⚠️ 以下所有内容总结都来自于 大语言模型的能力,如有错误,仅供参考,谨慎使用
🔴 请注意:千万不要用于严肃的学术场景,只能用于论文阅读前的初筛!
💗 如果您觉得我们的项目对您有帮助 ChatPaperFree ,还请您给我们一些鼓励!⭐️ HuggingFace免费体验
2025-05-01 更新
AI-GenBench: A New Ongoing Benchmark for AI-Generated Image Detection
Authors:Lorenzo Pellegrini, Davide Cozzolino, Serafino Pandolfini, Davide Maltoni, Matteo Ferrara, Luisa Verdoliva, Marco Prati, Marco Ramilli
The rapid advancement of generative AI has revolutionized image creation, enabling high-quality synthesis from text prompts while raising critical challenges for media authenticity. We present Ai-GenBench, a novel benchmark designed to address the urgent need for robust detection of AI-generated images in real-world scenarios. Unlike existing solutions that evaluate models on static datasets, Ai-GenBench introduces a temporal evaluation framework where detection methods are incrementally trained on synthetic images, historically ordered by their generative models, to test their ability to generalize to new generative models, such as the transition from GANs to diffusion models. Our benchmark focuses on high-quality, diverse visual content and overcomes key limitations of current approaches, including arbitrary dataset splits, unfair comparisons, and excessive computational demands. Ai-GenBench provides a comprehensive dataset, a standardized evaluation protocol, and accessible tools for both researchers and non-experts (e.g., journalists, fact-checkers), ensuring reproducibility while maintaining practical training requirements. By establishing clear evaluation rules and controlled augmentation strategies, Ai-GenBench enables meaningful comparison of detection methods and scalable solutions. Code and data are publicly available to ensure reproducibility and to support the development of robust forensic detectors to keep pace with the rise of new synthetic generators.
人工智能生成技术的快速发展彻底改变了图像创作的方式,它使得通过文本提示生成高质量图像成为可能,同时也为媒体真实性带来了重大挑战。我们推出了Ai-GenBench,这是一个新型基准测试平台,旨在解决现实场景中检测AI生成图像的需求。与其他在静态数据集上评估模型的现有解决方案不同,Ai-GenBench引入了时间评估框架,该框架通过在合成图像上逐步训练检测算法,并按其生成模型的顺序进行历史排序,以测试其适应新生成模型的能力,例如从生成对抗网络(GANs)到扩散模型的过渡。我们的基准测试平台专注于高质量、多样化的视觉内容,并克服了当前方法的关键局限性,包括任意数据集分割、不公平比较和过高的计算需求。Ai-GenBench提供了一个综合数据集、标准化的评估协议以及方便研究者和非专家(如记者、事实核查人员)使用的工具,确保实用性和可重复性训练要求。通过制定明确的评估规则和受控增强策略,Ai-GenBench能够实现检测方法的实质性比较和可扩展解决方案。为确保可重复性和支持开发稳健的取证检测器以应对新合成生成器的兴起,代码和数据均已公开可用。
论文及项目相关链接
PDF 9 pages, 6 figures, 4 tables, code available: https://github.com/MI-BioLab/AI-GenBench
Summary
生成式AI的迅速发展已推动图像创作领域革命,并能从文本提示中进行高质量合成,同时为媒体真实性带来重大挑战。我们推出Ai-GenBench,这是一个新颖的基准测试平台,旨在解决在真实场景中检测AI生成图像的需求问题。与其他在静态数据集上评估模型的解决方案不同,Ai-GenBench引入了临时评估框架,该框架按照生成模型的历史顺序对合成图像进行增量训练,以测试其对新生成模型(例如从GAN到扩散模型)的通用能力。我们的基准测试专注于高质量、多样化的视觉内容,并克服了当前方法的关键局限性,包括任意数据集分割、不公平比较和过高的计算需求。Ai-GenBench提供了一个综合数据集、标准化的评估协议以及方便研究者和非专家(例如记者、事实核查人员)使用的工具,以确保实用性培训要求的同时保证可重复性。通过建立明确的评估规则和受控的增强策略,Ai-GenBench实现了检测方法的有意义比较和可扩展解决方案。代码和数据公开,以确保可重复性和稳健的取证检测器的发展,以适应新的合成生成器的崛起。
Key Takeaways
- 生成式AI推动了图像创作的革命,实现从文本提示高质量合成图像。
- Ai-GenBench是一个新颖的基准测试平台,用于检测AI生成的图像。
- Ai-GenBench采用临时评估框架,通过增量训练检测方法来测试对新生成模型的通用性。
- 平台专注于高质量、多样化的视觉内容,并克服现有方法的局限性。
- Ai-GenBench提供综合数据集、标准化评估协议及方便使用的工具,适用于研究者和非专家。
- 明确的评估规则和受控增强策略确保检测方法的比较具有实际意义并推动可扩展解决方案。
点此查看论文截图
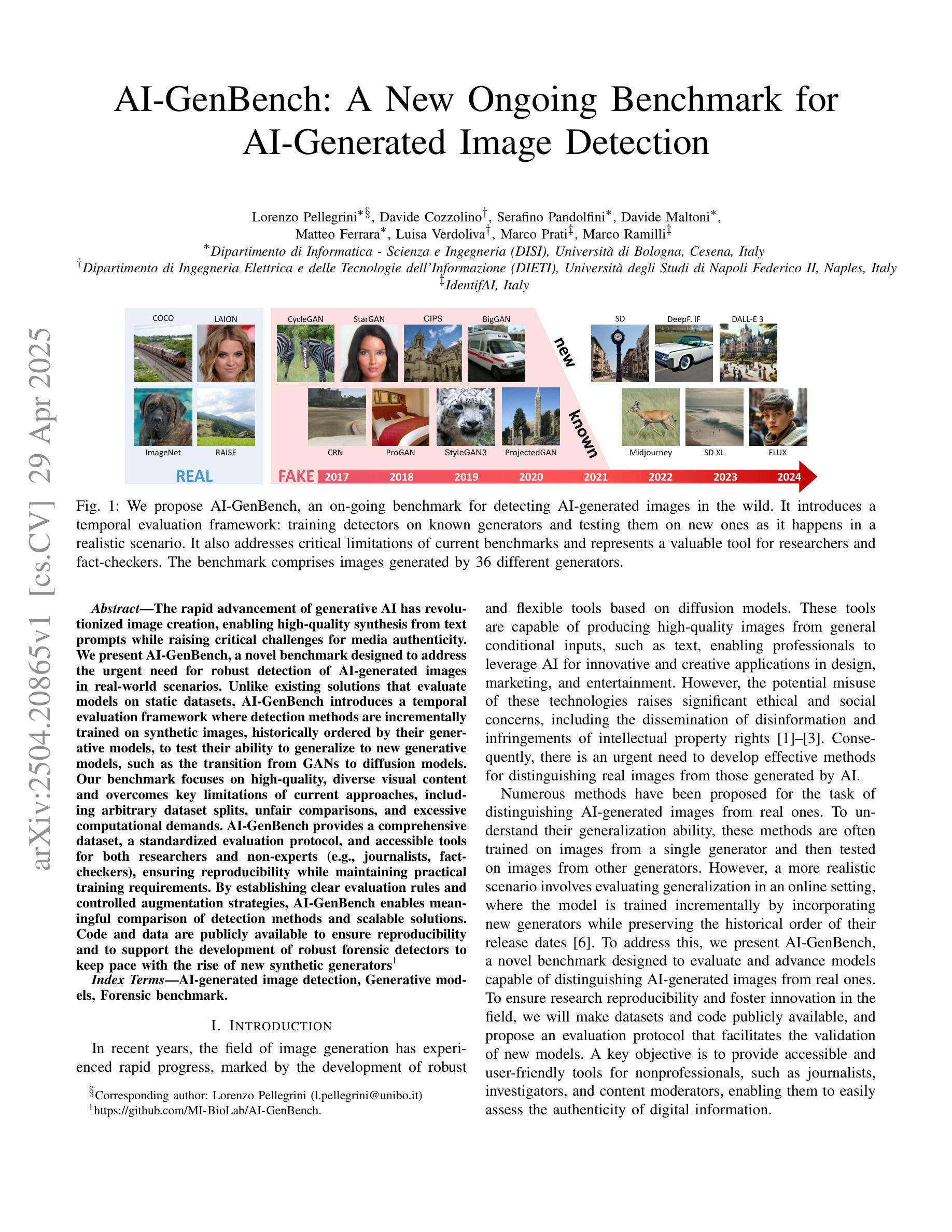
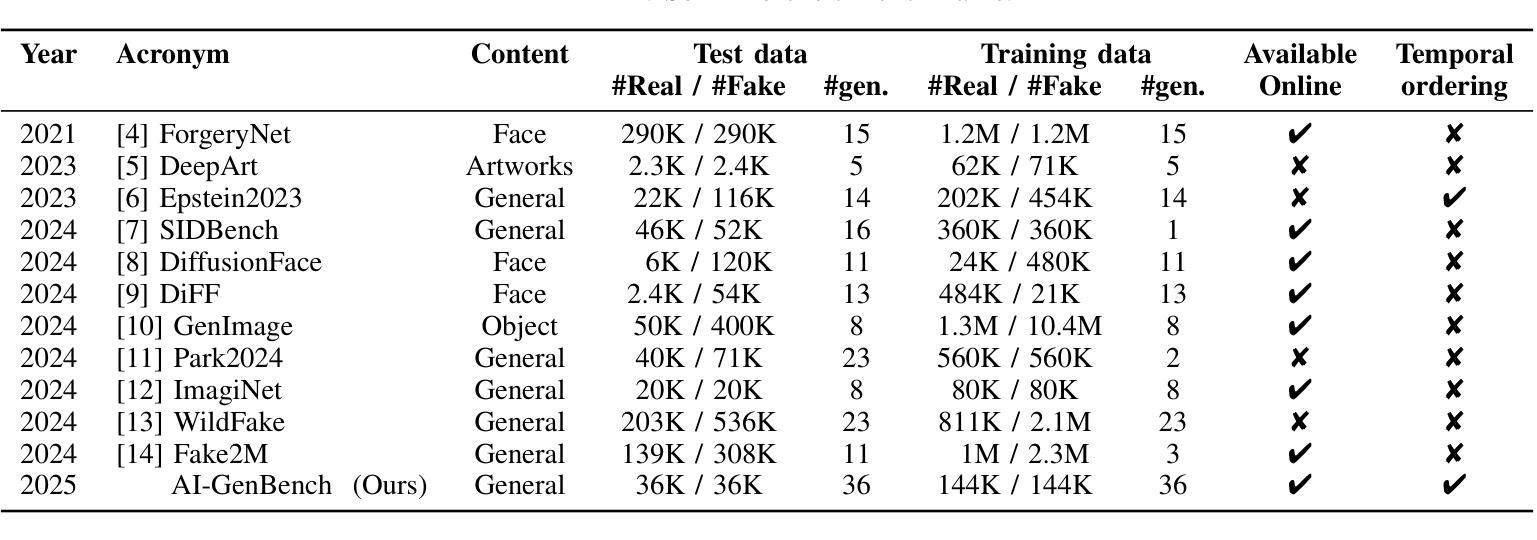
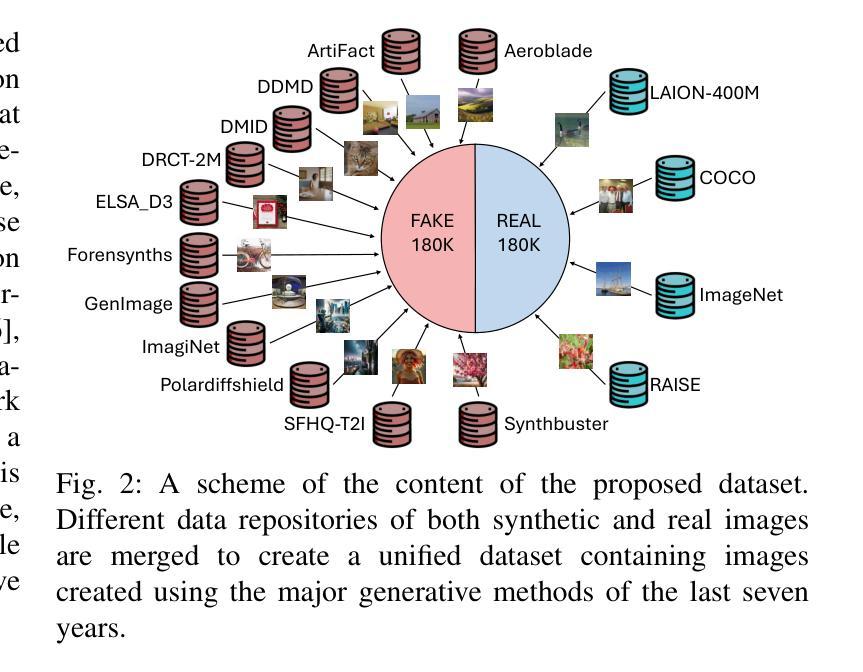
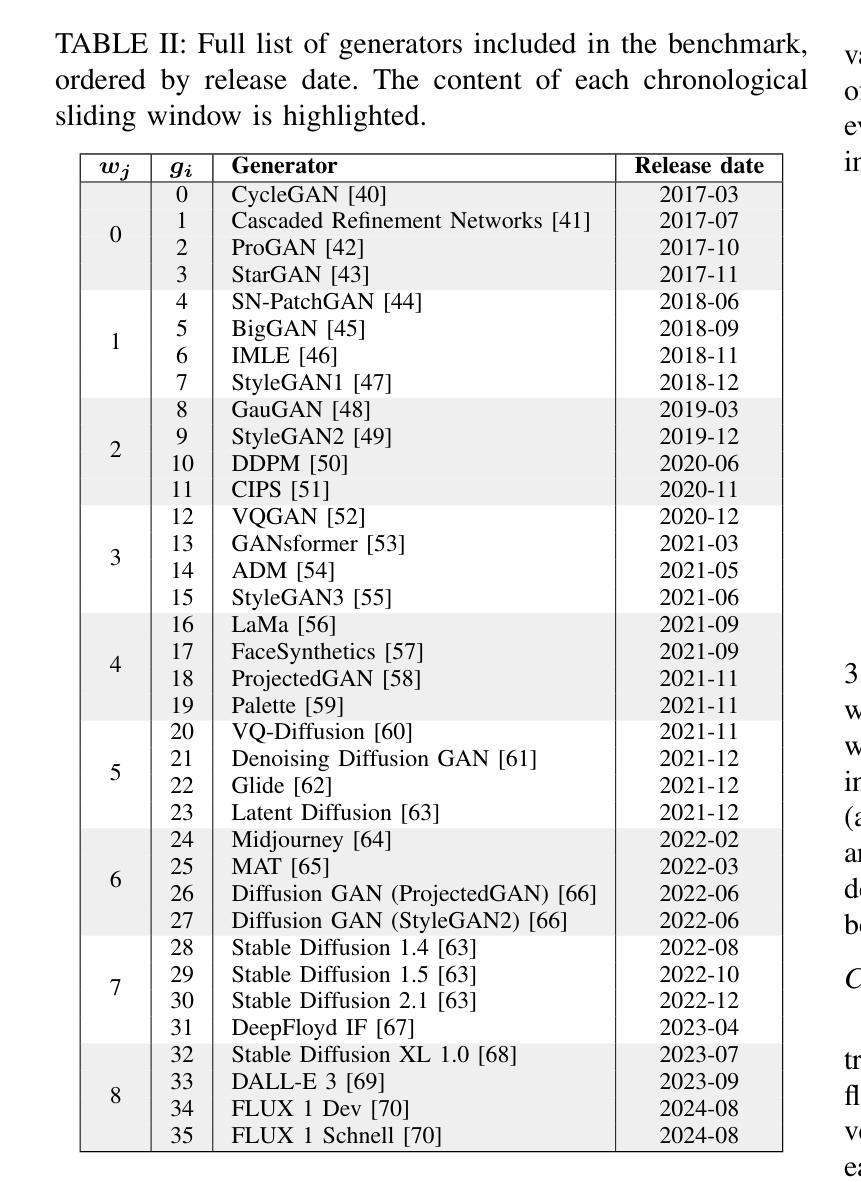
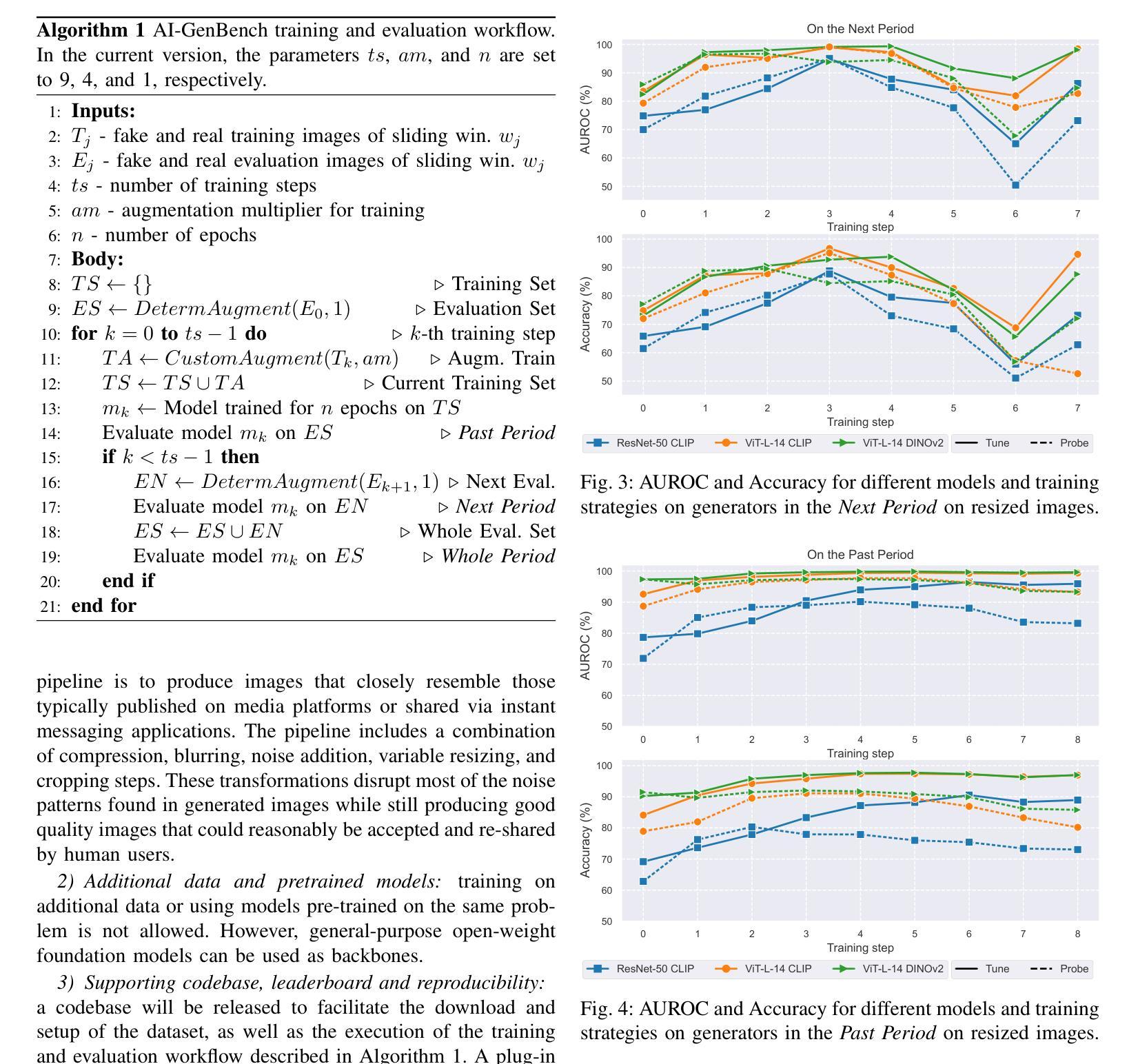
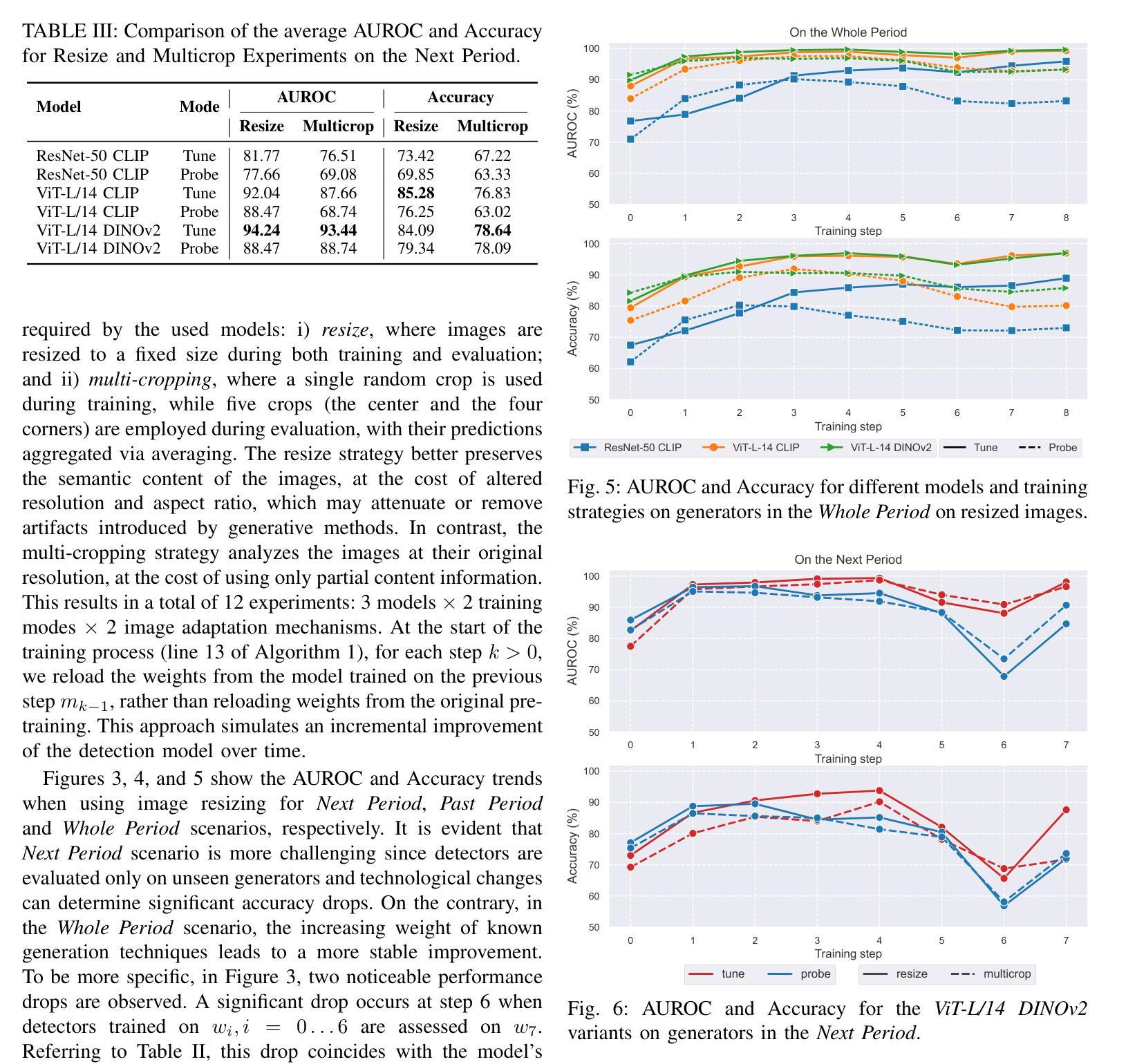