⚠️ 以下所有内容总结都来自于 大语言模型的能力,如有错误,仅供参考,谨慎使用
🔴 请注意:千万不要用于严肃的学术场景,只能用于论文阅读前的初筛!
💗 如果您觉得我们的项目对您有帮助 ChatPaperFree ,还请您给我们一些鼓励!⭐️ HuggingFace免费体验
2025-05-01 更新
FLIM-based Salient Object Detection Networks with Adaptive Decoders
Authors:Gilson Junior Soares, Matheus Abrantes Cerqueira, Jancarlo F. Gomes, Laurent Najman, Silvio Jamil F. Guimarães, Alexandre Xavier Falcão
Salient Object Detection (SOD) methods can locate objects that stand out in an image, assign higher values to their pixels in a saliency map, and binarize the map outputting a predicted segmentation mask. A recent tendency is to investigate pre-trained lightweight models rather than deep neural networks in SOD tasks, coping with applications under limited computational resources. In this context, we have investigated lightweight networks using a methodology named Feature Learning from Image Markers (FLIM), which assumes that the encoder’s kernels can be estimated from marker pixels on discriminative regions of a few representative images. This work proposes flyweight networks, hundreds of times lighter than lightweight models, for SOD by combining a FLIM encoder with an adaptive decoder, whose weights are estimated for each input image by a given heuristic function. Such FLIM networks are trained from three to four representative images only and without backpropagation, making the models suitable for applications under labeled data constraints as well. We study five adaptive decoders; two of them are introduced here. Differently from the previous ones that rely on one neuron per pixel with shared weights, the heuristic functions of the new adaptive decoders estimate the weights of each neuron per pixel. We compare FLIM models with adaptive decoders for two challenging SOD tasks with three lightweight networks from the state-of-the-art, two FLIM networks with decoders trained by backpropagation, and one FLIM network whose labeled markers define the decoder’s weights. The experiments demonstrate the advantages of the proposed networks over the baselines, revealing the importance of further investigating such methods in new applications.
显著性目标检测(SOD)方法可以定位图像中突出的目标,在显著性地图中为这些目标的像素分配更高的值,并通过二值化地图输出预测的分割掩膜。目前的一个趋势是在SOD任务中研究预训练的轻量级模型,而不是深度神经网络,以应对有限的计算资源下的应用。在此背景下,我们研究了使用名为“从图像标记学习特征”(FLIM)的方法的轻量级网络,该方法假设编码器的内核可以从少数代表性图像的判别区域的标记像素来估计。本研究提出了轻量级模型数百倍的轻量级网络,用于SOD。这是通过将FLIM编码器与自适应解码器相结合来实现的,自适应解码器的权重是通过给定启发式函数为每种输入图像估计得出的。这种FLIM网络仅需三至四张代表性图像进行训练,并且无需反向传播,使得模型非常适合受标签数据约束的应用。我们研究了五种自适应解码器,其中两种在这里介绍。与以前的那种每个像素只依赖一个神经元且权重共享不同,新的自适应解码器的启发式函数会估计每个神经元的权重。我们将带有自适应解码器的FLIM模型与两个具有挑战性的SOD任务进行了比较,包括三个来自最新技术的轻量级网络、两个通过反向传播训练的FLIM网络解码器,以及一个定义解码器权重的标记标记的FLIM网络。实验证明了所提出网络相对于基准线的优势,揭示了在新应用中进一步调查此类方法的重要性。
论文及项目相关链接
PDF This work has been submitted to the Journal of the Brazilian Computer Society (JBCS)
摘要
本文探讨了显著目标检测(SOD)中的轻量级网络模型。研究了一种名为特征学习自图像标记(FLIM)的方法,通过从少数代表性图像的判别区域的标记像素估计编码器内核。文章提出了轻量级网络模型——FLIM网络,该网络结合了FLIM编码器和自适应解码器,为SOD任务提供了数百倍轻量级的选择。这些网络仅通过三到四张代表性图像进行训练,无需反向传播,适合标签数据受限的应用场景。本文研究了五种自适应解码器,其中两种是新颖的。不同于以往的共享权重每个像素一个神经元的方法,新的自适应解码器的启发式函数估计每个神经元的权重。实验表明,与两个具有挑战性的SOD任务中的基线相比,所提出的网络具有优势,表明需要进一步研究此类方法在其他新应用中的应用前景。
关键见解
- 显著目标检测(SOD)可以定位图像中的突出对象,并在显著性地图中为这些对象分配更高的像素值。
- 最近的研究趋势是探索轻量级模型而非深度神经网络在SOD任务中的应用,以适应有限的计算资源。
- FLIM方法是一种用于轻量级网络的特征学习方法,通过从少数代表性图像的判别区域标记像素估计编码器内核。
- FLIM网络结合了FLIM编码器和自适应解码器,为SOD提供了轻量级解决方案。
- FLIM网络仅需要少量代表性图像进行训练,无需反向传播,适合标签数据受限的场景。
- 本文研究了五种自适应解码器,其中两种具有新颖的启发式函数,能够估计每个神经元的权重。
点此查看论文截图
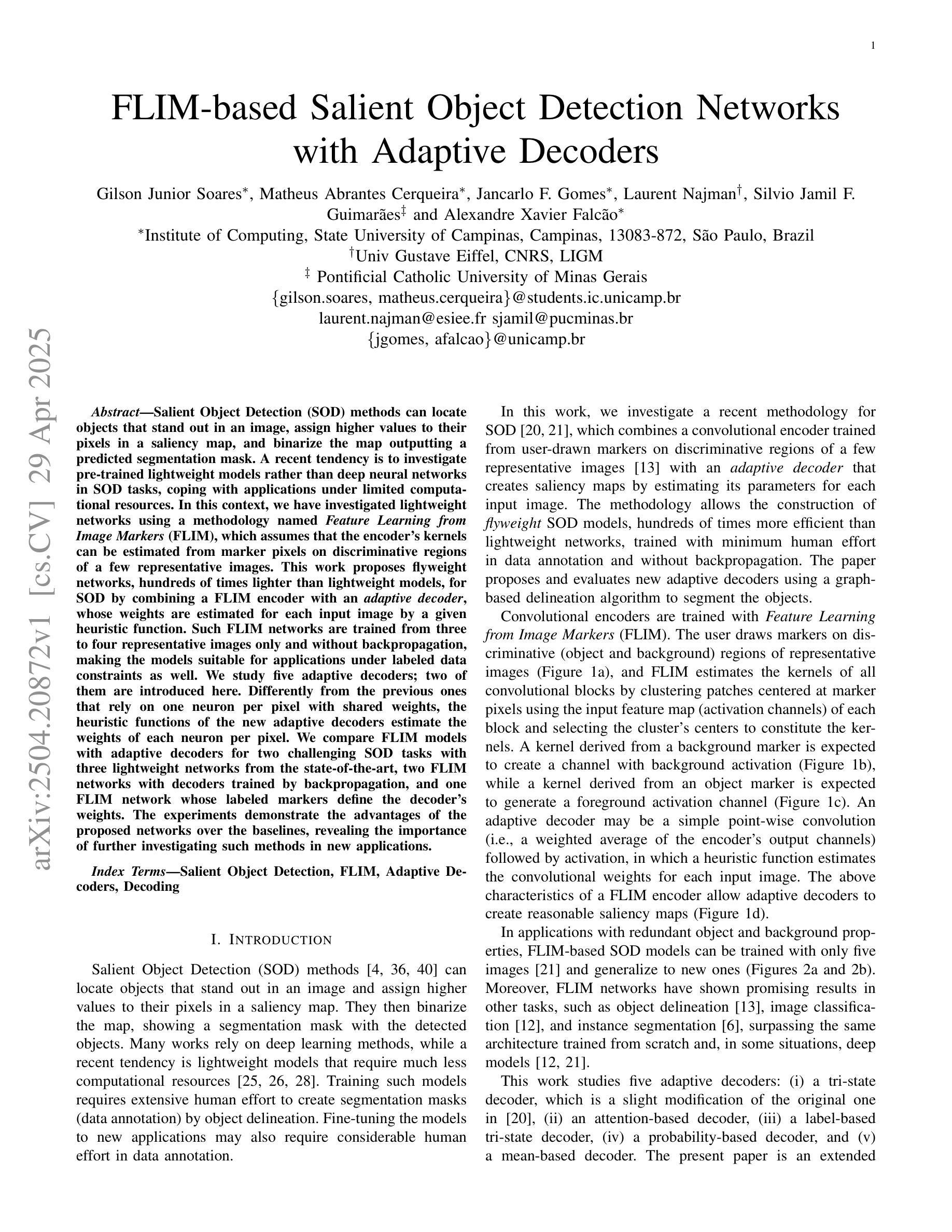
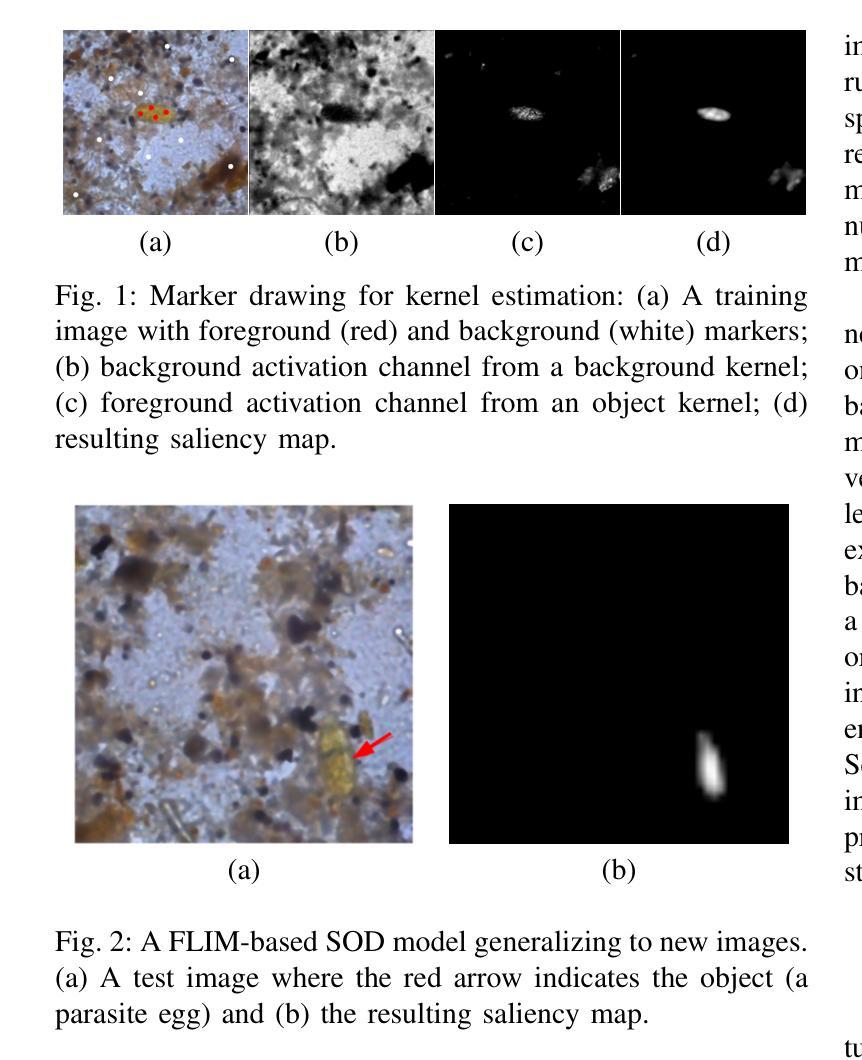
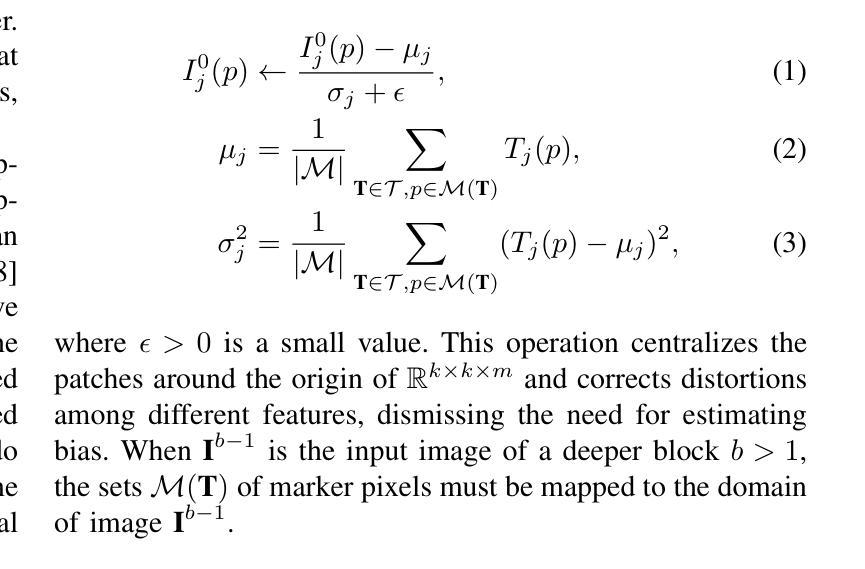
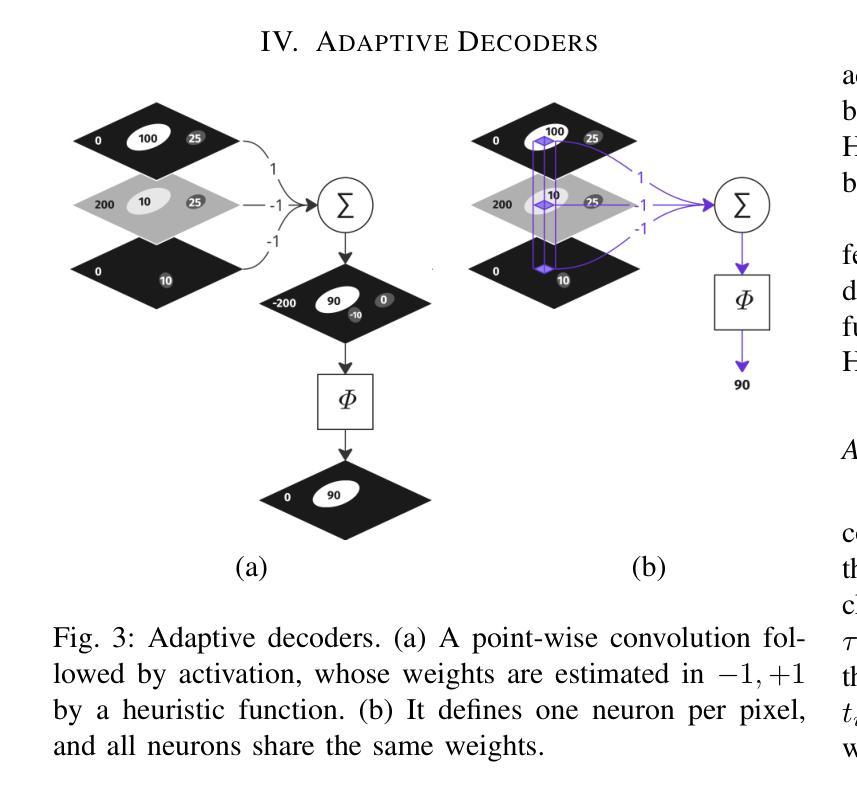
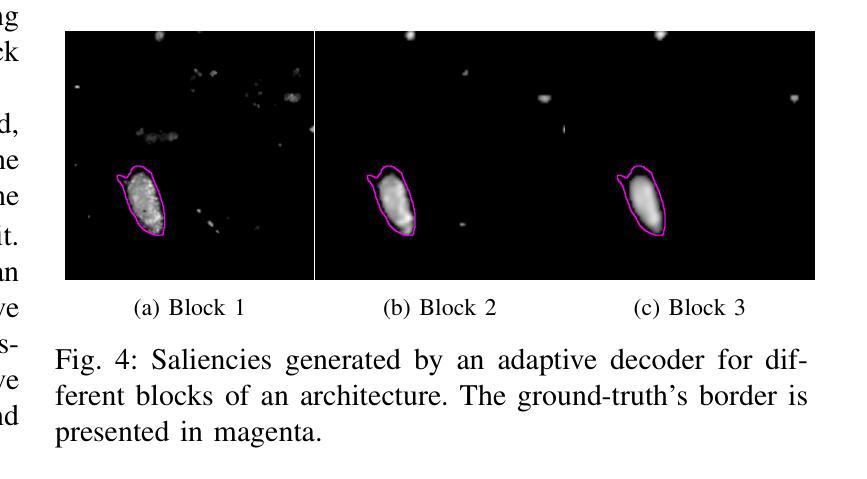
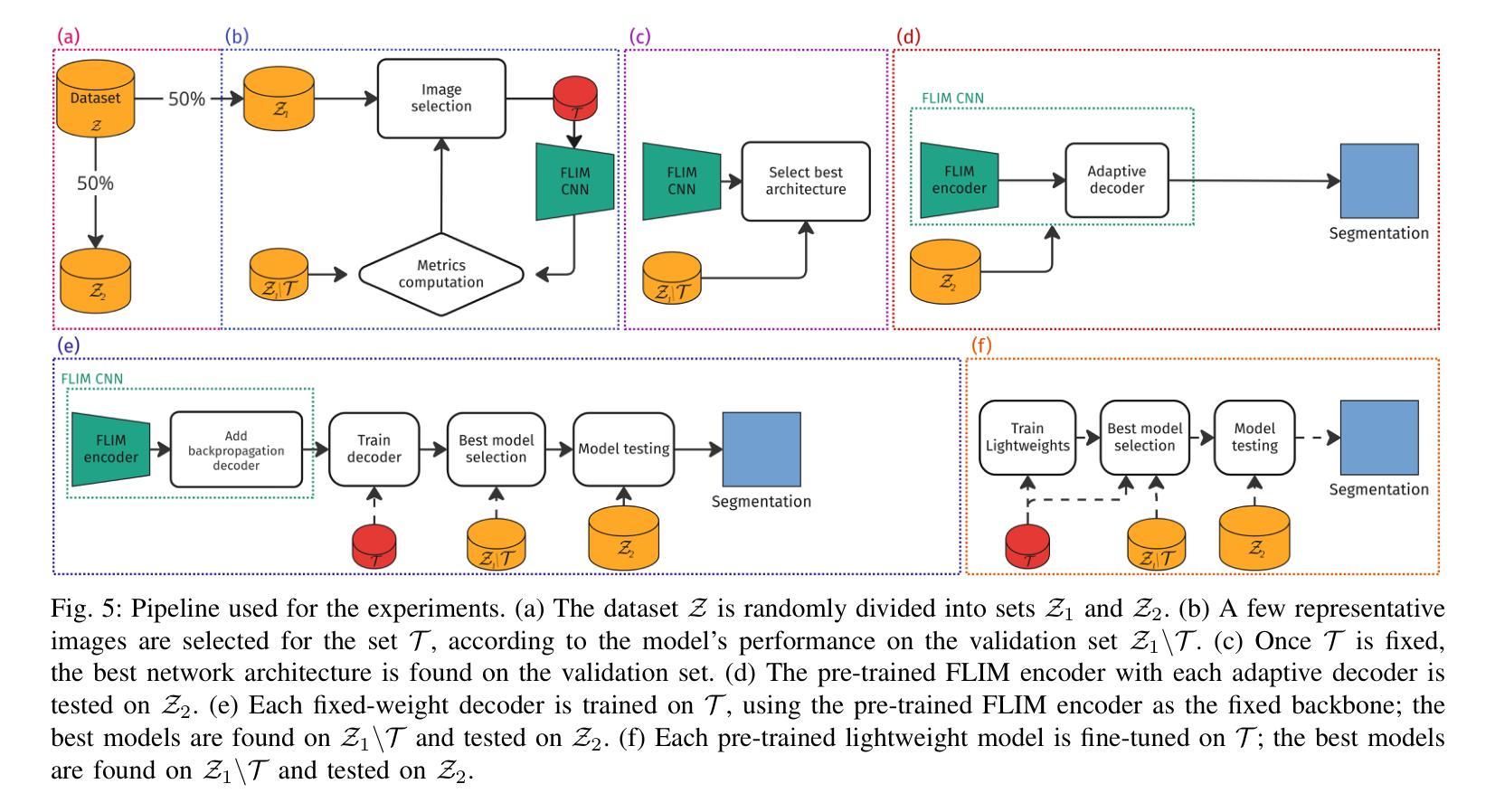