⚠️ 以下所有内容总结都来自于 大语言模型的能力,如有错误,仅供参考,谨慎使用
🔴 请注意:千万不要用于严肃的学术场景,只能用于论文阅读前的初筛!
💗 如果您觉得我们的项目对您有帮助 ChatPaperFree ,还请您给我们一些鼓励!⭐️ HuggingFace免费体验
2025-04-30 更新
Generative Adversarial Network based Voice Conversion: Techniques, Challenges, and Recent Advancements
Authors:Sandipan Dhar, Nanda Dulal Jana, Swagatam Das
Voice conversion (VC) stands as a crucial research area in speech synthesis, enabling the transformation of a speaker’s vocal characteristics to resemble another while preserving the linguistic content. This technology has broad applications, including automated movie dubbing, speech-to-singing conversion, and assistive devices for pathological speech rehabilitation. With the increasing demand for high-quality and natural-sounding synthetic voices, researchers have developed a wide range of VC techniques. Among these, generative adversarial network (GAN)-based approaches have drawn considerable attention for their powerful feature-mapping capabilities and potential to produce highly realistic speech. Despite notable advancements, challenges such as ensuring training stability, maintaining linguistic consistency, and achieving perceptual naturalness continue to hinder progress in GAN-based VC systems. This systematic review presents a comprehensive analysis of the voice conversion landscape, highlighting key techniques, key challenges, and the transformative impact of GANs in the field. The survey categorizes existing methods, examines technical obstacles, and critically evaluates recent developments in GAN-based VC. By consolidating and synthesizing research findings scattered across the literature, this review provides a structured understanding of the strengths and limitations of different approaches. The significance of this survey lies in its ability to guide future research by identifying existing gaps, proposing potential directions, and offering insights for building more robust and efficient VC systems. Overall, this work serves as an essential resource for researchers, developers, and practitioners aiming to advance the state-of-the-art (SOTA) in voice conversion technology.
语音转换(VC)是语音合成领域的一个重要研究方向,它能够使一个说话者的发音特征转变为另一个人的发音特征,同时保留语言内容。这项技术在多个领域都有广泛的应用,包括电影自动配音、歌曲合成及病态性言语恢复辅助装置等。随着高质量的自然语音合成声音的需求日益增长,研究人员已经开发出了多种VC技术。在这些技术中,基于生成对抗网络(GAN)的方法受到了广泛关注,因为它们具有强大的特征映射能力和产生高度逼真的语音的潜力。尽管已经取得了明显的进步,但确保训练稳定性、保持语言连贯性和实现感知自然性等挑战仍然阻碍着基于GAN的VC系统的进步。这篇综述对语音转换领域进行了全面的分析,重点介绍了关键技术、主要挑战以及GAN在该领域的变革性影响。本文对现有方法进行分类,探讨技术障碍,并对基于GAN的VC的最新发展进行了评价。通过整合文献中零散的研究成果,本综述对不同的方法的优缺点进行了结构化的理解。这篇综述的重要性在于,它能够通过识别现有差距、提出潜在方向以及为构建更强大、更有效的VC系统提供见解来指导未来的研究。总体而言,本工作对于旨在推动语音转换技术最新进展的研究人员、开发人员和从业者来说是必不可少的资源。
论文及项目相关链接
PDF 19 pages, 12 figures, 1 table
Summary
本文综述了语音转换领域的研究进展,重点介绍了基于生成对抗网络(GAN)的方法在该领域的应用及其重要性。文章详细分析了各种方法的优点和局限性,讨论了存在的挑战和潜在的研究方向,旨在为推进语音转换技术的发展提供指导。
Key Takeaways
- 语音转换(VC)是语音合成领域的重要研究方向,能够改变说话人的语音特征,使其类似于其他人同时保留语言内容。
- GAN在VC中的应用因其强大的特征映射能力和产生高度逼真的语音的潜力而受到广泛关注。
- VC技术面临的主要挑战包括确保训练稳定性、保持语言一致性和实现感知自然性。
- 文章综述了现有的VC方法,并分析了其技术障碍和最新发展。
- 该综述对文献中的研究结果进行了整合和合成,提供了对不同方法的优缺点和局限性的结构化理解。
- 文章强调了此综述的重要性在于其能够指导未来研究,识别现有差距,提出潜在方向,并为构建更强大、高效的VC系统提供见解。
点此查看论文截图
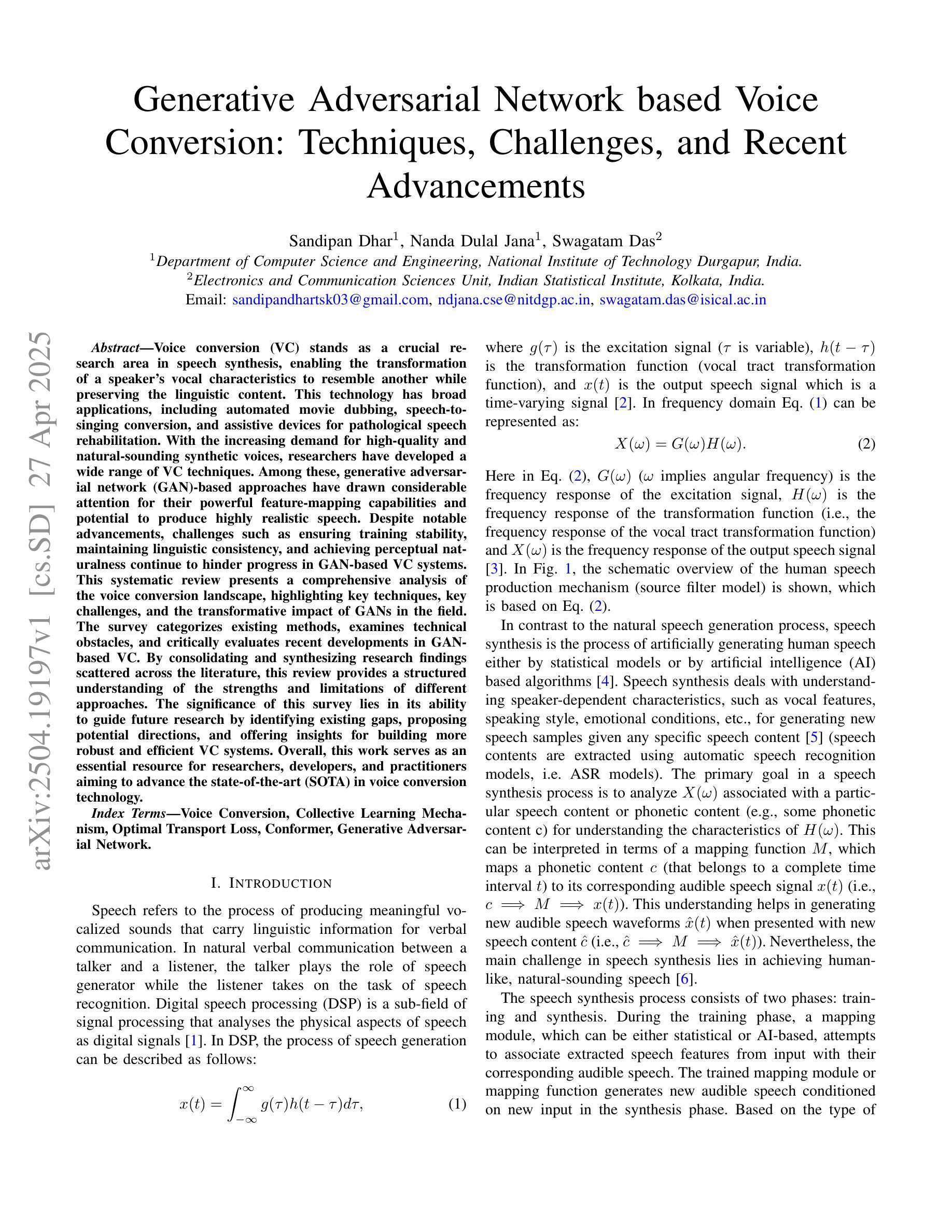
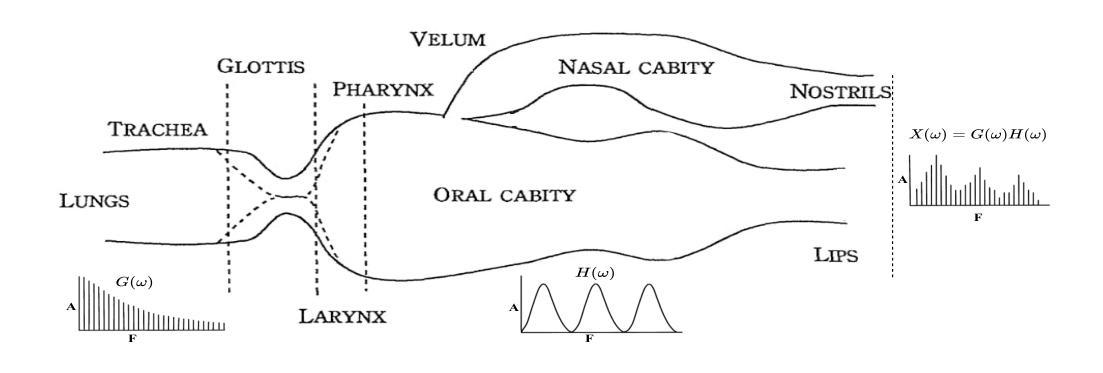
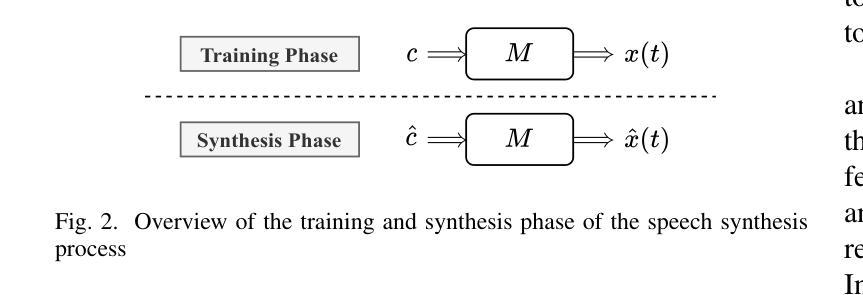
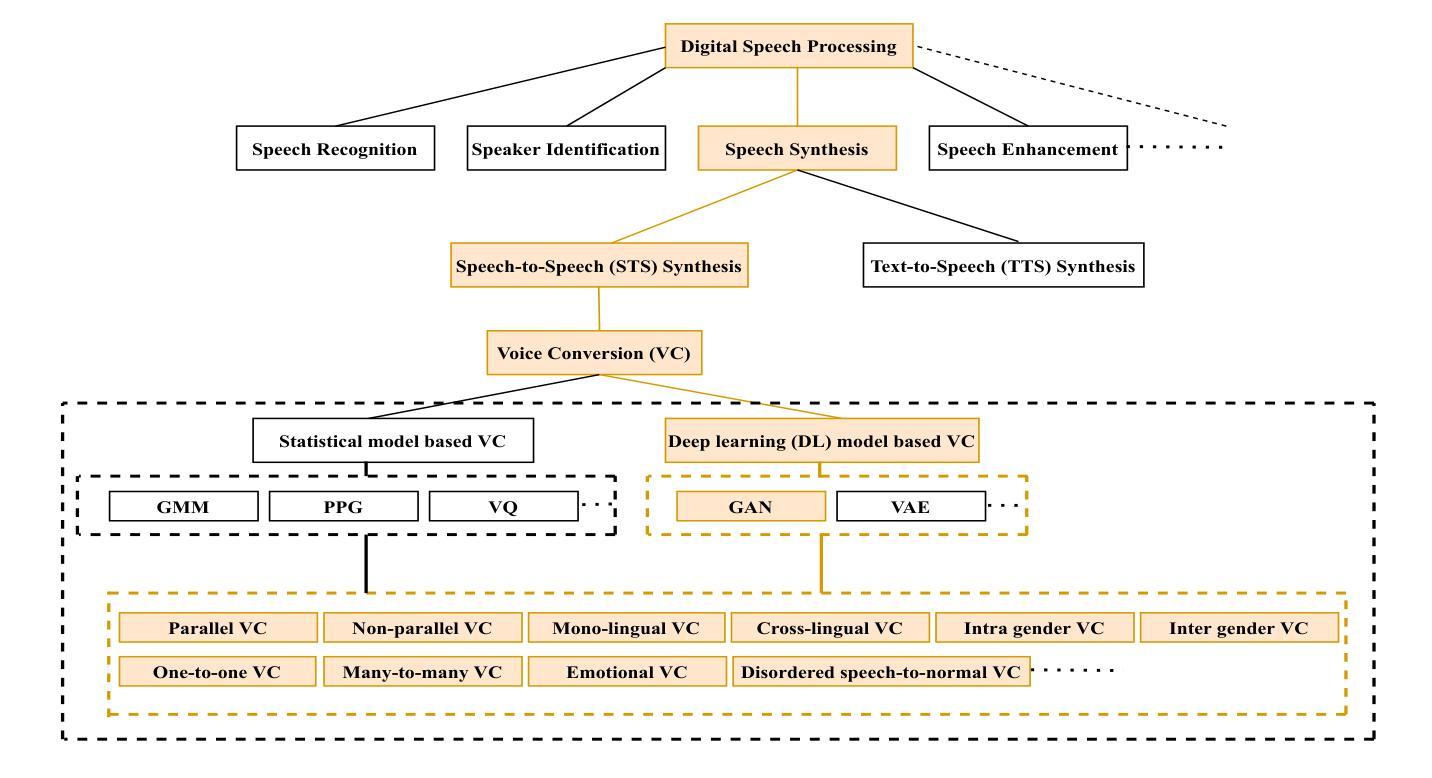
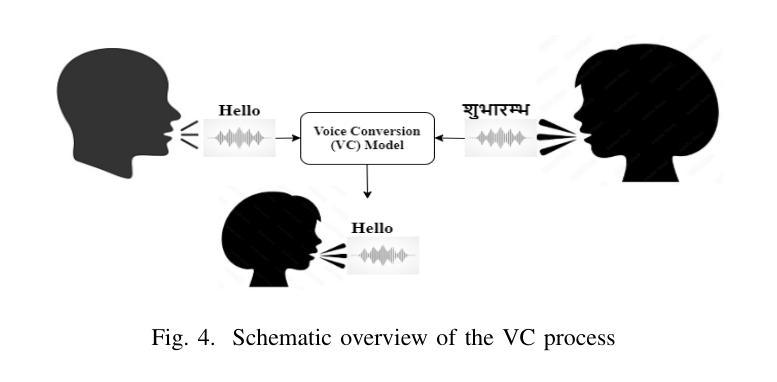
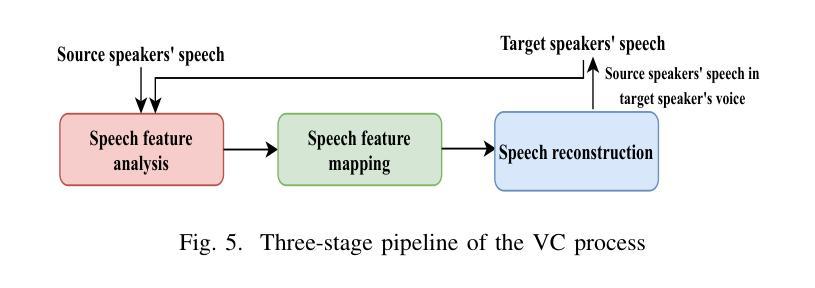
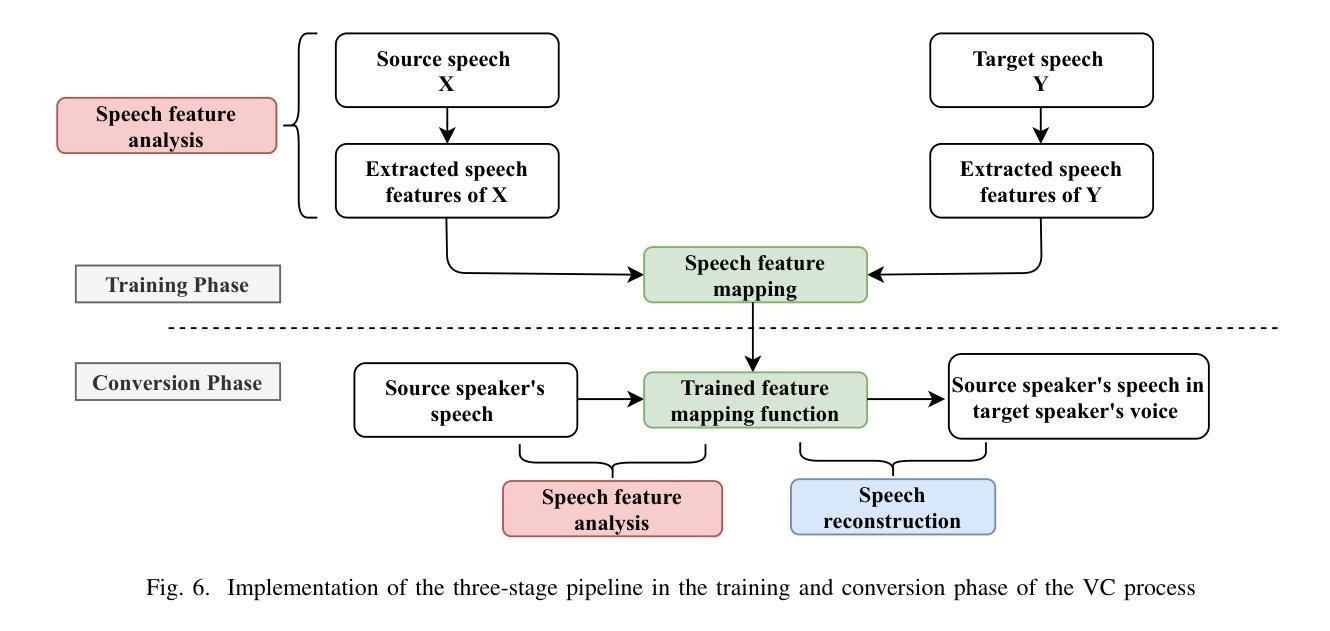
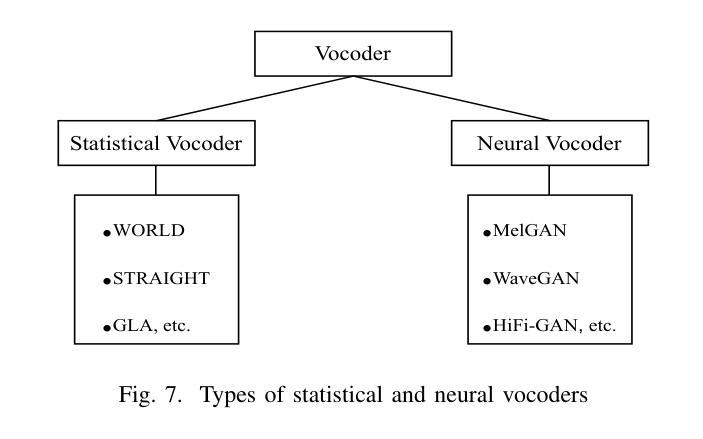
IM-Portrait: Learning 3D-aware Video Diffusion for PhotorealisticTalking Heads from Monocular Videos
Authors:Yuan Li, Ziqian Bai, Feitong Tan, Zhaopeng Cui, Sean Fanello, Yinda Zhang
We propose a novel 3D-aware diffusion-based method for generating photorealistic talking head videos directly from a single identity image and explicit control signals (e.g., expressions). Our method generates Multiplane Images (MPIs) that ensure geometric consistency, making them ideal for immersive viewing experiences like binocular videos for VR headsets. Unlike existing methods that often require a separate stage or joint optimization to reconstruct a 3D representation (such as NeRF or 3D Gaussians), our approach directly generates the final output through a single denoising process, eliminating the need for post-processing steps to render novel views efficiently. To effectively learn from monocular videos, we introduce a training mechanism that reconstructs the output MPI randomly in either the target or the reference camera space. This approach enables the model to simultaneously learn sharp image details and underlying 3D information. Extensive experiments demonstrate the effectiveness of our method, which achieves competitive avatar quality and novel-view rendering capabilities, even without explicit 3D reconstruction or high-quality multi-view training data.
我们提出了一种新型的基于三维感知扩散的方法,直接从单张身份图像和明确的控制信号(如表情)生成逼真的说话头部视频。我们的方法生成多平面图像(MPI),确保几何一致性,使其成为理想的沉浸式观看体验,如虚拟现实头盔的双眼视频。与通常需要单独阶段或联合优化来重建三维表示(如NeRF或三维高斯分布)的现有方法不同,我们的方法通过单个去噪过程直接生成最终输出,无需后处理步骤即可有效地呈现新颖的视角。为了有效地从单目视频中学习,我们引入了一种训练机制,该机制会随机重建目标或参考相机空间中的输出MPI。这种方法使模型能够同时学习清晰的图像细节和潜在的三维信息。大量实验证明了我们方法的有效性,即使在没有任何明确的三维重建或高质量的多视角训练数据的情况下,我们的方法也能达到竞争的化身质量和新颖的视图渲染能力。
论文及项目相关链接
PDF CVPR2025; project page: https://y-u-a-n-l-i.github.io/projects/IM-Portrait/
摘要
本文提出了一种新型的基于三维感知扩散的方法,直接从单张身份图像和明确的控制信号(如表情)生成逼真的讲话视频。该方法生成多平面图像(MPIs),确保几何一致性,使其成为适合虚拟现实头盔等沉浸式观看体验的双眼视频的理想选择。不同于通常需要单独阶段或联合优化来重建三维表示(如NeRF或高斯模型)的现有方法,我们的方法通过一个单一的降噪过程直接生成最终输出,无需后期制作步骤就能高效渲染新的视角。为了有效地从单眼视频中学习,我们引入了一种训练机制,该机制随机重建目标或参考相机空间中的输出MPI。这种方法使模型能够同时学习清晰的图像细节和潜在的三维信息。大量实验证明了我们方法的有效性,该方法在无需明确的三维重建或高质量多视角训练数据的情况下,也能达到竞争对手的形象品质和渲染新视角的能力。
要点解析
- 本文提出了一种创新的基于三维感知扩散的方法生成说话人的逼真视频。
- 通过生成多平面图像确保几何一致性,为虚拟现实等沉浸式体验提供理想选择。
- 与其他方法不同,无需单独阶段或联合优化重建三维表示,直接通过单一降噪过程生成最终输出。
- 训练机制能够在目标或参考相机空间中随机重建输出MPI,使得模型可以同时学习清晰的图像细节和潜在的三维信息。
点此查看论文截图
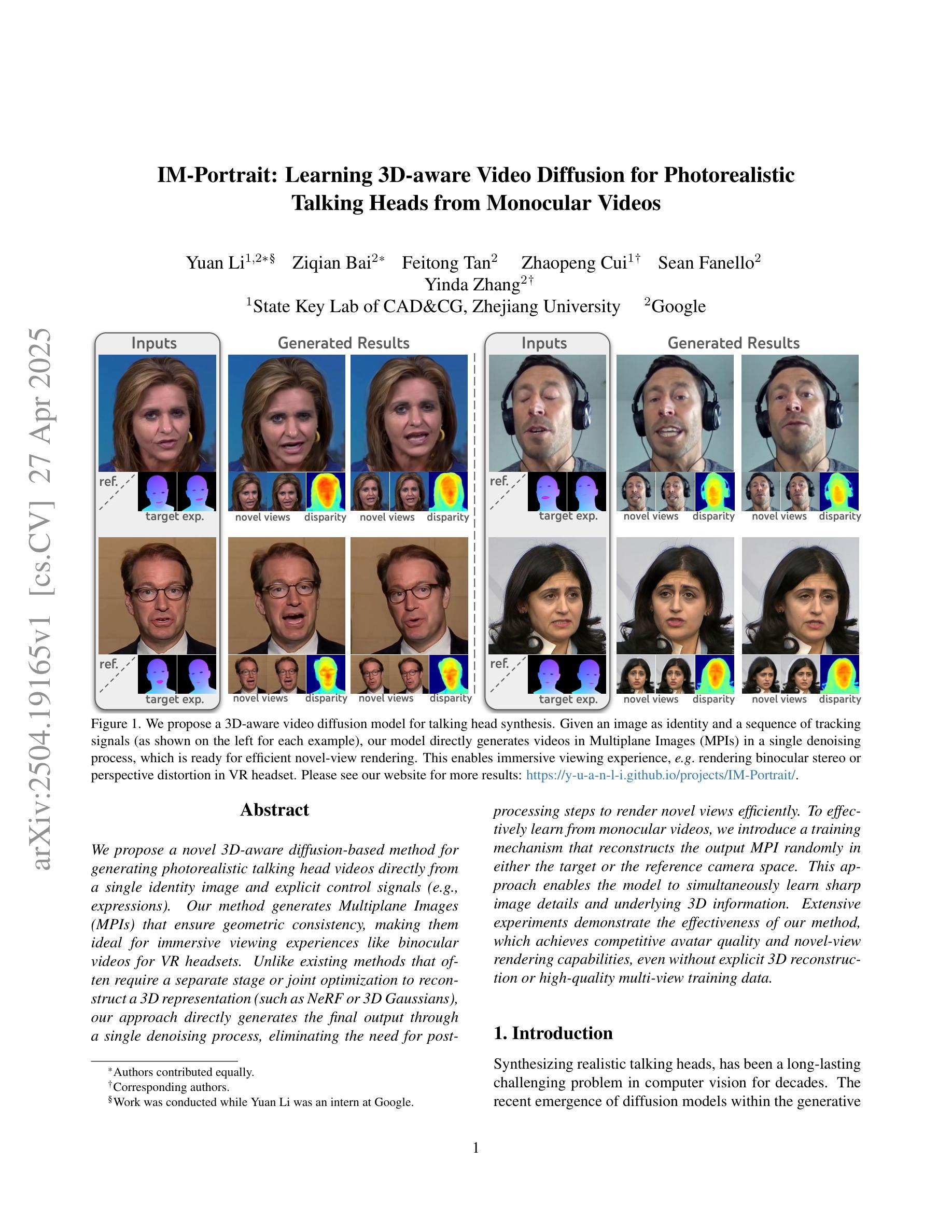
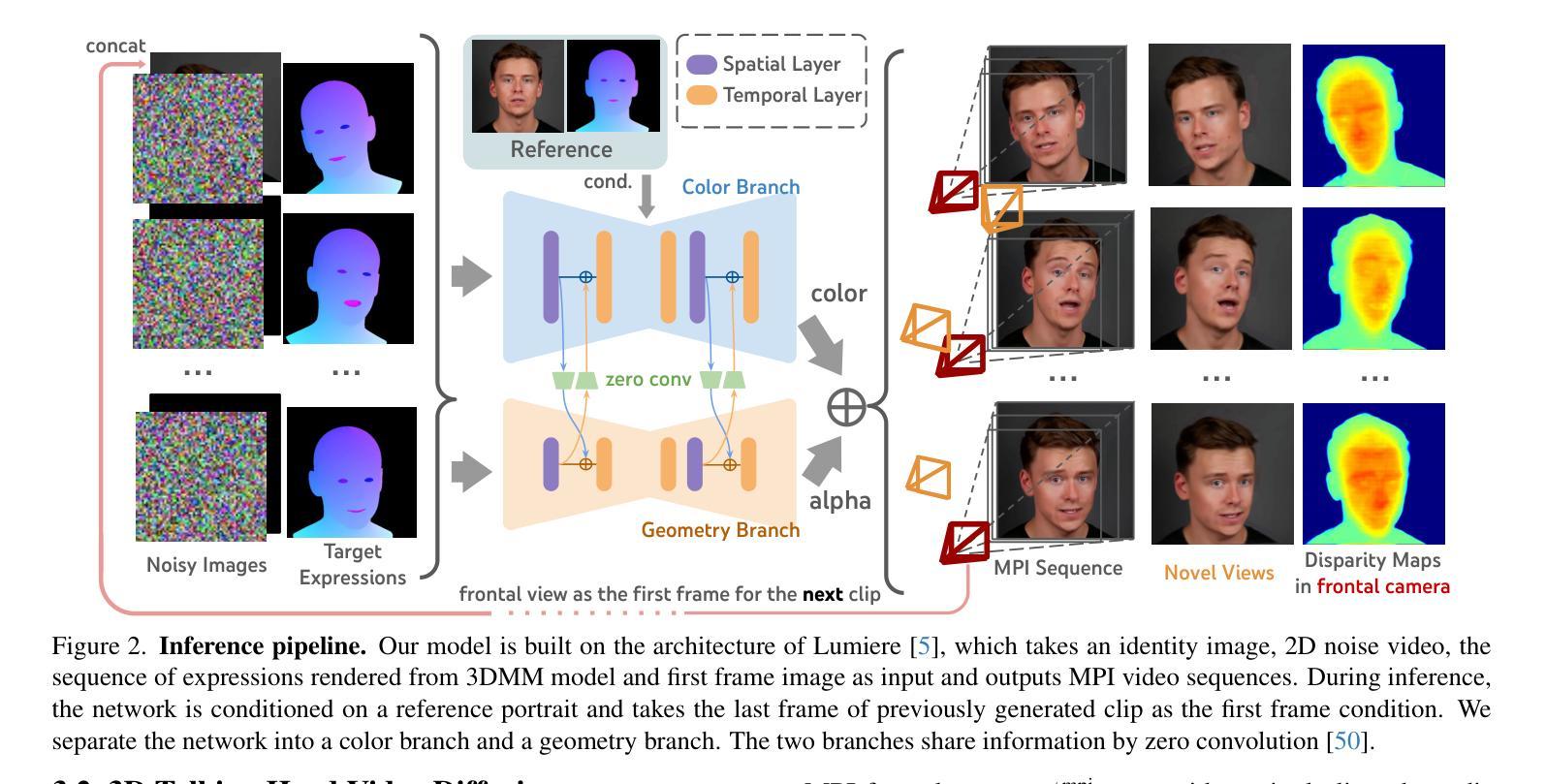
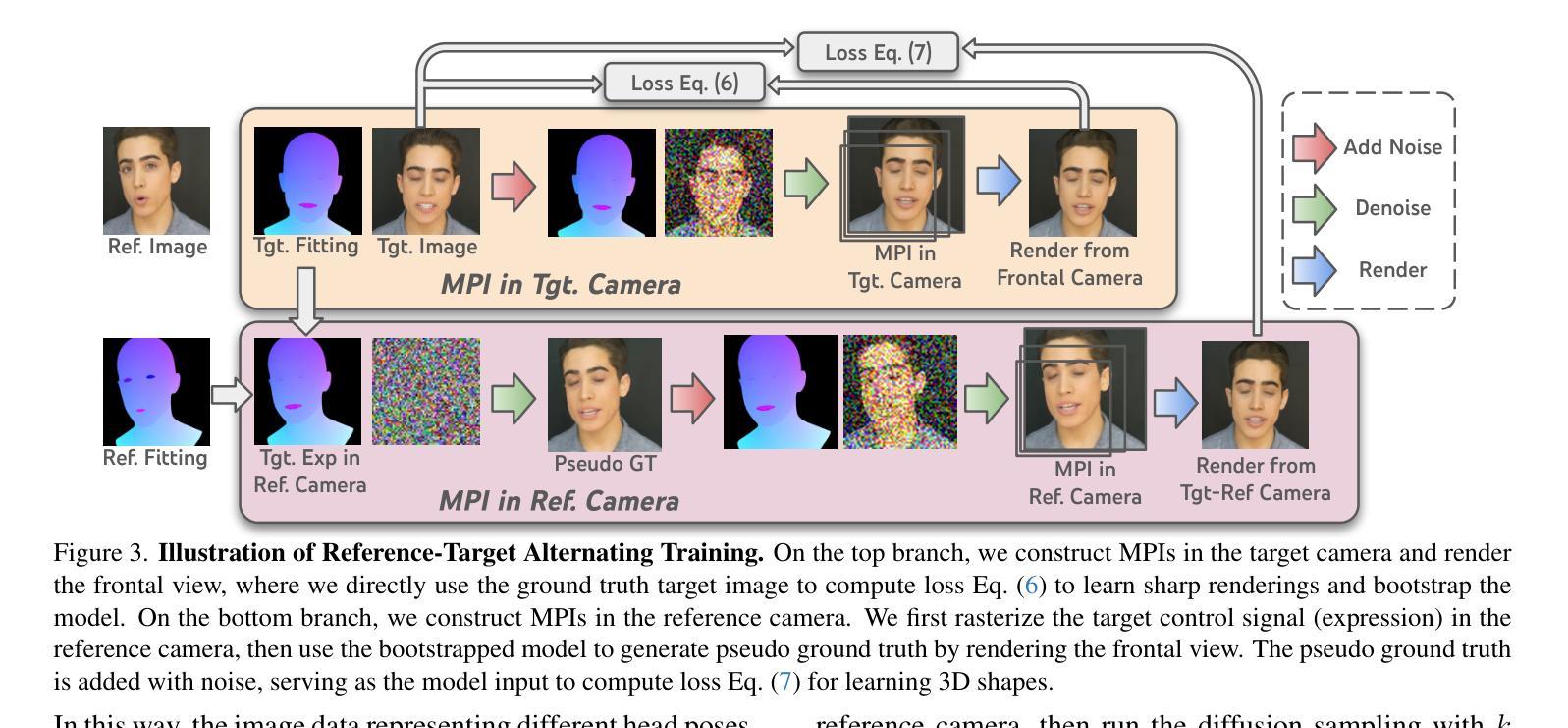
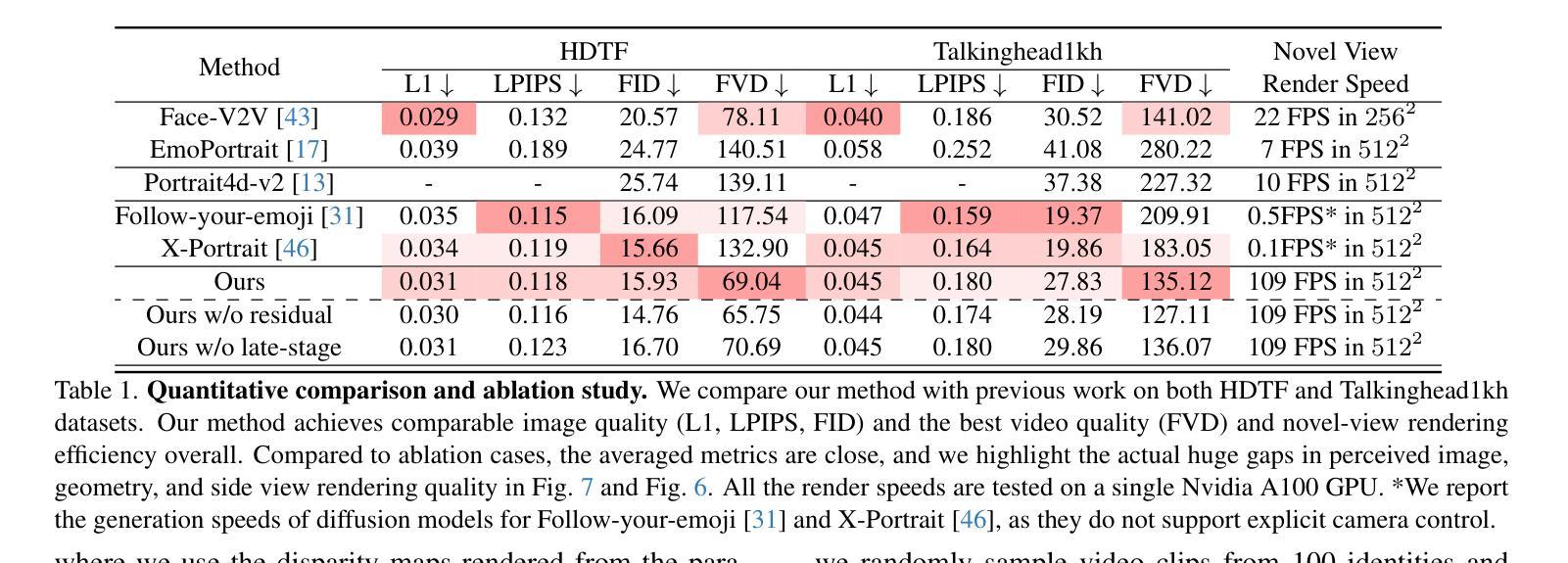