⚠️ 以下所有内容总结都来自于 大语言模型的能力,如有错误,仅供参考,谨慎使用
🔴 请注意:千万不要用于严肃的学术场景,只能用于论文阅读前的初筛!
💗 如果您觉得我们的项目对您有帮助 ChatPaperFree ,还请您给我们一些鼓励!⭐️ HuggingFace免费体验
2025-05-01 更新
Fine Grain Classification: Connecting Meta using Cross-Contrastive pre-training
Authors:Sumit Mamtani, Yash Thesia
Fine-grained visual classification aims to recognize objects belonging to multiple subordinate categories within a super-category. However, this remains a challenging problem, as appearance information alone is often insufficient to accurately differentiate between fine-grained visual categories. To address this, we propose a novel and unified framework that leverages meta-information to assist fine-grained identification. We tackle the joint learning of visual and meta-information through cross-contrastive pre-training. In the first stage, we employ three encoders for images, text, and meta-information, aligning their projected embeddings to achieve better representations. We then fine-tune the image and meta-information encoders for the classification task. Experiments on the NABirds dataset demonstrate that our framework effectively utilizes meta-information to enhance fine-grained recognition performance. With the addition of meta-information, our framework surpasses the current baseline on NABirds by 7.83%. Furthermore, it achieves an accuracy of 84.44% on the NABirds dataset, outperforming many existing state-of-the-art approaches that utilize meta-information.
细粒度视觉分类旨在识别属于超类别内多个子类别的对象。然而,这仍然是一个具有挑战性的问题,因为仅依靠外观信息往往不足以准确区分细粒度视觉类别。为了解决这一问题,我们提出了一个利用元信息辅助细粒度识别的全新统一框架。我们通过跨对比预训练来解决视觉和元信息的联合学习问题。在第一阶段,我们使用三个编码器分别处理图像、文本和元信息,通过对齐它们的投影嵌入来实现更好的表示。然后,我们对图像和元信息编码器进行微调,以完成分类任务。在NABirds数据集上的实验表明,我们的框架有效地利用了元信息来提高细粒度识别性能。通过添加元信息,我们的框架在NABirds上的表现超过了当前基线7.83%。此外,在NABirds数据集上,它达到了84.44%的准确率,超越了许多利用元信息的现有最先进的方法。
论文及项目相关链接
PDF 9 pages, 4 figures. Submitted to arXiv
Summary
该文本提出了一种利用元信息辅助精细粒度识别的统一框架,通过跨对比预训练联合学习视觉和元信息。该框架使用图像、文本和元信息三个编码器,对齐它们的投影嵌入以实现更好的表示,然后对图像和元信息编码器进行微调以进行分类任务。在NABirds数据集上的实验表明,该框架利用元信息有效提高了精细粒度识别的性能,超越了当前基线7.83%,并实现了84.44%的准确率,超越了许多现有的先进方法。
Key Takeaways
- 文本提出了一种新的统一框架,利用元信息辅助精细粒度识别。
- 该框架通过跨对比预训练联合学习视觉和元信息。
- 使用了图像、文本和元信息三个编码器,并进行了投影嵌入对齐。
- 通过微调图像和元信息编码器,实现了更好的分类性能。
- 在NABirds数据集上的实验结果表明,该框架利用元信息提高了精细粒度识别的准确率。
- 该框架超越了当前基线7.83%,并实现了84.44%的准确率。
点此查看论文截图
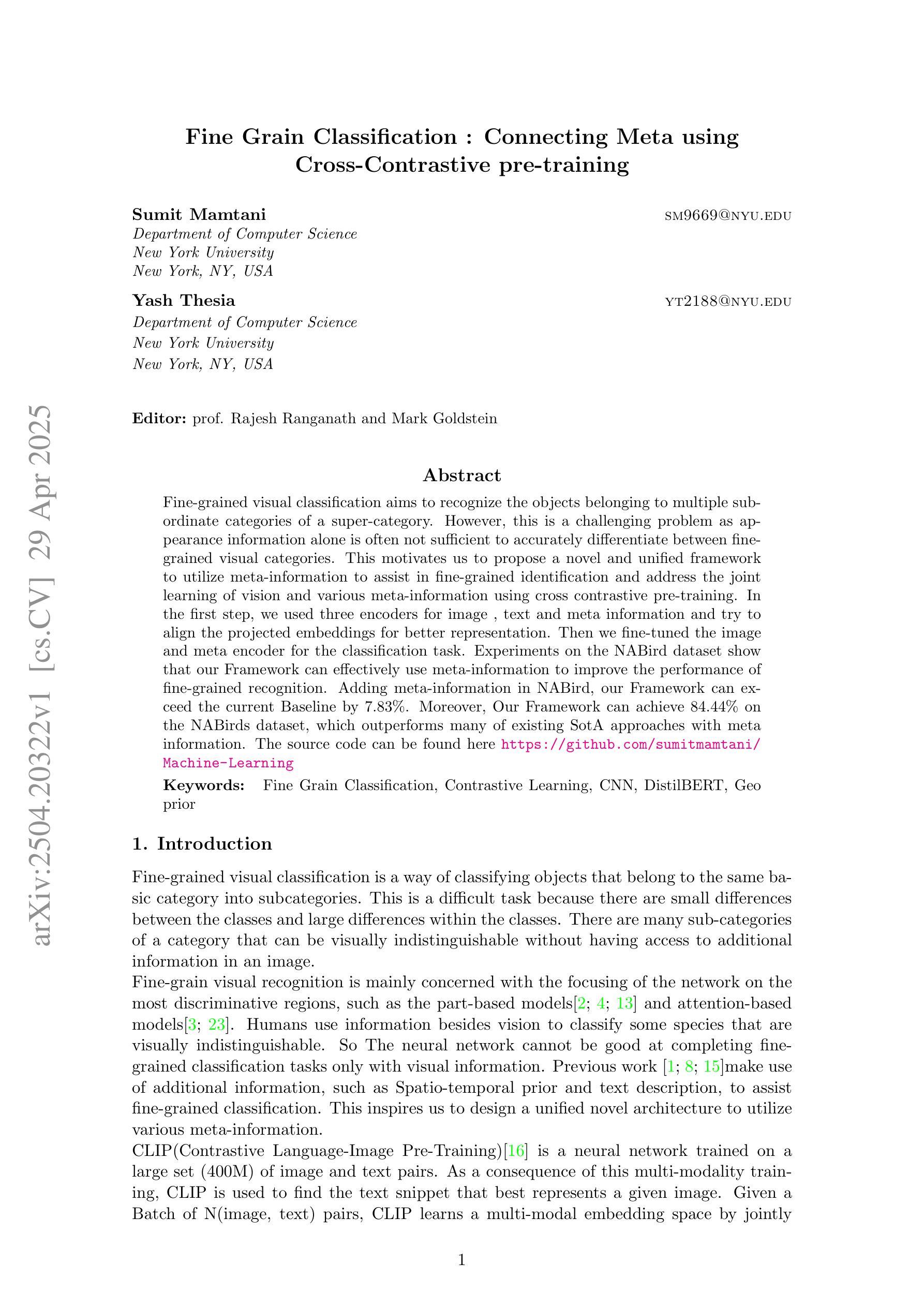
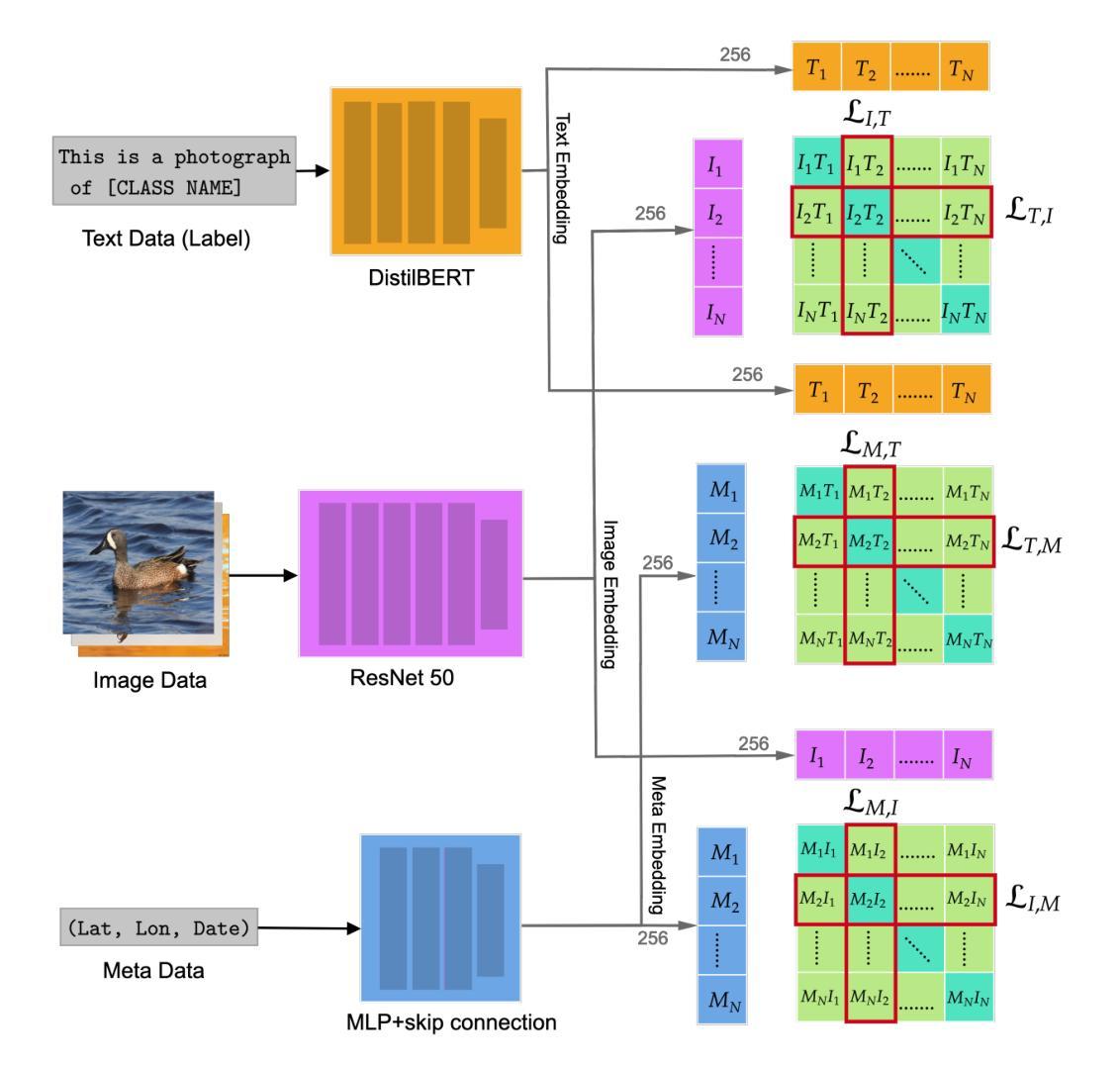
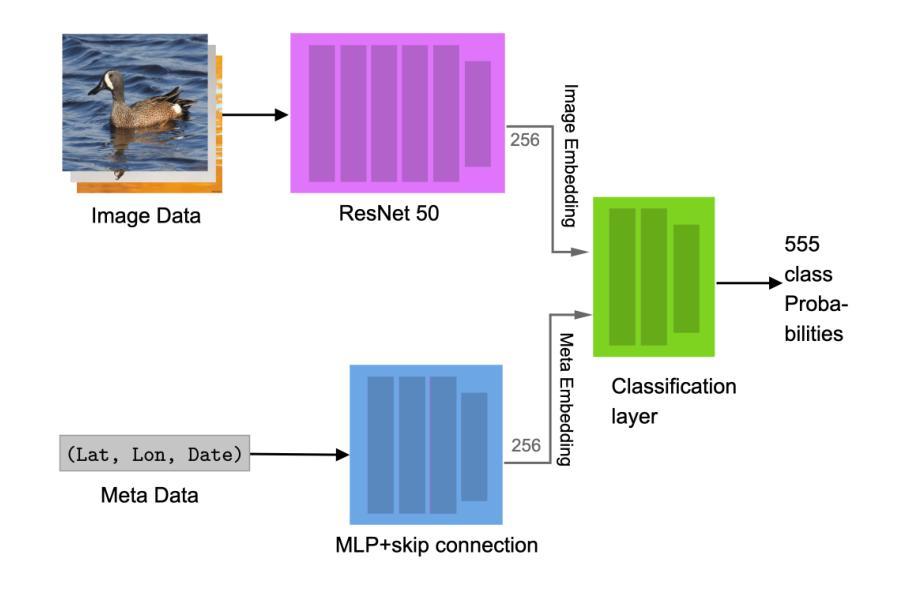
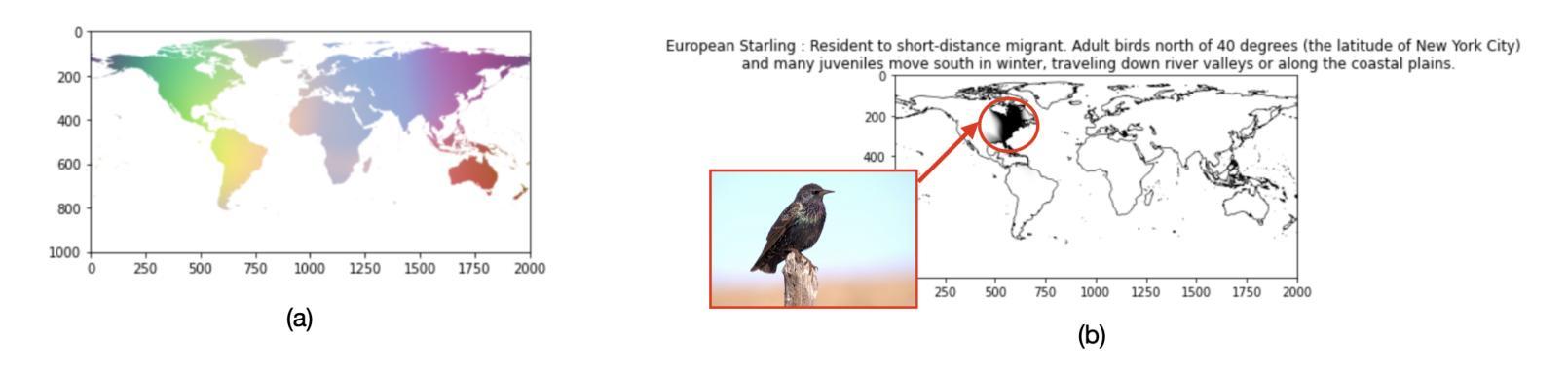
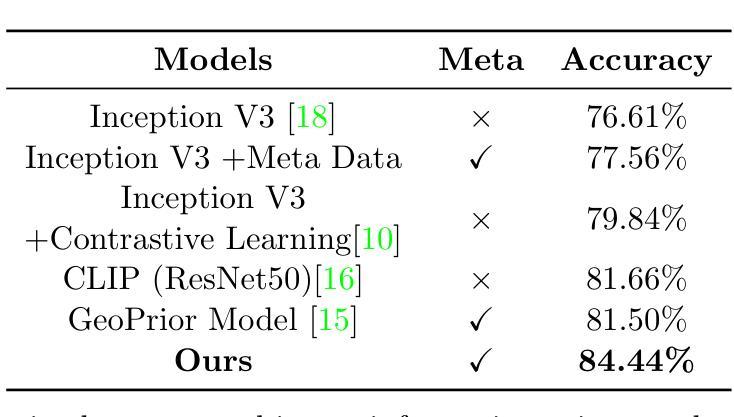
Towards Accurate and Interpretable Neuroblastoma Diagnosis via Contrastive Multi-scale Pathological Image Analysis
Authors:Zhu Zhu, Shuo Jiang, Jingyuan Zheng, Yawen Li, Yifei Chen, Manli Zhao, Weizhong Gu, Feiwei Qin, Jinhu Wang, Gang Yu
Neuroblastoma, adrenal-derived, is among the most common pediatric solid malignancies, characterized by significant clinical heterogeneity. Timely and accurate pathological diagnosis from hematoxylin and eosin-stained whole slide images is critical for patient prognosis. However, current diagnostic practices primarily rely on subjective manual examination by pathologists, leading to inconsistent accuracy. Existing automated whole slide image classification methods encounter challenges such as poor interpretability, limited feature extraction capabilities, and high computational costs, restricting their practical clinical deployment. To overcome these limitations, we propose CMSwinKAN, a contrastive-learning-based multi-scale feature fusion model tailored for pathological image classification, which enhances the Swin Transformer architecture by integrating a Kernel Activation Network within its multilayer perceptron and classification head modules, significantly improving both interpretability and accuracy. By fusing multi-scale features and leveraging contrastive learning strategies, CMSwinKAN mimics clinicians’ comprehensive approach, effectively capturing global and local tissue characteristics. Additionally, we introduce a heuristic soft voting mechanism guided by clinical insights to seamlessly bridge patch-level predictions to whole slide image-level classifications. We validate CMSwinKAN on the PpNTs dataset, which was collaboratively established with our partner hospital and the publicly accessible BreakHis dataset. Results demonstrate that CMSwinKAN performs better than existing state-of-the-art pathology-specific models pre-trained on large datasets. Our source code is available at https://github.com/JSLiam94/CMSwinKAN.
神经母细胞瘤是肾上腺衍生的一种最常见的儿童实体恶性肿瘤之一,具有显著的临床异质性。从苏木精和伊红染色的全切片图像进行及时准确的病理诊断对患者的预后至关重要。然而,目前的诊断方法主要依赖于病理医师的主观肉眼观察,导致诊断准确性不一致。现有的自动全切片图像分类方法面临可解释性差、特征提取能力有限和计算成本高的问题,限制了其在临床实际中的应用。为了克服这些局限性,我们提出了CMSwinKAN,这是一种基于对比学习的多尺度特征融合模型,专为病理图像分类而定制。CMSwinKAN增强了Swin Transformer架构,通过在其多层感知器和分类头模块中集成Kernel Activation Network,显著提高了可解释性和准确性。通过融合多尺度特征和利用对比学习策略,CMSwinKAN模仿了临床医生全面的诊断方法,有效地捕捉了全局和局部组织特征。此外,我们还引入了一种受临床见解启发的主观软投票机制,无缝地将斑块级别的预测与全切片图像级别的分类联系起来。我们在与合作伙伴医院共同建立的PpNTs数据集和可公开访问的BreakHis数据集上验证了CMSwinKAN。结果表明,CMSwinKAN的性能优于在大型数据集上预训练的现有最先进的病理学专用模型。我们的源代码可在https://github.com/JSLiam94/CMSwinKAN获得。
论文及项目相关链接
PDF 10pages, 8 figures
Summary
本文介绍了一种基于对比学习的多尺度特征融合模型CMSwinKAN,用于神经母细胞瘤等儿童实体恶性肿瘤的病理图像分类。该模型结合Swin Transformer架构和Kernel Activation Network,提高了模型的解释性和准确性。通过多尺度特征融合和对比学习策略,CMSwinKAN模拟医生全面的诊断方法,有效捕捉组织和细胞的全球和局部特征。在PpNTs和BreakHis数据集上的验证结果表明,CMSwinKAN的性能优于现有的大型预训练数据集上的病理学模型。
Key Takeaways
- 神经母细胞瘤是儿童常见的固体恶性肿瘤,具有显著的临床异质性,及时准确的病理诊断对预后至关重要。
- 当前诊断方法主要依赖病理医生的主观手动检查,存在准确性不一致的问题。
- CMSwinKAN模型结合了Swin Transformer和Kernel Activation Network,提高了模型的解释性和准确性。
- CMSwinKAN通过多尺度特征融合和对比学习策略,有效捕捉病理图像的全球和局部特征,模拟医生的综合诊断方法。
- 引入的启发式软投票机制能无缝连接斑块级别的预测到整个幻灯片图像级别的分类。
- 在PpNTs和BreakHis数据集上的验证结果显示,CMSwinKAN的性能优于现有的病理学模型。
点此查看论文截图
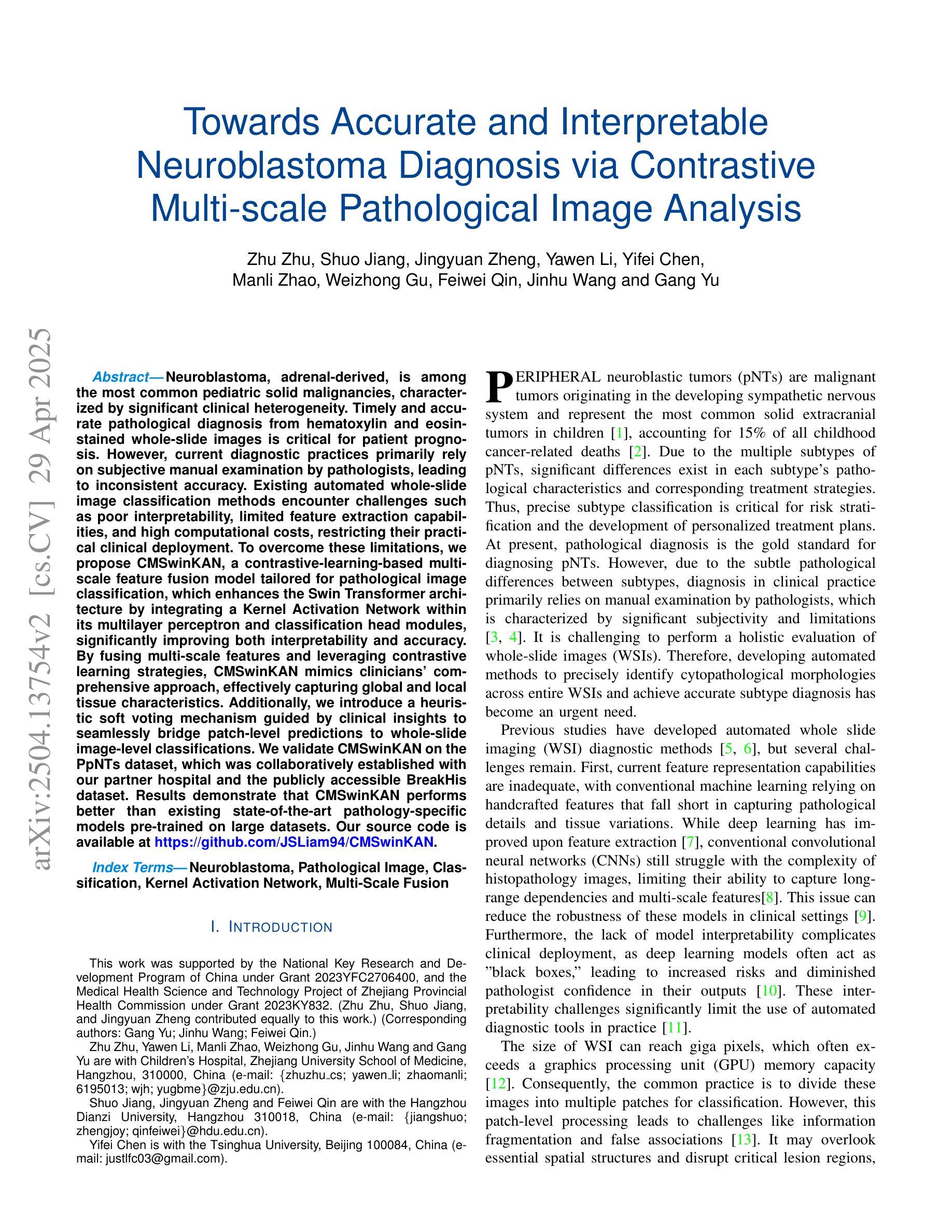
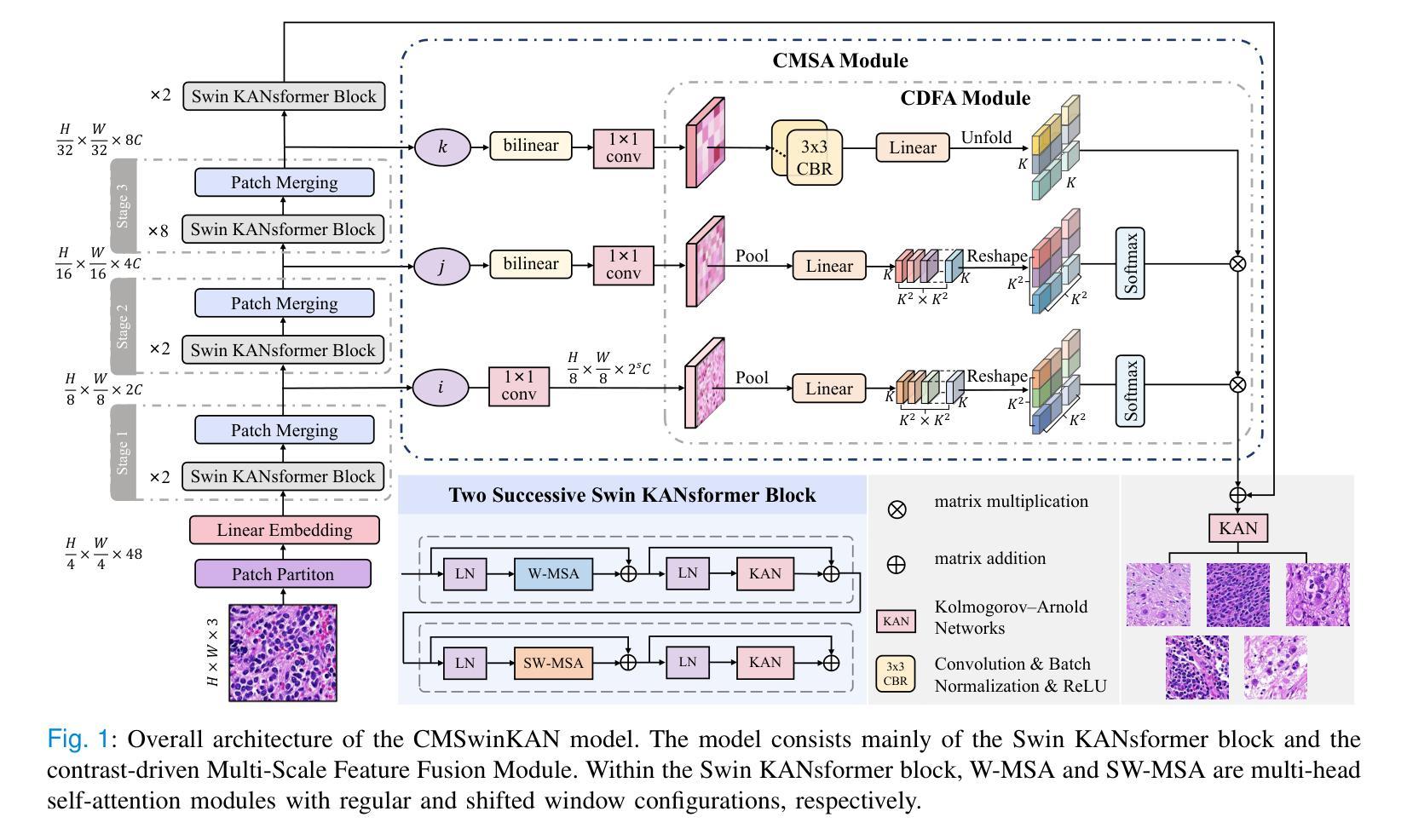
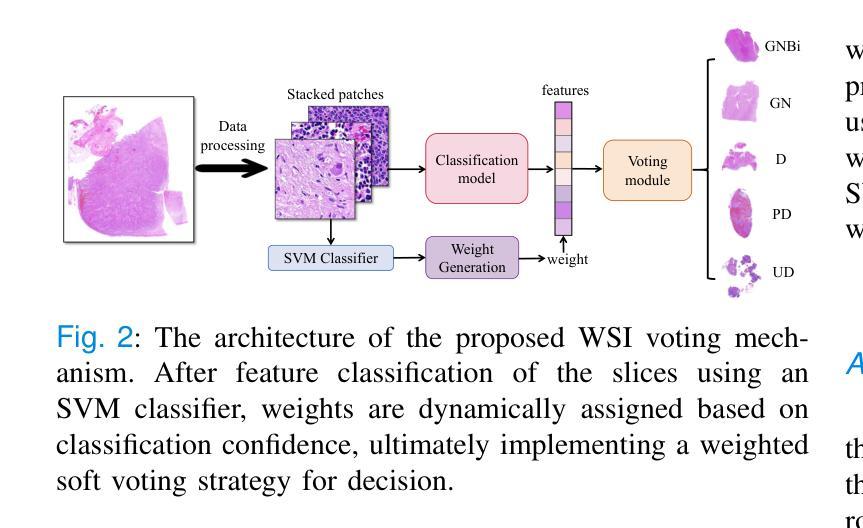
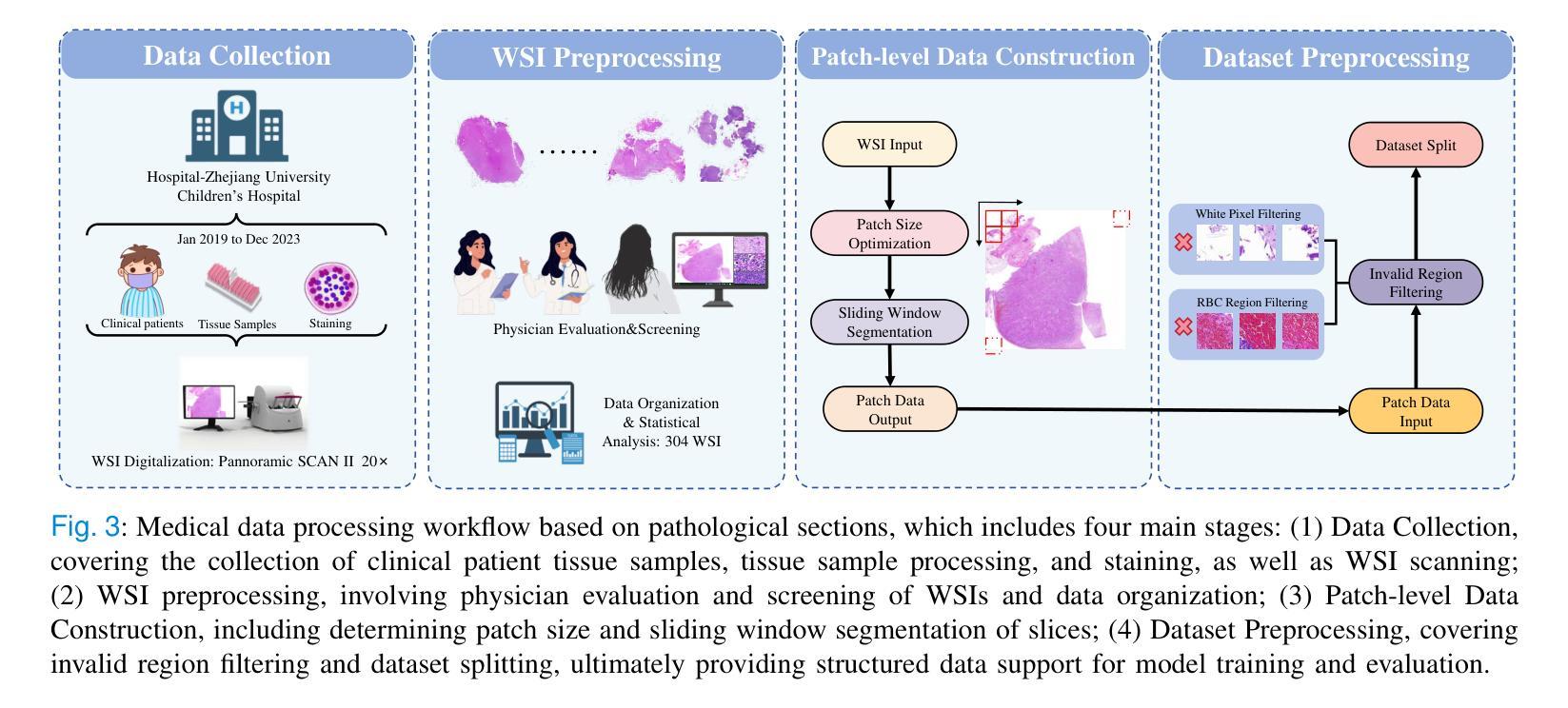
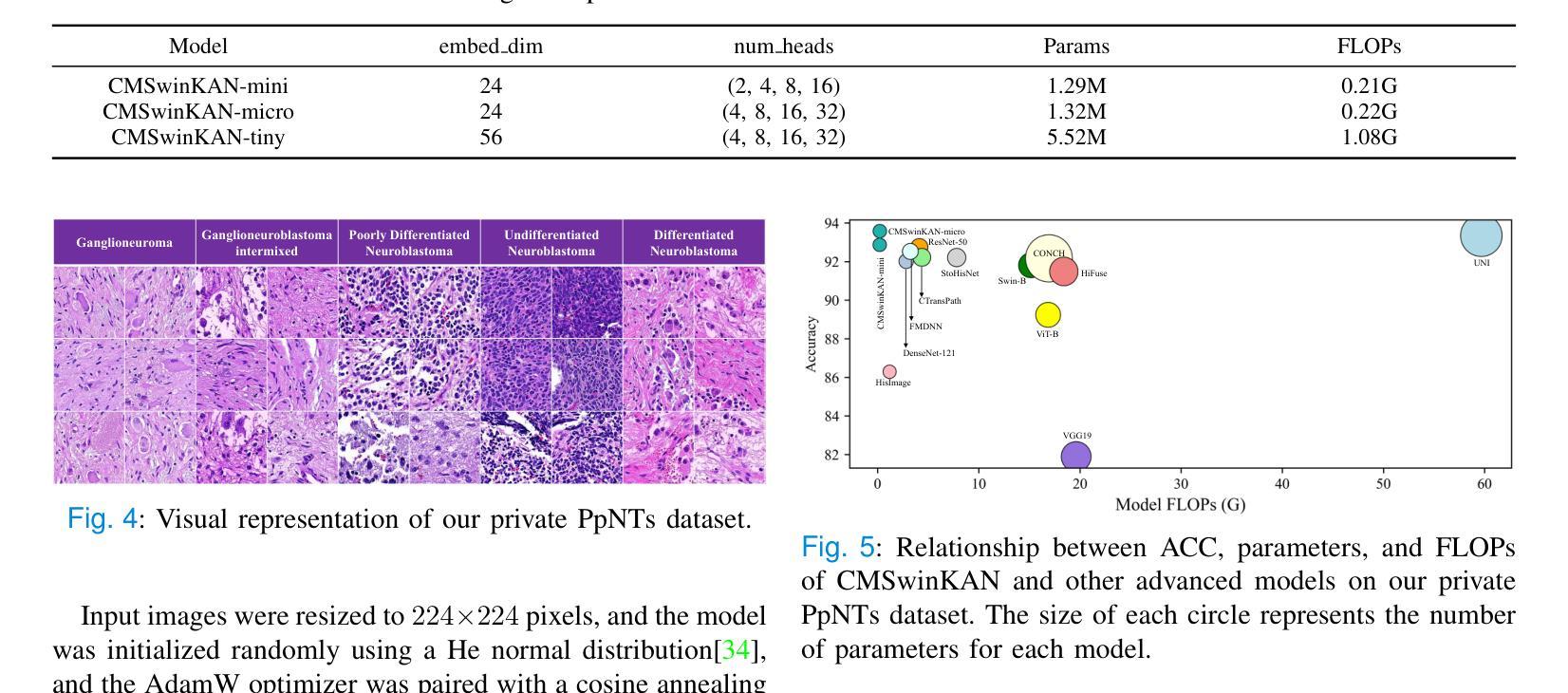
