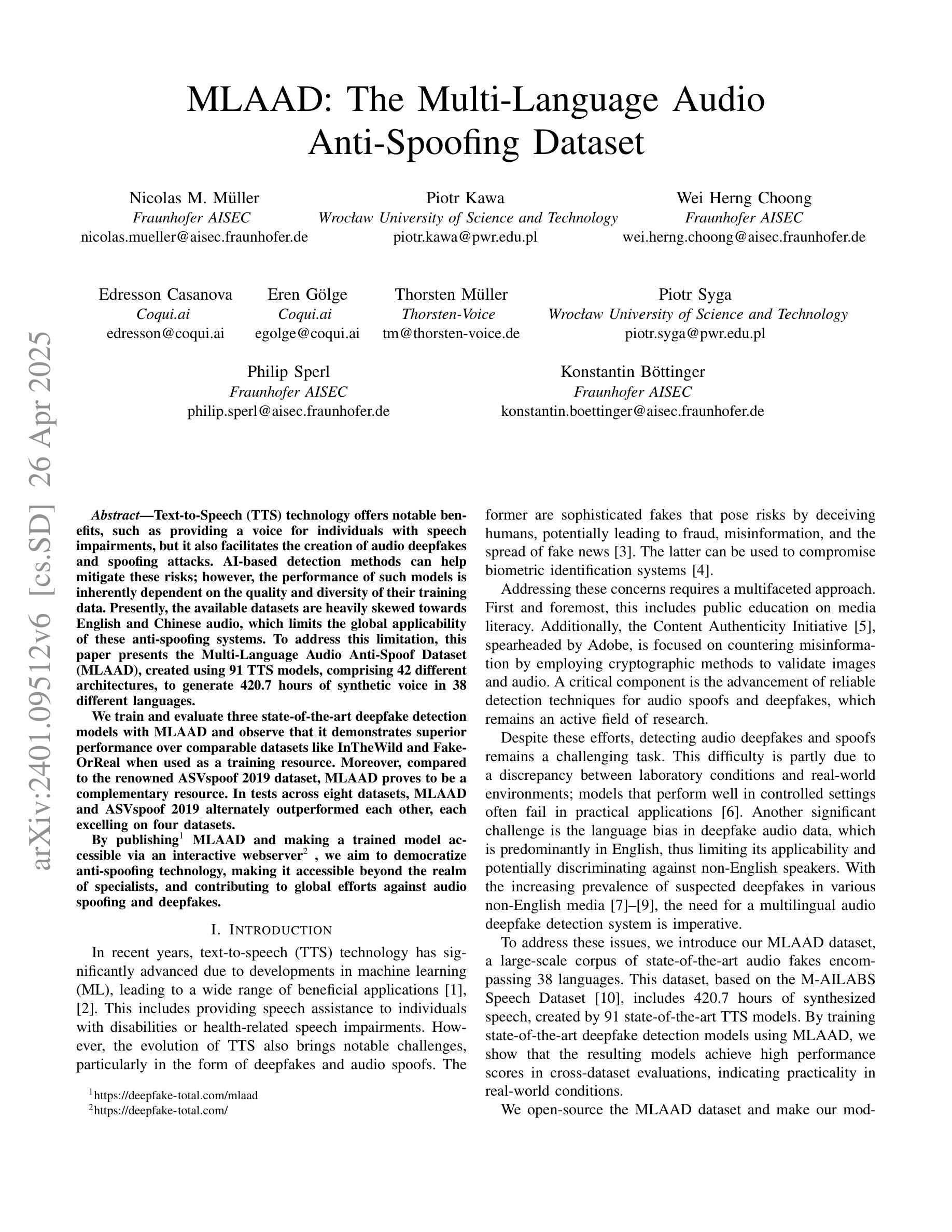
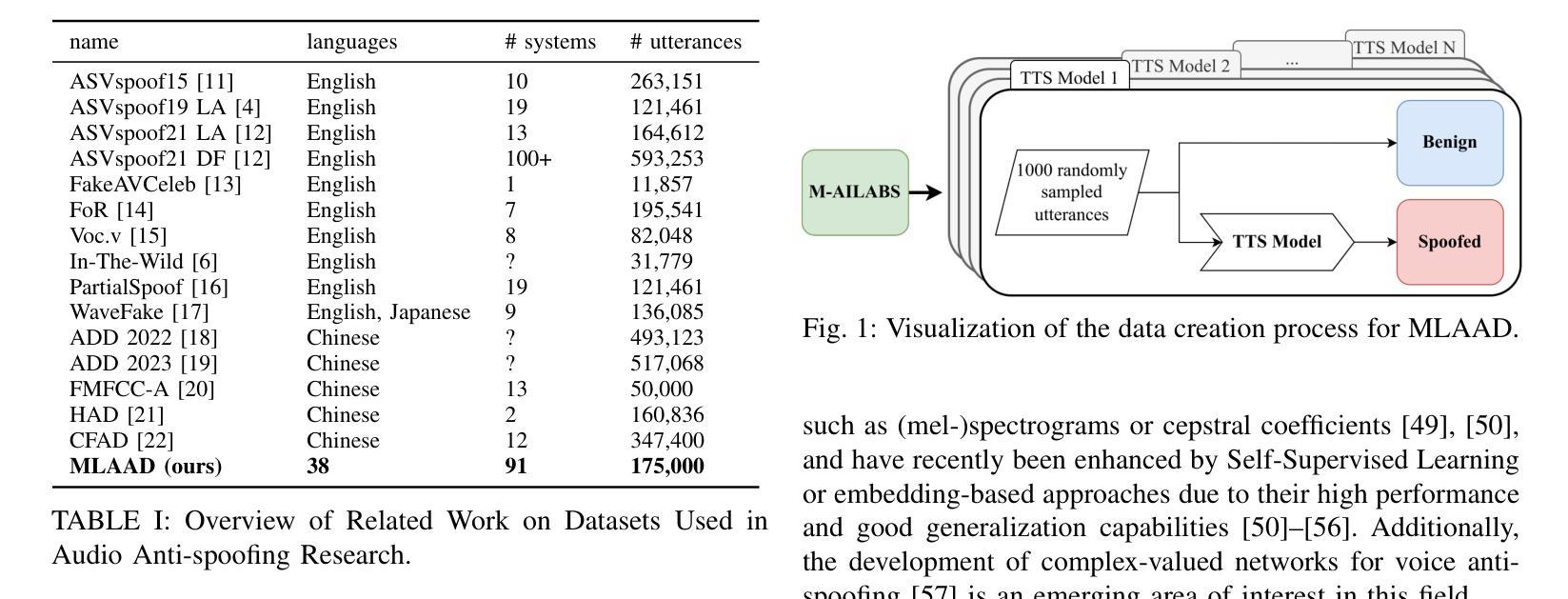
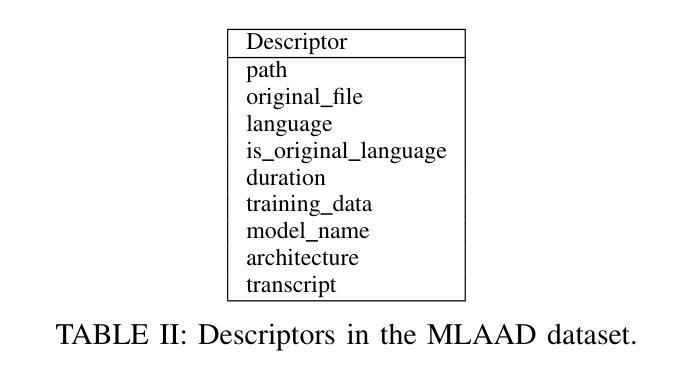
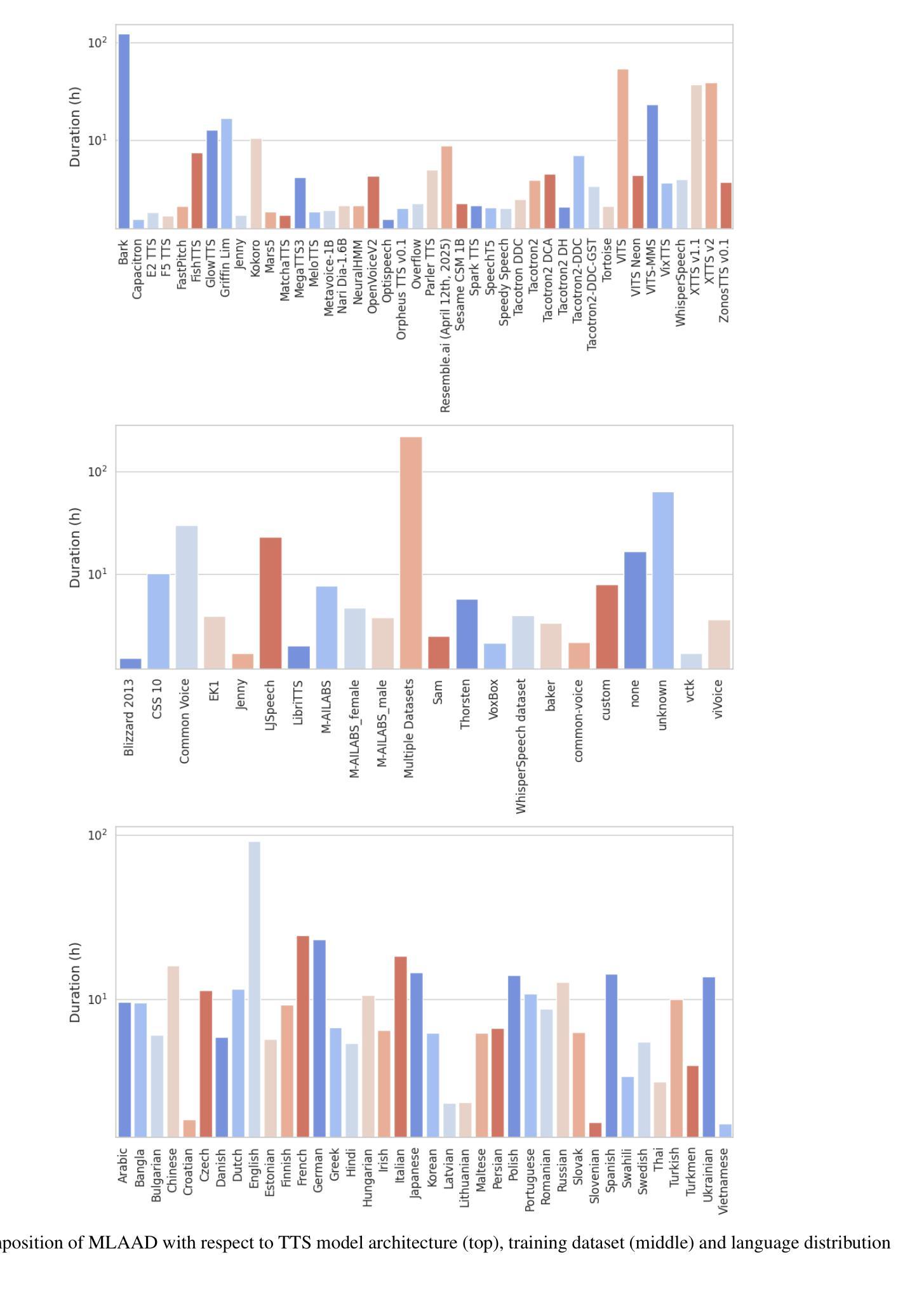
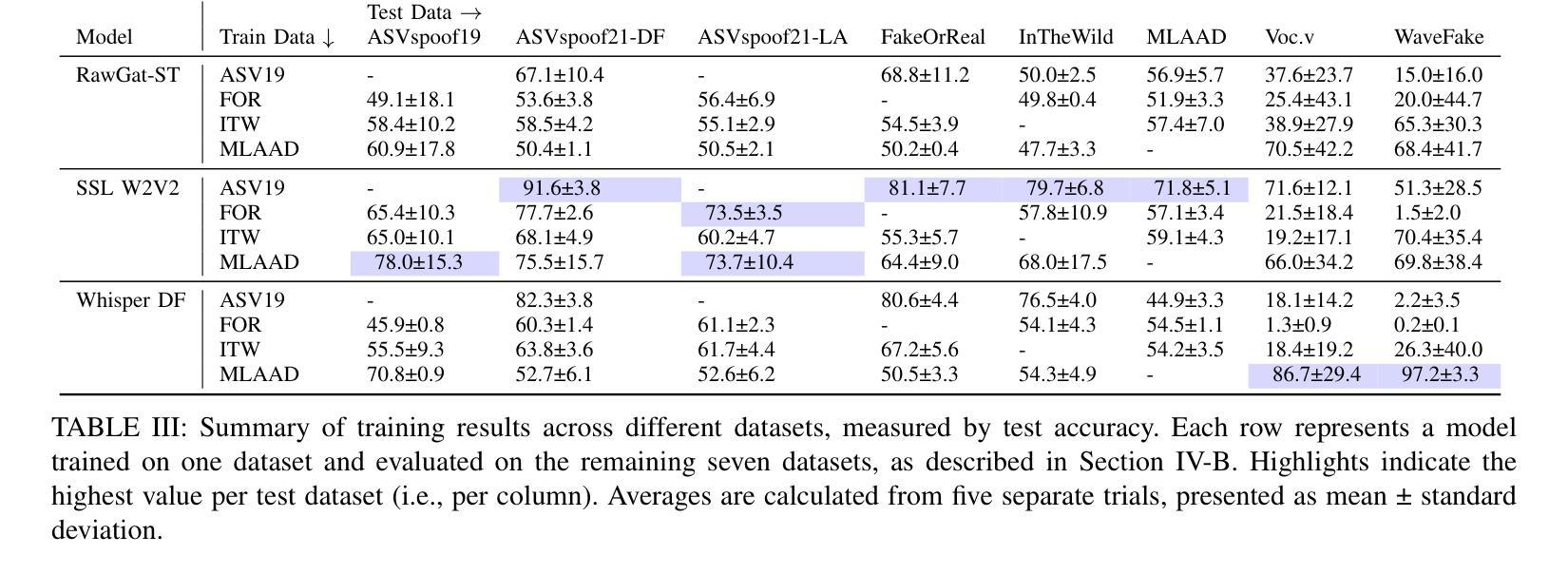
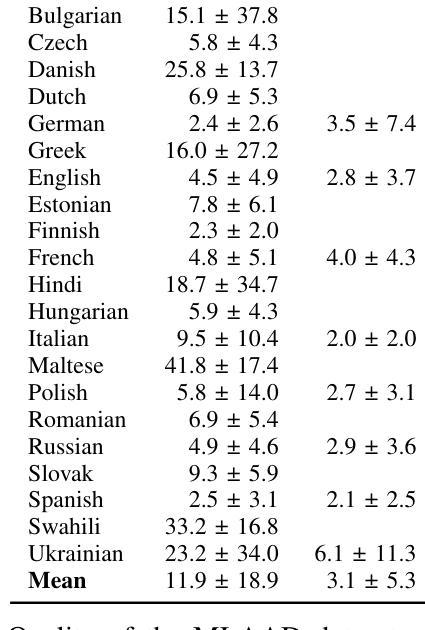