⚠️ 以下所有内容总结都来自于 大语言模型的能力,如有错误,仅供参考,谨慎使用
🔴 请注意:千万不要用于严肃的学术场景,只能用于论文阅读前的初筛!
💗 如果您觉得我们的项目对您有帮助 ChatPaperFree ,还请您给我们一些鼓励!⭐️ HuggingFace免费体验
2025-05-01 更新
Efficient Listener: Dyadic Facial Motion Synthesis via Action Diffusion
Authors:Zesheng Wang, Alexandre Bruckert, Patrick Le Callet, Guangtao Zhai
Generating realistic listener facial motions in dyadic conversations remains challenging due to the high-dimensional action space and temporal dependency requirements. Existing approaches usually consider extracting 3D Morphable Model (3DMM) coefficients and modeling in the 3DMM space. However, this makes the computational speed of the 3DMM a bottleneck, making it difficult to achieve real-time interactive responses. To tackle this problem, we propose Facial Action Diffusion (FAD), which introduces the diffusion methods from the field of image generation to achieve efficient facial action generation. We further build the Efficient Listener Network (ELNet) specially designed to accommodate both the visual and audio information of the speaker as input. Considering of FAD and ELNet, the proposed method learns effective listener facial motion representations and leads to improvements of performance over the state-of-the-art methods while reducing 99% computational time.
生成双人对话中的真实听者面部表情仍然是一个挑战,这主要是由于动作空间的高维度和时间的依赖性要求。现有方法通常考虑提取3D可变形模型(3DMM)系数并在3DMM空间中进行建模。然而,这使得3DMM的计算速度成为瓶颈,难以实现实时交互响应。为了解决这一问题,我们提出了面部动作扩散(FAD),它引入了图像生成领域的扩散方法,以实现高效的面部动作生成。我们还专门构建了高效听者网络(ELNet),以容纳说话者的视觉和音频信息作为输入。考虑到FAD和ELNet,所提出的方法学习了有效的听者面部表情表示,在性能上超过了最先进的方法,同时减少了99%的计算时间。
论文及项目相关链接
Summary
本文提出一种名为面部动作扩散(FAD)的方法,结合图像生成领域的扩散方法实现高效的面部动作生成,并建立了专门用于处理说话者视觉和音频信息的Efficient Listener Network (ELNet)。新方法能更高效地生成对话中的听者面部动作,性能优于现有方法,计算时间减少99%。
Key Takeaways
- 文中提出了一种新的方法——面部动作扩散(FAD),该方法利用图像生成领域的扩散方法实现面部动作的生成。
- 存在的问题是生成对话中的听者面部动作面临高维动作空间和时序依赖性的挑战,以及3D Morphable Model (3DMM)的计算速度瓶颈。
- FAD方法的引入有效地解决了这些问题,提高了生成面部动作的效率和性能。
- 建立了Efficient Listener Network (ELNet),能够同时处理说话者的视觉和音频信息。
- ELNet的设计使得新方法能够更有效地学习听者面部动作表示。
- 与现有方法相比,新方法的性能有所提高,计算时间减少了99%。
点此查看论文截图
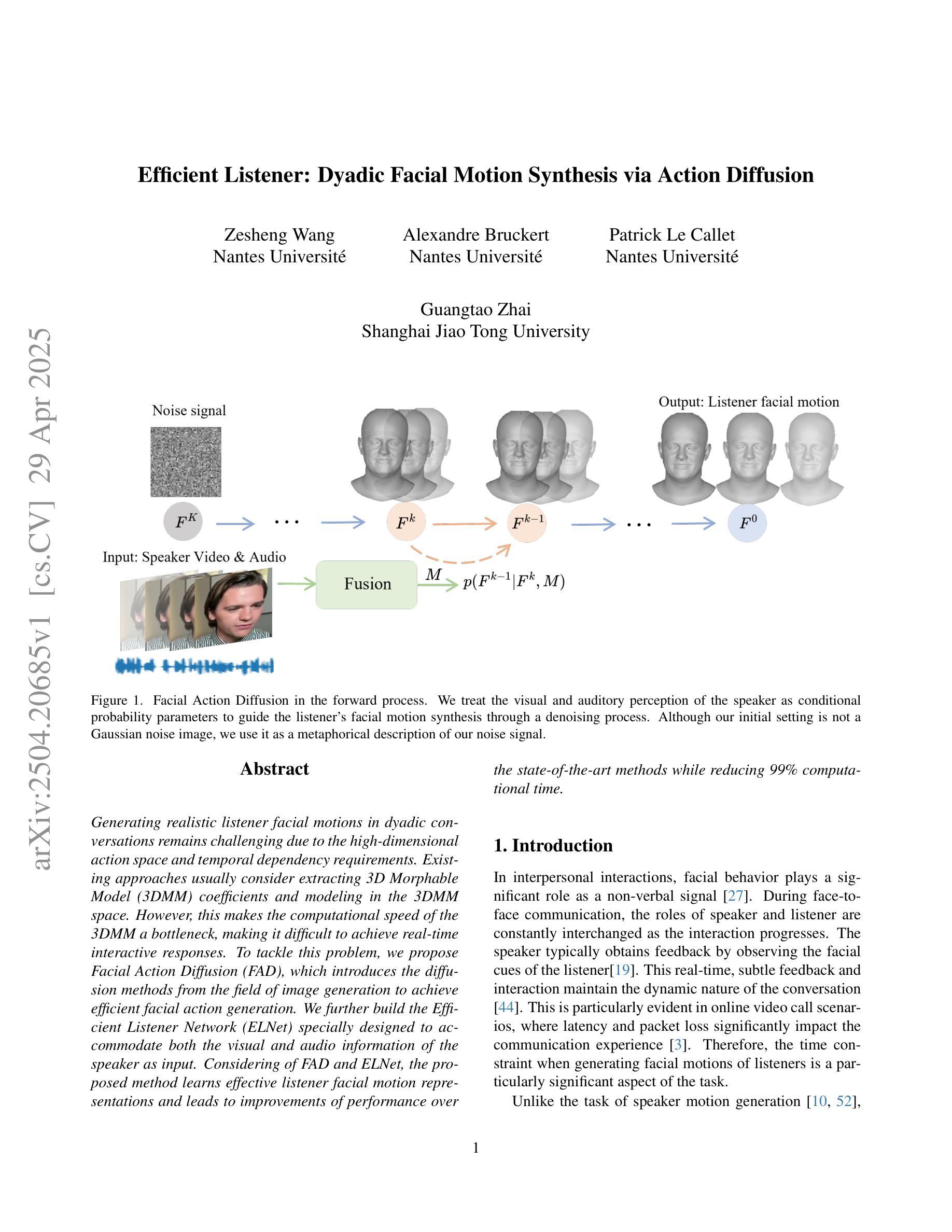
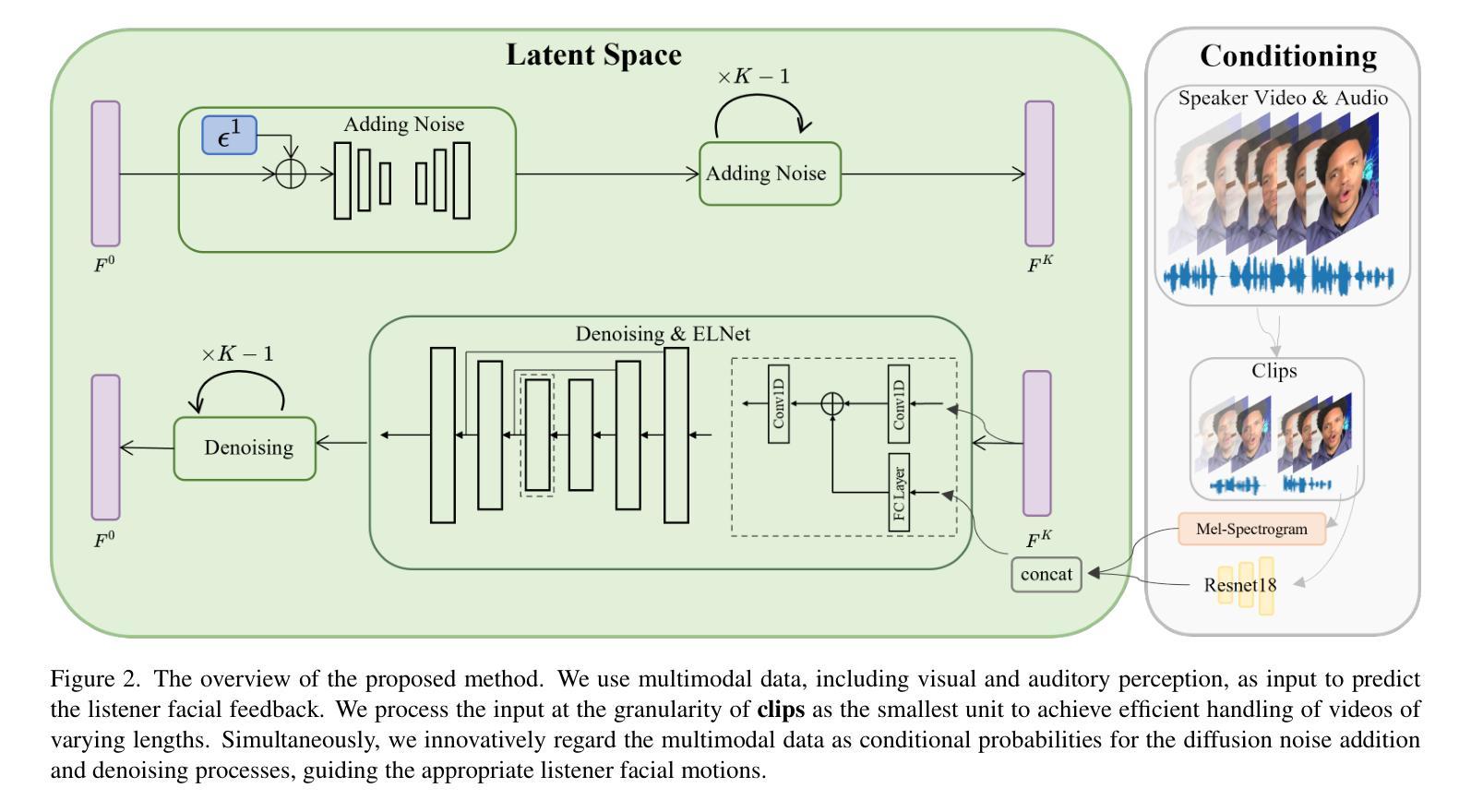
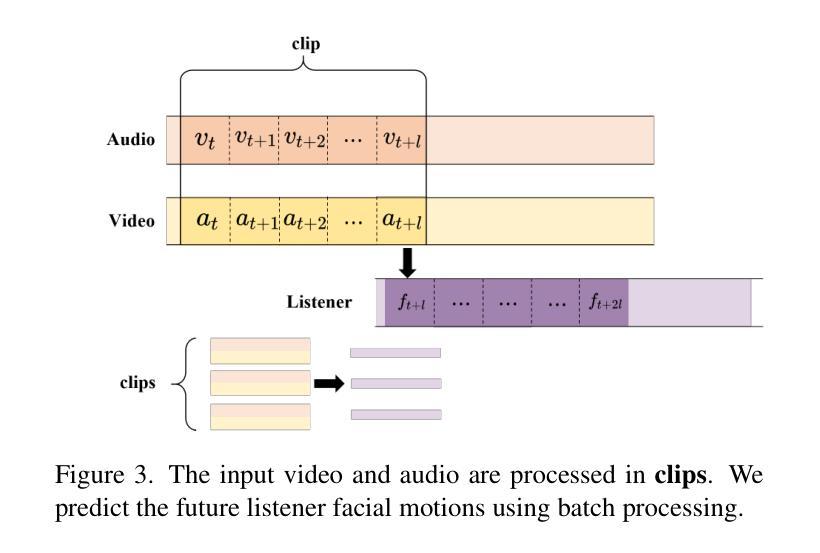
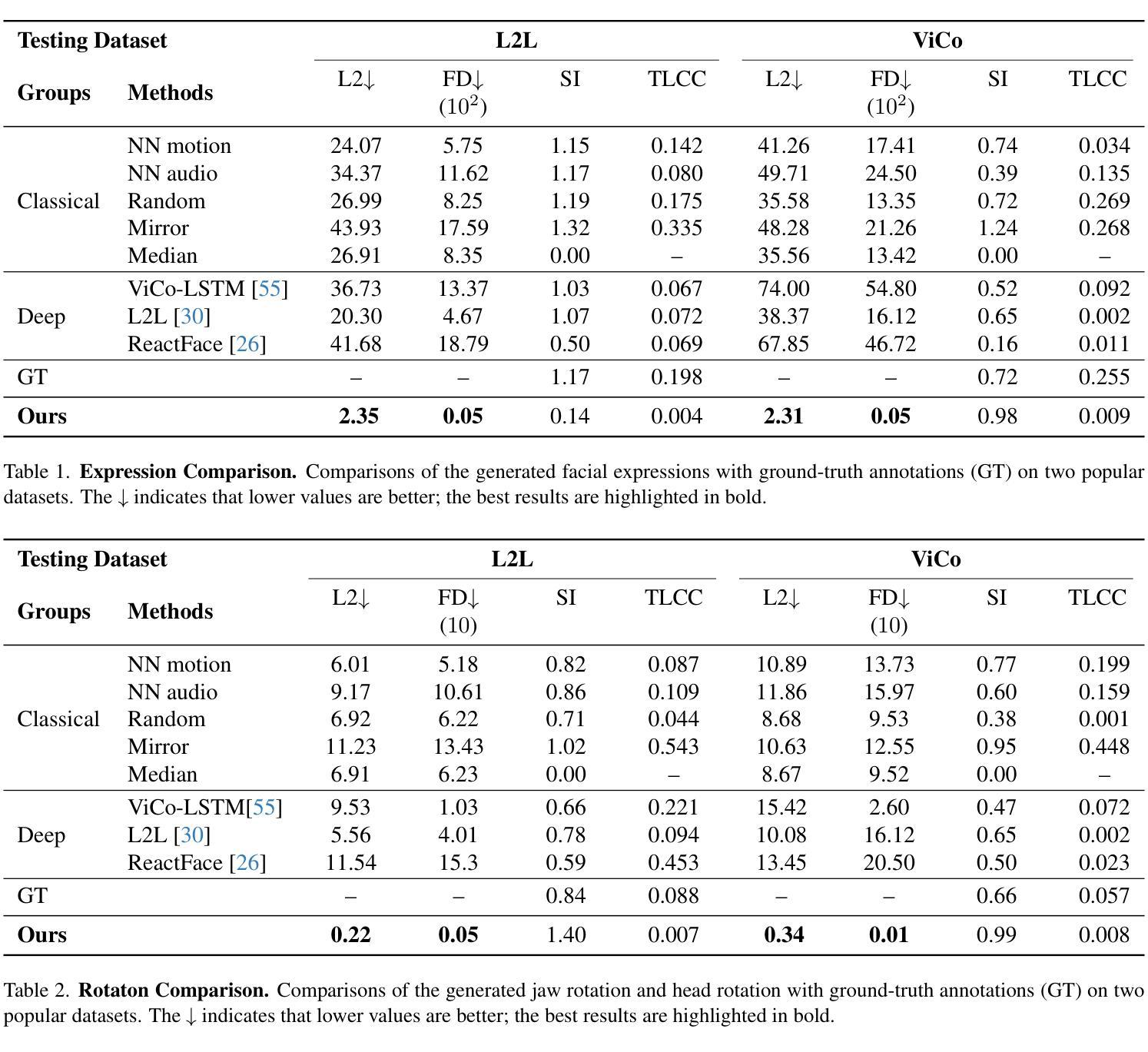