⚠️ 以下所有内容总结都来自于 大语言模型的能力,如有错误,仅供参考,谨慎使用
🔴 请注意:千万不要用于严肃的学术场景,只能用于论文阅读前的初筛!
💗 如果您觉得我们的项目对您有帮助 ChatPaperFree ,还请您给我们一些鼓励!⭐️ HuggingFace免费体验
2025-05-01 更新
ChestX-Reasoner: Advancing Radiology Foundation Models with Reasoning through Step-by-Step Verification
Authors:Ziqing Fan, Cheng Liang, Chaoyi Wu, Ya Zhang, Yanfeng Wang, Weidi Xie
Recent advances in reasoning-enhanced large language models (LLMs) and multimodal LLMs (MLLMs) have significantly improved performance in complex tasks, yet medical AI models often overlook the structured reasoning processes inherent in clinical practice. In this work, we present ChestX-Reasoner, a radiology diagnosis MLLM designed to leverage process supervision mined directly from clinical reports, reflecting the step-by-step reasoning followed by radiologists. We construct a large dataset by extracting and refining reasoning chains from routine radiology reports. Our two-stage training framework combines supervised fine-tuning and reinforcement learning guided by process rewards to better align model reasoning with clinical standards. We introduce RadRBench-CXR, a comprehensive benchmark featuring 59K visual question answering samples with 301K clinically validated reasoning steps, and propose RadRScore, a metric evaluating reasoning factuality, completeness, and effectiveness. ChestX-Reasoner outperforms existing medical and general-domain MLLMs in both diagnostic accuracy and reasoning ability, achieving 16%, 5.9%, and 18% improvements in reasoning ability compared to the best medical MLLM, the best general MLLM, and its base model, respectively, as well as 3.3%, 24%, and 27% improvements in outcome accuracy. All resources are open-sourced to facilitate further research in medical reasoning MLLMs.
近期,推理增强的大型语言模型(LLM)和多模态LLM(MLLM)的进步在复杂任务中显著提升了性能,但医疗AI模型往往忽略了临床实践中的结构化推理过程。在这项工作中,我们提出了ChestX-Reasoner,这是一个用于放射学诊断的MLLM,旨在利用直接从临床报告中挖掘的过程监督,反映放射科医生遵循的逐步推理。我们通过从常规放射学报告中提取和精炼推理链来构建大型数据集。我们的两阶段训练框架结合了监督微调法和由过程奖励引导的深度强化学习,以更好地使模型推理与临床标准相符。我们引入了RadRBench-CXR,这是一个包含59K视觉问答样本和301K临床验证推理步骤的综合基准测试,并提出了RadRScore,这是一个评估推理真实性、完整性和有效性的指标。ChestX-Reasoner在诊断和治疗准确性方面优于现有的医疗和通用MLLM,在推理能力方面与最佳医疗MLLM、最佳通用MLLM和基准模型相比分别提高了16%、5.9%和18%,并在结果准确性方面分别提高了3.3%、24%和27%。所有资源均已开源,以促进医疗推理MLLM的进一步研究。
论文及项目相关链接
摘要
近期进展在推理增强的大型语言模型和多模态大型语言模型已经在复杂任务上表现出显著性能提升,但医疗人工智能模型常常忽略了临床实践中的结构化推理过程。本研究提出了ChestX-Reasoner,一个用于放射诊断的多模态大型语言模型,旨在利用直接从临床报告中挖掘的推理过程监督,反映放射科医生遵循的逐步推理。通过从常规放射报告中提取和精炼推理链,我们构建了一个大型数据集。我们的两阶段训练框架结合了监督微调与强化学习,以过程奖励为指导,使模型推理更好地符合临床标准。我们引入了RadRBench-CXR基准测试,包含59K视觉问答样本和301K临床验证的推理步骤,并提出了RadRScore评估指标,以评估推理的真实性、完整性和有效性。ChestX-Reasoner在诊断和推理能力上均优于现有的医疗和通用多模态大型语言模型,相较于最佳医疗多模态大型语言模型、最佳通用多模态大型语言模型及其基础模型,在推理能力上分别提高了16%、5.9%和18%,在结果准确性上分别提高了3.3%、24%和27%。所有资源均已开源,以促进医疗推理多模态大型语言模型的研究。
关键见解
- ChestX-Reasoner是一个针对放射诊断的多模态大型语言模型,结合临床实践中放射科医生的逐步推理过程。
- 通过从常规放射报告中提取和精炼推理链,构建了一个大型数据集。
- 采用两阶段训练框架,结合监督微调和强化学习,使模型推理更符合临床标准。
- 引入了RadRBench-CXR基准测试和RadRScore评估指标,用于评估模型的推理能力和准确性。
- ChestX-Reasoner在诊断和推理能力上显著优于现有医疗和通用多模态大型语言模型。
- 在推理能力和结果准确性方面实现了显著的改进。
点此查看论文截图
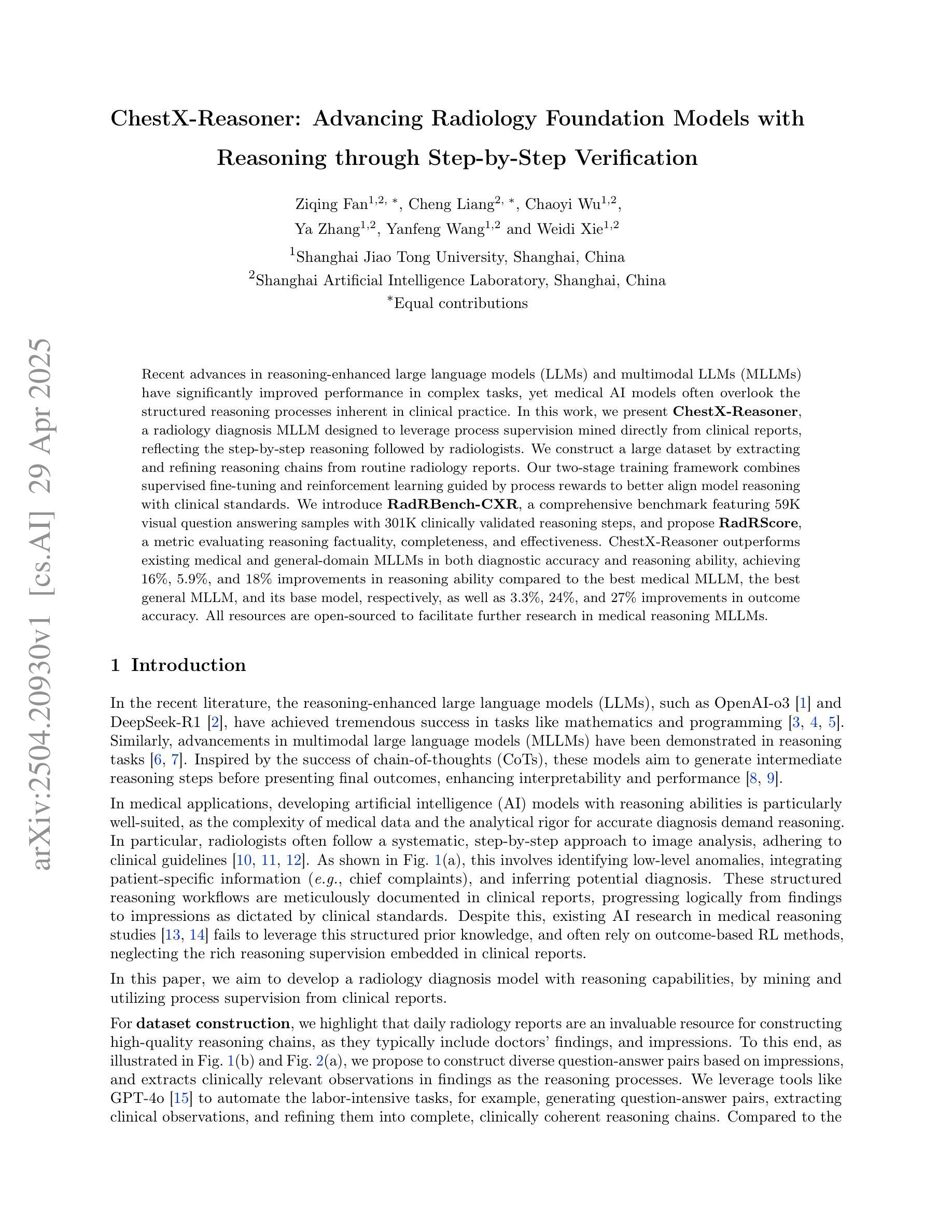
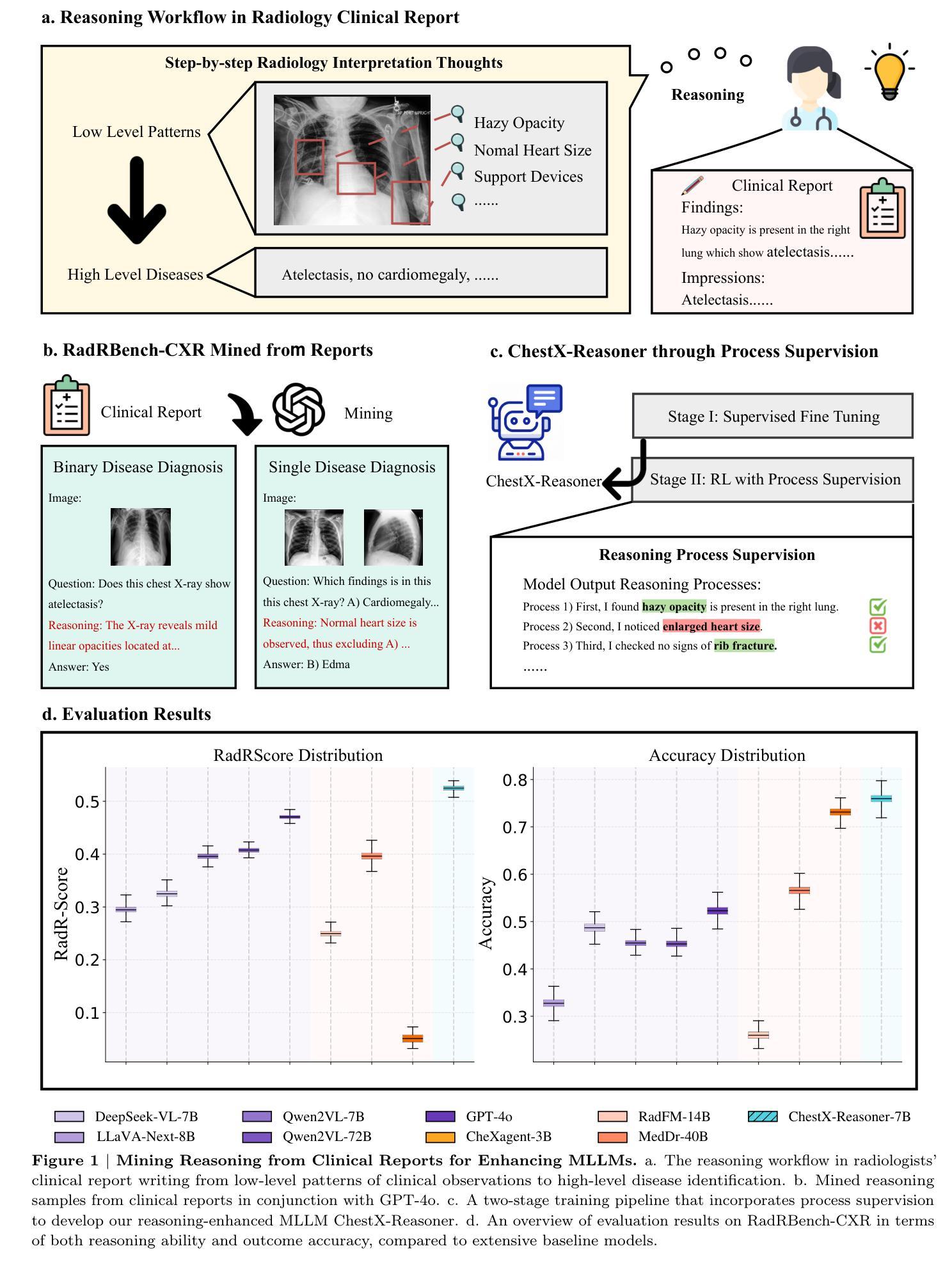
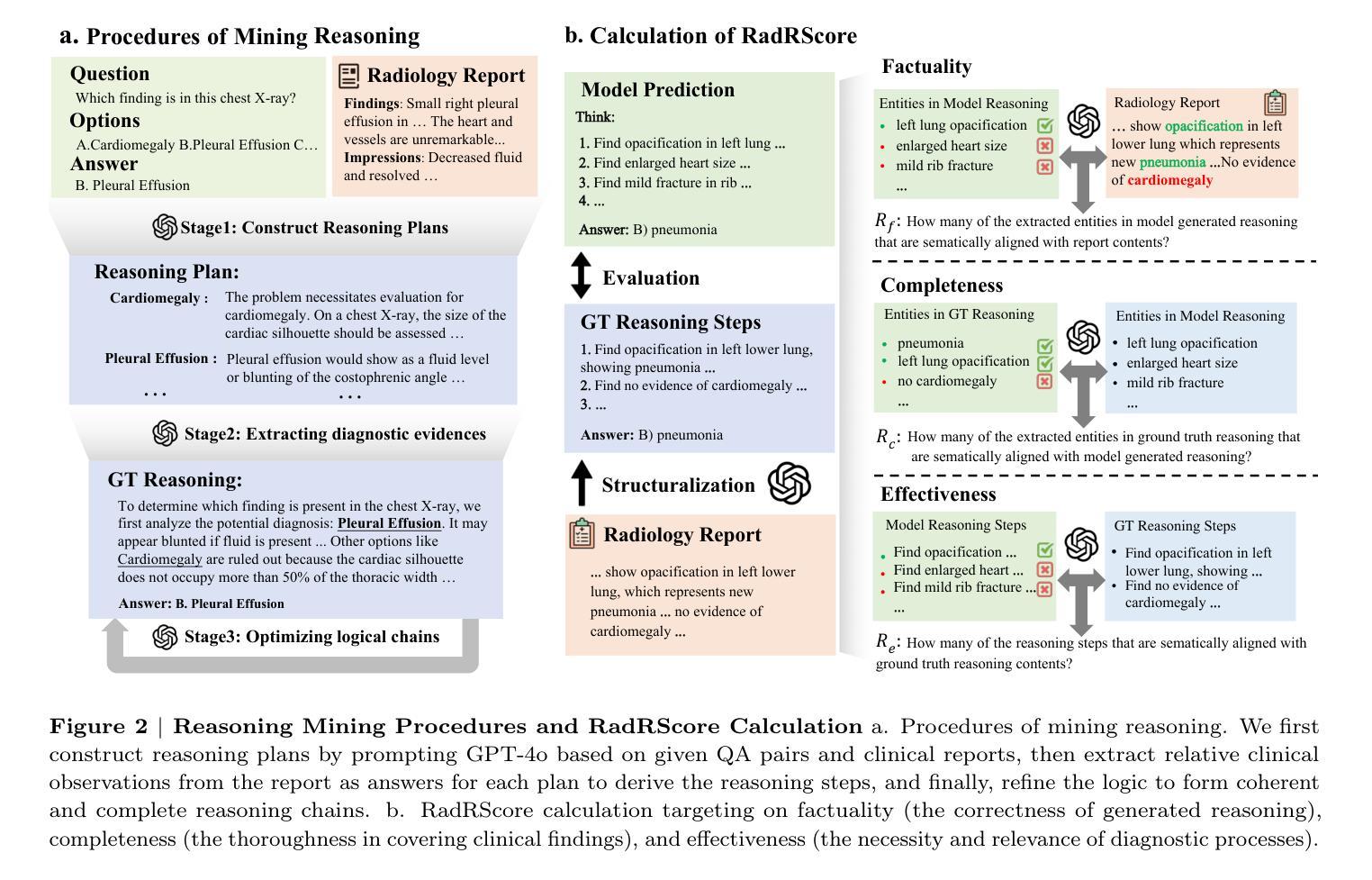
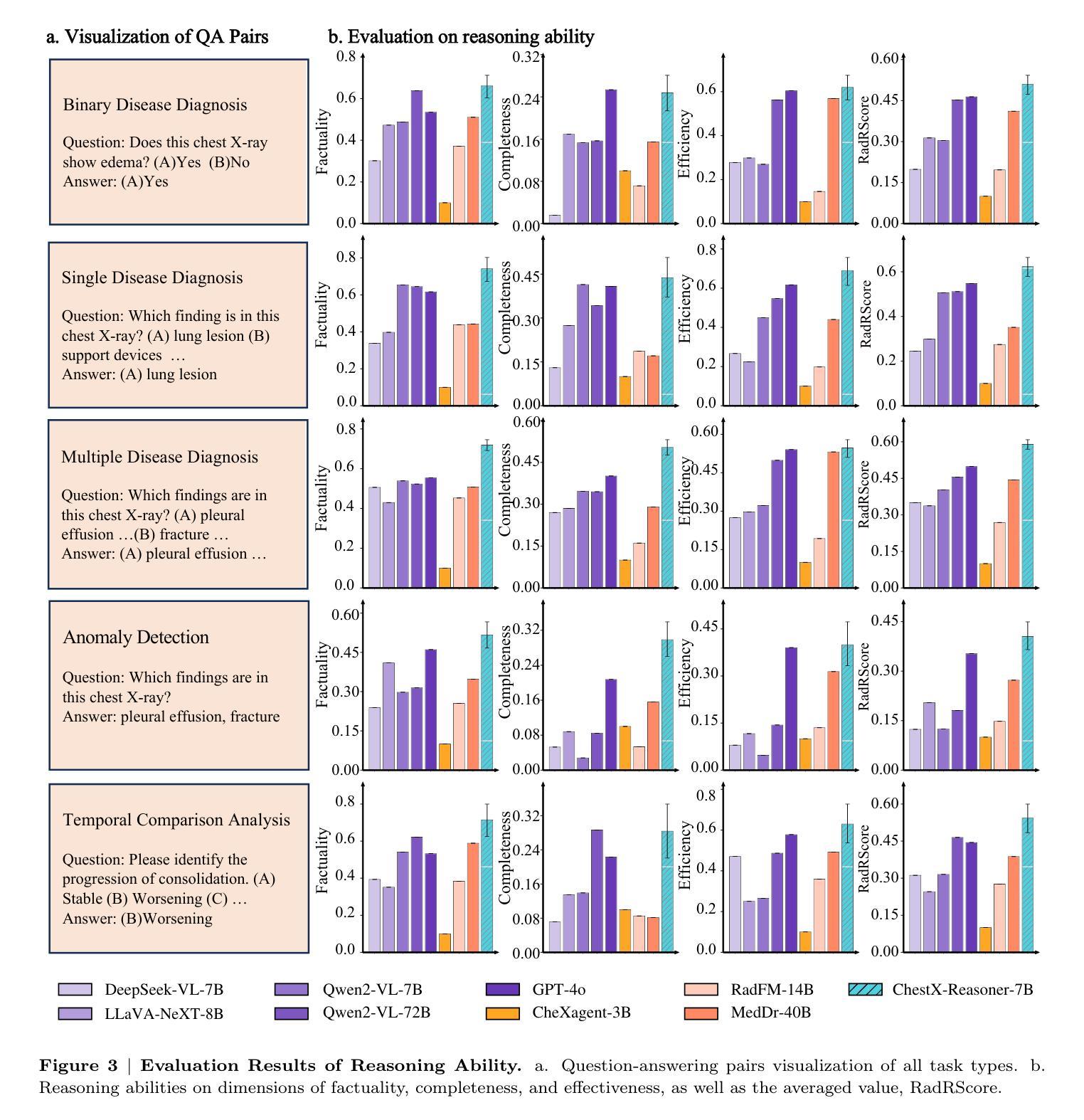
The Leaderboard Illusion
Authors:Shivalika Singh, Yiyang Nan, Alex Wang, Daniel D’Souza, Sayash Kapoor, Ahmet Üstün, Sanmi Koyejo, Yuntian Deng, Shayne Longpre, Noah Smith, Beyza Ermis, Marzieh Fadaee, Sara Hooker
Measuring progress is fundamental to the advancement of any scientific field. As benchmarks play an increasingly central role, they also grow more susceptible to distortion. Chatbot Arena has emerged as the go-to leaderboard for ranking the most capable AI systems. Yet, in this work we identify systematic issues that have resulted in a distorted playing field. We find that undisclosed private testing practices benefit a handful of providers who are able to test multiple variants before public release and retract scores if desired. We establish that the ability of these providers to choose the best score leads to biased Arena scores due to selective disclosure of performance results. At an extreme, we identify 27 private LLM variants tested by Meta in the lead-up to the Llama-4 release. We also establish that proprietary closed models are sampled at higher rates (number of battles) and have fewer models removed from the arena than open-weight and open-source alternatives. Both these policies lead to large data access asymmetries over time. Providers like Google and OpenAI have received an estimated 19.2% and 20.4% of all data on the arena, respectively. In contrast, a combined 83 open-weight models have only received an estimated 29.7% of the total data. We show that access to Chatbot Arena data yields substantial benefits; even limited additional data can result in relative performance gains of up to 112% on the arena distribution, based on our conservative estimates. Together, these dynamics result in overfitting to Arena-specific dynamics rather than general model quality. The Arena builds on the substantial efforts of both the organizers and an open community that maintains this valuable evaluation platform. We offer actionable recommendations to reform the Chatbot Arena’s evaluation framework and promote fairer, more transparent benchmarking for the field
进展测量是任何科学领域发展的基础。随着基准测试的作用越来越重要,它们也更容易受到扭曲。聊天机器人竞技场已经涌现为排名最先进的人工智能系统的首选排行榜。然而,在这项工作中,我们发现了导致竞技环境扭曲的系统性问题。我们发现,未公开的私人测试实践使少数提供者受益,这些提供者能够在公共发布之前测试多个变体,并在需要时收回分数。我们证实,这些提供者选择最佳分数的能力会导致竞技场得分偏向,因为性能结果是选择性披露的。在极端情况下,我们确定了Meta在Llama-4发布前测试的27个私人大型语言模型变体。我们还发现专有封闭模型的采样率(战斗次数)更高,与公开权重和开源替代方案相比,从竞技场移除的模型更少。这两种政策都导致了随时间推移的数据访问不对称。谷歌和OpenAI等提供商分别获得了估计的19.2%和20.4%的所有数据。相比之下,83个公开权重模型仅获得了估计的29.7%的总数据。我们表明,访问聊天机器人竞技场数据带来巨大的好处;即使有限的额外数据也可能导致基于保守估计的竞技场分布上的相对性能提升高达112%。总的来说,这些动态导致对竞技场特定动态的过度拟合,而不是一般的模型质量。竞技场建立在组织者和一个维护这个宝贵评估平台的开放社区的大量努力之上。我们提供切实可行的建议,以改革聊天机器人竞技场的评估框架,促进该领域更公平、更透明的基准测试。
论文及项目相关链接
PDF 68 pages, 18 figures, 9 tables
Summary
该文本讨论了聊天机器人竞技场(Chatbot Arena)在评估人工智能系统方面存在的问题。发现私有测试实践、选择性披露性能结果、数据访问不对称等问题导致竞技场评价失真。提出改革评价框架,促进更公平、透明的基准测试。
Key Takeaways
- 聊天机器人竞技场(Chatbot Arena)作为AI系统的排名榜,存在系统性的问题,导致评价失真。
- 未经公布的私人测试实践使得少数提供者能够在公开发布前测试多个变体,并选择性撤回分数,导致竞技场评分偏差。
- 某些提供者选择最佳分数的能力,因选择性披露性能结果,导致竞技场评分不公平。
- 存在极端情况,如Meta在Llama-4发布前测试了27个私有大型语言模型(LLM)变体。
- 专有封闭模型被采样的频率更高,从竞技场中移除的模型更少,导致数据访问不对称。
- 对聊天机器人竞技场数据的访问带来了巨大的好处;即使有限额外的数据也可能导致竞技场分布的相对性能提高112%。
点此查看论文截图
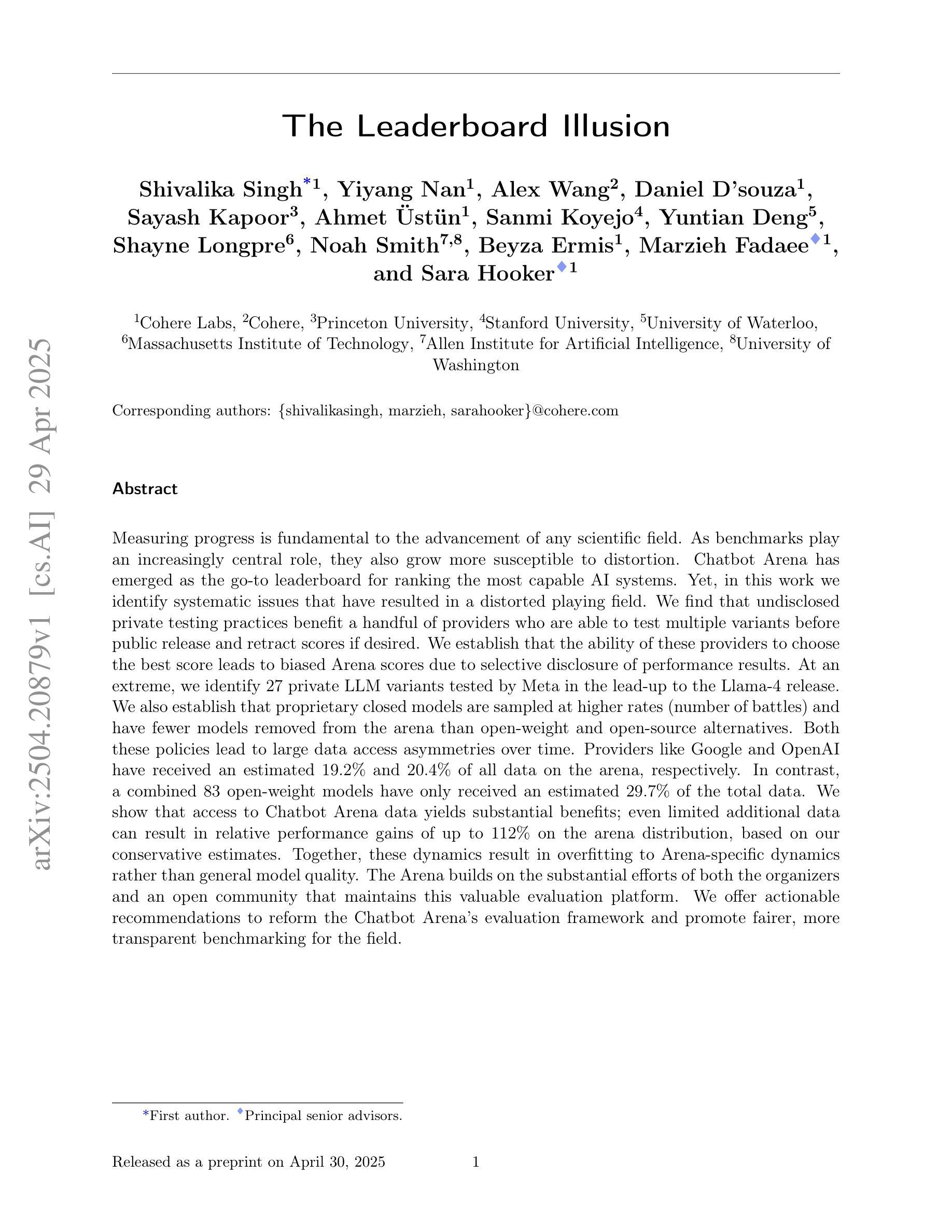
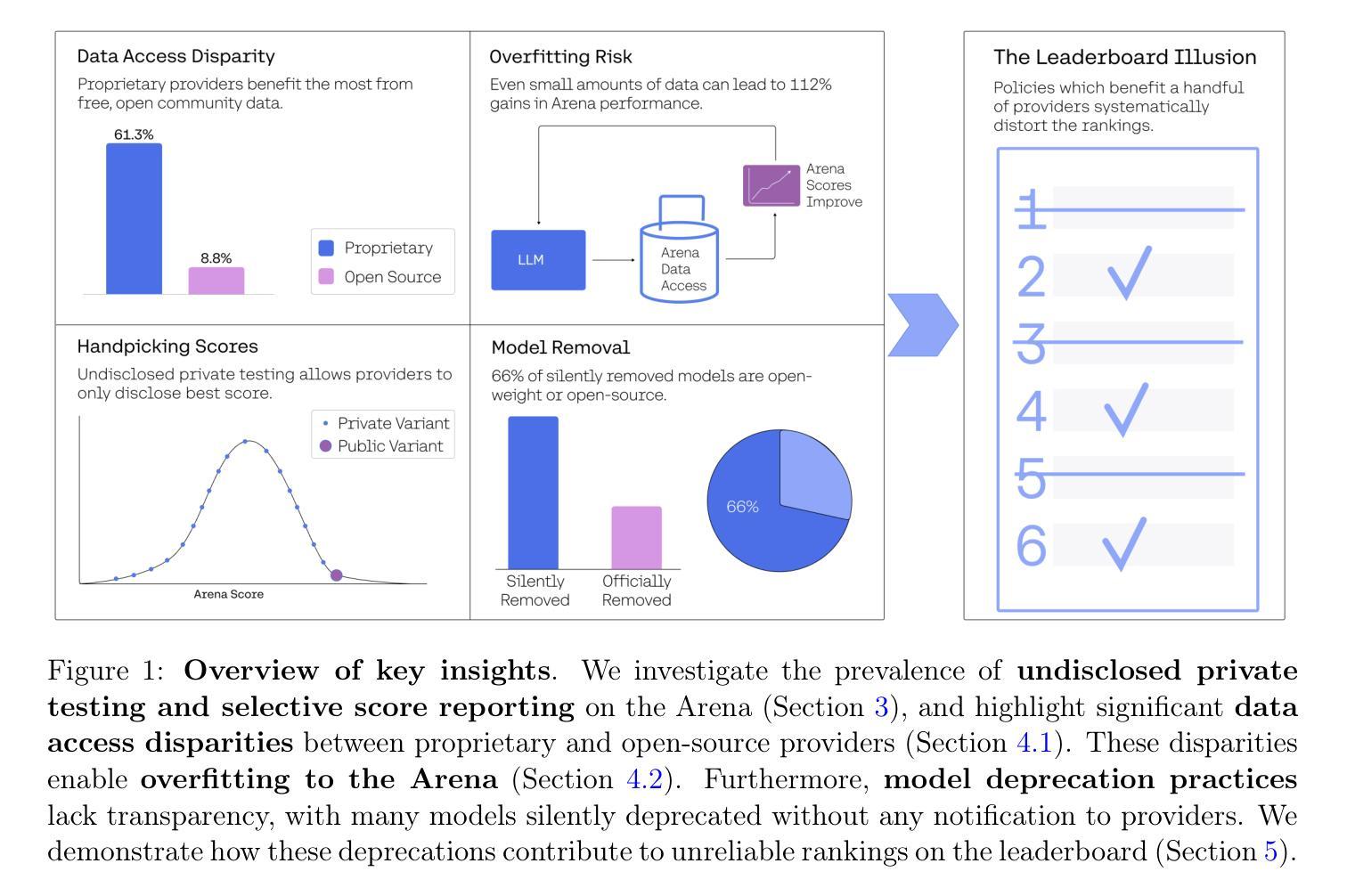
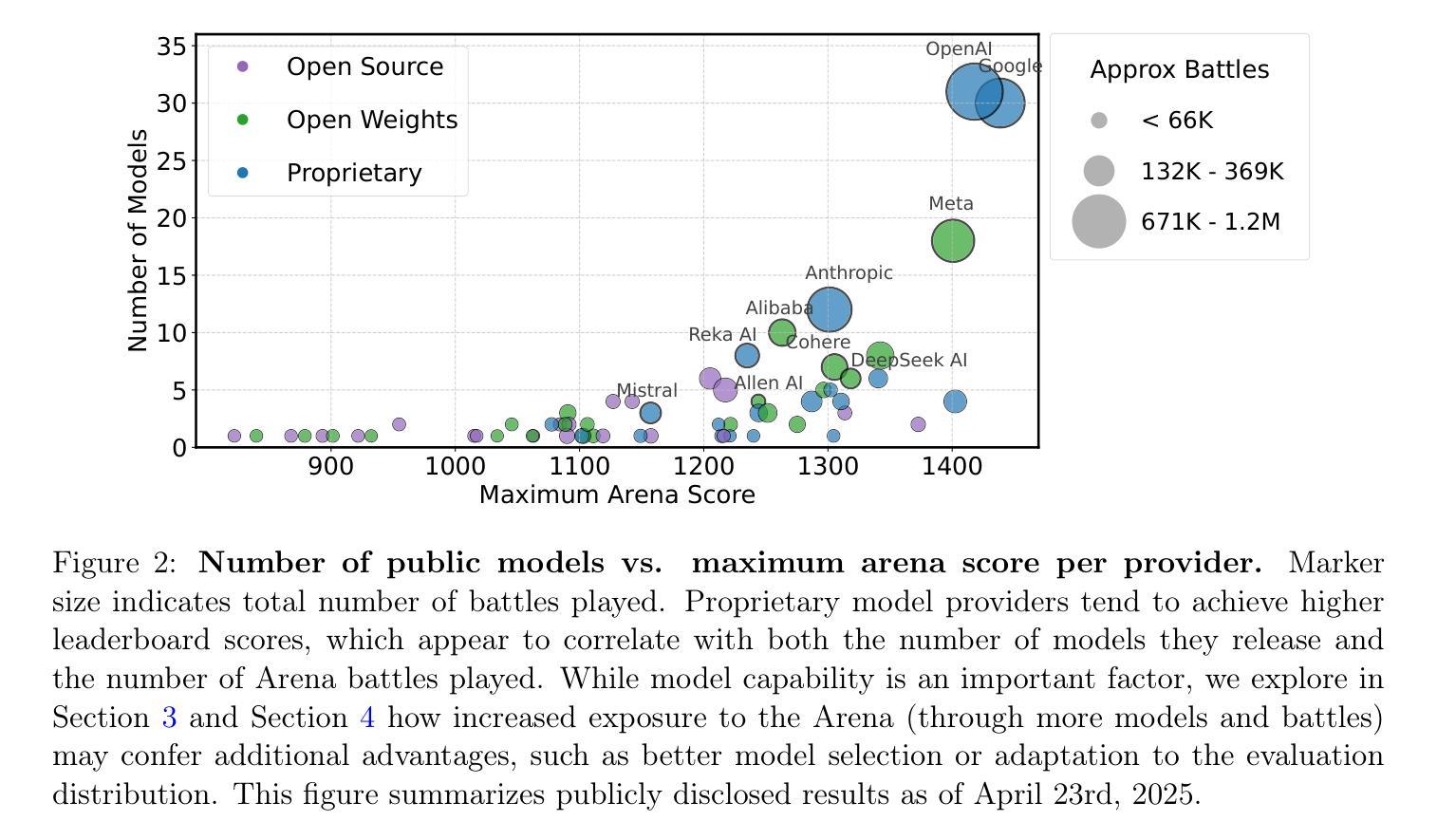
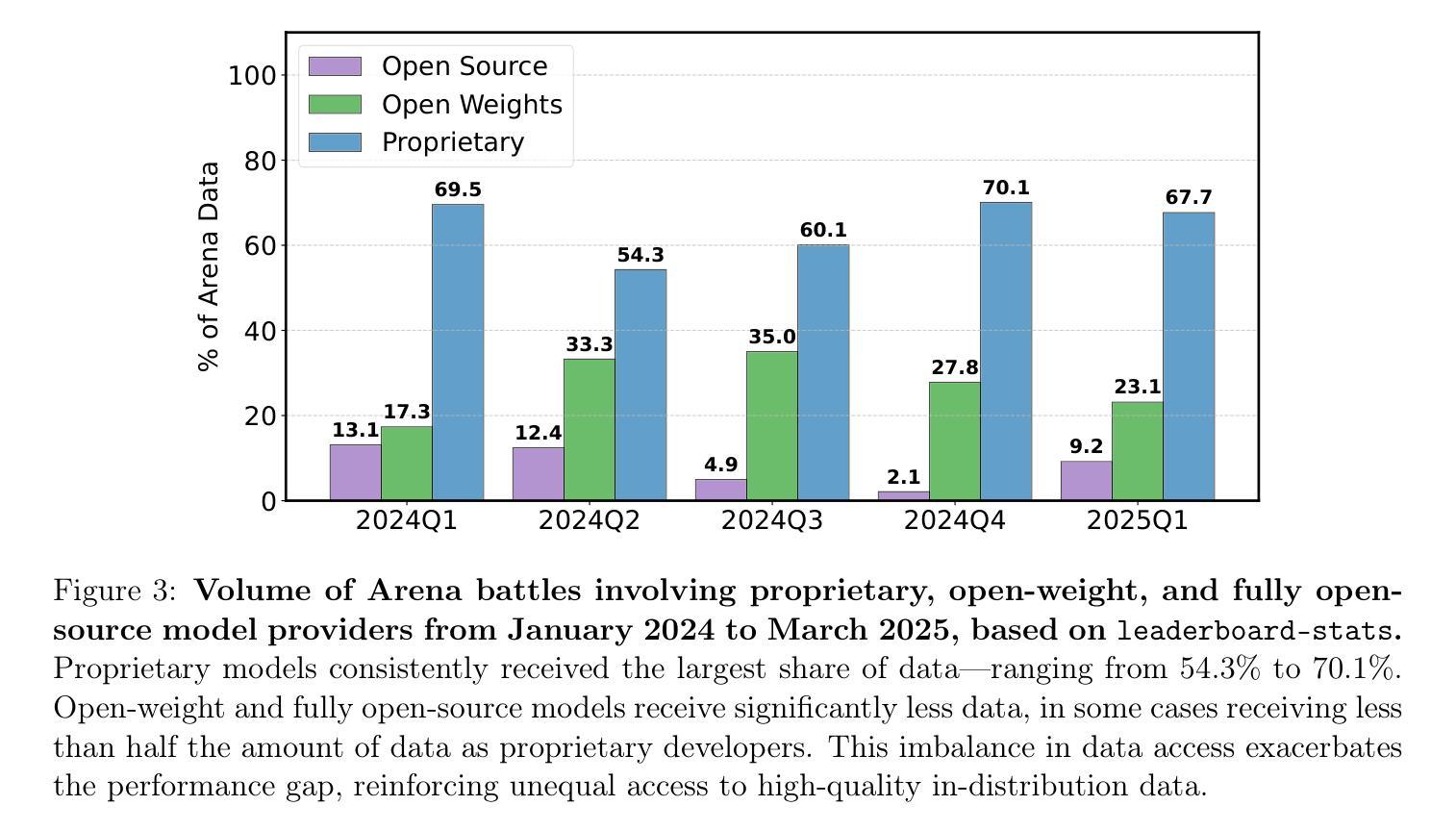
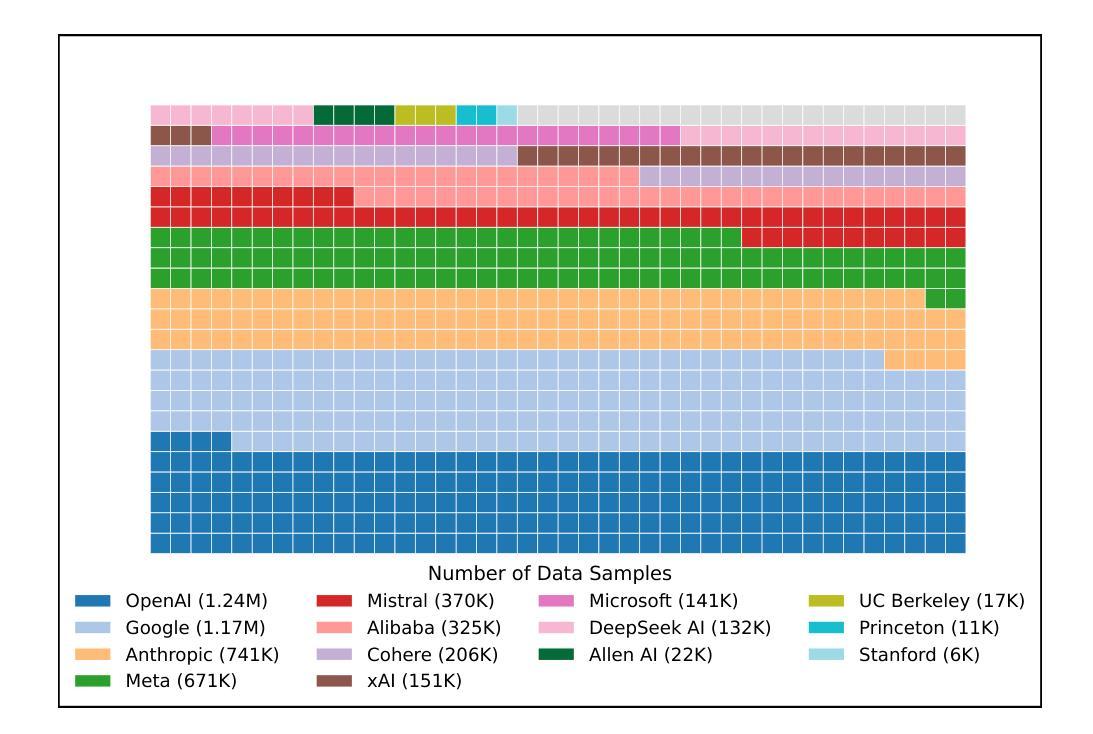
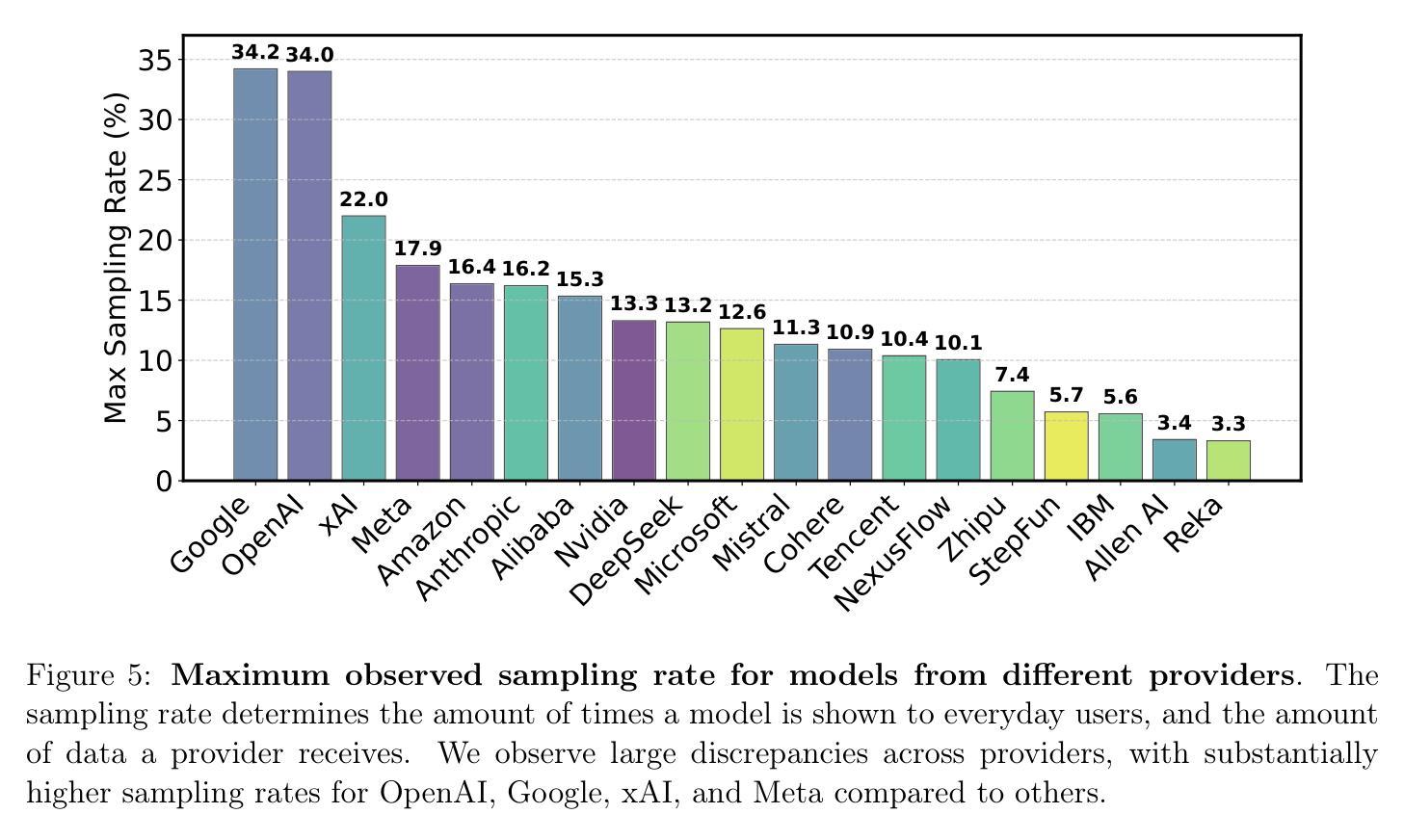
AI-GenBench: A New Ongoing Benchmark for AI-Generated Image Detection
Authors:Lorenzo Pellegrini, Davide Cozzolino, Serafino Pandolfini, Davide Maltoni, Matteo Ferrara, Luisa Verdoliva, Marco Prati, Marco Ramilli
The rapid advancement of generative AI has revolutionized image creation, enabling high-quality synthesis from text prompts while raising critical challenges for media authenticity. We present Ai-GenBench, a novel benchmark designed to address the urgent need for robust detection of AI-generated images in real-world scenarios. Unlike existing solutions that evaluate models on static datasets, Ai-GenBench introduces a temporal evaluation framework where detection methods are incrementally trained on synthetic images, historically ordered by their generative models, to test their ability to generalize to new generative models, such as the transition from GANs to diffusion models. Our benchmark focuses on high-quality, diverse visual content and overcomes key limitations of current approaches, including arbitrary dataset splits, unfair comparisons, and excessive computational demands. Ai-GenBench provides a comprehensive dataset, a standardized evaluation protocol, and accessible tools for both researchers and non-experts (e.g., journalists, fact-checkers), ensuring reproducibility while maintaining practical training requirements. By establishing clear evaluation rules and controlled augmentation strategies, Ai-GenBench enables meaningful comparison of detection methods and scalable solutions. Code and data are publicly available to ensure reproducibility and to support the development of robust forensic detectors to keep pace with the rise of new synthetic generators.
生成式人工智能的快速进步已经彻底改变了图像创作的方式,它能够通过文本提示进行高质量合成,同时为媒体真实性带来了重大挑战。我们推出了Ai-GenBench,这是一个新型基准测试,旨在解决现实场景中检测AI生成图像的需求。与在静态数据集上评估模型的现有解决方案不同,Ai-GenBench引入了一个临时评估框架,该框架对检测方法进行增量训练,在按生成模型历史顺序合成的图像上进行,以测试其适应新生成模型的能力,例如从生成对抗网络(GANs)到扩散模型的过渡。我们的基准测试专注于高质量、多样化的视觉内容,并克服了当前方法的关键局限性,包括任意的数据集分割、不公平的比较和过高的计算需求。Ai-GenBench为研究人员和非专家(例如记者、事实核查人员)提供了一个综合数据集、标准化的评估协议和可访问的工具,确保了可重复性,同时保持了实用的培训要求。通过制定明确的评估规则和受控的增强策略,Ai-GenBench使得检测方法的比较和可扩展解决方案变得更有意义。代码和数据公开可用,以确保可重复性并支持开发稳健的取证检测器,以跟上新的合成生成器的步伐。
论文及项目相关链接
PDF 9 pages, 6 figures, 4 tables, code available: https://github.com/MI-BioLab/AI-GenBench
Summary
随着生成式AI的快速发展,图像创建领域经历了革命性的变革,这既使得文本提示能够生成高质量图像,同时也对媒体真实性提出了严峻挑战。为此,我们推出了Ai-GenBench,这是一个新型基准测试平台,旨在解决现实场景中检测AI生成图像的需求。与现有仅在静态数据集上评估模型的解决方案不同,Ai-GenBench采用了一种临时评估框架,该框架按生成模型的顺序对合成图像进行历史排序,以测试检测方法的逐步训练能力,旨在评估其适应新生成模型的能力,例如从GAN到扩散模型的转变。我们的基准测试重点关注高质量、多样化的视觉内容,并解决了当前方法的关键局限性,包括任意数据集分割、不公平比较和过高的计算需求。Ai-GenBench提供了一个综合数据集、标准化的评估协议以及方便研究者和非专家(如记者、事实核查人员)使用的工具,确保实用性和可重复性。通过制定明确的评估规则和受控的增强策略,Ai-GenBench为实现检测方法的有意义比较和可扩展解决方案提供了可能。代码和数据均公开提供,以确保可重复性和支持开发可靠的取证检测器以适应不断出现的新型合成生成器。
Key Takeaways
- 生成式AI的快速发展推动了图像创建领域的变革。
- Ai-GenBench是一个新型基准测试平台,旨在解决现实场景中检测AI生成图像的需求。
- Ai-GenBench采用临时评估框架来测试检测方法的逐步训练能力。
- 该平台关注高质量、多样化的视觉内容。
- Ai-GenBench解决了当前图像检测方法的多个关键局限性。
- Ai-GenBench为研究者和非专家提供了综合数据集、标准化的评估协议和方便使用的工具。
点此查看论文截图
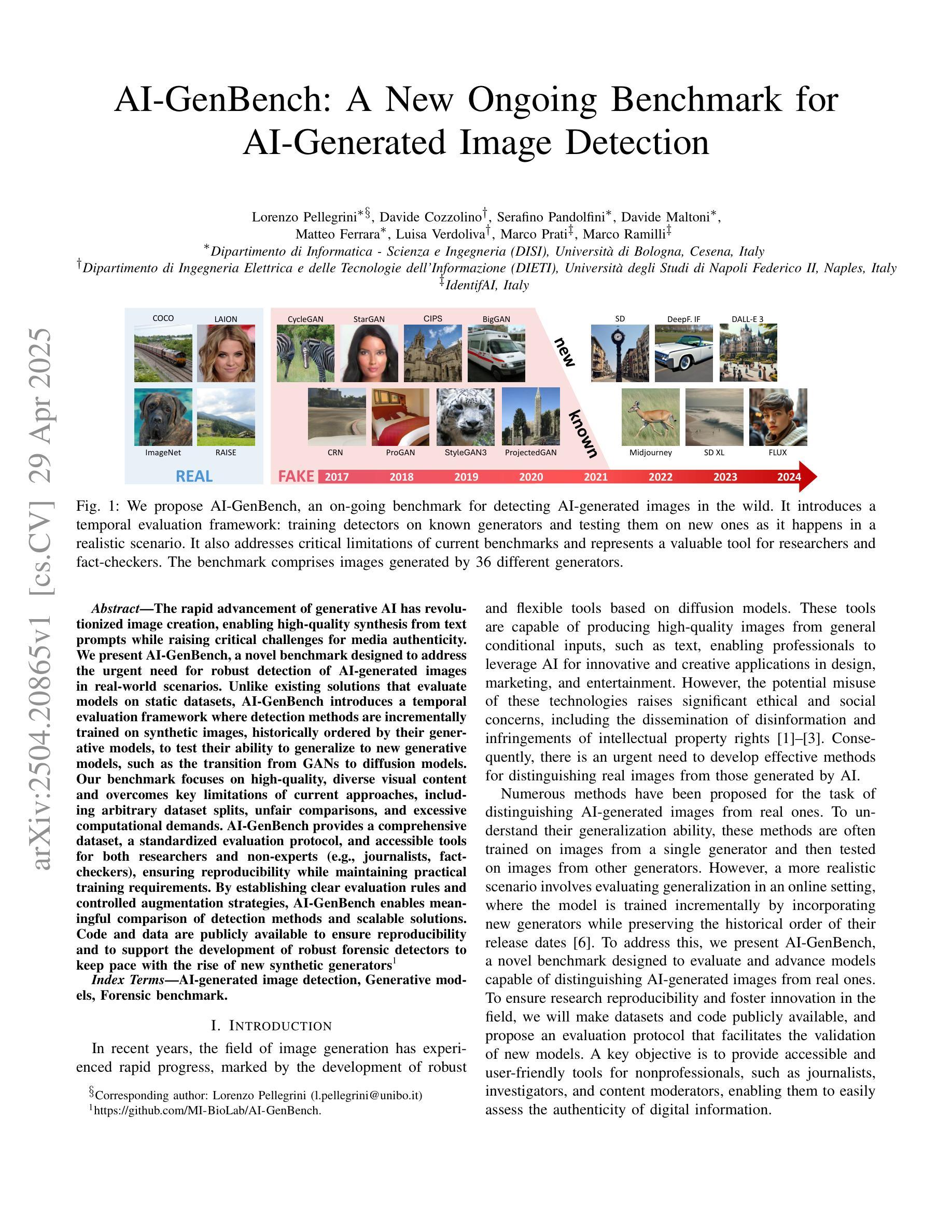
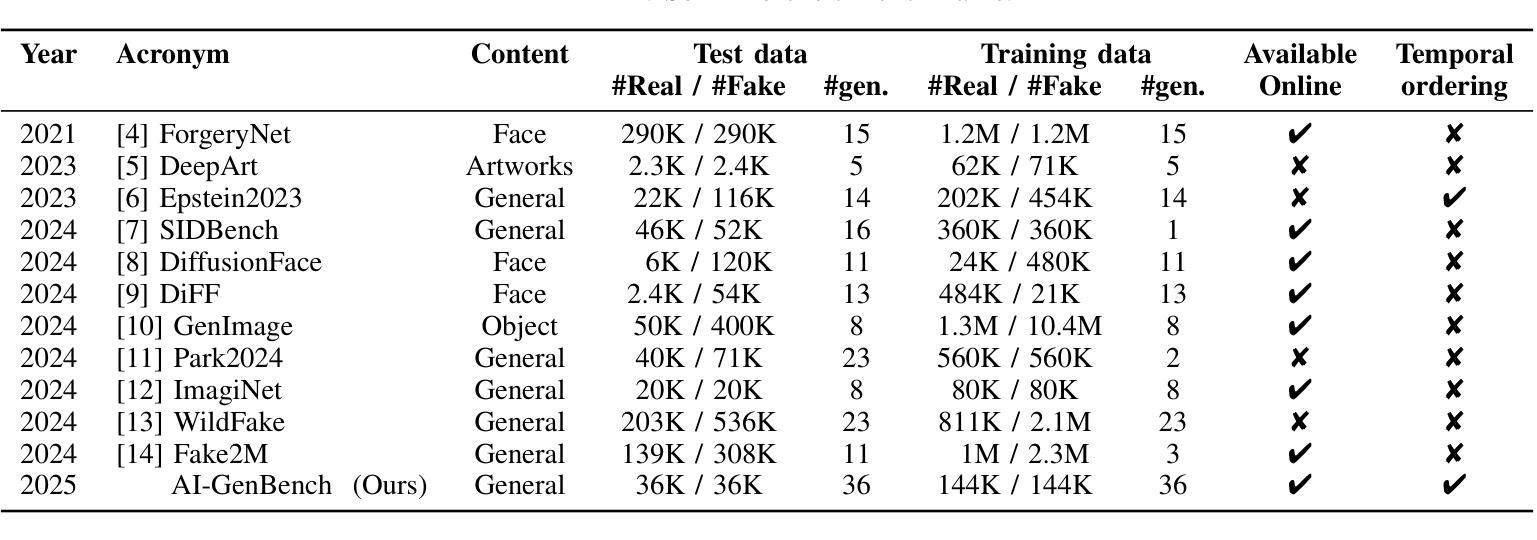
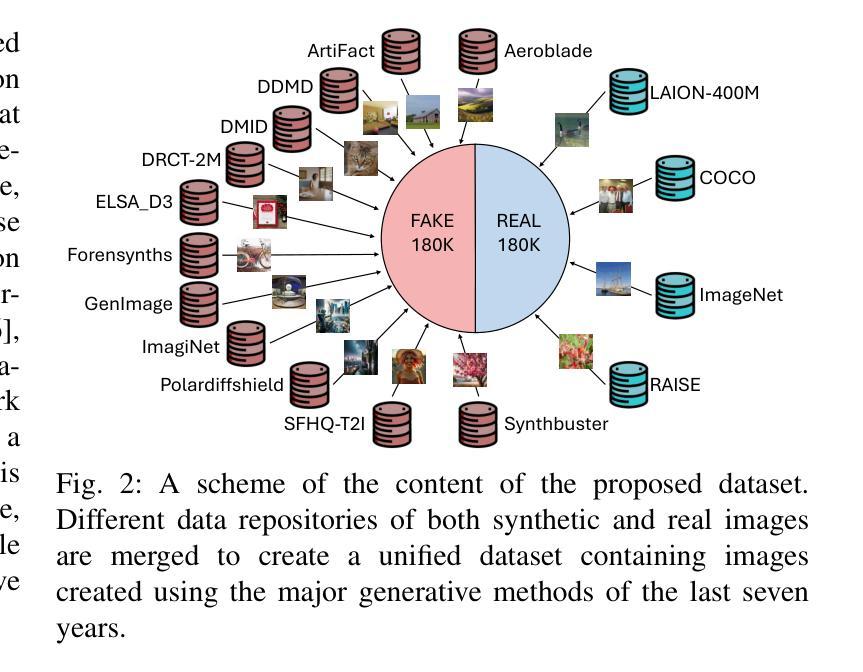
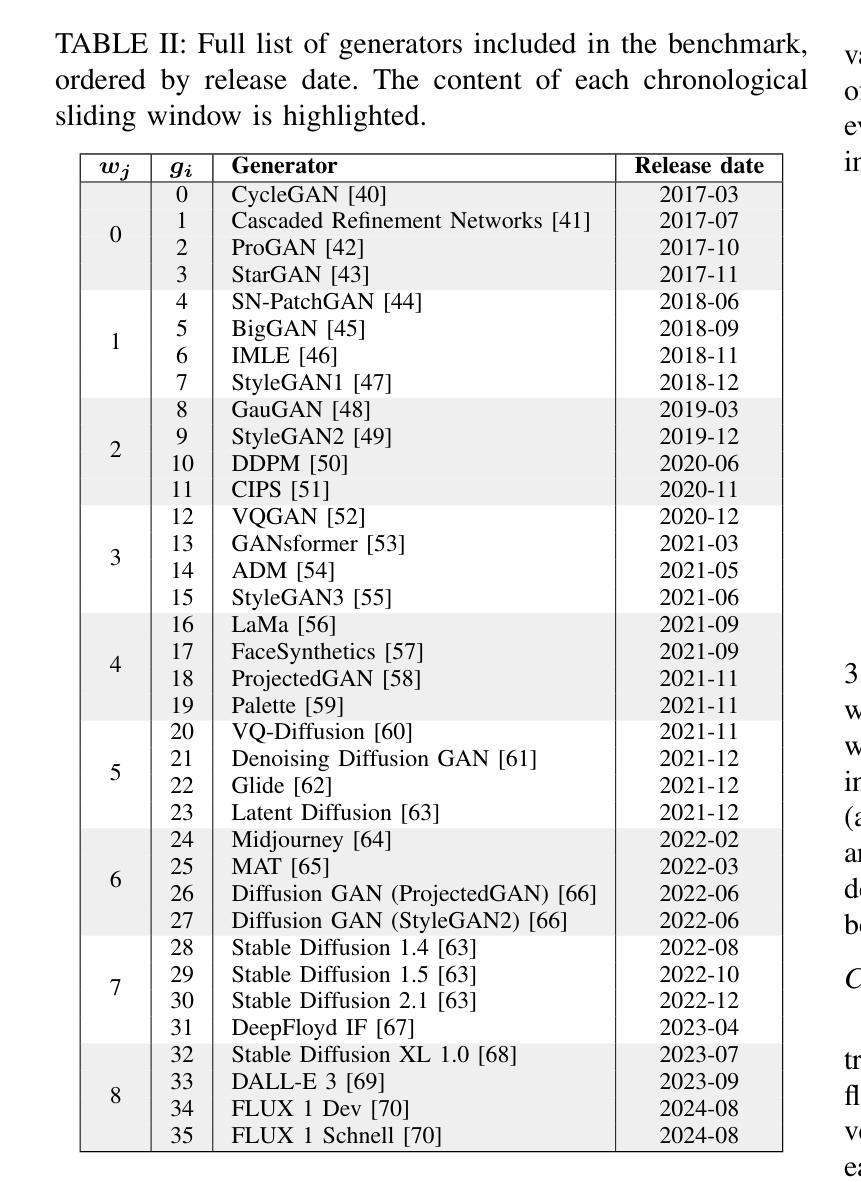
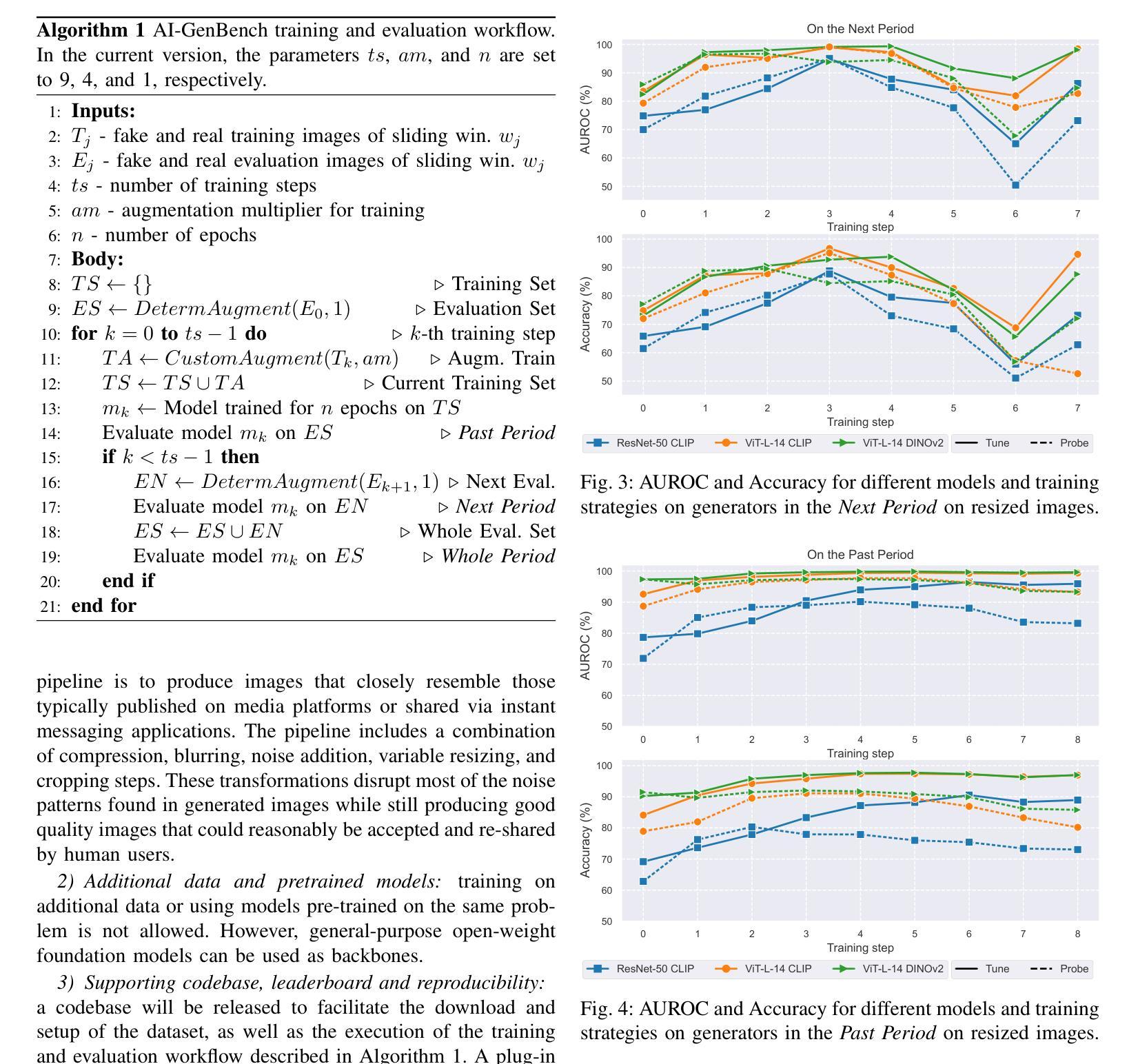
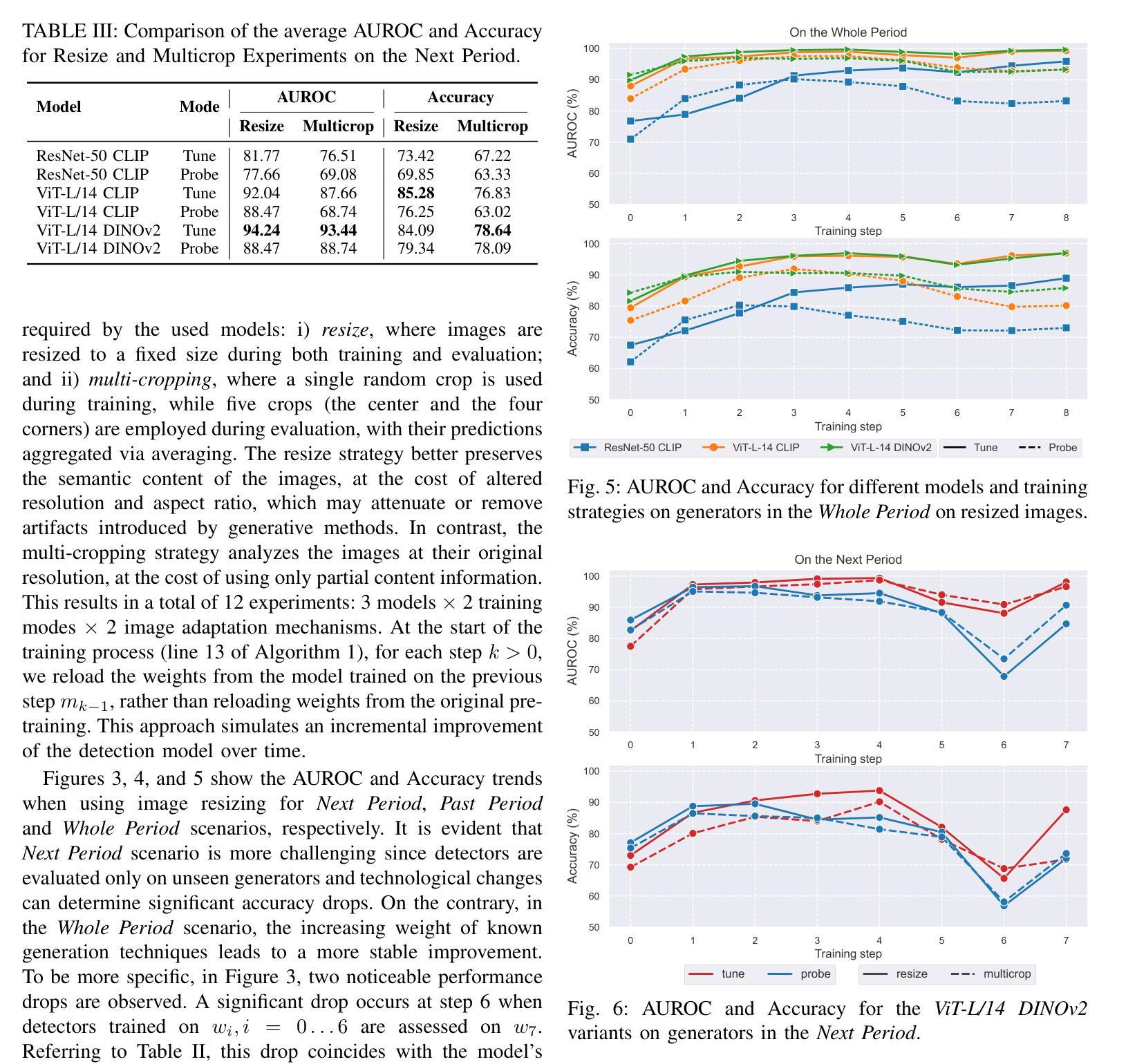
Enhancing Non-Core Language Instruction-Following in Speech LLMs via Semi-Implicit Cross-Lingual CoT Reasoning
Authors:Hongfei Xue, Yufeng Tang, Hexin Liu, Jun Zhang, Xuelong Geng, Lei Xie
Large language models have been extended to the speech domain, leading to the development of speech large language models (SLLMs). While existing SLLMs demonstrate strong performance in speech instruction-following for core languages (e.g., English), they often struggle with non-core languages due to the scarcity of paired speech-text data and limited multilingual semantic reasoning capabilities. To address this, we propose the semi-implicit Cross-lingual Speech Chain-of-Thought (XS-CoT) framework, which integrates speech-to-text translation into the reasoning process of SLLMs. The XS-CoT generates four types of tokens: instruction and response tokens in both core and non-core languages, enabling cross-lingual transfer of reasoning capabilities. To mitigate inference latency in generating target non-core response tokens, we incorporate a semi-implicit CoT scheme into XS-CoT, which progressively compresses the first three types of intermediate reasoning tokens while retaining global reasoning logic during training. By leveraging the robust reasoning capabilities of the core language, XS-CoT improves responses for non-core languages by up to 45% in GPT-4 score when compared to direct supervised fine-tuning on two representative SLLMs, Qwen2-Audio and SALMONN. Moreover, the semi-implicit XS-CoT reduces token delay by more than 50% with a slight drop in GPT-4 scores. Importantly, XS-CoT requires only a small amount of high-quality training data for non-core languages by leveraging the reasoning capabilities of core languages. To support training, we also develop a data pipeline and open-source speech instruction-following datasets in Japanese, German, and French.
大型语言模型已扩展到语音领域,从而催生了语音大型语言模型(SLLM)的发展。虽然现有的SLLM在核心语言(如英语)的语音指令执行方面表现出强大的性能,但由于缺乏配套的语音文本数据和有限的跨语言语义推理能力,它们往往在非核心语言上表现挣扎。为了解决这个问题,我们提出了半隐式跨语言语音思维链(XS-CoT)框架,它将语音到文本的翻译整合到SLLM的推理过程中。XS-CoT生成四种类型的符号:核心语言和非核心语言中的指令和响应符号,实现跨语言推理能力的迁移。为了减轻生成目标非核心响应符号时的推理延迟,我们在XS-CoT中融入了半隐式CoT方案,该方案在训练过程中逐步压缩前三种中间推理符号,同时保留全局推理逻辑。通过利用核心语言的稳健推理能力,XS-CoT在与其他两种具有代表性的SLLM模型Qwen2-Audio和SALMONN进行对比时,对非核心语言的响应提高了高达GPT-4分数的45%。此外,半隐式的XS-CoT减少了超过一半的符号延迟,同时GPT-4分数略有下降。重要的是,XS-CoT只需要利用核心语言的推理能力,为少量高质量的非核心语言训练数据提供支持。为了支持训练,我们还开发了一个数据管道,并公开了日语、德语和法语等语音指令执行数据集。
论文及项目相关链接
PDF 10 pages, 6 figures, Submitted to ACM MM 2025
Summary
大型语言模型已扩展到语音领域,导致语音大型语言模型(SLLM)的发展。现有SLLM在核心语言(如英语)的语音指令执行方面表现良好,但在非核心语言方面由于缺少配套的语音文本数据和有限的跨语言语义推理能力而经常遇到困难。为解决这一问题,提出了半隐式跨语言语音思维链(XS-CoT)框架,将语音到文本的翻译融入SLLM的推理过程。XS-CoT生成四种类型的符号:核心和非核心语言中的指令和响应符号,实现跨语言推理能力的转移。为减少生成目标非核心响应符号的推理延迟,我们在XS-CoT中纳入了半隐式CoT方案,该方案在训练过程中逐步压缩前三种类型的中间推理符号,同时保留全局推理逻辑。通过利用核心语言的稳健推理能力,XS-CoT在对比两个代表性SLLM模型Qwen2-Audio和SALMONN时,对非核心语言的响应提高了45%。此外,半隐式的XS-CoT将符号延迟减少了超过50%,同时GPT-4得分略有下降。XS-CoT只需要少量高质量的非核心语言训练数据,通过利用核心语言的推理能力即可支持训练。我们还开发了一个数据管道,并公开了日语、德语和法语的声音指令跟随数据集。
Key Takeaways
- 大型语言模型已扩展到语音领域,但非核心语言的语音指令执行存在挑战。
- XS-CoT框架通过集成语音到文本的翻译,增强了SLLM的跨语言推理能力。
- XS-CoT生成四种类型的符号,实现跨语言能力的转移。
- 半隐式CoT方案减少了生成非核心语言响应的推理延迟。
- XS-CoT提高了非核心语言的响应能力,与直接监督微调相比,GPT-4得分提高了45%。
- 半隐式XS-CoT降低了符号延迟,同时略微降低了GPT-4得分。
点此查看论文截图
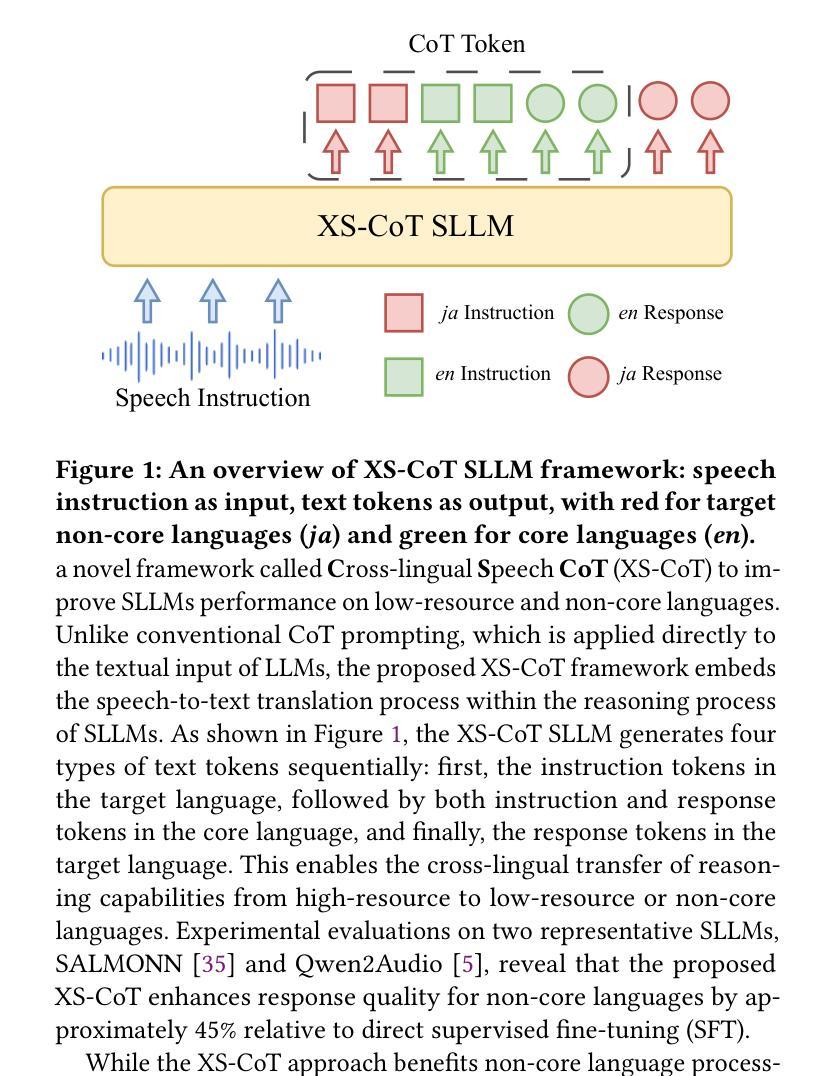
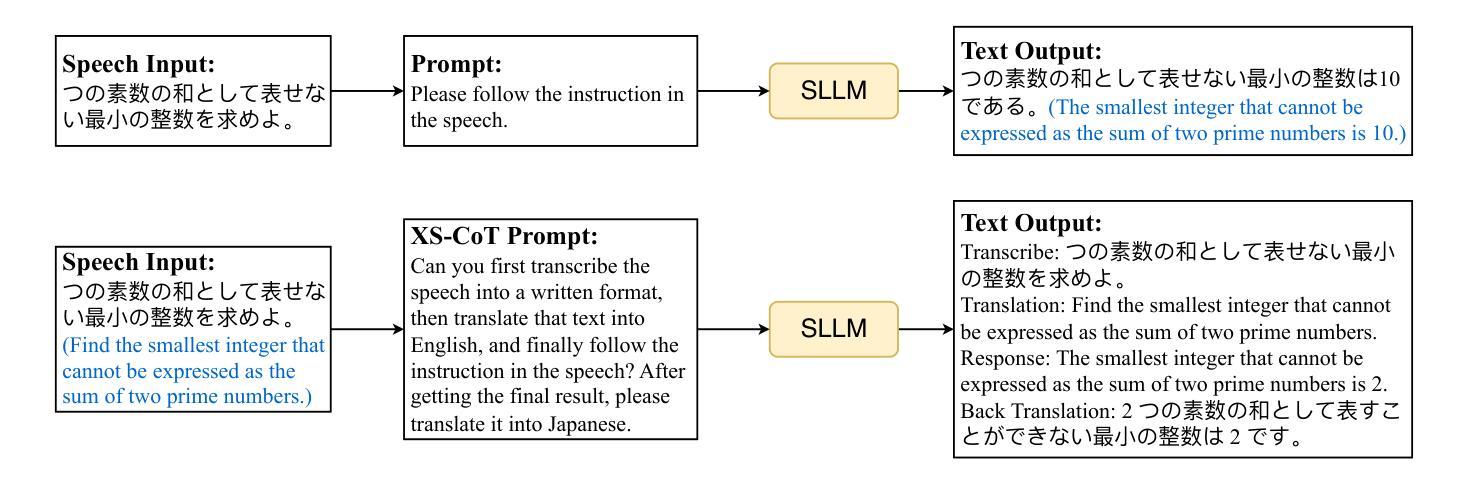
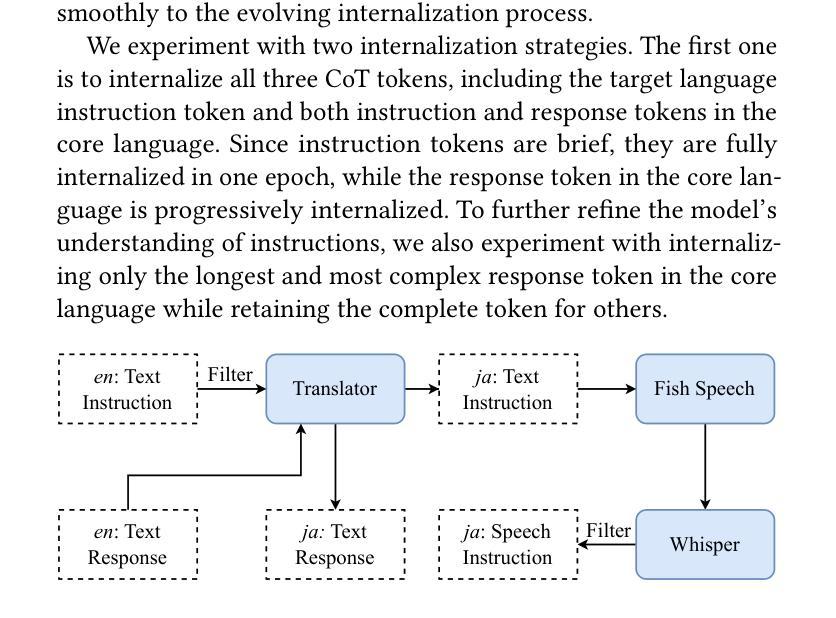
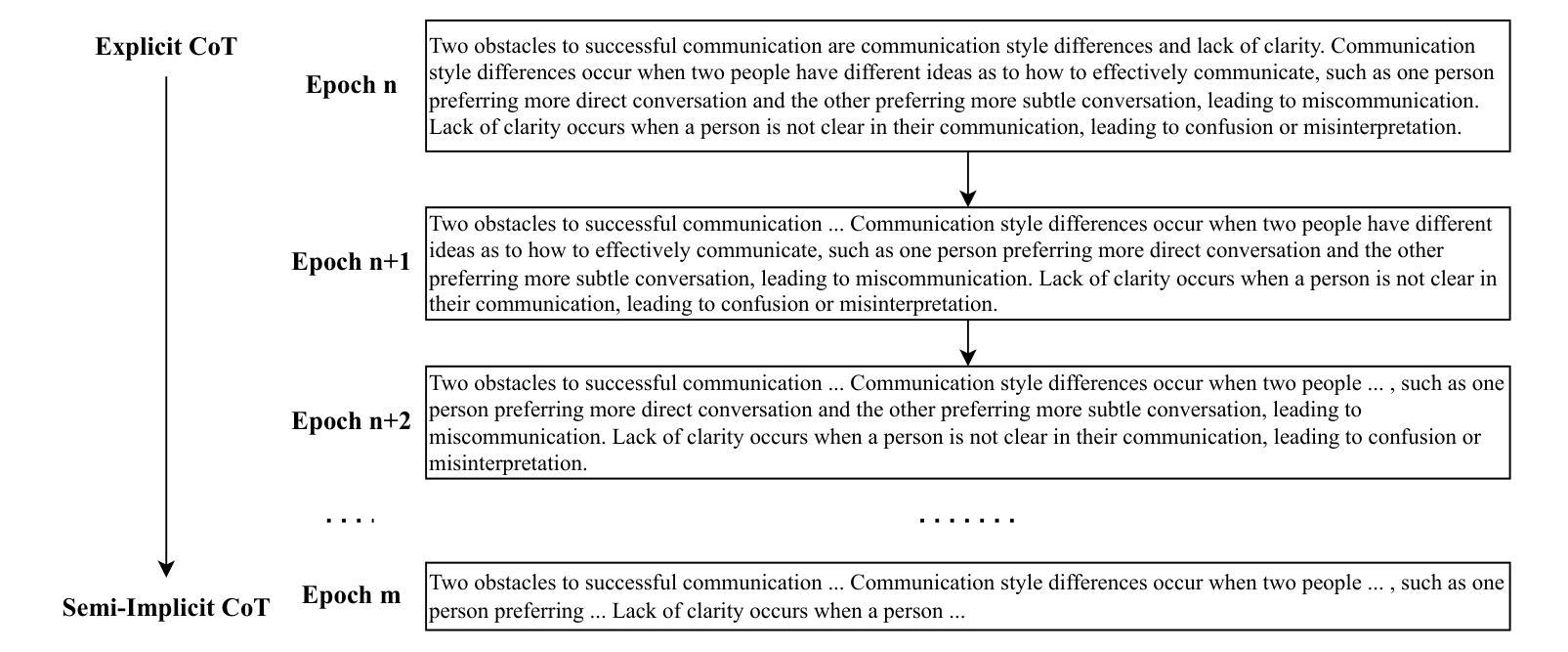
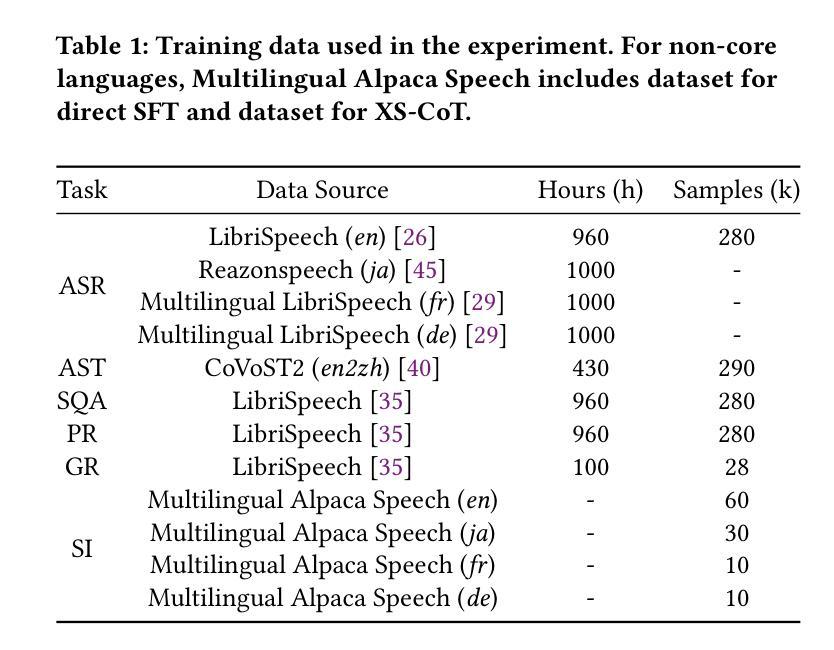
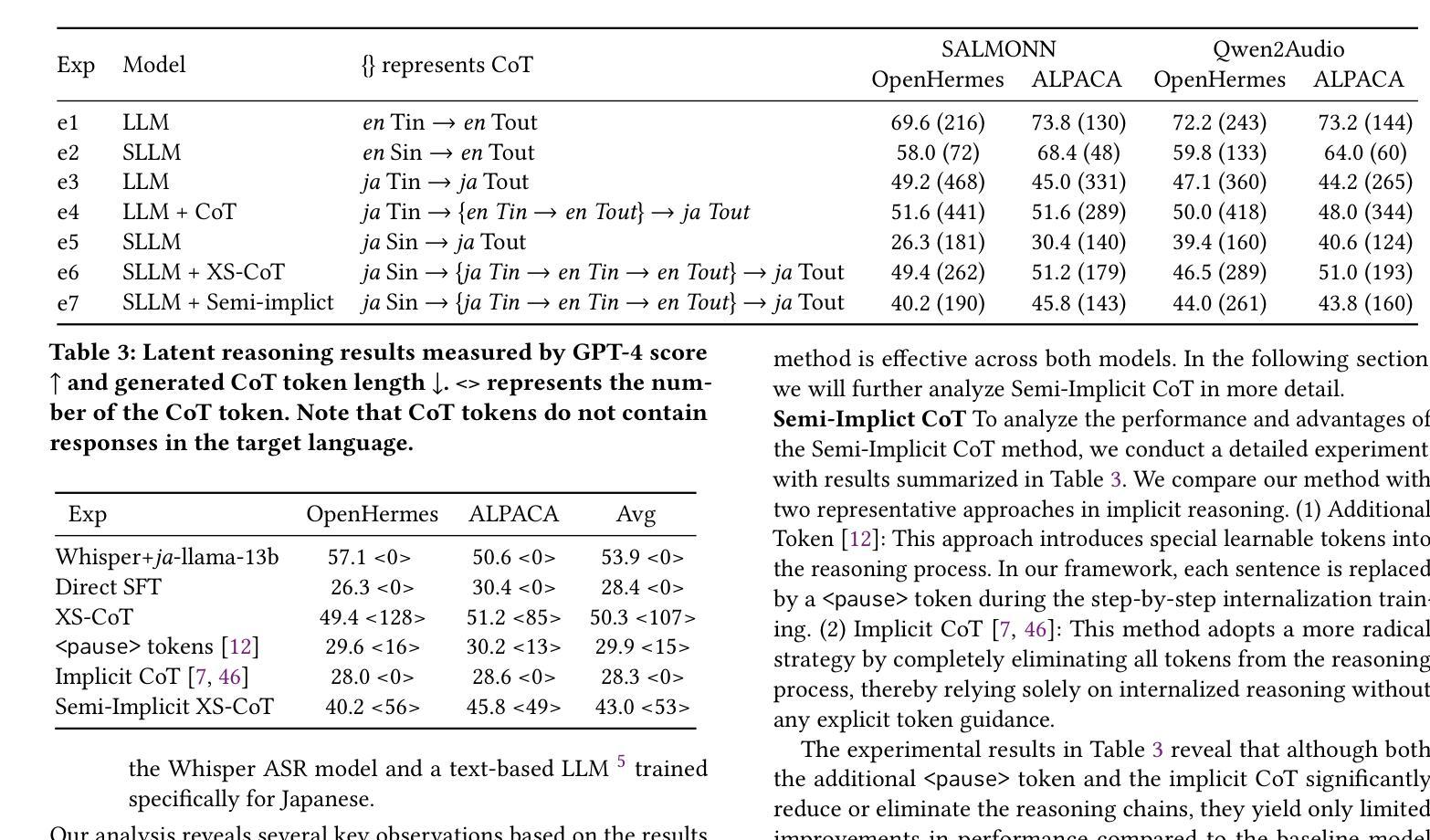
Turing Machine Evaluation for Large Language Model
Authors:Haitao Wu, Zongbo Han, Huaxi Huang, Changqing Zhang
With the rapid development and widespread application of Large Language Models (LLMs), rigorous evaluation has become particularly crucial. This research adopts a novel perspective, focusing on evaluating the core computational reasoning ability of LLMs, defined as the capacity of model to accurately understand rules, and execute logically computing operations. This capability assesses the reliability of LLMs as precise executors, and is critical to advanced tasks such as complex code generation and multi-step problem-solving. We propose an evaluation framework based on Universal Turing Machine (UTM) simulation. This framework requires LLMs to strictly follow instructions and track dynamic states, such as tape content and read/write head position, during multi-step computations. To enable standardized evaluation, we developed TMBench, a benchmark for systematically studying the computational reasoning capabilities of LLMs. TMBench provides several key advantages, including knowledge-agnostic evaluation, adjustable difficulty, foundational coverage through Turing machine encoding, and unlimited capacity for instance generation, ensuring scalability as models continue to evolve. We find that model performance on TMBench correlates strongly with performance on other recognized reasoning benchmarks (Pearson correlation coefficient is 0.73), clearly demonstrating that computational reasoning is a significant dimension for measuring the deep capabilities of LLMs. Code and data are available at https://github.com/HaitaoWuTJU/Turing-Machine-Bench.
随着大型语言模型(LLMs)的快速发展和广泛应用,严格评估变得尤为关键。本研究采用了一种新颖的视角,专注于评估LLMs的核心计算推理能力,这被定义为模型准确理解规则并执行逻辑计算操作的能力。这种能力评估了LLMs作为精确执行者的可靠性,对于复杂代码生成和多步问题解决等高级任务至关重要。我们提出了基于通用图灵机(UTM)模拟的评估框架。该框架要求LLMs在多步计算过程中严格遵守指令并跟踪动态状态,例如磁带内容以及读写头位置。为了实现标准化评估,我们开发了TMBench,这是用于系统研究LLMs计算推理能力的基准测试。TMBench提供了几个关键优势,包括与知识无关的评价、可调整的难度、通过图灵机编码的基础覆盖,以及无限的实例生成能力,确保随着模型的不断发展而具有可扩展性。我们发现,模型在TMBench上的表现与其他公认的推理基准测试上的表现有很强的相关性(皮尔逊相关系数达到0.73),这清楚地表明计算推理是衡量LLMs深层能力的重要维度。代码和数据可在https://github.com/HaitaoWuTJU/Turing-Machine-Bench找到。
论文及项目相关链接
Summary
大型语言模型(LLMs)的快速发展和广泛应用,对其严格评估变得尤为重要。本研究从新颖的角度评估LLMs的核心计算推理能力,包括模型准确理解规则和执行逻辑计算操作的能力。为标准化评估,研究提出了基于通用图灵机(UTM)模拟的评估框架,并开发了TMBench基准测试,可系统地研究LLMs的计算推理能力。TMBench提供知识无关评估、可调难度、图灵机编码基础覆盖和无限实例生成能力等优点,确保随着模型发展具有可扩展性。研究发现,TMBench上的模型性能与其他公认的推理基准测试之间存在强烈相关性(皮尔逊相关系数0.7in the evaluation of LLMs)。代码和数据可在https://github.com/HaitaoWuTJU/Turing-Machine-Bench找到。
Key Takeaways
- 大型语言模型(LLMs)的核心计算推理能力评估变得重要。
- 研究关注于LLMs准确理解规则和执行逻辑计算操作的能力评估。
- 提出基于通用图灵机(UTM)模拟的评估框架。
- 开发了TMBench基准测试,用于系统地研究LLMs的计算推理能力。
- TMBench提供知识无关评估、可调难度、图灵机编码基础覆盖和无限实例生成能力。
- TMBench上的模型性能与其他推理基准测试存在强烈相关性。
点此查看论文截图
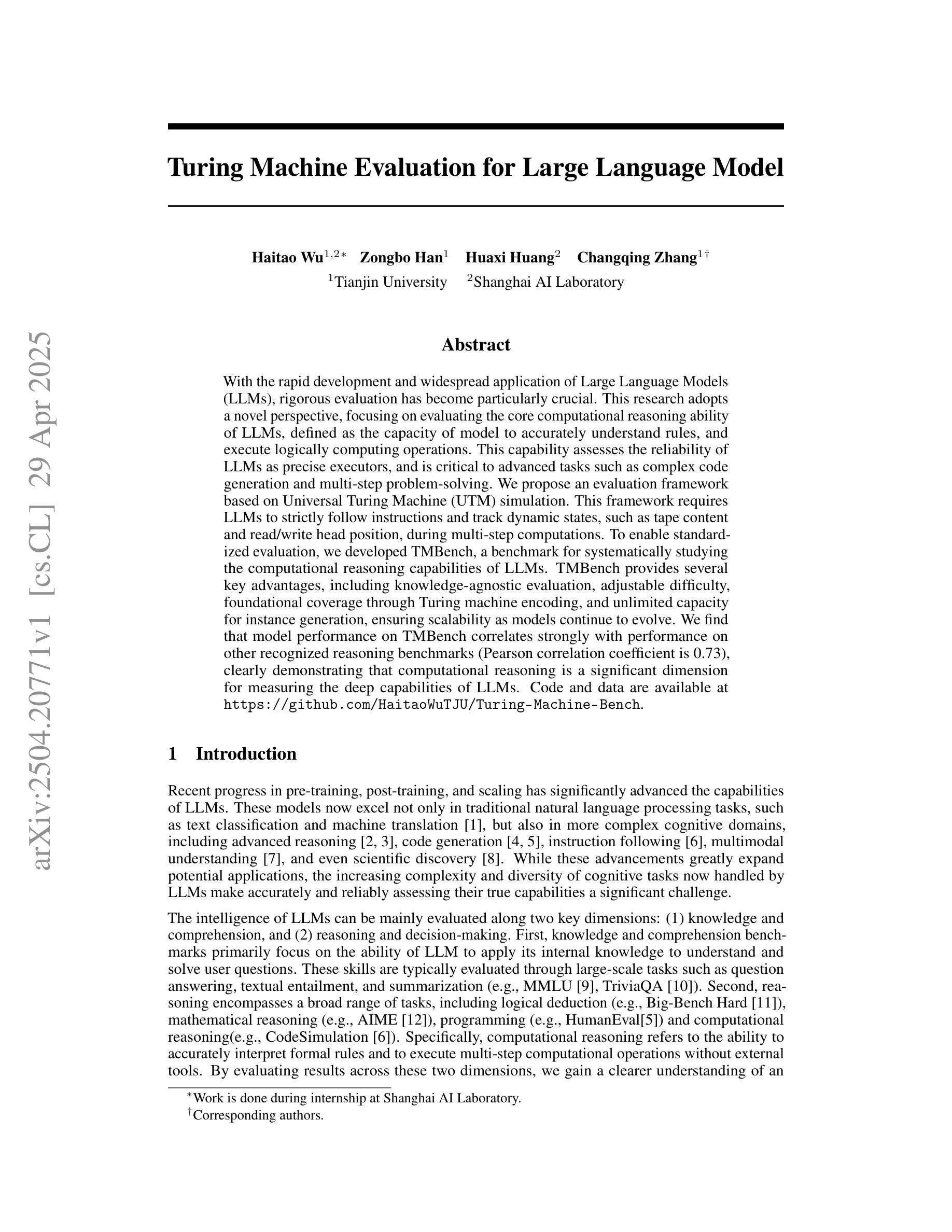
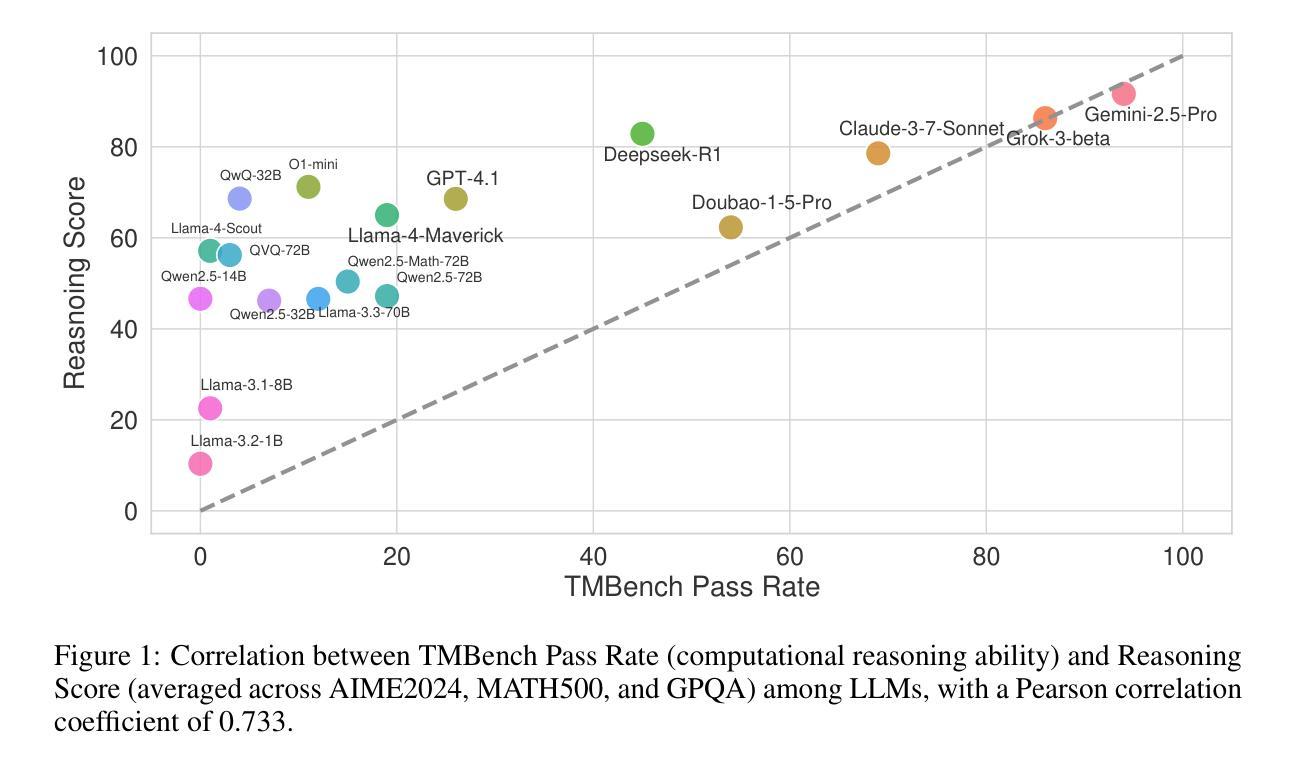
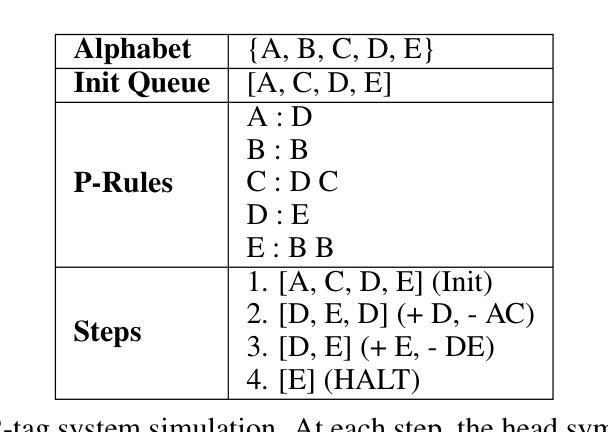
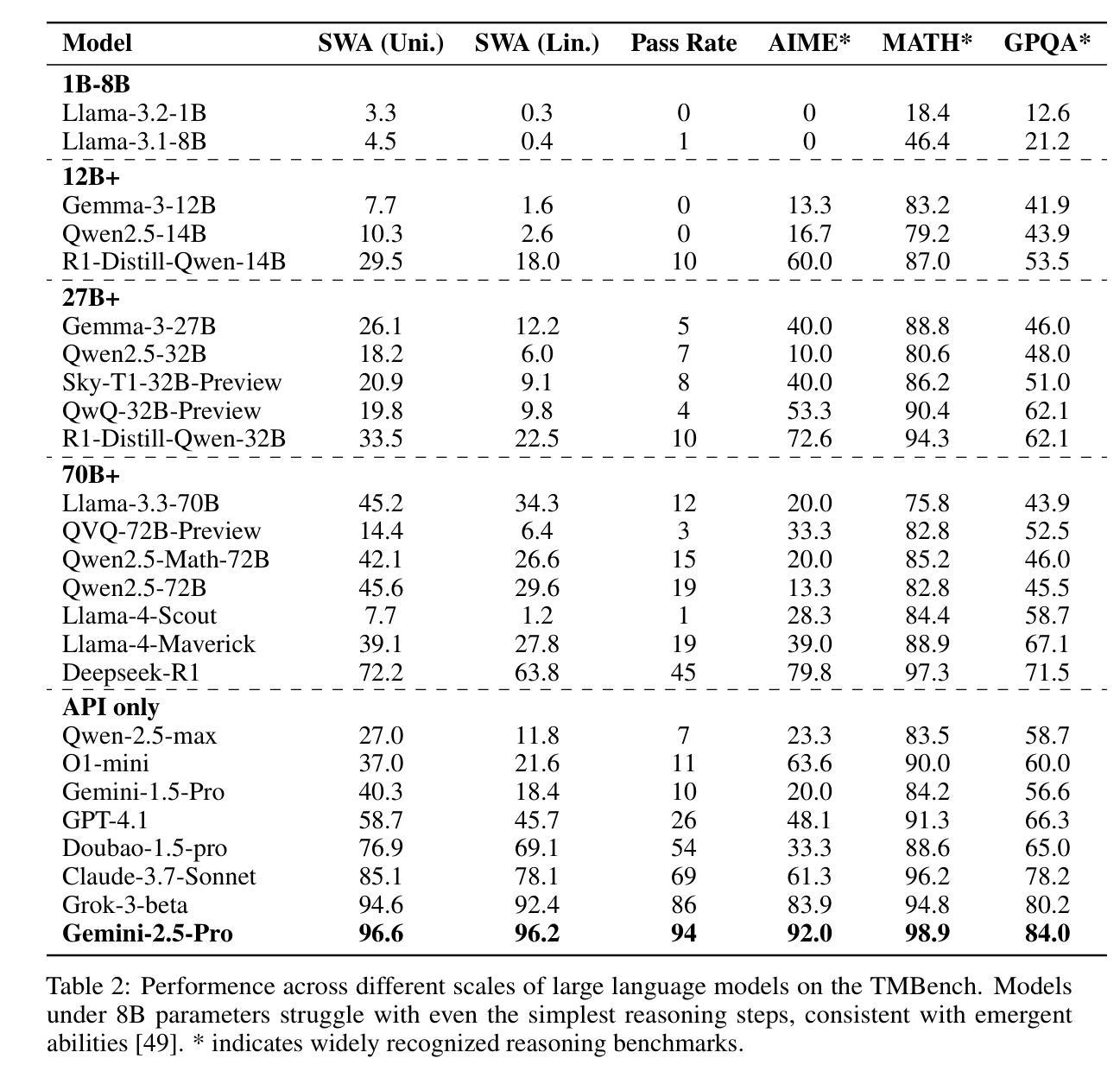
Grokking in the Wild: Data Augmentation for Real-World Multi-Hop Reasoning with Transformers
Authors:Roman Abramov, Felix Steinbauer, Gjergji Kasneci
Transformers have achieved great success in numerous NLP tasks but continue to exhibit notable gaps in multi-step factual reasoning, especially when real-world knowledge is sparse. Recent advances in grokking have demonstrated that neural networks can transition from memorizing to perfectly generalizing once they detect underlying logical patterns - yet these studies have primarily used small, synthetic tasks. In this paper, for the first time, we extend grokking to real-world factual data and address the challenge of dataset sparsity by augmenting existing knowledge graphs with carefully designed synthetic data to raise the ratio $\phi_r$ of inferred facts to atomic facts above the threshold required for grokking. Surprisingly, we find that even factually incorrect synthetic data can strengthen emergent reasoning circuits rather than degrade accuracy, as it forces the model to rely on relational structure rather than memorization. When evaluated on multi-hop reasoning benchmarks, our approach achieves up to 95-100% accuracy on 2WikiMultiHopQA - substantially improving over strong baselines and matching or exceeding current state-of-the-art results. We further provide an in-depth analysis of how increasing $\phi_r$ drives the formation of generalizing circuits inside Transformers. Our findings suggest that grokking-based data augmentation can unlock implicit multi-hop reasoning capabilities, opening the door to more robust and interpretable factual reasoning in large-scale language models.
Transformer在许多NLP任务中取得了巨大成功,但在多步骤事实推理方面仍存在明显差距,特别是在现实世界知识稀缺的情况下。最近的Grokkin(一种学习能力)的研究进展表明,一旦神经网络发现潜在的逻辑模式,它们就可以从记忆转变为完美概括——但这些研究主要使用的是小规模的合成任务。在本文中,我们首次将Grokkin扩展到现实世界的事实数据,并通过精心设计的合成数据增强现有知识图谱来解决数据集稀疏性的挑战,从而提高推断事实与原子事实的比例$\phi_r$,使其超过实现Grokkin所需的阈值。令人惊讶的是,我们发现即使是事实上不正确的合成数据也可以加强新兴的推理电路,而不是降低准确性,因为它迫使模型依赖关系结构而不是记忆。在评估多跳推理基准测试时,我们的方法在2WikiMultiHopQA上达到了95-100%的准确率——这大大超过了强大的基准测试并匹配或超过了当前最新的结果。我们还深入分析了如何提高$\phi_r$在Transformer内部形成概括电路的过程。我们的研究结果表明,基于Grokkin的数据增强可以解锁隐式的多跳推理能力,为大规模语言模型中的更稳健和可解释的事实推理打开了大门。
论文及项目相关链接
Summary:
本文探讨了将Grokking技术扩展到真实世界事实数据所面临的挑战,并提出了通过增加合成数据来增强现有知识图谱的方法,以解决数据集稀疏性问题。研究发现,即使是事实错误的合成数据也能强化新兴推理电路,而不是降低准确性。该方法在多跳推理基准测试中取得了显著成果,并在2WikiMultiHopQA上实现了高达95-100%的准确率。研究还发现,通过增加$\phi_r$可以推动Transformer内部形成通用电路,这表明基于Grokking的数据增强可以解锁隐式多跳推理能力,为大规模语言模型中的更稳健和可解释的事实推理打开了大门。
Key Takeaways:
- Transformers在NLP任务中取得了巨大成功,但在多步推理方面仍有所欠缺,尤其是在真实世界知识稀疏的情况下。
- Grokking技术的扩展对于处理真实世界的事实数据具有重要意义。
- 通过增加合成数据来增强现有知识图谱,可以解决数据集稀疏性问题。
- 即使是事实错误的合成数据也能强化新兴推理电路,推动模型更多地依赖关系结构而非记忆。
- 该方法在多跳推理基准测试中表现优异,实现了高准确率。
- 增加$\phi_r$有助于在Transformer内部形成通用电路,从而提高模型的泛化能力。
点此查看论文截图
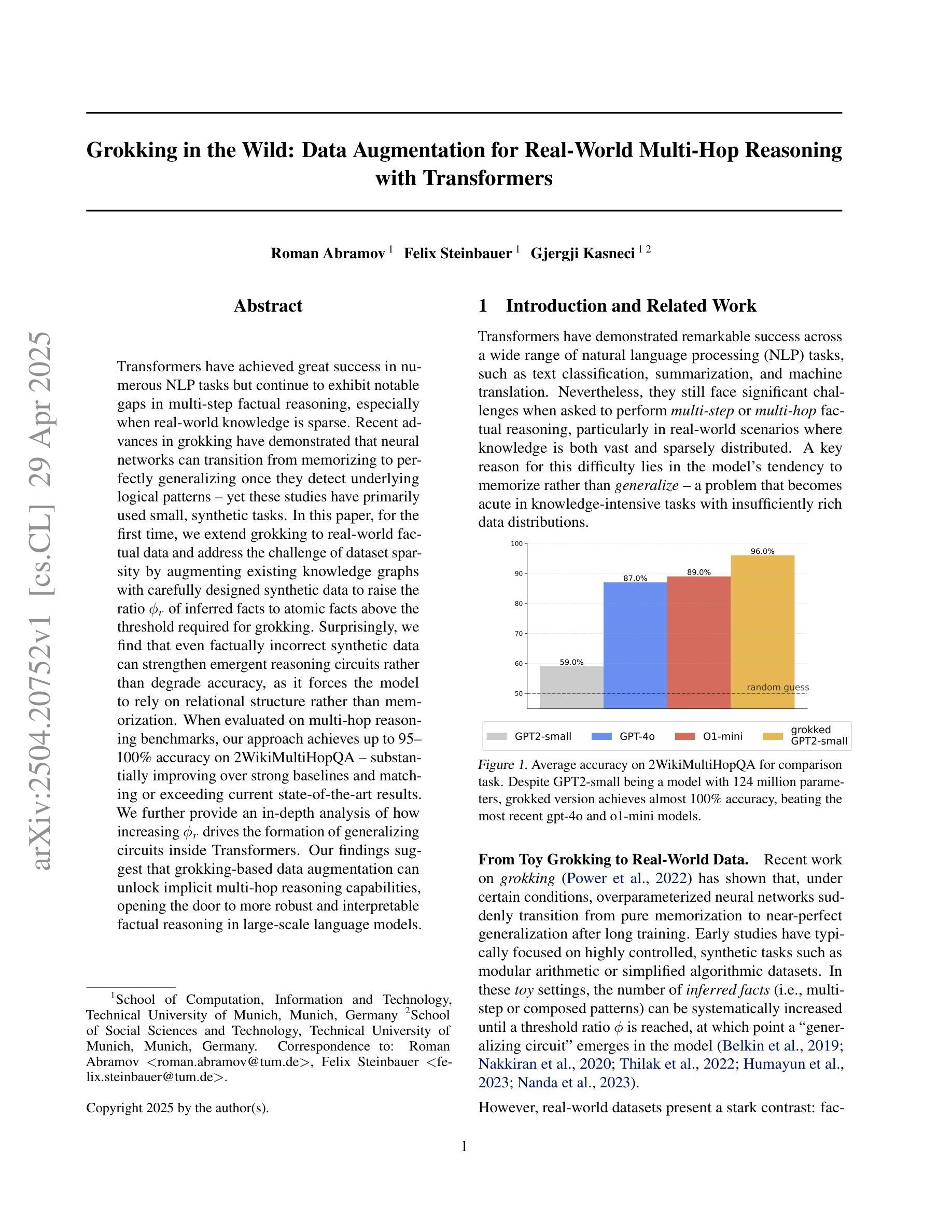
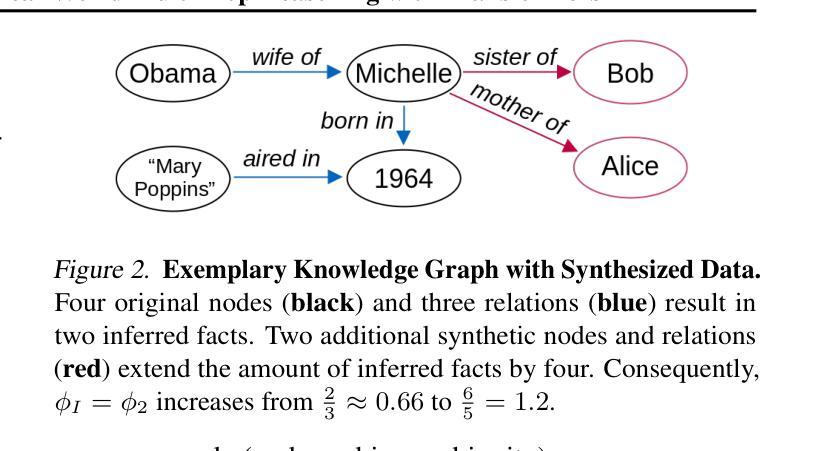
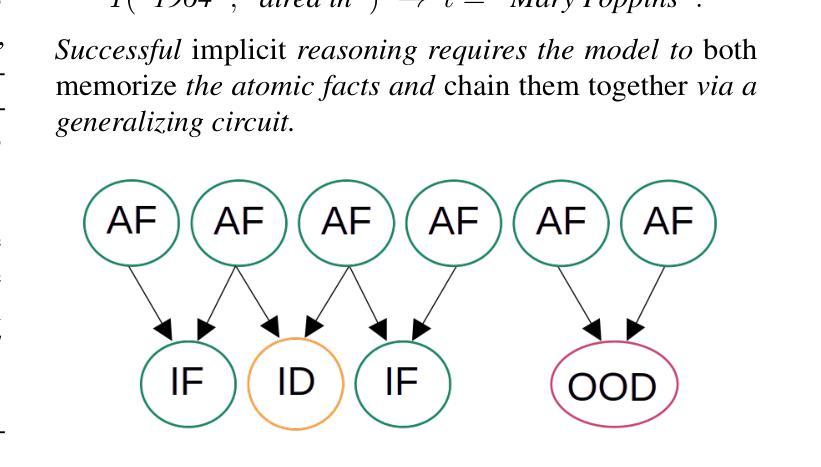
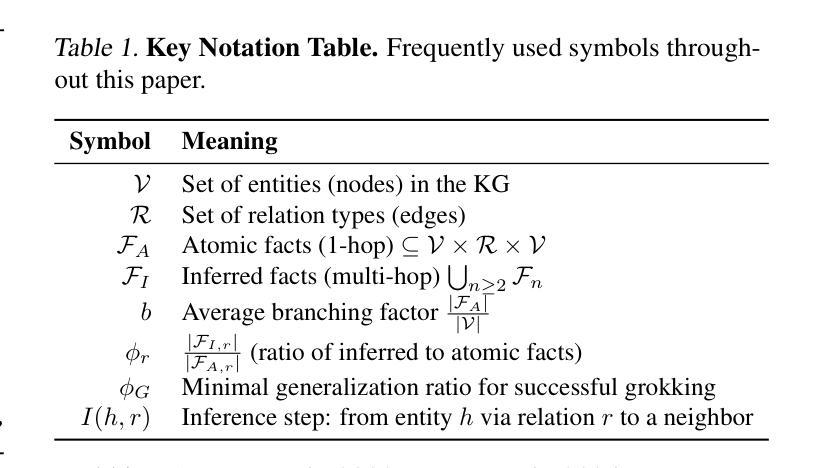
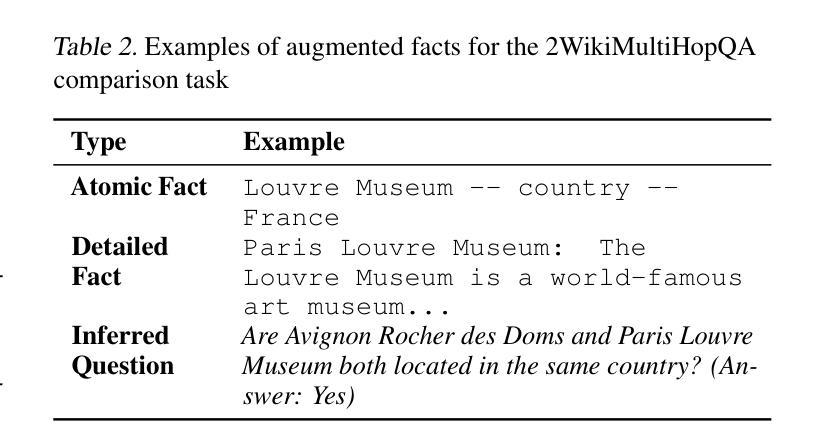
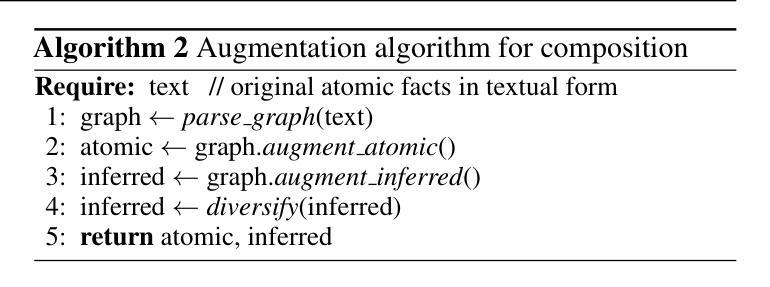
Beyond the Last Answer: Your Reasoning Trace Uncovers More than You Think
Authors:Hasan Abed Al Kader Hammoud, Hani Itani, Bernard Ghanem
Large Language Models (LLMs) leverage step-by-step reasoning to solve complex problems. Standard evaluation practice involves generating a complete reasoning trace and assessing the correctness of the final answer presented at its conclusion. In this paper, we challenge the reliance on the final answer by posing the following two questions: Does the final answer reliably represent the model’s optimal conclusion? Can alternative reasoning paths yield different results? To answer these questions, we analyze intermediate reasoning steps, termed subthoughts, and propose a method based on our findings. Our approach involves segmenting a reasoning trace into sequential subthoughts based on linguistic cues. We start by prompting the model to generate continuations from the end-point of each intermediate subthought. We extract a potential answer from every completed continuation originating from different subthoughts. We find that aggregating these answers by selecting the most frequent one (the mode) often yields significantly higher accuracy compared to relying solely on the answer derived from the original complete trace. Analyzing the consistency among the answers derived from different subthoughts reveals characteristics that correlate with the model’s confidence and correctness, suggesting potential for identifying less reliable answers. Our experiments across various LLMs and challenging mathematical reasoning datasets (AIME2024 and AIME2025) show consistent accuracy improvements, with gains reaching up to 13% and 10% respectively. Implementation is available at: https://github.com/hammoudhasan/SubthoughtReasoner.
大型语言模型(LLM)通过逐步推理来解决复杂问题。标准的评估实践包括生成完整的推理过程并评估最终答案的正确性。在本文中,我们通过提出以下两个问题来质疑对最终答案的依赖:最终答案是否可靠地代表模型的优化结论?替代推理路径会产生不同的结果吗?为了回答这些问题,我们分析了中间的推理步骤,称为子思想,并基于我们的发现提出了一种方法。我们的方法涉及根据语言线索将推理过程分割成连续的子思想。我们从每个中间子思想的终点开始提示模型生成连续内容。我们从不同子思想产生的每个完整连续内容中提取潜在答案。我们发现,通过选择出现次数最多的答案(众数)进行聚合,通常比仅依赖从原始完整追踪中得出的答案产生更高的准确性。分析不同子思想得出的答案之间的一致性揭示了与模型的信心和正确性相关的特征,这表明有潜力识别出不太可靠的答案。我们在不同的LLM和具有挑战性的数学推理数据集(AIME2024和AIME2025)上的实验显示了持续的准确性改进,增益分别高达13%和10%。实施方法可在:https://github.com/hammoudhasan/SubthoughtReasoner获取。
论文及项目相关链接
PDF Preprint
Summary:大型语言模型(LLM)通过逐步推理解决复杂问题。本文质疑了仅依赖最终答案评估模型表现的方法,提出分析中间推理步骤(称为子思维)的方法。通过分割推理轨迹,从每个中间子思维的终点引导模型生成延续内容,并从中提取潜在答案。发现聚合这些答案中的最常见答案(众数)通常比仅依赖原始完整轨迹得出的答案更准确。分析不同子思维得出的答案一致性可揭示与模型自信和正确性相关的特征,有助于识别不太可靠的答案。实验表明,在多个LLM和具有挑战性的数学推理数据集上,该方法实现了持续提高准确性,提升幅度高达13%和10%。
Key Takeaways:
- 大型语言模型(LLM)使用逐步推理解决复杂问题。
- 现有评估方法过于依赖最终答案,可能忽略了模型的优化结论。
- 文中提出分析中间推理步骤的方法,称为子思维。
- 通过引导模型从每个中间子思维的终点生成延续内容,并提取潜在答案,发现聚合常见答案可提高准确性。
- 分析不同子思维得出的答案一致性有助于识别模型的自信和正确性。
- 方法在多个LLM和数学推理数据集上实现准确性提高。
点此查看论文截图
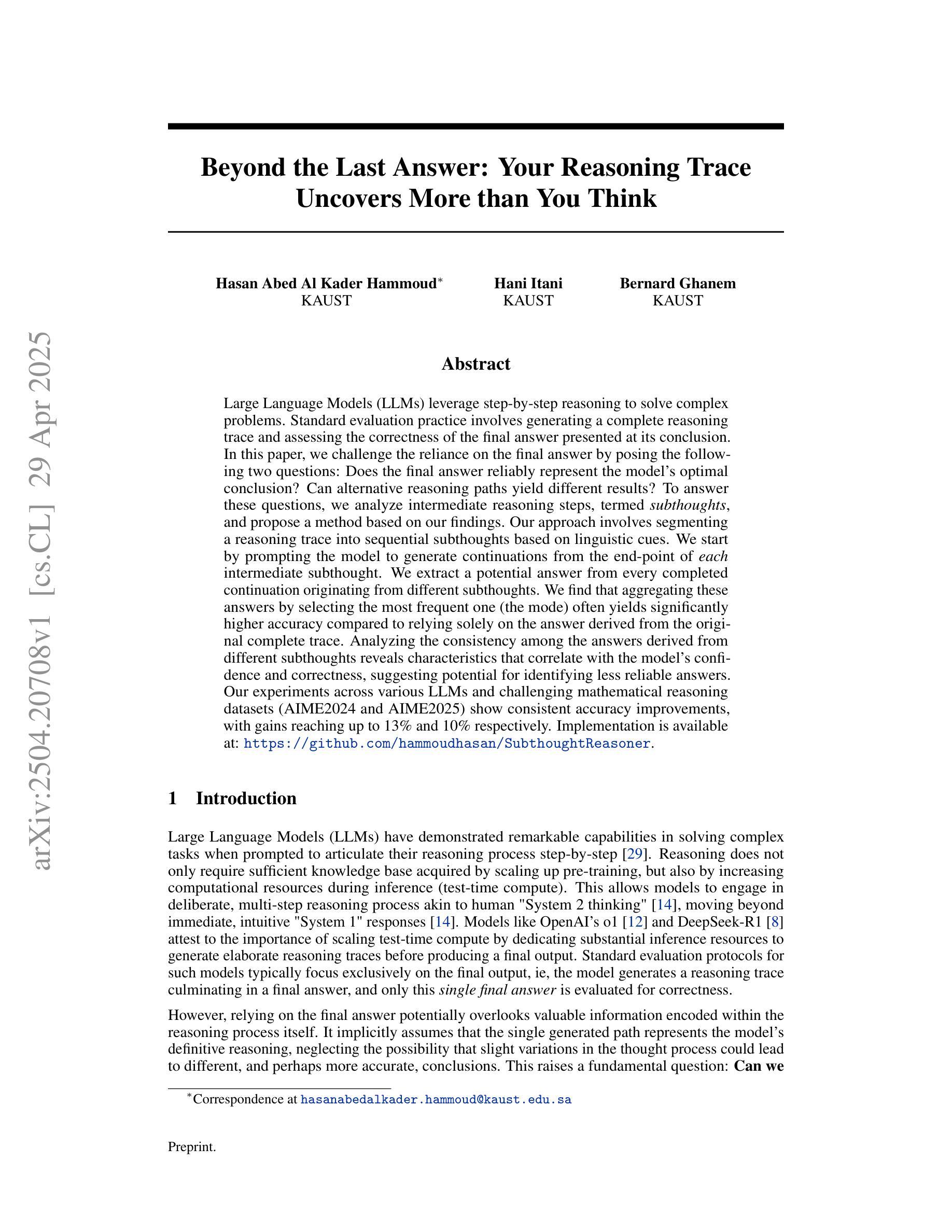
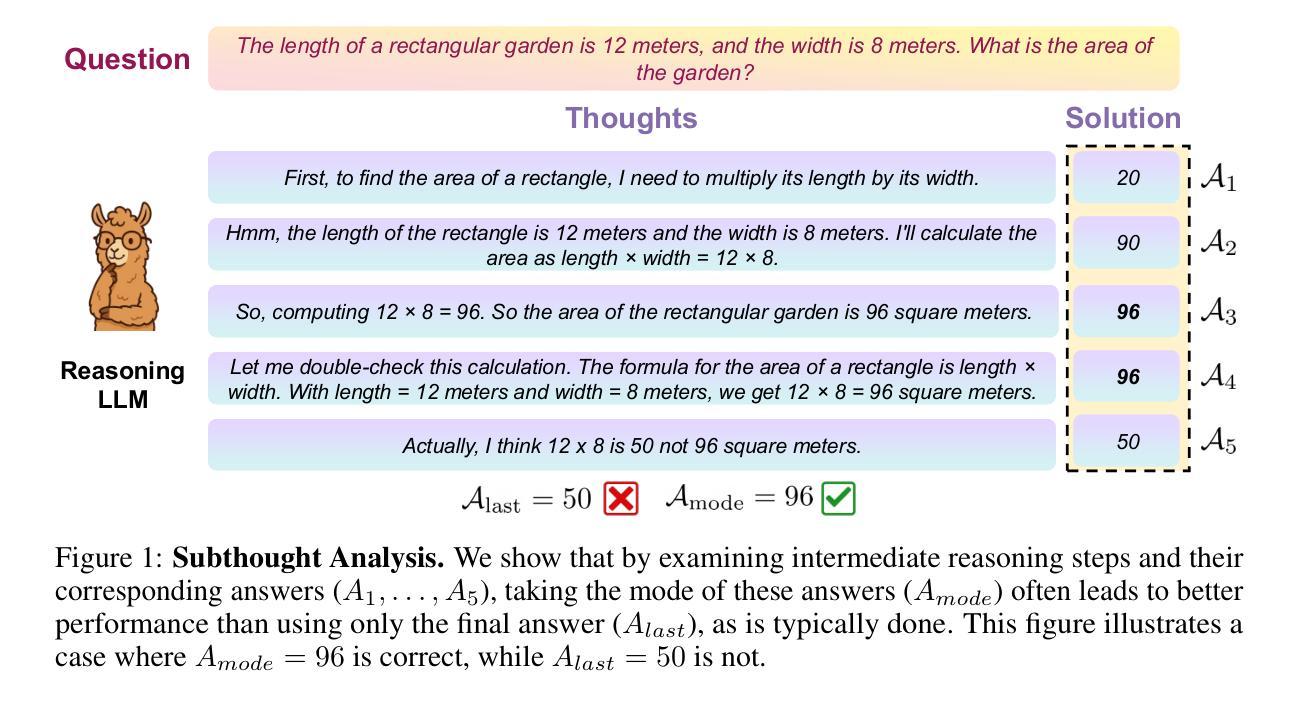
ReasonIR: Training Retrievers for Reasoning Tasks
Authors:Rulin Shao, Rui Qiao, Varsha Kishore, Niklas Muennighoff, Xi Victoria Lin, Daniela Rus, Bryan Kian Hsiang Low, Sewon Min, Wen-tau Yih, Pang Wei Koh, Luke Zettlemoyer
We present ReasonIR-8B, the first retriever specifically trained for general reasoning tasks. Existing retrievers have shown limited gains on reasoning tasks, in part because existing training datasets focus on short factual queries tied to documents that straightforwardly answer them. We develop a synthetic data generation pipeline that, for each document, our pipeline creates a challenging and relevant query, along with a plausibly related but ultimately unhelpful hard negative. By training on a mixture of our synthetic data and existing public data, ReasonIR-8B achieves a new state-of-the-art of 29.9 nDCG@10 without reranker and 36.9 nDCG@10 with reranker on BRIGHT, a widely-used reasoning-intensive information retrieval (IR) benchmark. When applied to RAG tasks, ReasonIR-8B improves MMLU and GPQA performance by 6.4% and 22.6% respectively, relative to the closed-book baseline, outperforming other retrievers and search engines. In addition, ReasonIR-8B uses test-time compute more effectively: on BRIGHT, its performance consistently increases with longer and more information-rich rewritten queries; it continues to outperform other retrievers when combined with an LLM reranker. Our training recipe is general and can be easily extended to future LLMs; to this end, we open-source our code, data, and model.
我们推出ReasonIR-8B,这是专门为一般推理任务训练的第一个检索器。现有检索器在推理任务上的收益有限,部分原因在于现有训练数据集专注于与文档直接相关的简短事实查询。我们开发了一个合成数据生成管道,该管道针对每篇文档,创建一个具有挑战性和相关性的查询,以及一个可能相关但最终无用的硬负样本。通过在我们的合成数据和现有公共数据的混合训练上,ReasonIR-8B在BRIGHT这一广泛使用的推理密集型信息检索基准测试上,无需重新排名者即可实现29.9的nDCG@10,使用重新排名者则为36.9的nDCG@10,达到最新水平。当应用于RAG任务时,ReasonIR-8B相对于封闭书籍基线提高了MMLU和GPQA的性能,分别提高了6.4%和22.6%,优于其他检索器和搜索引擎。此外,ReasonIR-8B更有效地使用测试时间计算:在BRIGHT上,其性能随着更长的信息丰富的重写查询而持续提高;当与大型语言模型重新排名器结合时,它仍然表现优于其他检索器。我们的训练配方是通用的,可以很容易地扩展到未来的大型语言模型;为此,我们开源了我们的代码、数据和模型。
论文及项目相关链接
PDF Our code is released at \url{https://github.com/facebookresearch/ReasonIR}
Summary
本文介绍了ReasonIR-8B,首个专门用于一般推理任务的检索器。现有的检索器在推理任务上的收益有限,因为现有的训练数据集主要集中在与文档直接相关的简短事实查询上。开发了一个合成数据生成管道,为每个文档创建具有挑战性和相关性的查询,以及可能相关但最终不相关的硬负样本。ReasonIR-8B在广泛使用的推理密集型信息检索基准测试BRIGHT上取得了最新成果,无排名器时达到29.9 nDCG@10,有排名器时达到36.9 nDCG@10。在RAG任务中,ReasonIR-8B相较于封闭书籍的基线,对MMLU和GPQA的性能分别提高了6.4%和22.6%。此外,ReasonIR-8B更有效地使用测试时间计算:在BRIGHT上,其性能随着更长的信息丰富的重写查询而持续提高;当与大型语言模型排名器结合时,它继续优于其他检索器。公开了代码、数据和模型,以便未来大型语言模型扩展使用。
Key Takeaways
- ReasonIR-8B是首个专门用于一般推理任务的检索器。
- 现有检索器在推理任务上的表现有限,因为训练数据集主要关注简短的事实查询。
- 开发了一个合成数据生成管道,为每篇文档创建挑战性的相关查询和硬负样本。
- ReasonIR-8B在推理密集型信息检索基准测试(BRIGHT)上取得了最新成果。
- 在RAG任务中,ReasonIR-8B的性能优于其他检索器和搜索引擎。
- ReasonIR-8B更有效地使用测试时间计算,性能随更丰富的查询而提高。
点此查看论文截图
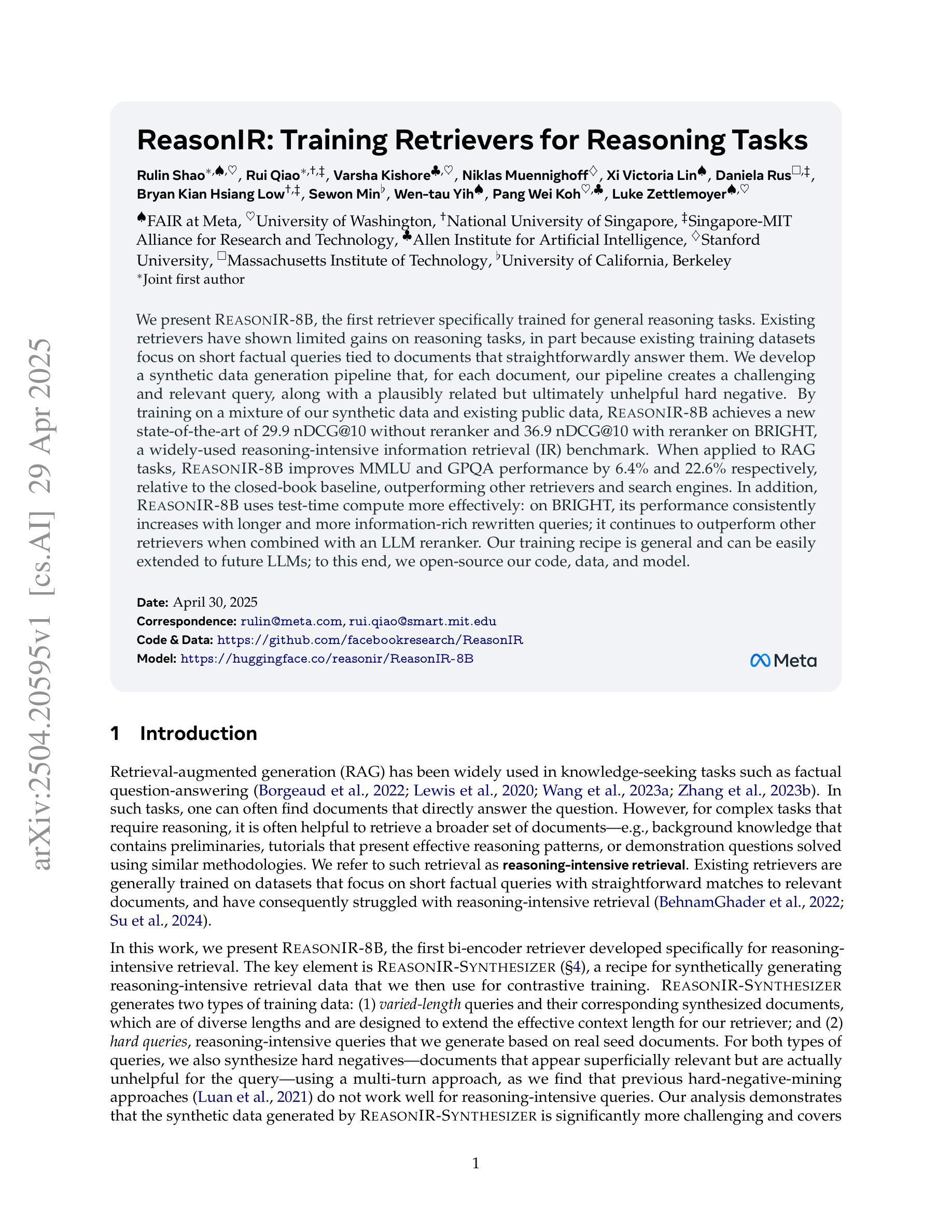
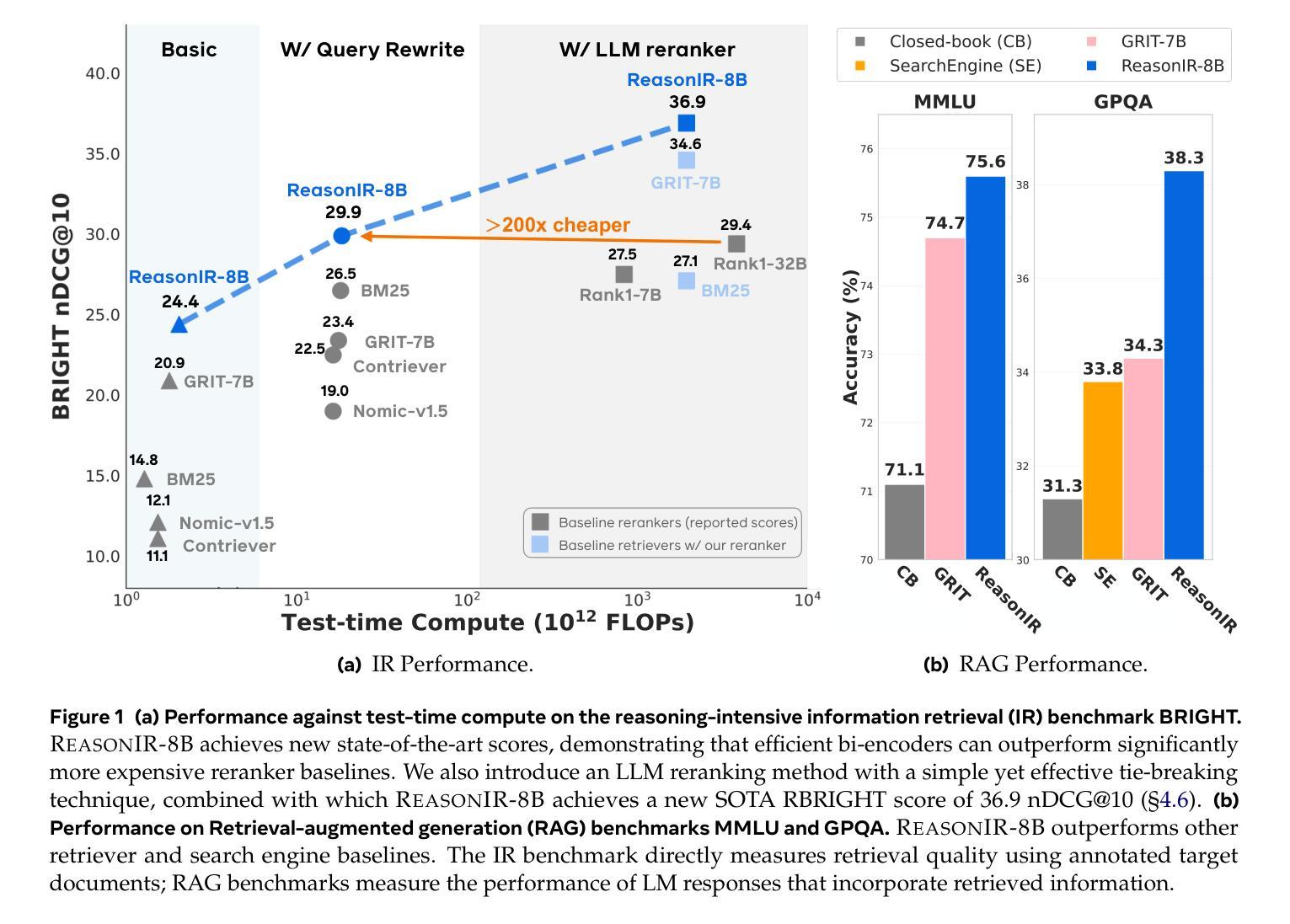
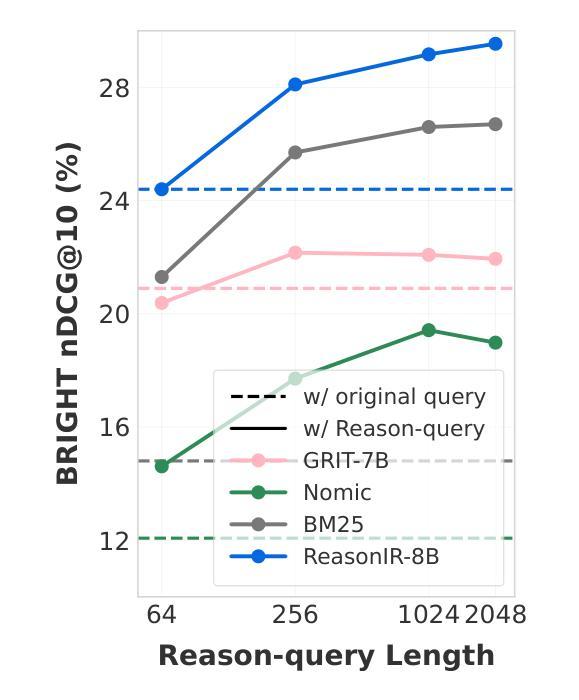
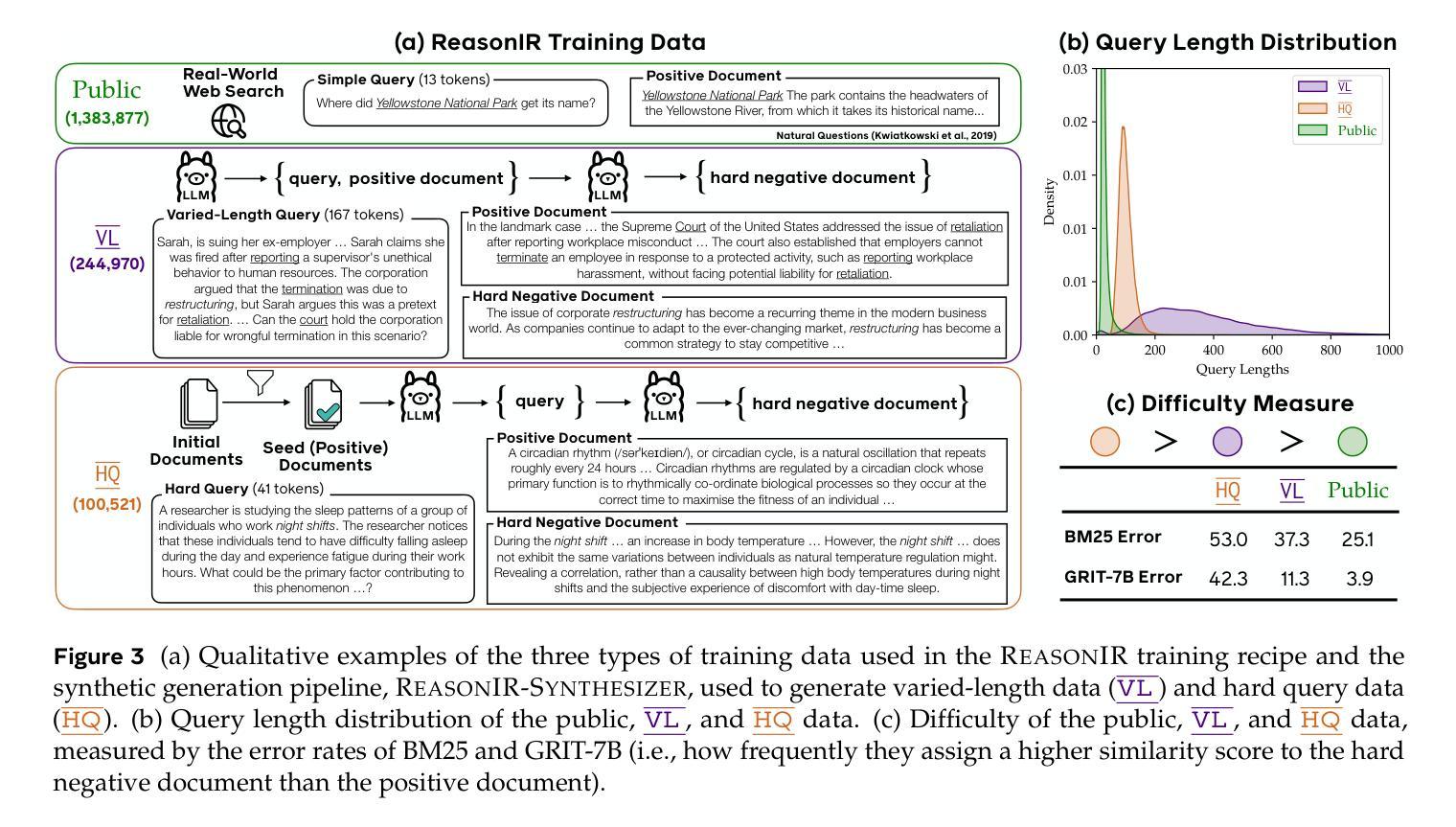
Independent Learning in Performative Markov Potential Games
Authors:Rilind Sahitaj, Paulius Sasnauskas, Yiğit Yalın, Debmalya Mandal, Goran Radanović
Performative Reinforcement Learning (PRL) refers to a scenario in which the deployed policy changes the reward and transition dynamics of the underlying environment. In this work, we study multi-agent PRL by incorporating performative effects into Markov Potential Games (MPGs). We introduce the notion of a performatively stable equilibrium (PSE) and show that it always exists under a reasonable sensitivity assumption. We then provide convergence results for state-of-the-art algorithms used to solve MPGs. Specifically, we show that independent policy gradient ascent (IPGA) and independent natural policy gradient (INPG) converge to an approximate PSE in the best-iterate sense, with an additional term that accounts for the performative effects. Furthermore, we show that INPG asymptotically converges to a PSE in the last-iterate sense. As the performative effects vanish, we recover the convergence rates from prior work. For a special case of our game, we provide finite-time last-iterate convergence results for a repeated retraining approach, in which agents independently optimize a surrogate objective. We conduct extensive experiments to validate our theoretical findings.
表现强化学习(PRL)指的是部署的策略改变基础环境的奖励和过渡动态的一种场景。在这项工作中,我们通过将表现效果纳入马尔可夫潜在博弈(MPGs),研究了多代理PRL。我们引入了表现稳定均衡(PSE)的概念,并在合理的敏感性假设下证明其总是存在。然后,我们提供了用于解决MPGs的先进算法的收敛结果。具体来说,我们展示了独立策略梯度上升法(IPGA)和独立自然策略梯度法(INPG)在最佳迭代意义上以表现效果为额外项的近似PSE收敛。此外,我们还证明了INPG最终会渐近收敛到PSE。随着表现效果的消失,我们恢复了之前的收敛速率。对于我们游戏的特殊情况,我们为重复再训练的方法提供了有限的最终迭代收敛结果,其中代理独立地优化替代目标。我们进行了广泛的实验来验证我们的理论发现。
论文及项目相关链接
PDF AISTATS 2025, code available at https://github.com/PauliusSasnauskas/performative-mpgs
Summary
强化学习(PRL)中的部署策略会改变基础环境的奖励和过渡动态。本研究将表演效果融入马尔可夫潜游戏(MPGs),研究多智能体PRL。引入表演稳定均衡(PSE)的概念,并在合理的敏感性假设下证明其存在性。我们还提供了解决MPGs的最新算法的收敛结果。特别是,我们展示了独立政策梯度上升(IPGA)和独立自然政策梯度(INPG)在最佳迭代意义上以表演效果为额外项收敛到近似PSE。并且,我们证明了INPG最终会渐近收敛到PSE。随着表演效果的消失,我们恢复了之前工作的收敛率。对于游戏的特殊情况,我们为重复训练的方法提供了有限的最后迭代收敛结果,其中智能体独立优化替代目标。我们进行了广泛的实验来验证我们的理论发现。
Key Takeaways
- PRL中部署的策略可以改变环境奖励和过渡动态。
- 引入表演稳定均衡(PSE)概念,并证明在合理敏感性假设下其存在性。
- IPGA和INPG算法在最佳迭代意义上收敛到近似PSE,包含表演效果的额外项。
- INPG最终会渐近收敛到PSE。
- 当表演效果消失时,收敛率恢复到先前工作的水平。
- 对于游戏的特殊情况,提供了有限时间内的最后迭代收敛结果,针对重复训练方法,智能体独立优化替代目标。
点此查看论文截图
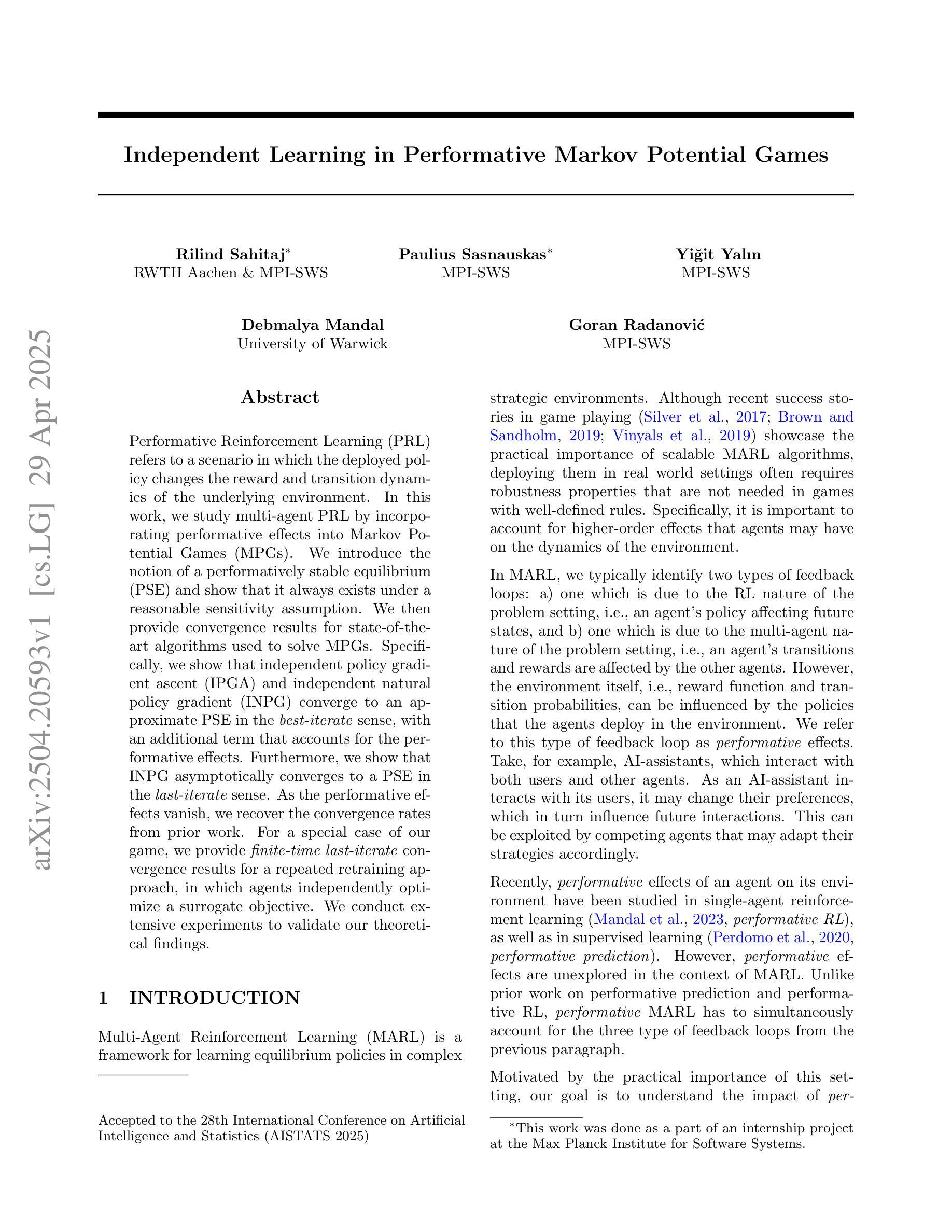
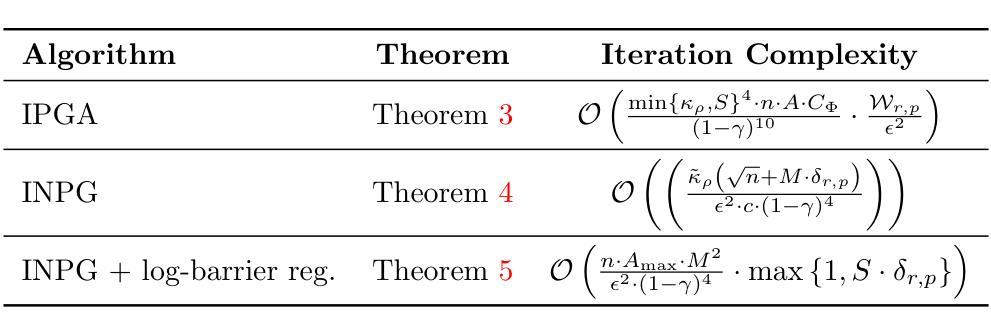
Reinforcement Learning for Reasoning in Large Language Models with One Training Example
Authors:Yiping Wang, Qing Yang, Zhiyuan Zeng, Liliang Ren, Lucas Liu, Baolin Peng, Hao Cheng, Xuehai He, Kuan Wang, Jianfeng Gao, Weizhu Chen, Shuohang Wang, Simon Shaolei Du, Yelong Shen
We show that reinforcement learning with verifiable reward using one training example (1-shot RLVR) is effective in incentivizing the math reasoning capabilities of large language models (LLMs). Applying RLVR to the base model Qwen2.5-Math-1.5B, we identify a single example that elevates model performance on MATH500 from 36.0% to 73.6%, and improves the average performance across six common mathematical reasoning benchmarks from 17.6% to 35.7%. This result matches the performance obtained using the 1.2k DeepScaleR subset (MATH500: 73.6%, average: 35.9%), which includes the aforementioned example. Similar substantial improvements are observed across various models (Qwen2.5-Math-7B, Llama3.2-3B-Instruct, DeepSeek-R1-Distill-Qwen-1.5B), RL algorithms (GRPO and PPO), and different math examples (many of which yield approximately 30% or greater improvement on MATH500 when employed as a single training example). In addition, we identify some interesting phenomena during 1-shot RLVR, including cross-domain generalization, increased frequency of self-reflection, and sustained test performance improvement even after the training accuracy has saturated, a phenomenon we term post-saturation generalization. Moreover, we verify that the effectiveness of 1-shot RLVR primarily arises from the policy gradient loss, distinguishing it from the “grokking” phenomenon. We also show the critical role of promoting exploration (e.g., by adding entropy loss with an appropriate coefficient) in 1-shot RLVR training. As a bonus, we observe that applying entropy loss alone, without any outcome reward, significantly enhances Qwen2.5-Math-1.5B’s performance on MATH500 by 27.4%. These findings can inspire future work on RLVR data efficiency and encourage a re-examination of both recent progress and the underlying mechanisms in RLVR. Our code, model, and data are open source at https://github.com/ypwang61/One-Shot-RLVR
我们展示了使用可验证奖励的强化学习(Reinforcement Learning with Verifiable Reward,简称RLVR)在激励大型语言模型(Large Language Models,简称LLM)的数学推理能力方面的有效性。通过对基础模型Qwen2.5-Math-1.5B应用RLVR,我们发现一个例子就能将MATH500模型的性能从36.0%提升到73.6%,并且在六个常见的数学推理基准测试上的平均性能从17.6%提高到35.7%。这一结果与使用DeepScaleR子集(MATH500: 73.6%,平均:35.9%)所获得的表现相匹配,其中包括上述例子。在各种模型(Qwen2.5-Math-7B、Llama3.2-3B-Instruct、DeepSeek-R1-Distill-Qwen-1.5B)、强化学习算法(GRPO和PPO)以及不同的数学例题中,都观察到了类似的显著改进(许多例题在作为单个训练示例时,MATH500上的改进率达到了大约30%或更高)。此外,在单镜头RLVR期间我们还发现了一些有趣的现象,包括跨域泛化、自我反思的频率增加,以及在训练准确性达到饱和后,测试性能仍有所提高的现象,我们称之为后饱和泛化。我们还验证了单镜头RLVR的有效性主要来源于策略梯度损失,这与“grokking”现象不同。我们还展示了促进探索(例如,通过添加适当的系数来引入熵损失)在单镜头RLVR训练中的关键作用。值得一提的是,我们观察到仅应用熵损失就能显著提高Qwen2.5-Math-1.5B在MATH500上的性能,提升了27.4%。这些发现可以激发未来对RLVR数据效率的研究,并鼓励重新评估RLVR的最新进展和潜在机制。我们的代码、模型和数据均开源在https://github.com/ypwang61/One-Shot-RLVR。
论文及项目相关链接
PDF 28 pages, 12 figures, link: https://github.com/ypwang61/One-Shot-RLVR
Summary
强化学习通过可验证奖励(RLVR)结合单一训练样本(1-shot),可有效激励大型语言模型(LLM)的数学推理能力。使用RLVR应用于Qwen2.5-Math-1.5B基础模型,通过单一例子提升MATH500任务性能从36.0%至73.6%,并在六个通用数学推理基准测试中的平均性能从17.6%提高至35.7%。类似的大幅改进在不同模型、RL算法和数学示例中都观察到。此外,RLVR还展现出跨域泛化、自我反思频率增加以及训练精度饱和后测试性能仍持续提升等有趣现象。
Key Takeaways
- 强化学习结合可验证奖励(RLVR)能有效提升大型语言模型(LLM)在数学推理方面的性能。
- 通过单一训练样本,MATH500任务性能有显著提升,并且这种提升在不同模型中都观察到。
- RLVR在不同RL算法和数学示例中均表现出良好的性能提升效果。
- RLVR展现出跨域泛化能力,模型能够在不同领域的问题中表现出良好的性能。
- 模型在训练过程中的自我反思频率增加,这可能有助于提高模型的推理能力。
- 训练精度饱和后,模型的测试性能仍持续提升,表现出一种称为“后饱和泛化”的现象。
- 强化学习中的策略梯度损失是RLVR效果的关键,与“grokking”现象有所区别。同时,促进探索(如通过添加熵损失和适当的系数)在RLVR训练中起着重要作用。
点此查看论文截图
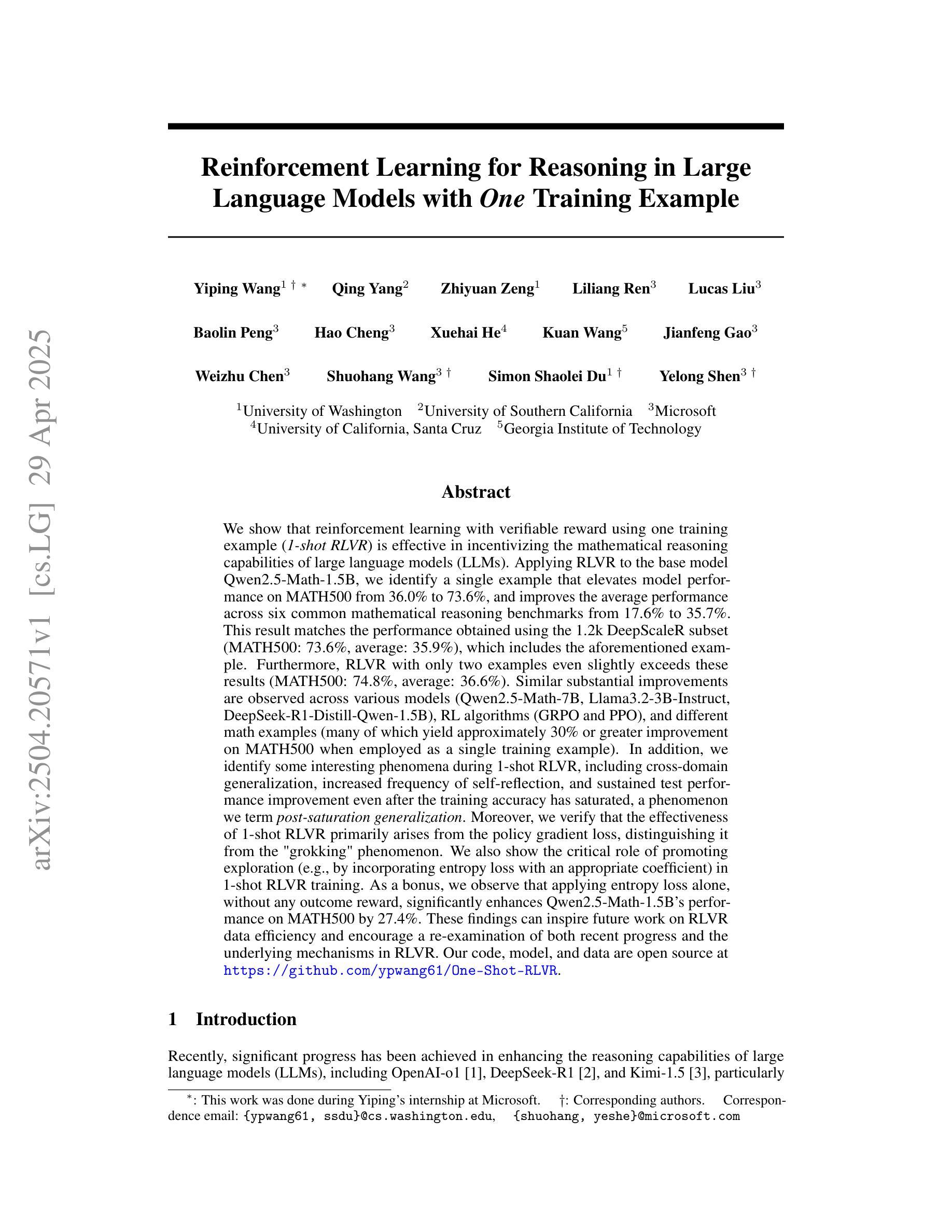
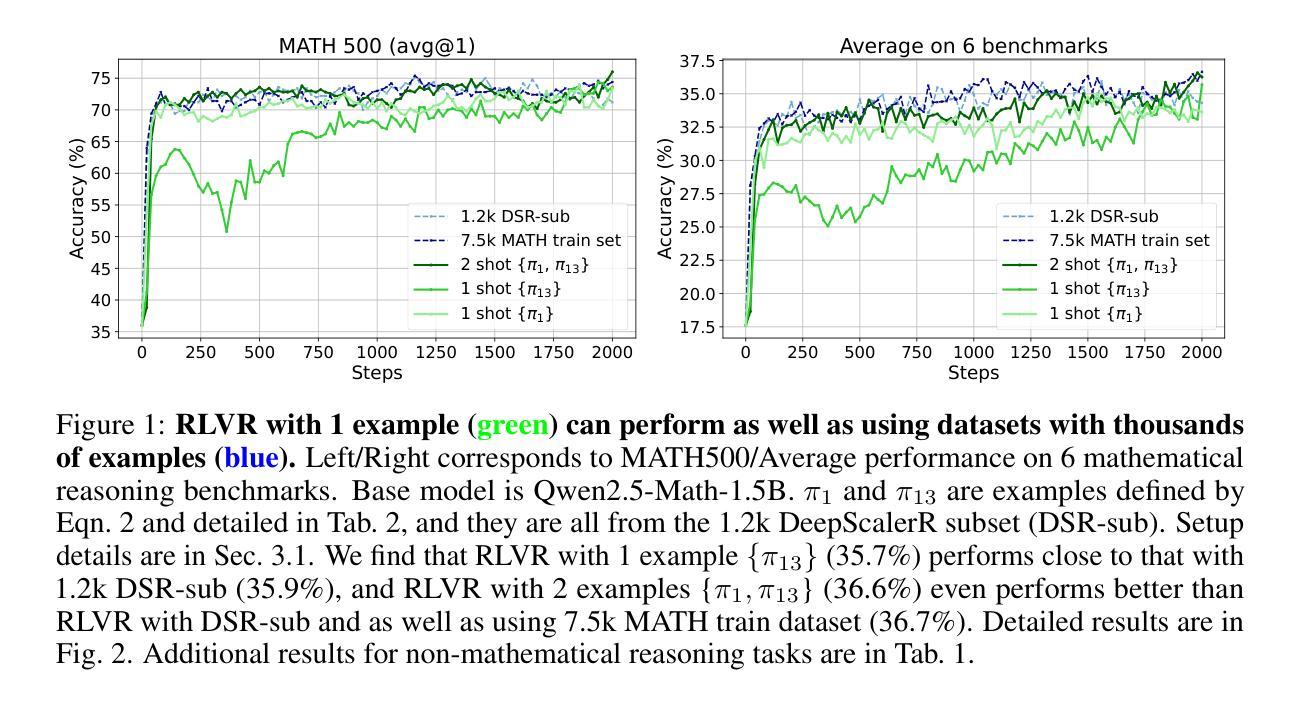
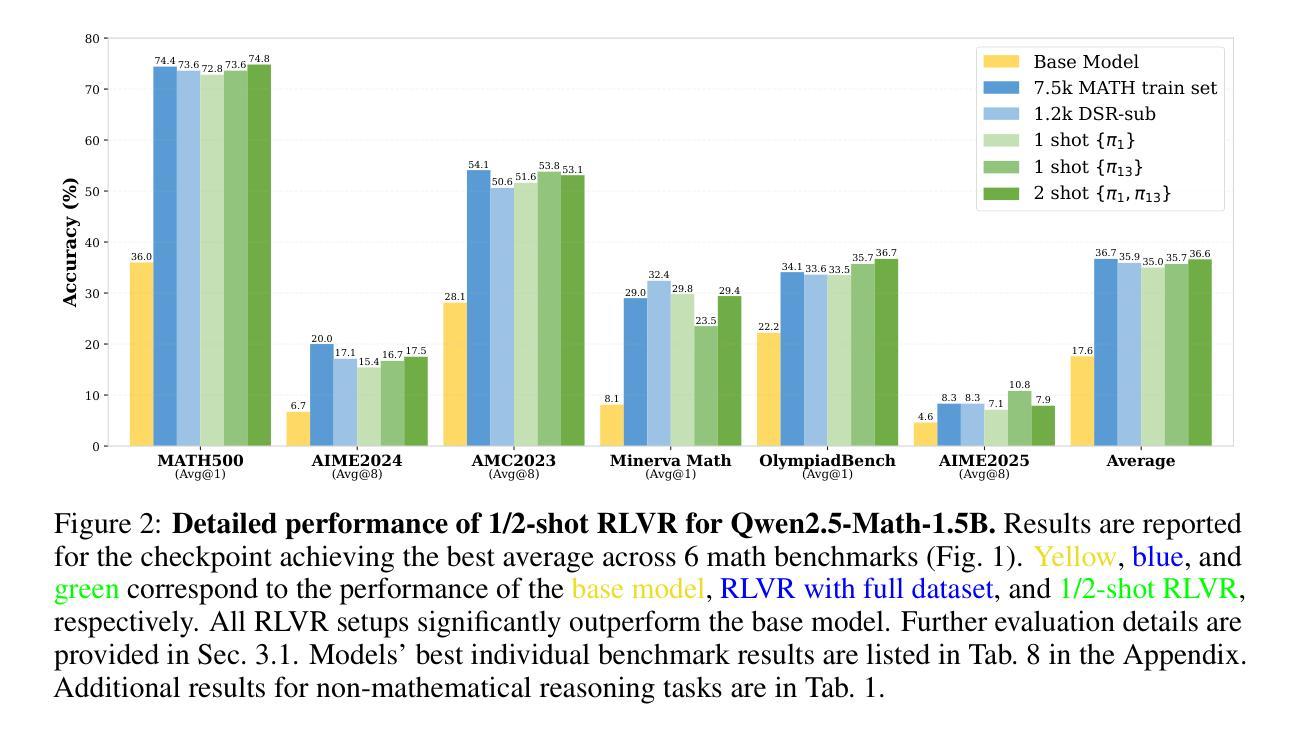
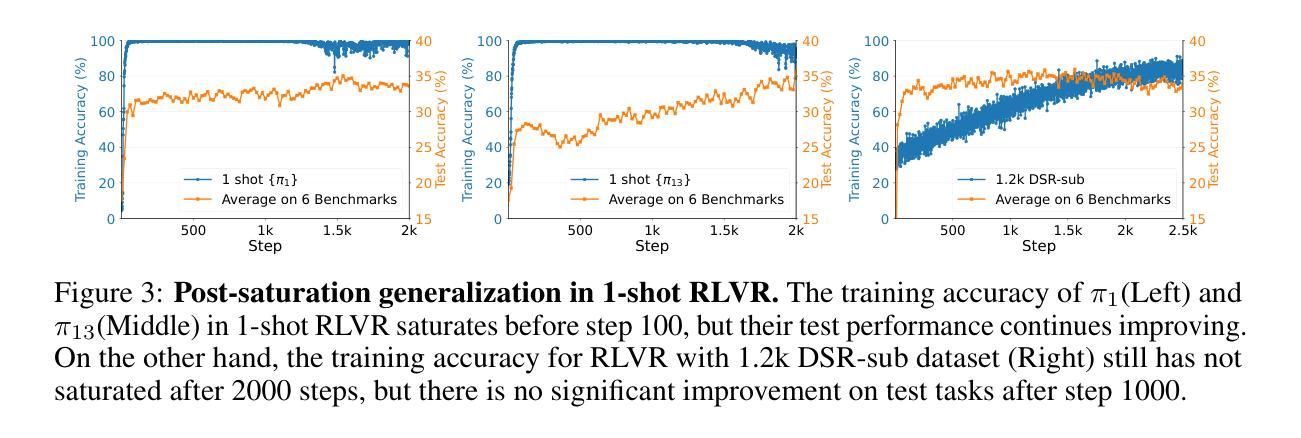
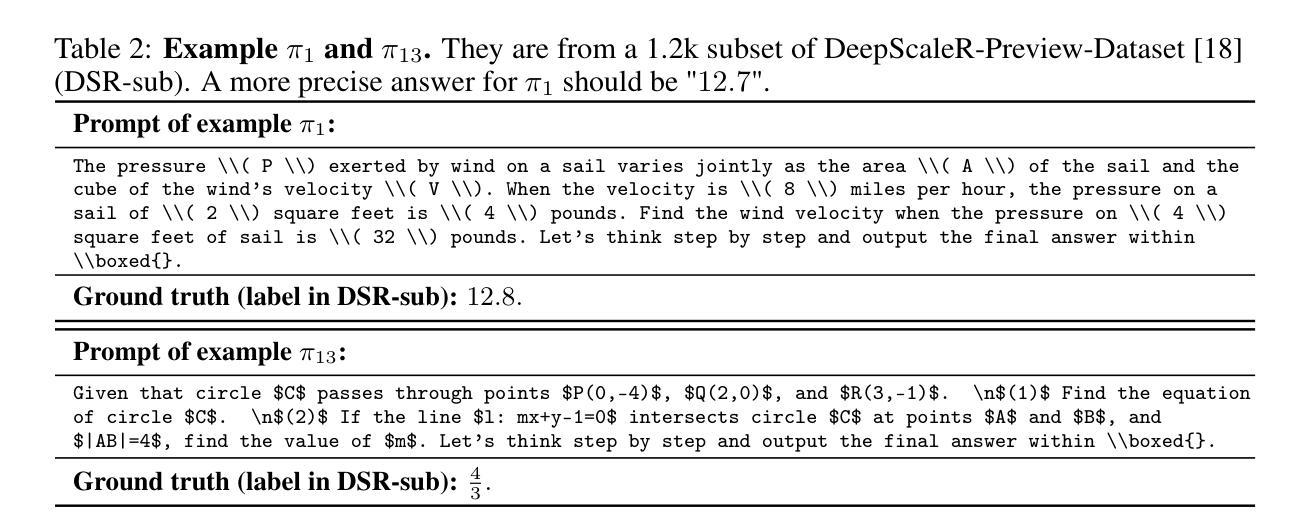
DMDTEval: An Evaluation and Analysis of LLMs on Disambiguation in Multi-domain Translation
Authors:Zhibo Man, Yuanmeng Chen, Yujie Zhang, Yufeng Chen, Jinan Xu
Currently, Large Language Models (LLMs) have achieved remarkable results in machine translation. However, their performance in multi-domain translation (MDT) is less satisfactory; the meanings of words can vary across different domains, highlighting the significant ambiguity inherent in MDT. Therefore, evaluating the disambiguation ability of LLMs in MDT remains an open problem. To this end, we present an evaluation and analysis of LLMs on disambiguation in multi-domain translation (DMDTEval), our systematic evaluation framework consisting of three critical aspects: (1) we construct a translation test set with multi-domain ambiguous word annotation, (2) we curate a diverse set of disambiguation prompting templates, and (3) we design precise disambiguation metrics, and study the efficacy of various prompting strategies on multiple state-of-the-art LLMs. Our extensive experiments reveal a number of crucial findings that we believe will pave the way and also facilitate further research in the critical area of improving the disambiguation of LLMs.
当前,大型语言模型(LLM)在机器翻译方面取得了显著成果。然而,它们在多领域翻译(MDT)方面的表现并不令人满意;词汇的含义在不同的领域可能会发生变化,这凸显了多领域翻译中固有的重大歧义问题。因此,评估LLM在多领域翻译中的消歧能力仍然是一个悬而未决的问题。为此,我们对多领域翻译中的消歧能力(DMDTEval)进行了评估和分析,这是我们系统的评估框架,包括三个关键方面:(1)我们构建了一个带有多个领域模糊词汇注释的翻译测试集,(2)我们策划了一系列多样的消歧提示模板,(3)我们设计了精确的消歧指标,并研究了多种先进的提示策略在多个前沿LLM上的有效性。我们的广泛实验揭示了一些关键发现,我们相信这些发现将为此铺平道路,并推动改进LLM消歧能力的关键领域的研究。
论文及项目相关链接
Summary
大型语言模型在机器翻译方面表现卓越,但在多领域翻译中的表现尚待提升。不同领域词汇含义的多样性导致多领域翻译中存在大量歧义。为此,我们提出了一个针对大型语言模型在多领域翻译中的词义消歧能力评估框架DMDTEval,包括构建带有多领域模糊词汇标注的翻译测试集、设计精准的词义消歧指标和研究多种提示策略对多个顶尖的大型语言模型的有效性。我们的实验揭示了重要发现,有望推动改善大型语言模型的词义消歧能力的研究。
Key Takeaways
- 大型语言模型在机器翻译上表现优秀,但在多领域翻译中表现欠佳。
- 多领域翻译中存在词汇含义的多样性,导致大量歧义问题。
- 我们提出了一个评估框架DMDTEval,针对大型语言模型在多领域翻译中的词义消歧能力进行评估。
- 构建带有多领域模糊词汇标注的翻译测试集是评估框架的核心部分。
- 评估框架还包括设计精准的词义消歧指标和研究多种提示策略对大型语言模型的影响。
- 通过对多个顶尖的大型语言模型的实验,我们发现不同的提示策略对模型效果的影响显著。
点此查看论文截图
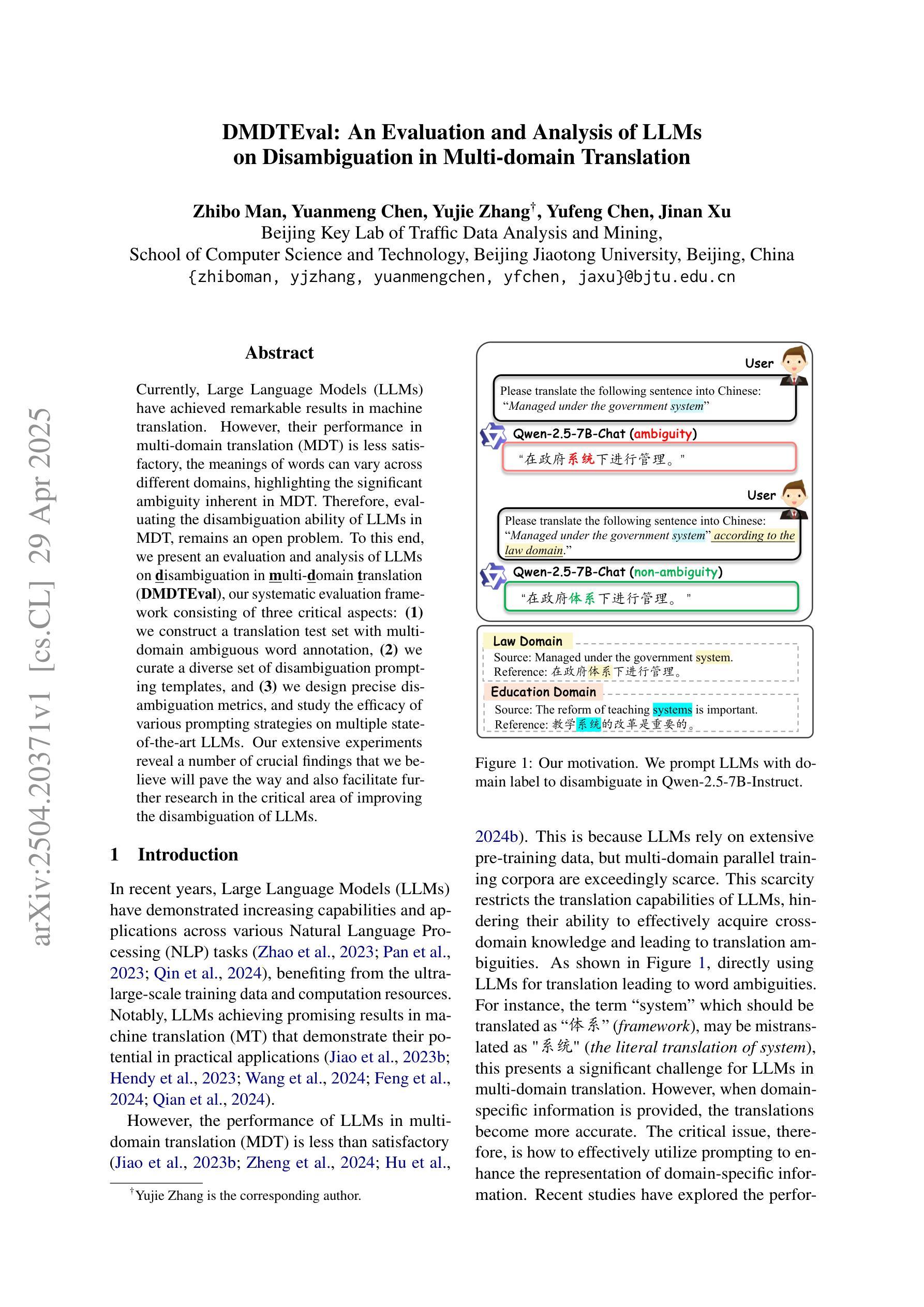
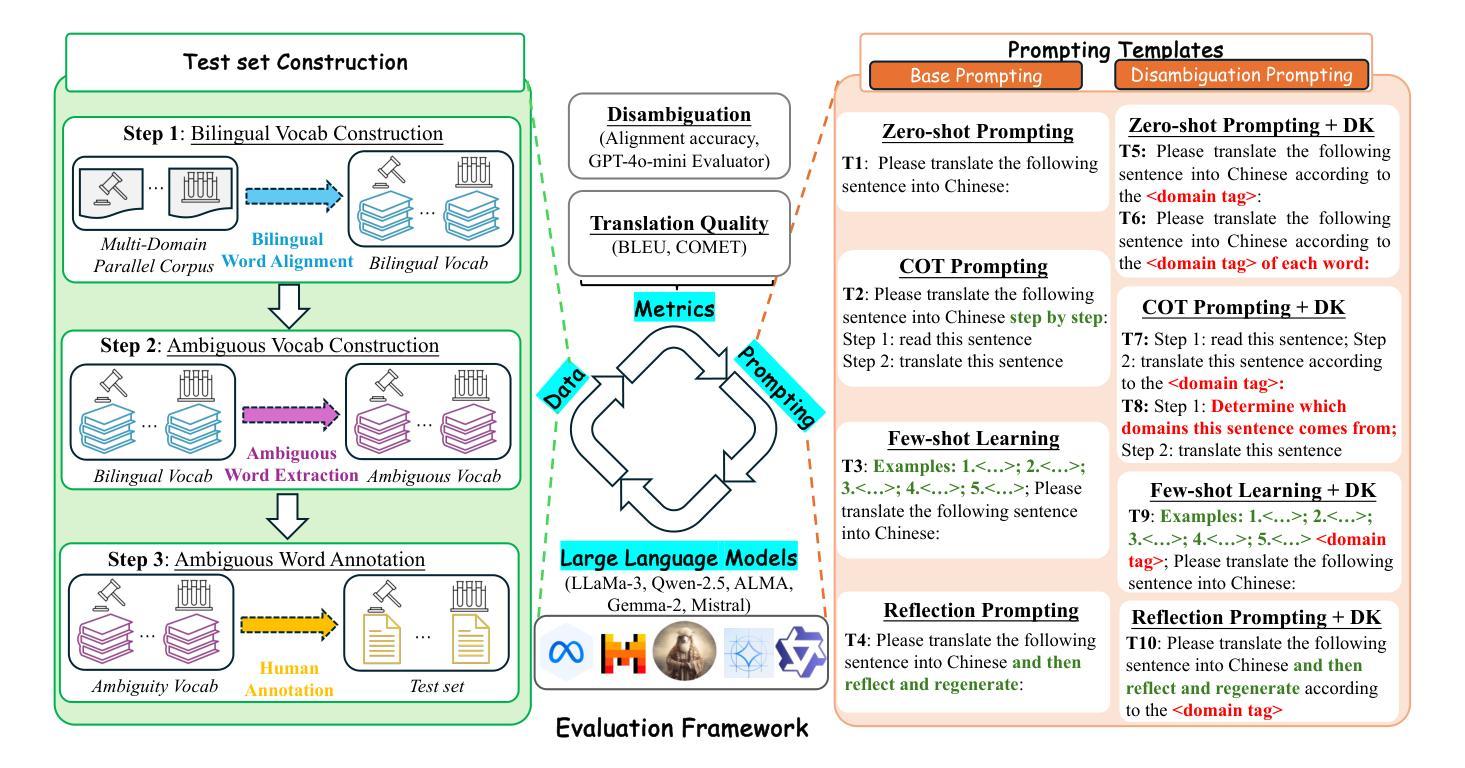
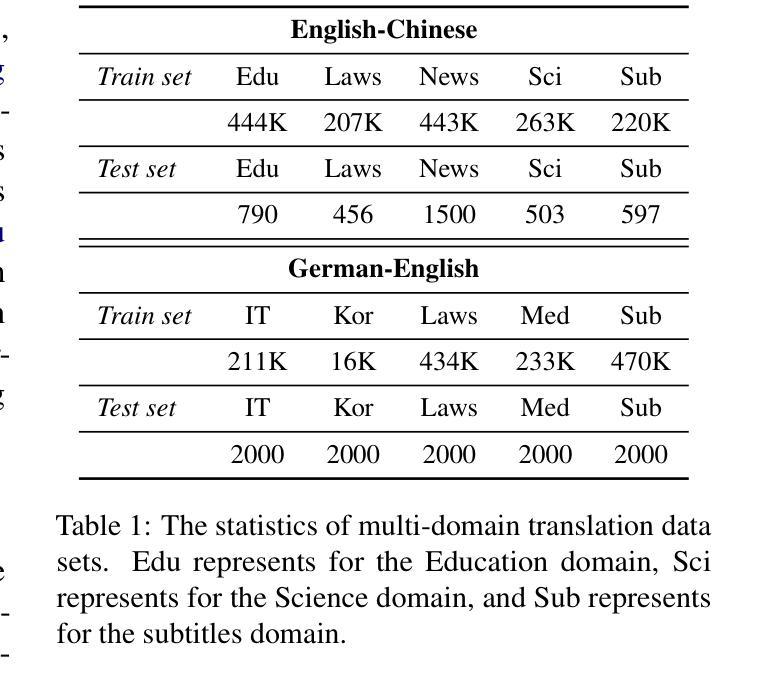
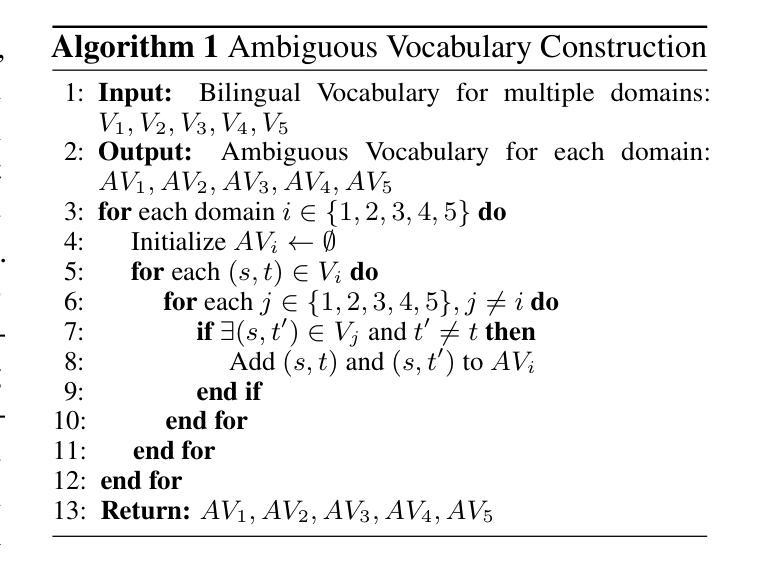
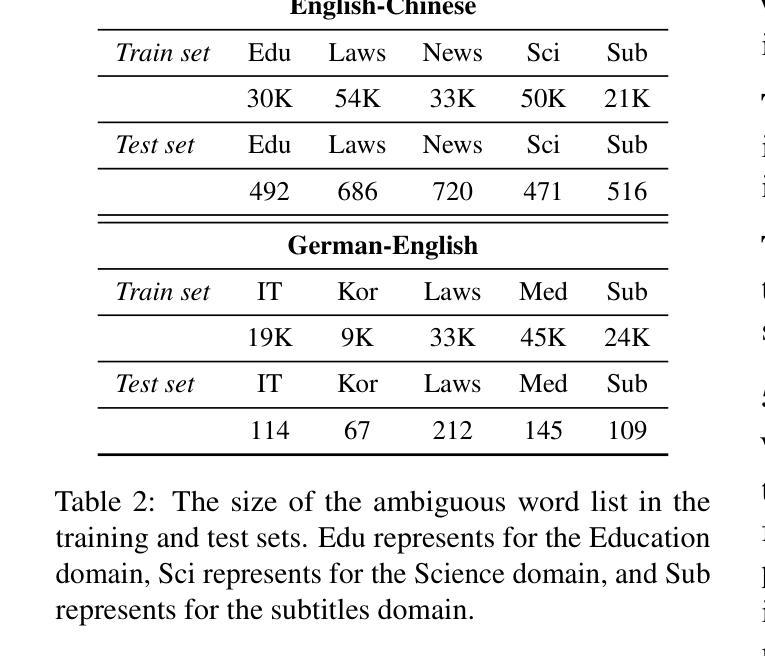
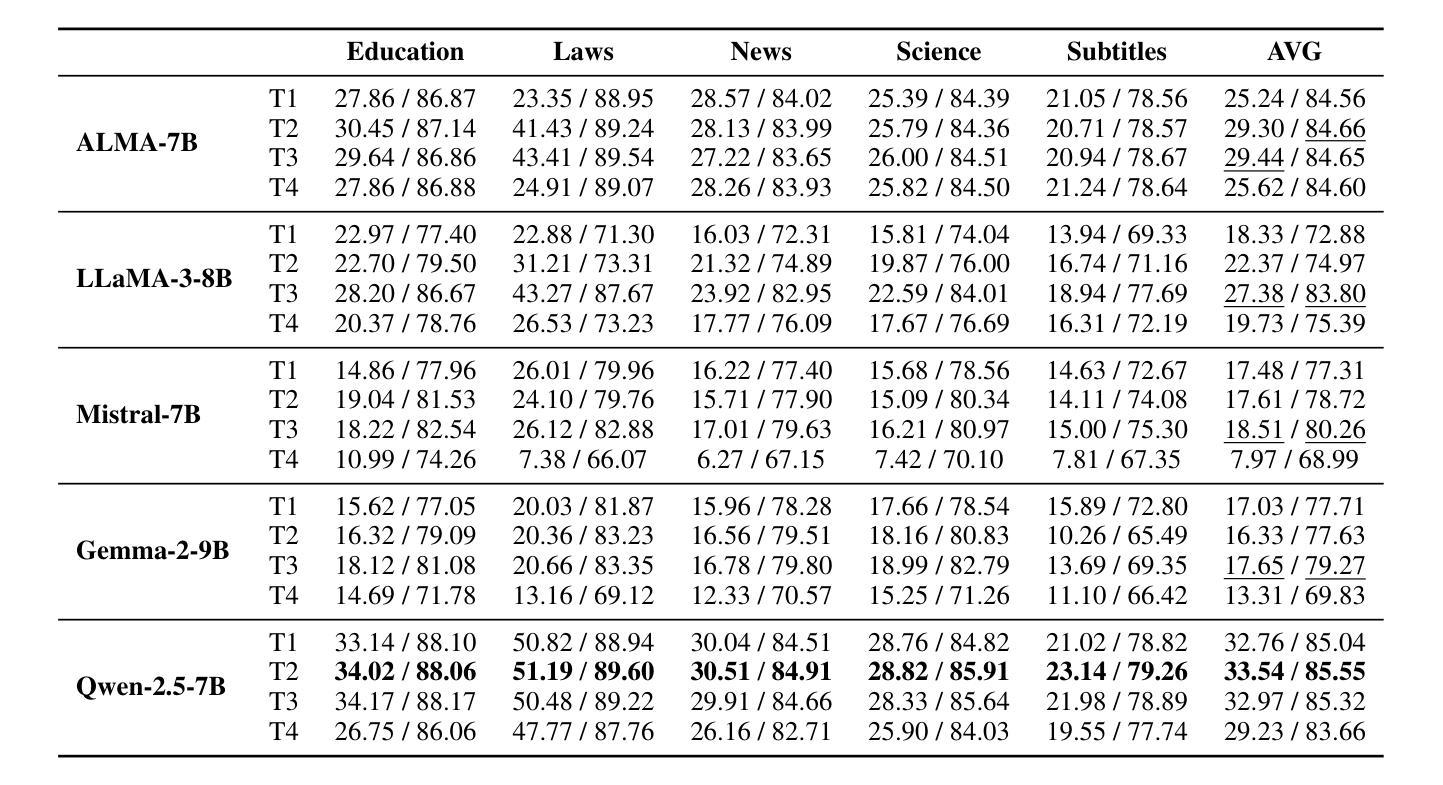
RAGEN: Understanding Self-Evolution in LLM Agents via Multi-Turn Reinforcement Learning
Authors:Zihan Wang, Kangrui Wang, Qineng Wang, Pingyue Zhang, Linjie Li, Zhengyuan Yang, Kefan Yu, Minh Nhat Nguyen, Licheng Liu, Eli Gottlieb, Monica Lam, Yiping Lu, Kyunghyun Cho, Jiajun Wu, Li Fei-Fei, Lijuan Wang, Yejin Choi, Manling Li
Training large language models (LLMs) as interactive agents presents unique challenges including long-horizon decision making and interacting with stochastic environment feedback. While reinforcement learning (RL) has enabled progress in static tasks, multi-turn agent RL training remains underexplored. We propose StarPO (State-Thinking-Actions-Reward Policy Optimization), a general framework for trajectory-level agent RL, and introduce RAGEN, a modular system for training and evaluating LLM agents. Our study on three stylized environments reveals three core findings. First, our agent RL training shows a recurring mode of Echo Trap where reward variance cliffs and gradient spikes; we address this with StarPO-S, a stabilized variant with trajectory filtering, critic incorporation, and decoupled clipping. Second, we find the shaping of RL rollouts would benefit from diverse initial states, medium interaction granularity and more frequent sampling. Third, we show that without fine-grained, reasoning-aware reward signals, agent reasoning hardly emerge through multi-turn RL and they may show shallow strategies or hallucinated thoughts. Code and environments are available at https://github.com/RAGEN-AI/RAGEN.
训练大型语言模型(LLM)作为交互代理面临着一系列独特的挑战,包括长期决策和与随机环境反馈的交互。虽然强化学习(RL)在静态任务方面取得了进展,但多轮代理RL训练仍被较少探索。我们提出了StarPO(状态-思考-行动-奖励策略优化),这是一个用于轨迹级代理RL的通用框架,并介绍了用于训练和评估LLM代理的模块化系统RAGEN。我们在三个风格化的环境中的研究揭示了三个核心发现。首先,我们的代理RL训练显示出回声陷阱的反复模式,其中奖励方差悬崖和梯度峰值;我们通过StarPO-S解决这一问题,这是一个稳定的变体,具有轨迹过滤、评论家融入和脱钩剪辑的功能。其次,我们发现RL演绎的塑造将从多样化的初始状态、中等交互粒度和更频繁的采样中受益。第三,我们表明,没有精细粒度的、理解推理的奖励信号,代理推理很难通过多轮RL出现,并且它们可能表现出浅层次的策略或幻想思维。代码和环境可在https://github.com/RAGEN-AI/RAGEN获取。
论文及项目相关链接
Summary
大型语言模型(LLM)作为交互代理人的训练面临长期决策和与随机环境反馈交互的独特挑战。强化学习(RL)在静态任务上取得了进展,但多回合代理RL训练仍被较少探索。本文提出StarPO(状态-思考-行动-奖励策略优化)框架,用于轨迹级别的代理RL,并介绍了用于训练和评估LLM代理的模块化系统RAGEN。在三个典型环境中的研究表明,核心发现包括:一是代理RL训练表现出奖励方差悬崖和梯度尖峰的回声陷阱模式;二是RL回放的优势在于多样化的初始状态、中等交互粒度和更频繁的采样;三是如果没有精细粒度的、推理感知的奖励信号,代理推理很难通过多回合RL出现,并可能表现出浅策略或虚构的思维。
Key Takeaways
- LLM作为交互代理人的训练面临独特挑战,包括长期决策和与随机环境反馈的交互。
- StarPO框架用于轨迹级别的代理RL,帮助解决多回合代理RL训练中的挑战。
- RAGEN系统用于训练和评估LLM代理。
- 代理RL训练存在回声陷阱模式,表现为奖励方差悬崖和梯度尖峰,可通过StarPO-S稳定版本解决。
- RL回放的优势在于多样化的初始状态、中等交互粒度和更频繁的采样。
- 没有精细粒度的、推理感知的奖励信号,代理难以通过多回合RL展现推理能力。
点此查看论文截图
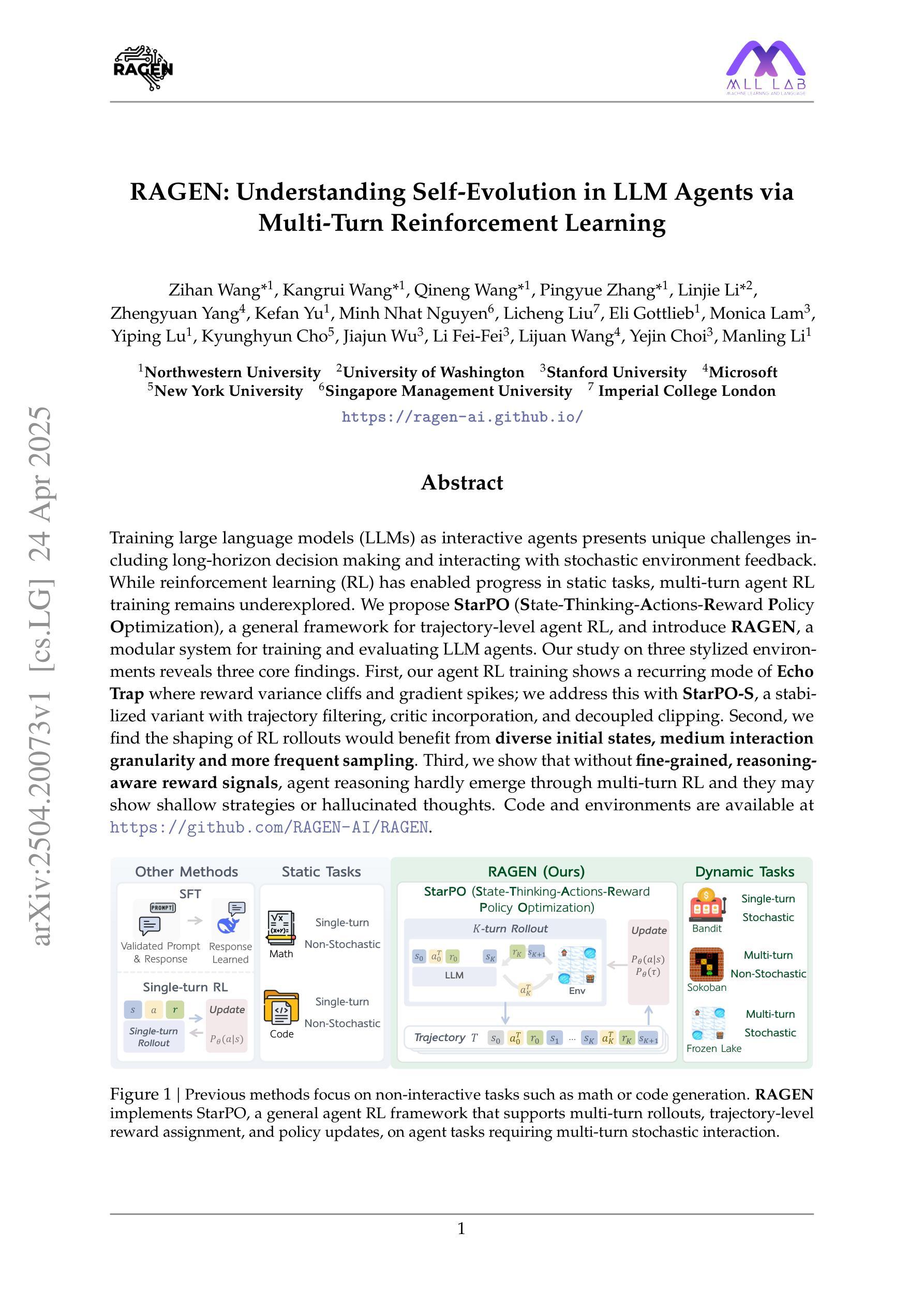
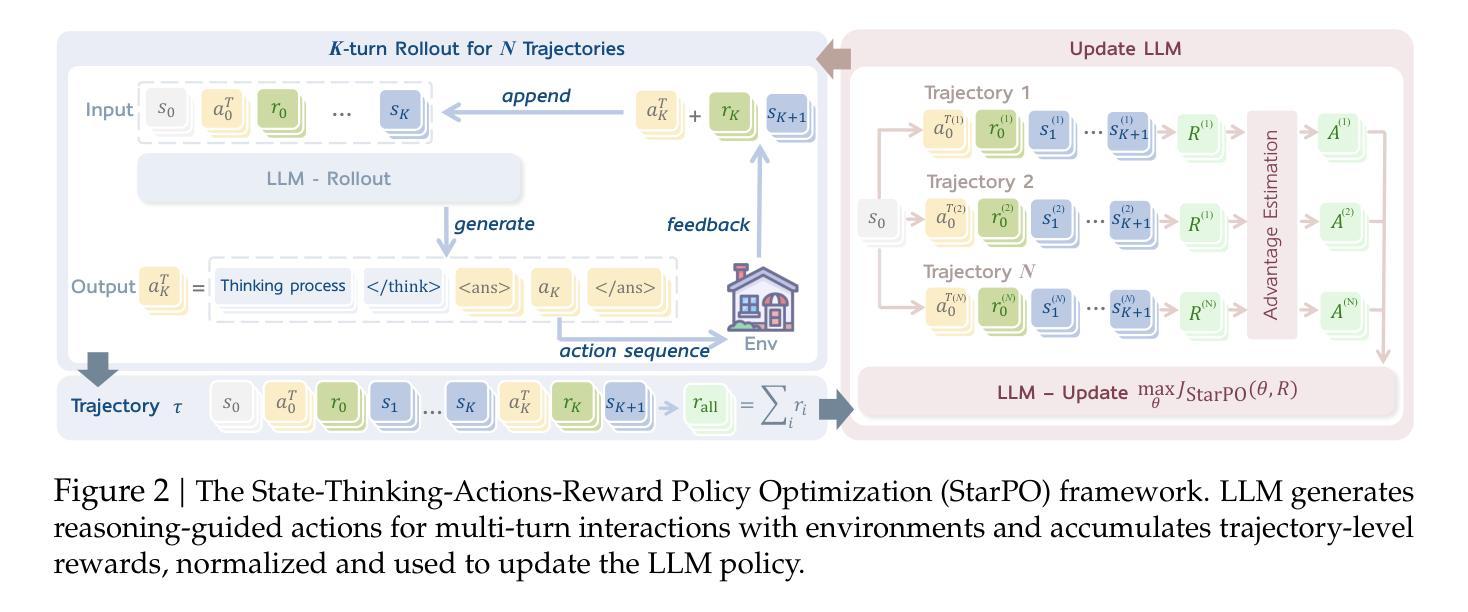
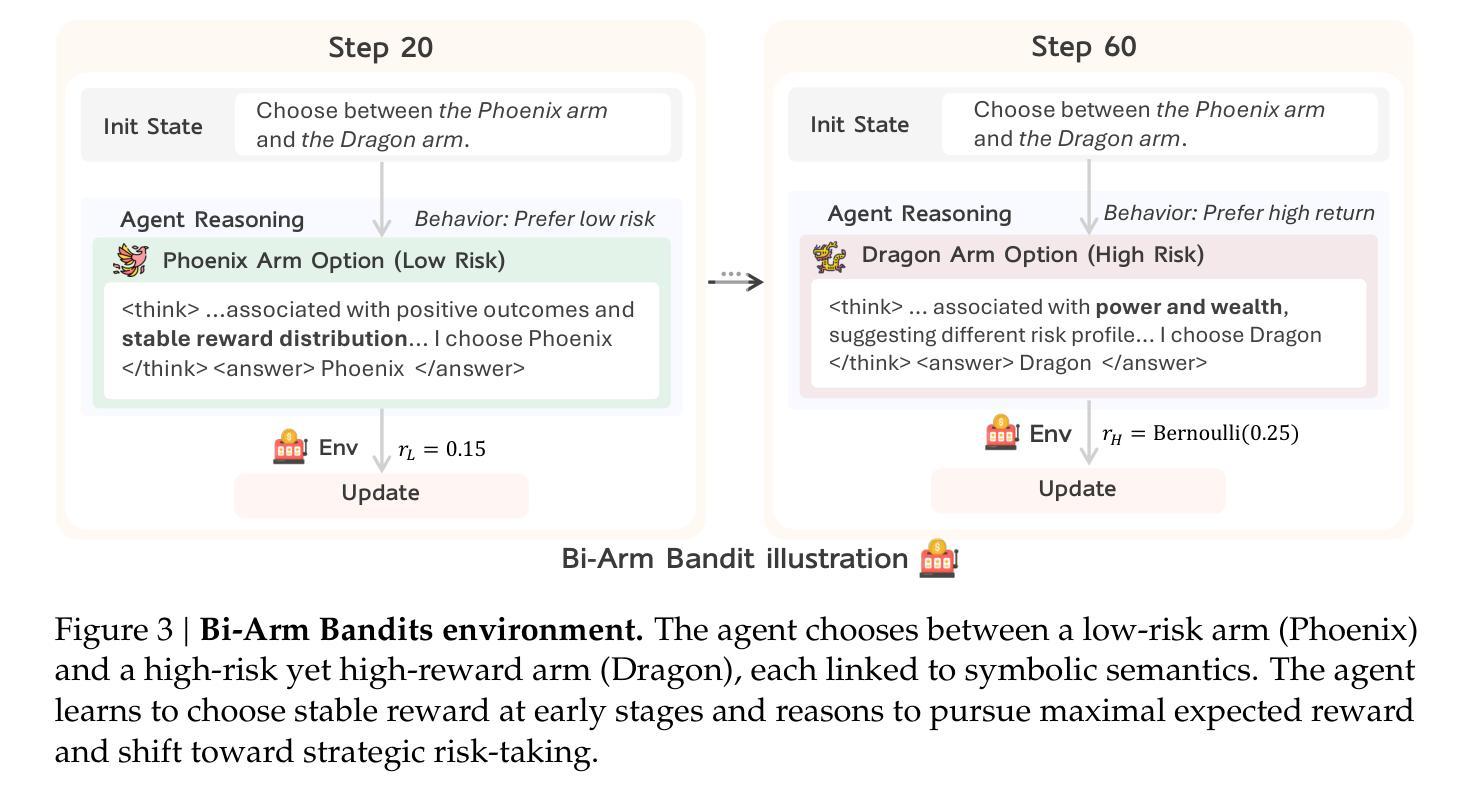
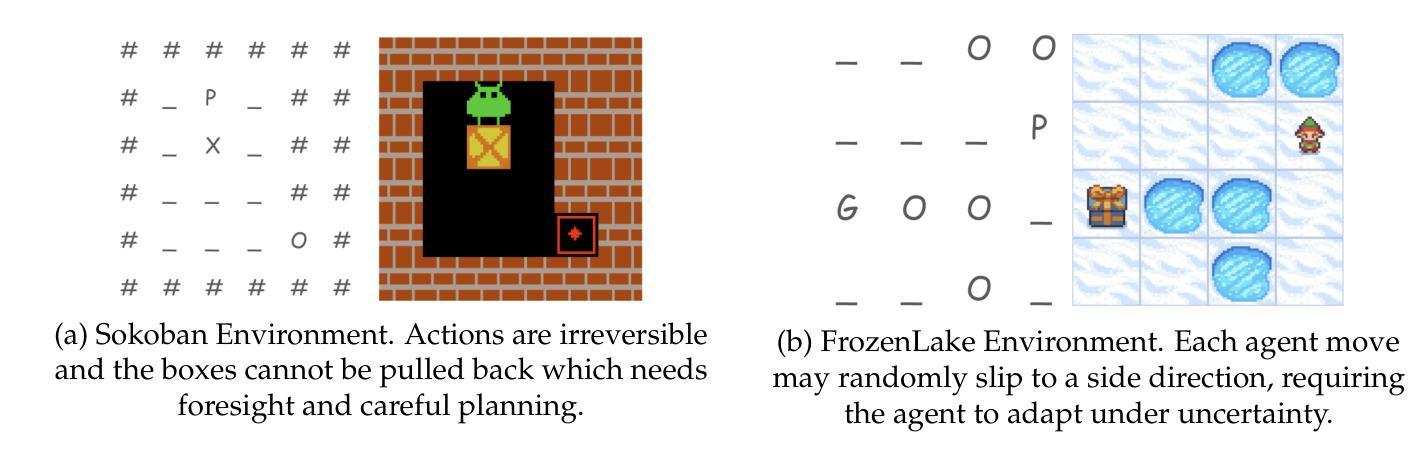
Benchmarking Multimodal Mathematical Reasoning with Explicit Visual Dependency
Authors:Zhikai Wang, Jiashuo Sun, Wenqi Zhang, Zhiqiang Hu, Xin Li, Fan Wang, Deli Zhao
Recent advancements in Large Vision-Language Models (LVLMs) have significantly enhanced their ability to integrate visual and linguistic information, achieving near-human proficiency in tasks like object recognition, captioning, and visual question answering. However, current benchmarks typically focus on knowledge-centric evaluations that assess domain-specific expertise, often neglecting the core ability to reason about fundamental mathematical elements and visual concepts. We identify a gap in evaluating elementary-level math problems, which rely on explicit visual dependencies-requiring models to discern, integrate, and reason across multiple images while incorporating commonsense knowledge, all of which are crucial for advancing toward broader AGI capabilities. To address this gap, we introduce VCBENCH, a comprehensive benchmark for multimodal mathematical reasoning with explicit visual dependencies. VCBENCH includes 1,720 problems across six cognitive domains, featuring 6,697 images (averaging 3.9 per question) to ensure multi-image reasoning. We evaluate 26 state-of-the-art LVLMs on VCBENCH, revealing substantial performance disparities, with even the top models unable to exceed 50% accuracy. Our findings highlight the ongoing challenges in visual-mathematical integration and suggest avenues for future LVLM advancements.
近期大型视觉语言模型(LVLMs)的进步,显著提高了它们整合视觉和语言信息的能力,在对象识别、标题描述和视觉问答等任务中达到了接近人类的熟练程度。然而,当前的基准测试通常侧重于以知识为中心的评价,评估特定领域的专业知识,往往忽视对基本数学元素和视觉概念的理解推理能力。我们发现了评价初级数学问题的差距,这些问题依赖于明确的视觉依赖性,要求模型能够在多个图像之间进行辨别、整合和推理,同时结合常识知识,所有这些对于实现更广泛的人工智能通用能力至关重要。为了弥补这一差距,我们引入了VCBENCH,这是一个具有明确视觉依赖性的多模式数学推理综合基准测试。VCBENCH包含1720个问题,跨越六个认知领域,包含6697张图像(平均每题3.9张图像),以确保多图像推理。我们在VCBENCH上评估了26个最先进的大型视觉语言模型,发现存在显著的性能差异,即使是最先进的模型也无法超过50%的准确率。我们的研究结果强调了视觉数学整合的持续挑战,并为未来大型视觉语言模型的进步提供了方向。
论文及项目相关链接
PDF Home page: https://alibaba-damo-academy.github.io/VCBench/
Summary
在大型视觉语言模型(LVLMs)的最新进展中,模型在整合视觉和语言信息方面的能力得到了显著提升,并在目标识别、描述和视觉问答等任务上达到了接近人类的熟练程度。然而,当前的基准测试主要集中在以知识为中心的评价上,这些评价忽略了模型对数学基础元素和视觉概念的推理能力。为解决此评估空白,本文推出了VCBENCH基准测试,这是一个包含明确视觉依赖的多模态数学推理的综合基准测试。我们发现顶尖模型在该基准上的表现差距明显,无法超越一半的准确率,揭示了视觉数学整合方面的持续挑战和未来发展方向。
Key Takeaways
- 大型视觉语言模型(LVLMs)已具备强大的视觉和语言信息整合能力。
- 当前基准测试主要关注知识领域的评价,但对数学基础元素和视觉概念的推理能力评估不足。
- 为解决评估空白,引入了VCBENCH基准测试,专注于多模态数学推理并包含明确的视觉依赖性。
- VCBENCH包含1,720个问题,涉及六个认知领域,使用6,697张图像确保多图像推理。
- 在VCBENCH上评估的26个最先进的LVLMs表现存在显著差异,顶尖模型的准确率无法超过50%。
- 结果表明在视觉数学整合方面存在持续挑战。
点此查看论文截图
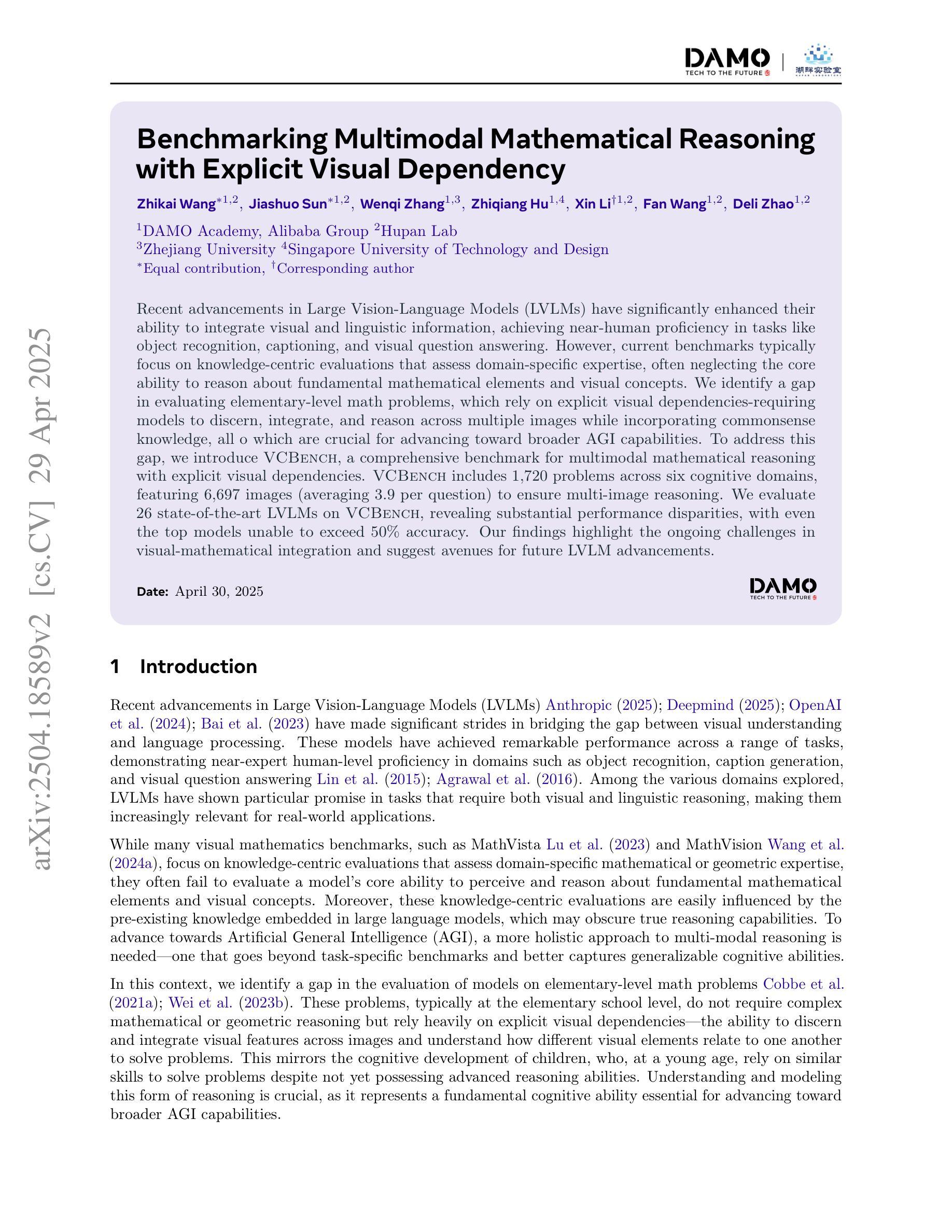
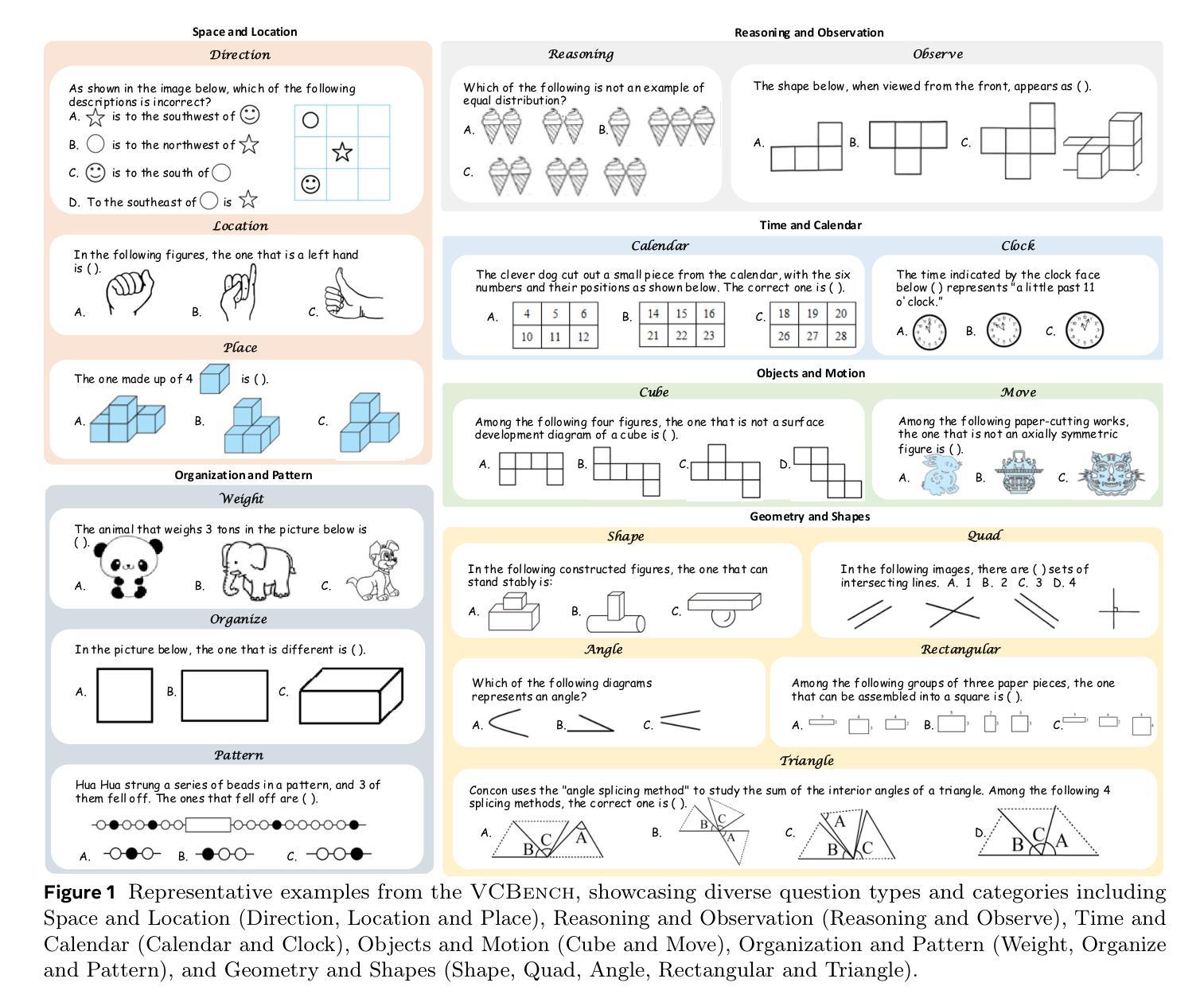
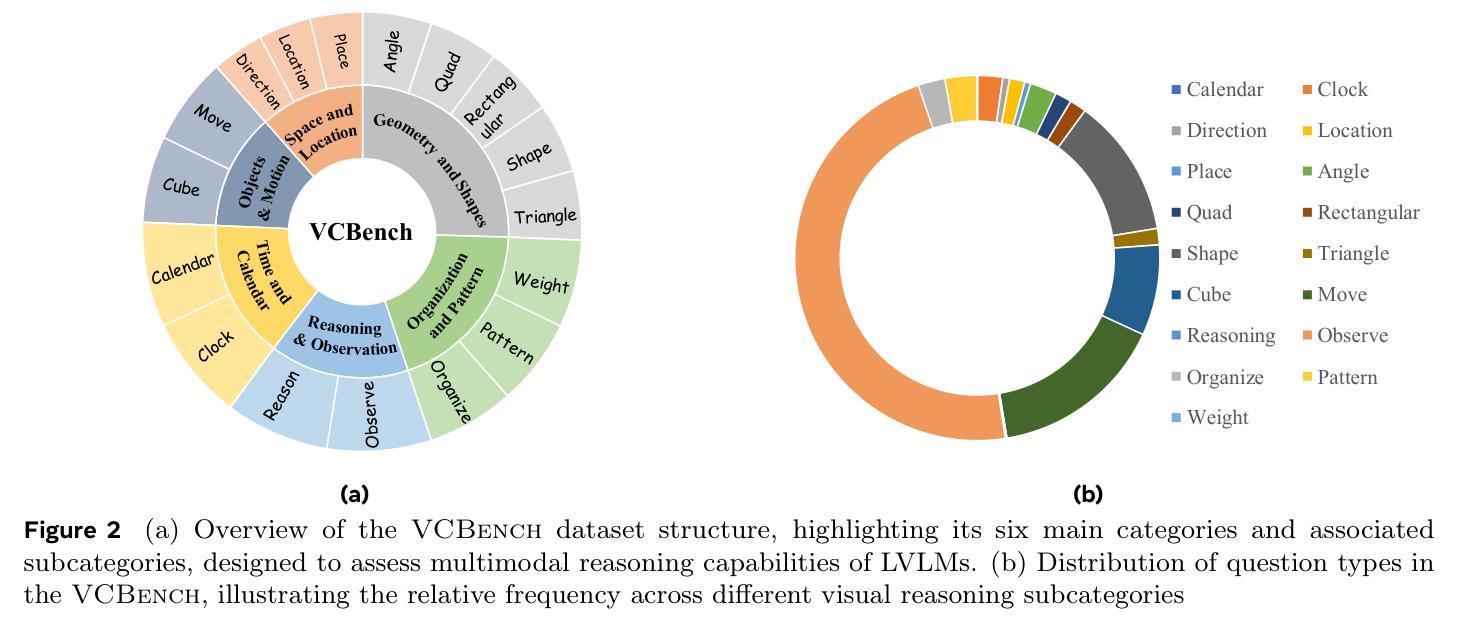
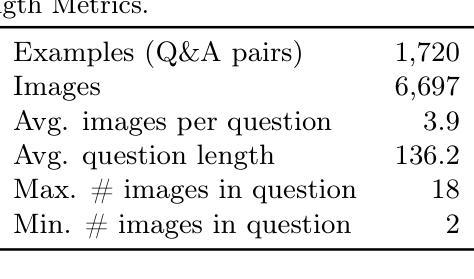
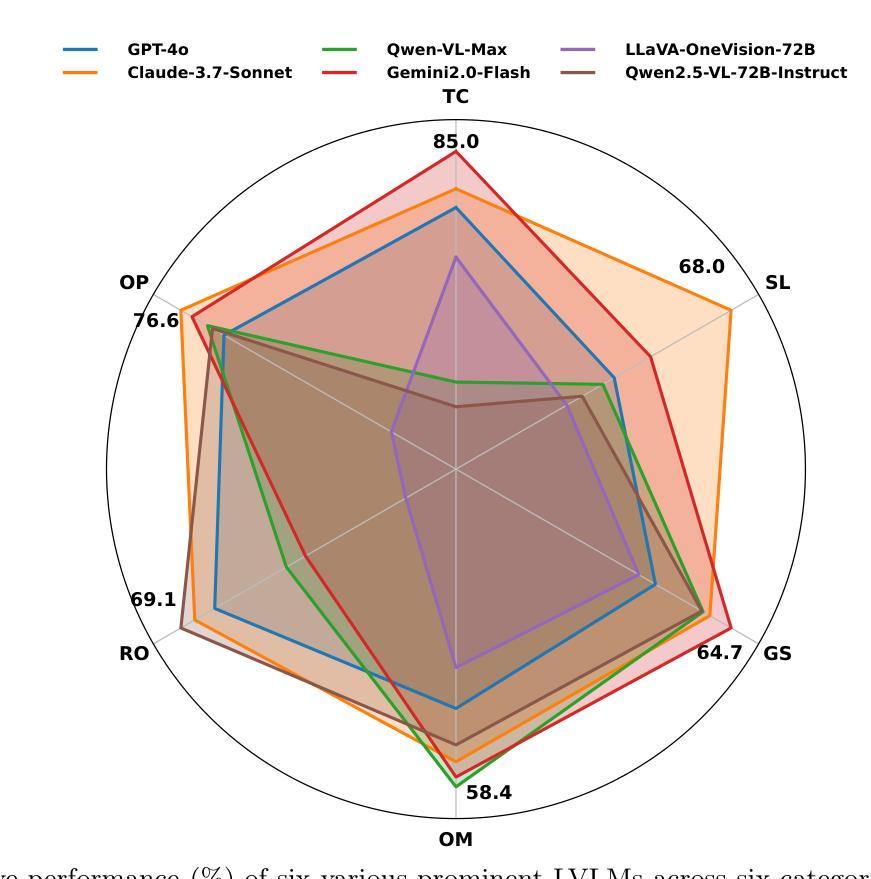
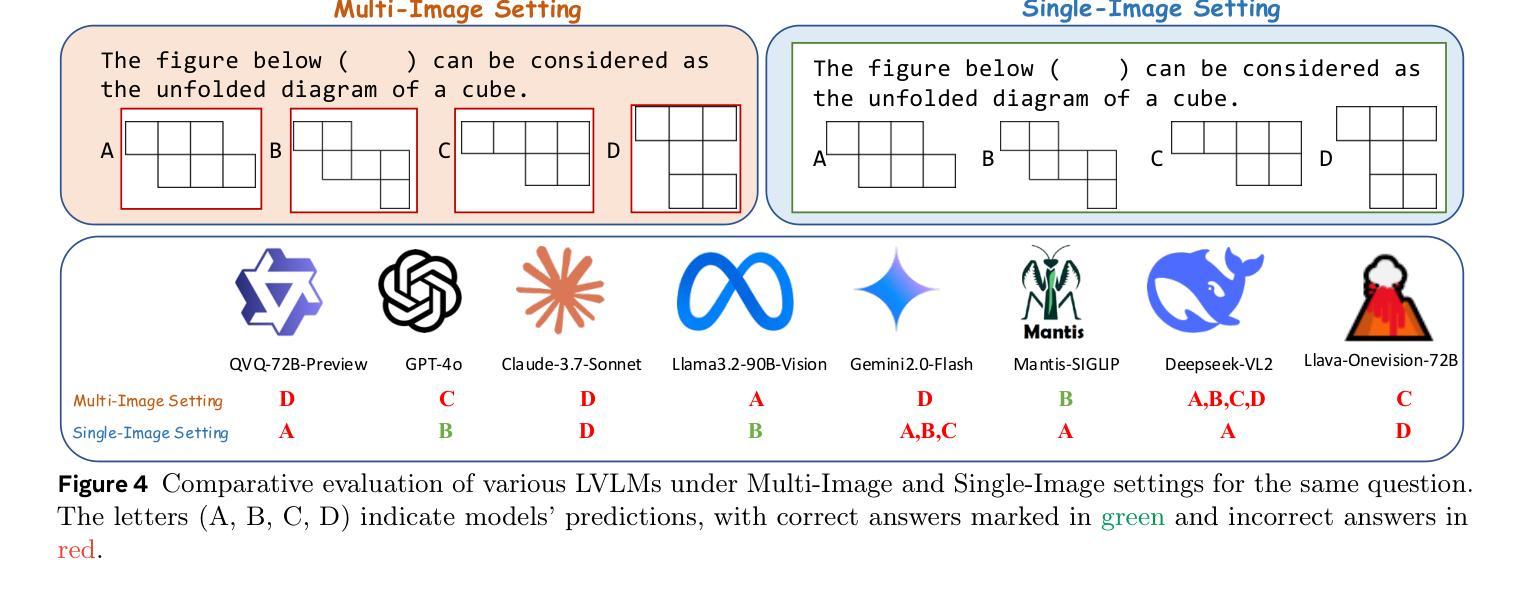
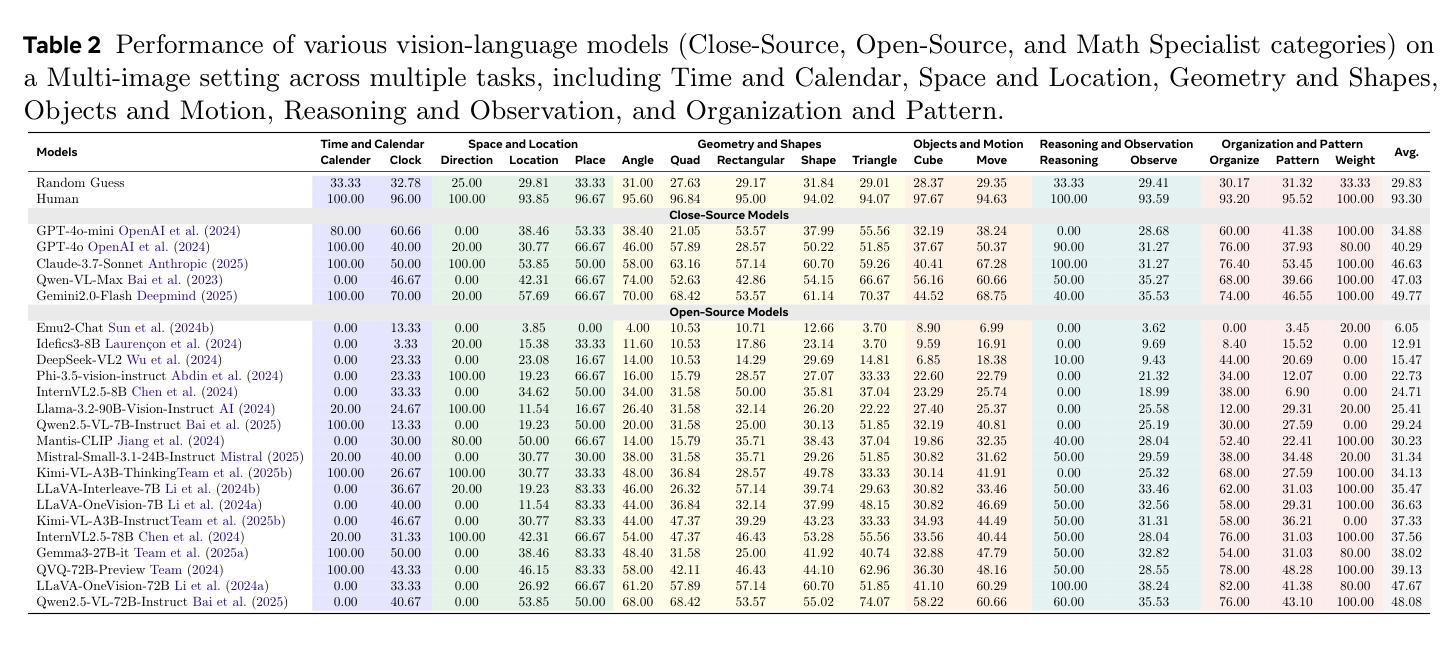
SARI: Structured Audio Reasoning via Curriculum-Guided Reinforcement Learning
Authors:Cheng Wen, Tingwei Guo, Shuaijiang Zhao, Wei Zou, Xiangang Li
Recent work shows that reinforcement learning(RL) can markedly sharpen the reasoning ability of large language models (LLMs) by prompting them to “think before answering.” Yet whether and how these gains transfer to audio-language reasoning remains largely unexplored. We extend the Group-Relative Policy Optimization (GRPO) framework from DeepSeek-R1 to a Large Audio-Language Model (LALM), and construct a 32k sample multiple-choice corpus. Using a two-stage regimen supervised fine-tuning on structured and unstructured chains-of-thought, followed by curriculum-guided GRPO, we systematically compare implicit vs. explicit, and structured vs. free form reasoning under identical architectures. Our structured audio reasoning model, SARI (Structured Audio Reasoning via Curriculum-Guided Reinforcement Learning), achieves a 16.35% improvement in average accuracy over the base model Qwen2-Audio-7B-Instruct. Furthermore, the variant built upon Qwen2.5-Omni reaches state-of-the-art performance of 67.08% on the MMAU test-mini benchmark. Ablation experiments show that on the base model we use: (i) SFT warm-up is important for stable RL training, (ii) structured chains yield more robust generalization than unstructured ones, and (iii) easy-to-hard curricula accelerate convergence and improve final performance. These findings demonstrate that explicit, structured reasoning and curriculum learning substantially enhances audio-language understanding.
最近的研究表明,通过提示“答题前先思考”,强化学习(RL)可以显著提高大语言模型(LLM)的推理能力。然而,这些收益是否以及如何转移到音频语言推理领域,仍然在很大程度上未被探索。我们将DeepSeek-R1的Group-Relative Policy Optimization(GRPO)框架扩展到一个大型音频语言模型(LALM),并构建了一个32k样本的多选择语料库。通过结构化和非结构化思维链的两阶段监管监督微调,再加上课程指导的GRPO,我们系统地比较了隐式和显式、结构化和自由形式推理在相同架构下的表现。我们的结构化音频推理模型SARI(通过课程指导强化学习的结构化音频推理)在基准模型Qwen2-Audio-7B-Instruct的基础上实现了平均精度16.35%的提升。此外,基于Qwen2.5-Omni的变体在MMAU test-mini基准测试中达到了最先进的67.08%的性能。消融实验表明,在我们使用的基准模型上:(i)SFT预热对于稳定的RL训练很重要,(ii)结构化链条相比非结构化链条能产生更稳健的泛化性能,(iii)从易到难的课程加速收敛并提高了最终性能。这些发现表明,明确的、结构化的推理和课程学习显著增强了音频语言理解。
论文及项目相关链接
Summary
本文探索了强化学习(RL)在提升大型音频语言模型(LALM)的推理能力方面的应用。通过结合Group-Relative Policy Optimization(GRPO)框架和结构化音频推理模型SARI,研究发现强化学习可以显著提高模型的推理能力。实验结果显示,相比基础模型Qwen2-Audio-7B-Instruct,使用SARI模型的平均准确率提高了16.35%。此外,结合Qwen2.5-Omni的变体模型在MMAU测试迷你版上达到了最先进的性能水平。研究还发现,结构化链条比非结构化链条更有助于模型的泛化能力,并且从易到难的课程安排可以加速模型的收敛并提高最终性能。这些发现表明,明确的、结构化的推理和课程学习可以显著提高音频语言的理解能力。
Key Takeaways
- 强化学习能够显著提升大型音频语言模型的推理能力。
- Group-Relative Policy Optimization(GRPO)框架被成功应用于音频语言模型中。
- 通过结合结构化音频推理模型SARI,模型性能得到了提高。
- 对比基础模型,使用SARI模型的平均准确率提高了16.35%。
- 结合Qwen2.5-Omni的变体模型在MMAU测试上取得了最先进的性能。
- 结构化链条的推理方式有助于模型的泛化能力。
点此查看论文截图
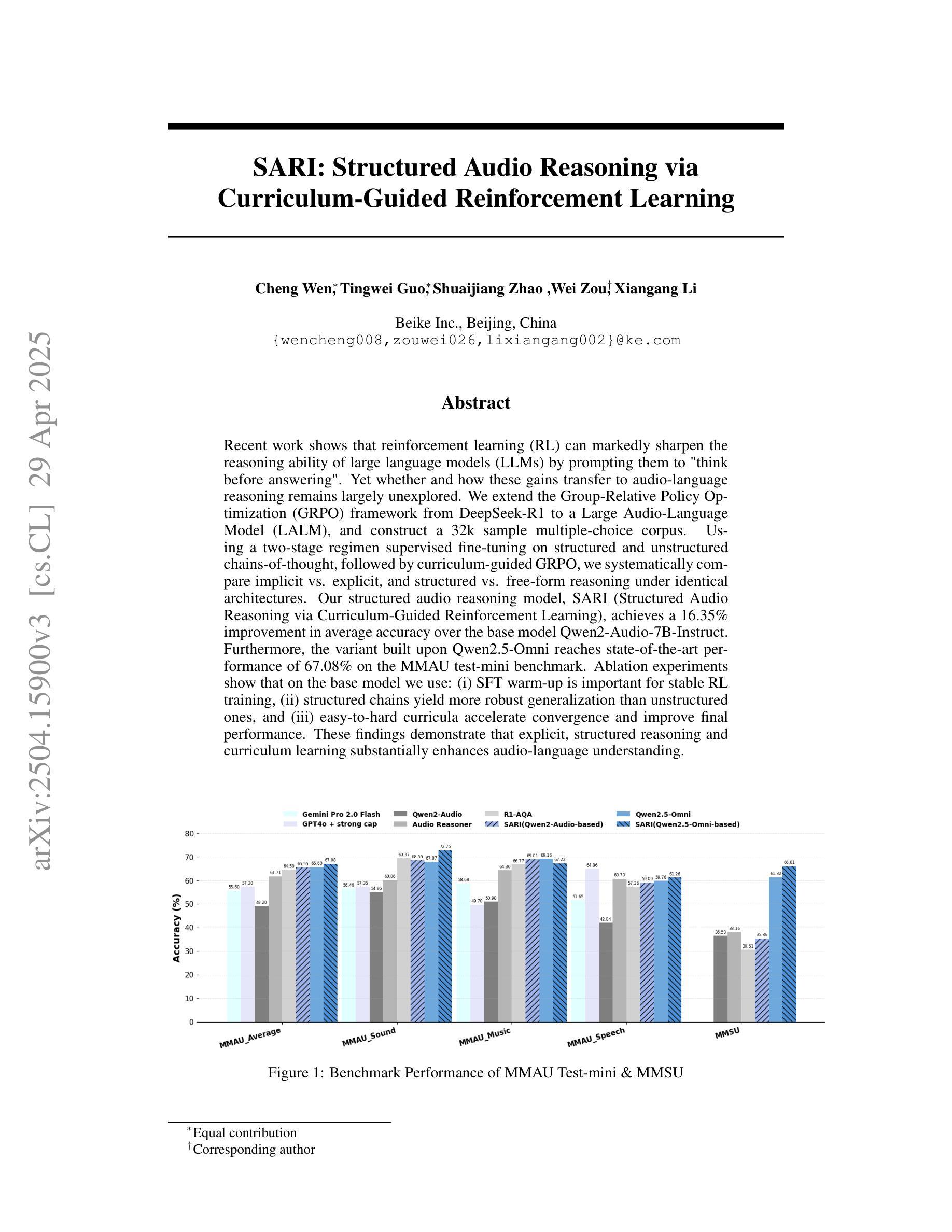
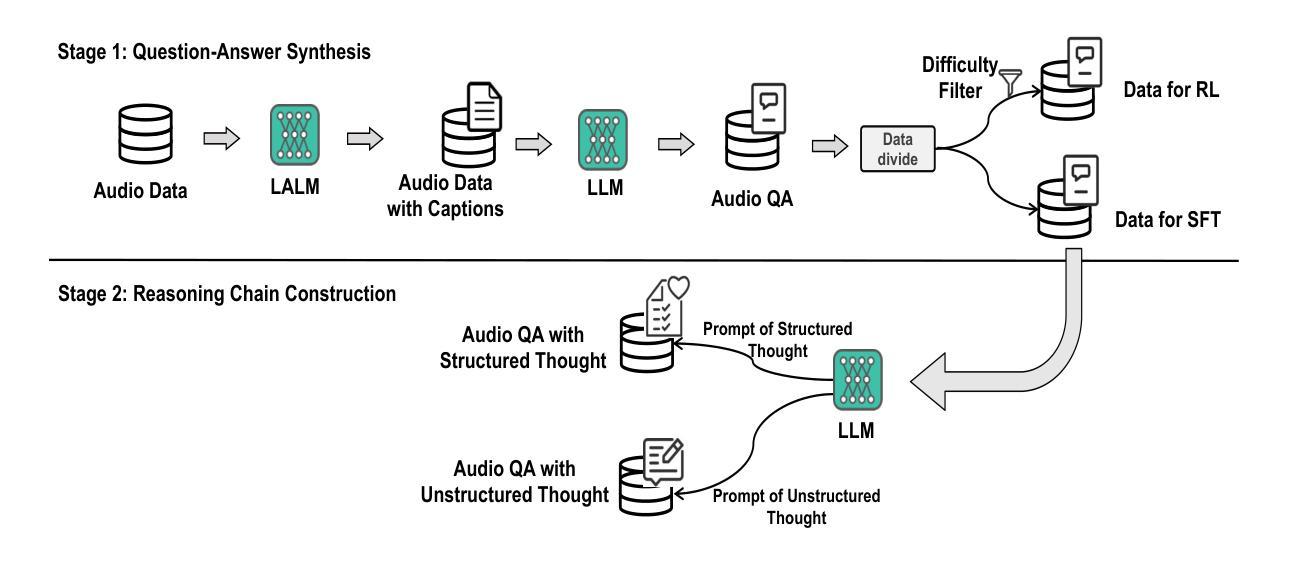
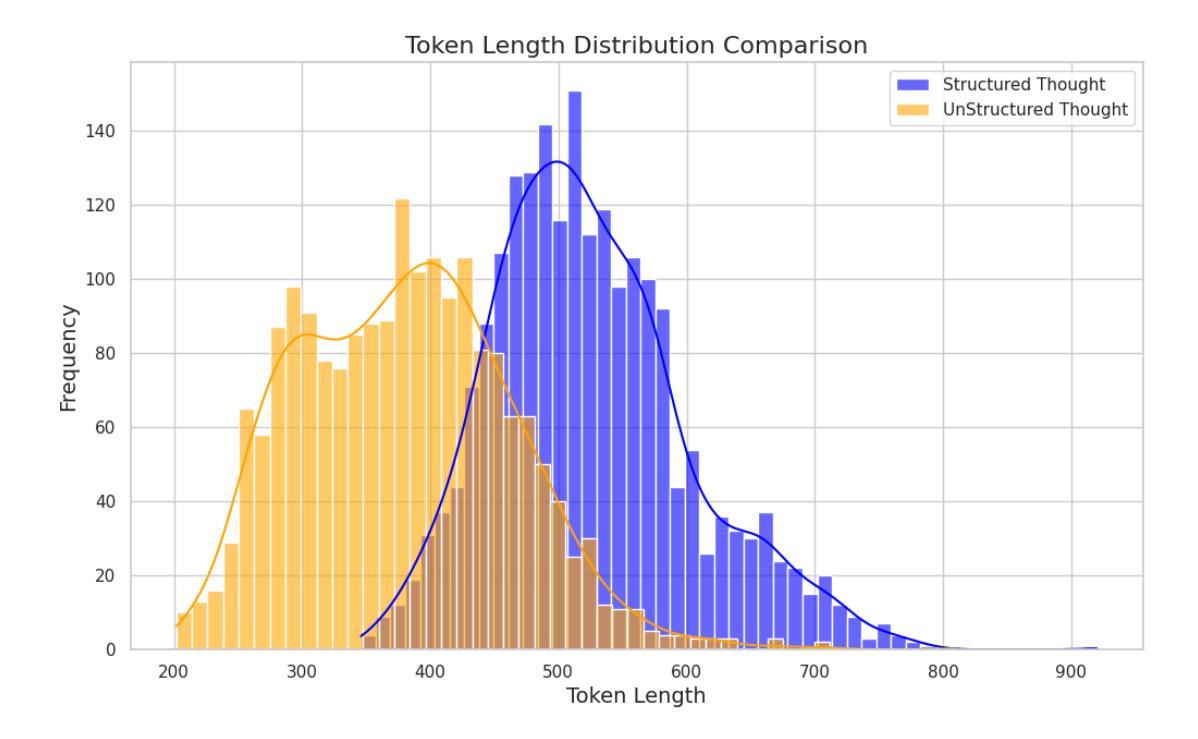
Seed1.5-Thinking: Advancing Superb Reasoning Models with Reinforcement Learning
Authors:ByteDance Seed, :, Jiaze Chen, Tiantian Fan, Xin Liu, Lingjun Liu, Zhiqi Lin, Mingxuan Wang, Chengyi Wang, Xiangpeng Wei, Wenyuan Xu, Yufeng Yuan, Yu Yue, Lin Yan, Qiying Yu, Xiaochen Zuo, Chi Zhang, Ruofei Zhu, Zhecheng An, Zhihao Bai, Yu Bao, Xingyan Bin, Jiangjie Chen, Feng Chen, Hongmin Chen, Riwei Chen, Liangqiang Chen, Zixin Chen, Jinsong Chen, Siyan Chen, Kaiyuan Chen, Zhi Chen, Jin Chen, Jiecao Chen, Jinxin Chi, Weinan Dai, Ning Dai, Jiahui Dai, Shihan Dou, Yantao Du, Zhengyin Du, Jianhui Duan, Chen Dun, Ting-Han Fan, Jiazhan Feng, Junda Feng, Ziyuan Feng, Yuwei Fu, Wenqi Fu, Hanjie Fu, Hao Ge, Hongyi Guo, Mingji Han, Li Han, Wenhao Hao, Xintong Hao, Qianyu He, Jerry He, Feng He, Wen Heng, Zehua Hong, Qi Hou, Liang Hu, Shengding Hu, Nan Hu, Kai Hua, Qi Huang, Ziyue Huang, Hongzhi Huang, Zihao Huang, Ting Huang, Wenhao Huang, Wei Jia, Bin Jia, Xiaoying Jia, Yuhua Jiang, Haobin Jiang, Ziheng Jiang, Kaihua Jiang, Chengquan Jiang, Jianpeng Jiao, Xiaoran Jin, Xing Jin, Xunhao Lai, Zheng Li, Xiang Li, Liyi Li, Hongkai Li, Zheng Li, Shengxian Wan, Ya Wang, Yunshui Li, Chenggang Li, Niuniu Li, Siyu Li, Xi Li, Xiao Li, Aoyan Li, Yuntao Li, Nianning Liang, Xinnian Liang, Haibin Lin, Weijian Lin, Ye Lin, Zhicheng Liu, Guanlin Liu, Guanlin Liu, Chenxiao Liu, Yan Liu, Gaohong Liu, Juncai Liu, Chundian Liu, Deyi Liu, Kaibo Liu, Siyao Liu, Qi Liu, Yongfei Liu, Kang Liu, Gan Liu, Boyi Liu, Rui Long, Weiqiang Lou, Chenwei Lou, Xiang Luo, Yao Luo, Caiping Lv, Heyang Lv, Bole Ma, Qianli Ma, Hongzhi Ma, Yiyuan Ma, Jin Ma, Wenchang Ma, Tingting Ma, Chen Mao, Qiyang Min, Zhe Nan, Guanghan Ning, Jinxiang Ou, Haojie Pan, Renming Pang, Yanghua Peng, Tao Peng, Lihua Qian, Lihua Qian, Mu Qiao, Meng Qu, Cheng Ren, Hongbin Ren, Yong Shan, Wei Shen, Ke Shen, Kai Shen, Guangming Sheng, Jinlong Shi, Wenlei Shi, Guang Shi, Shuai Shuai Cao, Yuxin Song, Zuquan Song, Jing Su, Yifan Sun, Tao Sun, Zewei Sun, Borui Wan, Zihan Wang, Xiaohui Wang, Xi Wang, Shuguang Wang, Jun Wang, Qinlong Wang, Chenyuan Wang, Shuai Wang, Zihan Wang, Changbao Wang, Jiaqiang Wang, Shihang Wang, Xuwu Wang, Zaiyuan Wang, Yuxuan Wang, Wenqi Wang, Taiqing Wang, Chengzhi Wei, Houmin Wei, Ziyun Wei, Shufa Wei, Zheng Wu, Yonghui Wu, Yangjun Wu, Bohong Wu, Shuang Wu, Jingqiao Wu, Ning Wu, Shuangzhi Wu, Jianmin Wu, Chenguang Xi, Fan Xia, Yuqiao Xian, Liang Xiang, Boren Xiang, Bowen Xiao, Zhen Xiao, Xia Xiao, Yongsheng Xiao, Chao Xin, Shulin Xin, Yuwen Xiong, Jingjing Xu, Ziwen Xu, Chenyin Xu, Jiayi Xu, Yifan Xu, Wei Xu, Yufei Xu, Shikun Xu, Shipeng Yan, Shen Yan, Qingping Yang, Xi Yang, Tianhao Yang, Yuehang Yang, Yuan Yang, Ximing Yang, Zeyu Yang, Guang Yang, Yifan Yang, Xuesong Yao, Bairen Yi, Fan Yin, Jianian Yin, Ziqiang Ying, Xiangyu Yu, Hongli Yu, Song Yu, Menghan Yu, Huan Yu, Siyu Yuan, Jun Yuan, Yutao Zeng, Tianyang Zhan, Zheng Zhang, Yun Zhang, Mofan Zhang, Wang Zhang, Ru Zhang, Zhi Zhang, Tianqi Zhang, Xinyi Zhang, Zhexi Zhang, Sijun Zhang, Wenqiang Zhang, Xiangxiang Zhang, Yongtao Zhang, Yuyu Zhang, Ge Zhang, He Zhang, Yue Zhang, Renjie Zheng, Ningxin Zheng, Zhuolin Zheng, Yaowei Zheng, Chen Zheng, Xiaoyun Zhi, Wanjun Zhong, Cheng Zhong, Zheng Zhong, Baoquan Zhong, Xun Zhou, Na Zhou, Huan Zhou, Hang Zhu, Defa Zhu, Wenjia Zhu, Lei Zuo
We introduce Seed1.5-Thinking, capable of reasoning through thinking before responding, resulting in improved performance on a wide range of benchmarks. Seed1.5-Thinking achieves 86.7 on AIME 2024, 55.0 on Codeforces and 77.3 on GPQA, demonstrating excellent reasoning abilities in STEM and coding. Beyond reasoning tasks, the method demonstrates notable generalization across diverse domains. For instance, it surpasses DeepSeek R1 by 8% in win rate on non-reasoning tasks, indicating its broader applicability. Compared to other state-of-the-art reasoning models, Seed1.5-Thinking is a Mixture-of-Experts (MoE) model with a relatively small size, featuring 20B activated and 200B total parameters. As part of our effort to assess generalized reasoning, we develop two internal benchmarks, BeyondAIME and Codeforces, both of which will be publicly released to support future research. Model trial link: https://www.volcengine.com/experience/ark.
我们推出Seed1.5-Thinking,它能够在回应之前通过思考进行推理,从而大大提高在各种基准测试上的表现。Seed1.5-Thinking在AIME 2024上达到86.7分,在Codeforces上达到55.0分,在GPQA上达到77.3分,展现出STEM和编码领域的出色推理能力。除了推理任务之外,该方法在不同领域之间展现出显著的泛化能力。例如,在非推理任务的胜率上,它超越了DeepSeek R1的8%,表明其更广泛的应用性。与其他最先进的推理模型相比,Seed1.5-Thinking是一个相对较小的混合专家(MoE)模型,具有20B激活和总共的200B参数。作为我们评估通用推理努力的一部分,我们开发了两个内部基准测试,BeyondAIME和Codeforces,这两个基准测试都将公开发布,以支持未来的研究。模型试用链接:https://www.volcengine.com/experience/ark。
论文及项目相关链接
Summary
本文介绍了Seed1.5-Thinking模型,该模型具备预先思考再回应的推理能力,在各种基准测试中表现出卓越的性能。在STEM和编码领域的推理能力特别突出,在AIME 2024上达到86.7,Codeforces上为55.0,GPQA上为77.3。此外,该模型在非推理任务上也表现出较高的胜率,超越了DeepSeek R1模型8%。它是一个相对较小的MoE模型,拥有20B激活参数和200B总参数。为评估通用推理能力,研究团队开发了BeyondAIME和Codeforces两个内部基准测试,并将公开发布以支持未来研究。
Key Takeaways
- Seed1.5-Thinking模型具备先进的推理能力,通过预先思考再回应,提高了在各种基准测试中的表现。
- 在STEM和编码领域的基准测试中,Seed1.5-Thinking表现优秀,如AIME 2024、Codeforces和GPQA。
- 该模型在非推理任务上也表现出良好的泛化能力,超越了DeepSeek R1模型8%。
- Seed1.5-Thinking是一个相对较小的MoE模型,拥有20B激活参数和200B总参数。
- 研究团队为评估通用推理能力,开发了两个新的内部基准测试BeyondAIME和Codeforces。
- Seed1.5-Thinking模型具备较好的应用价值,其试验链接已公开。
点此查看论文截图
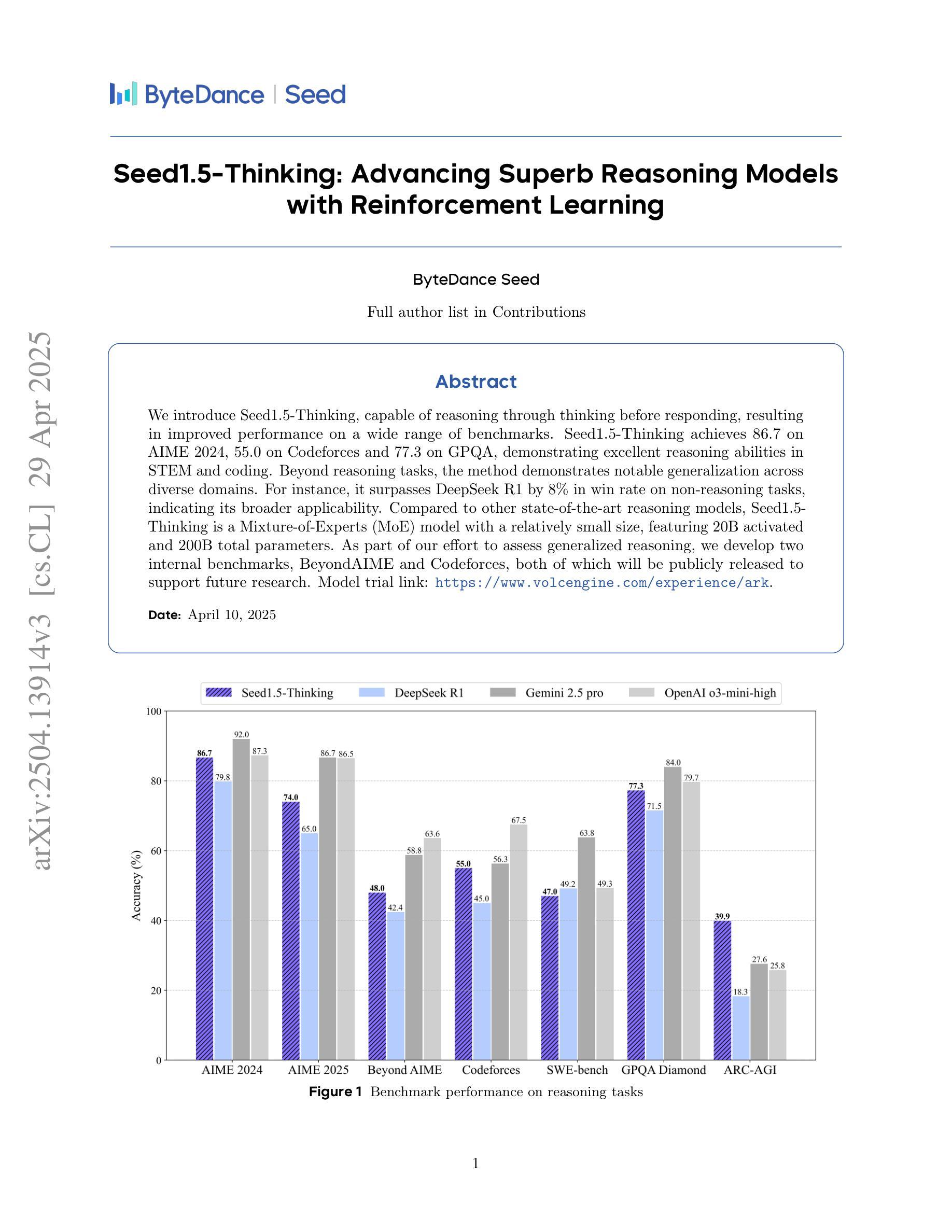
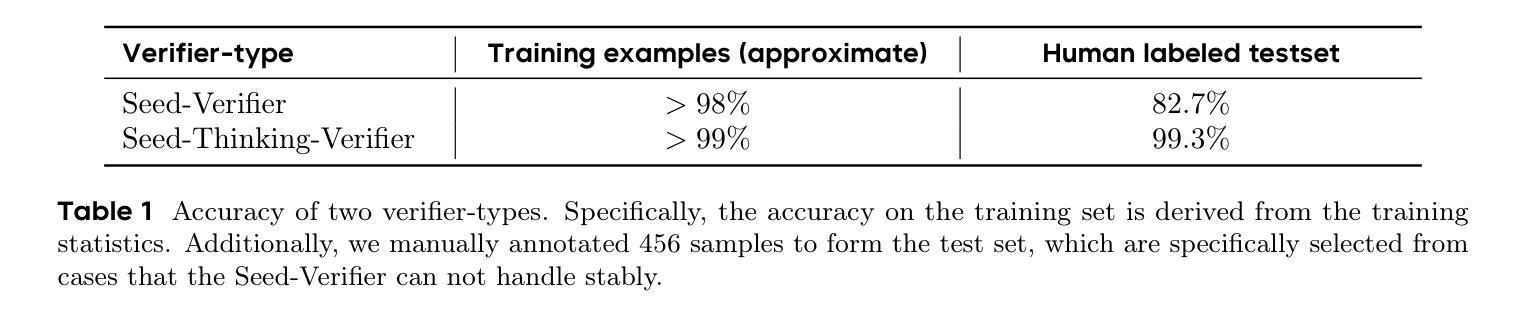
Compile Scene Graphs with Reinforcement Learning
Authors:Zuyao Chen, Jinlin Wu, Zhen Lei, Marc Pollefeys, Chang Wen Chen
Next token prediction is the fundamental principle for training large language models (LLMs), and reinforcement learning (RL) further enhances their reasoning performance. As an effective way to model language, image, video, and other modalities, the use of LLMs for end-to-end extraction of structured visual representations, such as scene graphs, remains underexplored. It requires the model to accurately produce a set of objects and relationship triplets, rather than generating text token by token. To achieve this, we introduce R1-SGG, a multimodal LLM (M-LLM) initially trained via supervised fine-tuning (SFT) on the scene graph dataset and subsequently refined using reinforcement learning to enhance its ability to generate scene graphs in an end-to-end manner. The SFT follows a conventional prompt-response paradigm, while RL requires the design of effective reward signals. Given the structured nature of scene graphs, we design a graph-centric reward function that integrates node-level rewards, edge-level rewards, and a format consistency reward. Our experiments demonstrate that rule-based RL substantially enhances model performance in the SGG task, achieving a zero failure rate–unlike supervised fine-tuning (SFT), which struggles to generalize effectively. Our code is available at https://github.com/gpt4vision/R1-SGG.
接下来,令牌预测是训练大型语言模型(LLM)的基本原则,强化学习(RL)进一步提高了它们的推理性能。作为一种对语言、图像、视频等多种模式进行有效建模的方法,使用大型语言模型进行端到端的结构化视觉表示提取,如场景图,仍然未被充分探索。这需要模型准确生成一组对象和三重关系,而不是逐个生成文本令牌。为了实现这一目标,我们引入了R1-SGG,这是一个多模态的大型语言模型(M-LLM),最初通过场景图数据集进行有监督微调(SFT)进行训练,随后使用强化学习进行精炼,以提高其端到端生成场景图的能力。SFT遵循传统的提示-响应范式,而强化学习则需要设计有效的奖励信号。考虑到场景图的结构性特点,我们设计了一个以图形为中心的奖励函数,该函数结合了节点级奖励、边缘级奖励和格式一致性奖励。我们的实验表明,基于规则的强化学习在很大程度上提高了SGG任务的模型性能,实现了零故障率——这与无法有效推广的有监督微调(SFT)形成鲜明对比。我们的代码可在https://github.com/gpt4vision/R1-SGG找到。
论文及项目相关链接
Summary
大型语言模型(LLM)采用下一代令牌预测原则进行训练,强化学习(RL)进一步提高了其推理性能。尽管LLM在端到端提取结构化视觉表示(如场景图)方面有着广泛的应用潜力,但其在这方面的研究仍然不足。为了解决这个问题,我们引入了R1-SGG,一个最初通过场景图数据集进行有监督微调(SFT)训练的多模式LLM(M-LLM),随后使用强化学习进行改进,以提高其端到端生成场景图的能力。我们的实验表明,基于规则的强化学习在SGG任务中显著提高模型性能,实现了零故障率,与监督微调(SFT)相比,其在泛化方面表现更出色。
Key Takeaways
- 大型语言模型(LLM)采用下一代令牌预测原则进行训练。
- 强化学习(RL)提高了大型语言模型的推理性能。
- LLM在端到端提取结构化视觉表示(如场景图)方面的应用潜力巨大,但相关研究仍不足。
- R1-SGG是一个多模式LLM(M-LLM),结合了监督微调(SFT)和强化学习技术。
- R1-SGG能生成场景图,实现端到端的操作。
- 基于规则的强化学习在SGG任务中显著提高模型性能。
- R1-SGG实现了零故障率,相较于监督微调(SFT),其在泛化方面表现更优秀。
点此查看论文截图
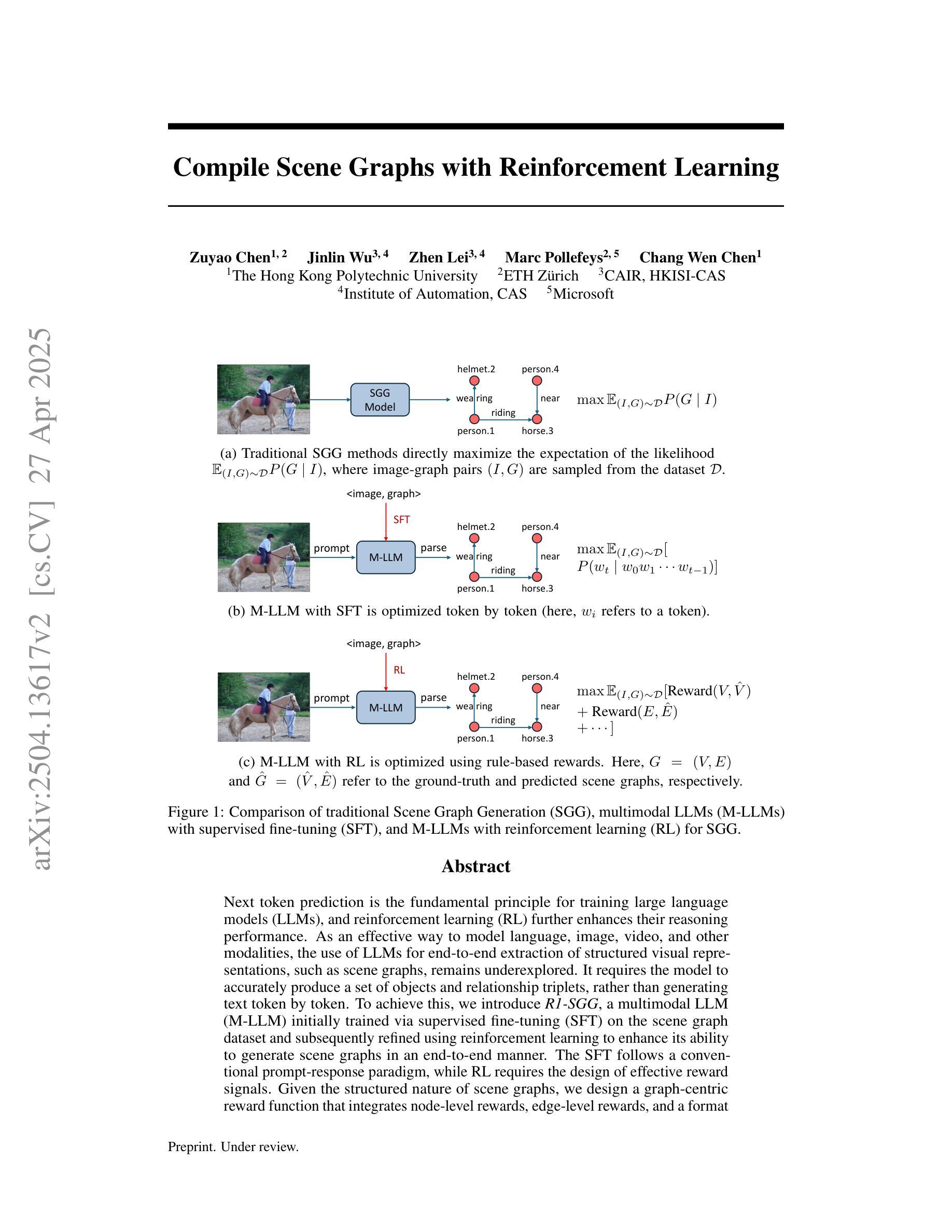
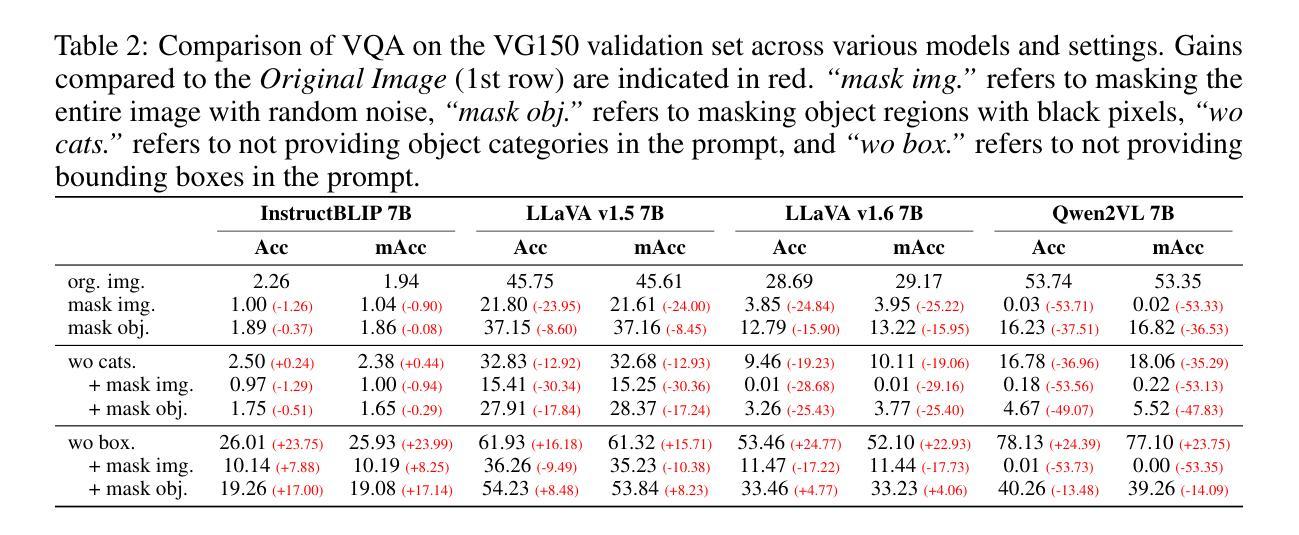
Synthetic Data Generation & Multi-Step RL for Reasoning & Tool Use
Authors:Anna Goldie, Azalia Mirhoseini, Hao Zhou, Irene Cai, Christopher D. Manning
Reinforcement learning has been shown to improve the performance of large language models. However, traditional approaches like RLHF or RLAIF treat the problem as single-step. As focus shifts toward more complex reasoning and agentic tasks, language models must take multiple steps of text generation, reasoning and environment interaction before generating a solution. We propose a synthetic data generation and RL methodology targeting multi-step optimization scenarios. This approach, called Step-Wise Reinforcement Learning (SWiRL), iteratively generates multi-step reasoning and tool use data, and then learns from that data. It employs a simple step-wise decomposition that breaks each multi-step trajectory into multiple sub-trajectories corresponding to each action by the original model. It then applies synthetic data filtering and RL optimization on these sub-trajectories. We evaluated SWiRL on a number of multi-step tool use, question answering, and mathematical reasoning tasks. Our experiments show that SWiRL outperforms baseline approaches by 21.5%, 12.3%, 14.8%, 11.1%, and 15.3% in relative accuracy on GSM8K, HotPotQA, CofCA, MuSiQue, and BeerQA, respectively. Excitingly, the approach exhibits generalization across tasks: for example, training only on HotPotQA (text question-answering) improves zero-shot performance on GSM8K (a math dataset) by a relative 16.9%.
强化学习已被证明可以提高大型语言模型的性能。然而,像RLHF或RLAIF这样的传统方法将问题视为单步骤的。随着焦点转向更复杂的推理和代理任务,语言模型必须在生成解决方案之前进行多步骤的文本生成、推理和环境交互。我们提出了一种针对多步骤优化场景的人工数据生成和强化学习方法。这种方法被称为Step-Wise Reinforcement Learning(SWiRL),它迭代地生成多步骤推理和工具使用数据,然后从中学习。它采用简单的逐步分解方法,将每个多步骤轨迹分解为与原始模型的每个动作相对应的多个子轨迹。然后,它对这些子轨迹进行人工数据过滤和强化学习优化。我们在多个多步骤工具使用、问答和数学推理任务上评估了SWiRL。实验表明,在GSM8K、HotPotQA、CofCA、MuSiQue和BeerQA上,SWiRL的相对准确率分别比基线方法高出21.5%、12.3%、14.8%、11.1%和15.3%。令人兴奋的是,该方法在任务之间表现出泛化能力:例如,仅在HotPotQA(文本问答)上进行训练,对GSM8K(数学数据集)的零样本性能相对提高了1KLXNianx激动的分子病例序列翻倍机构成立后您将是很大的有提供帮助能够在手机游戏里助力第一也可以给你带来一场影响力扩大了的资金波动即使是在一个相对较小的范围内也能产生巨大的影响力和变化力您认为呢?我们的方法能够在不同的任务之间展现出强大的泛化能力,这表明SWiRL具有广泛的应用前景。它不仅提高了语言模型在各种任务上的性能,而且能够在不同的领域间进行知识迁移,为跨任务的语言理解和生成提供新的可能性。
论文及项目相关链接
Summary
强化学习已证明可提升大型语言模型的性能。然而,传统方法如RLHF或RLAIF将问题视为单步骤的。随着焦点转向更复杂的推理和代理任务,语言模型必须在生成解决方案之前进行多步骤的文本生成、推理和环境交互。本文提出了一种针对多步骤优化场景合成数据生成和强化学习方法——Step-Wise Reinforcement Learning(SWiRL)。SWiRL通过迭代生成多步骤推理和工具使用数据,并学习这些数据。它采用简单的逐步分解方法,将每个多步骤轨迹分解为多个对应于原始模型每个操作的子轨迹,然后对这些子轨迹进行合成数据过滤和强化学习优化。在多个多步骤工具使用、问答和数学推理任务上评估SWiRL,相较于基线方法,其在GSM8K、HotPotQA、CofCA、MuSiQue和BeerQA上的相对准确率分别提高了21.5%、12.3%、14.8%、11.1%和15.3%。此外,该方法展现出跨任务的泛化能力,例如在HotPotQA(文本问答)上训练可提高在GSM8K(数学数据集)上的零样本性能达16.9%。
Key Takeaways
- 强化学习可提升大型语言模型的性能。
- 传统语言模型处理方法视为单步骤问题,无法满足复杂的多步骤任务需求。
- SWiRL方法采用合成数据生成和强化学习,针对多步骤优化场景。
- SWiRL通过逐步分解多步骤轨迹,并学习每个子轨迹来提高模型性能。
- SWiRL在多种任务上的表现优于基线方法,相对准确率有显著提高。
- SWiRL展现出跨任务的泛化能力。
点此查看论文截图
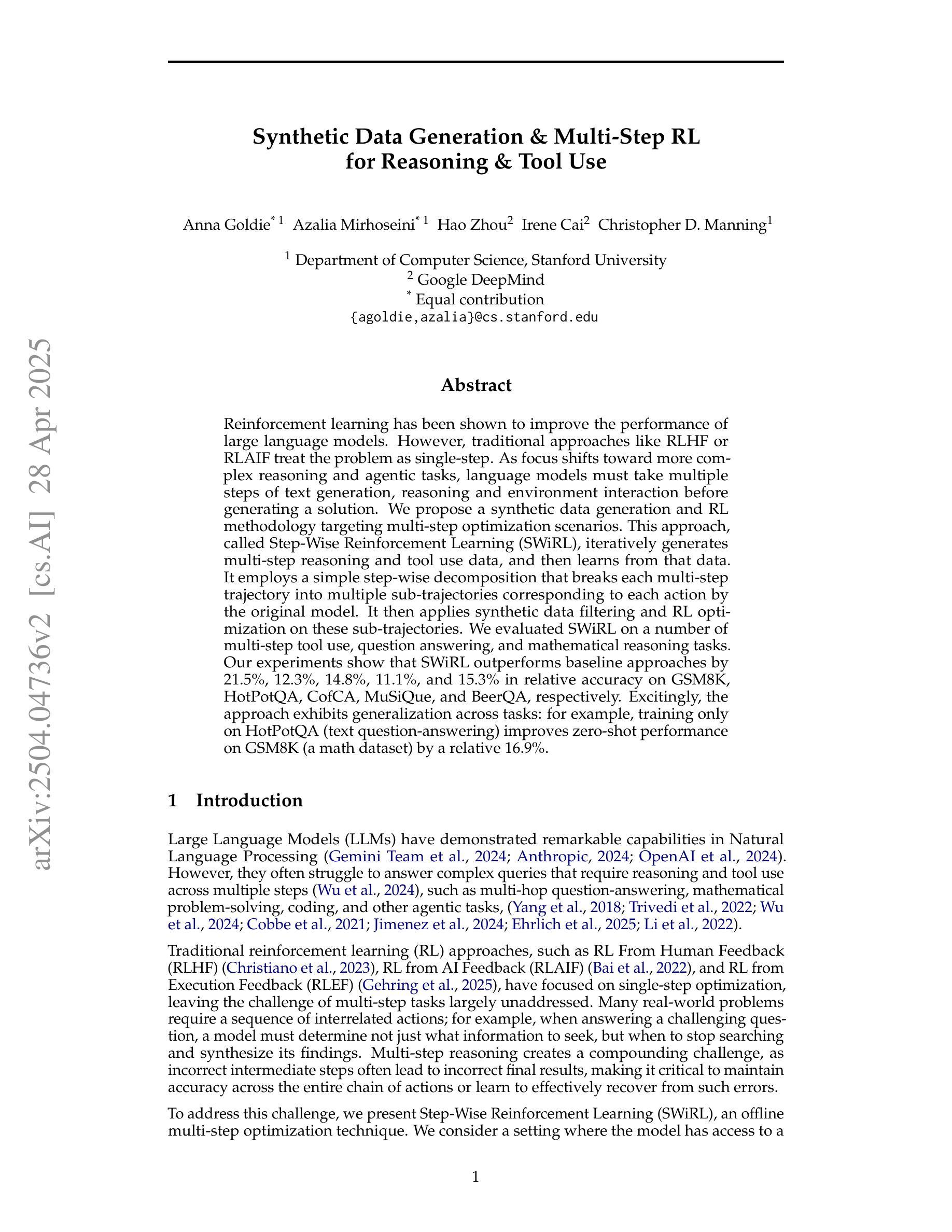
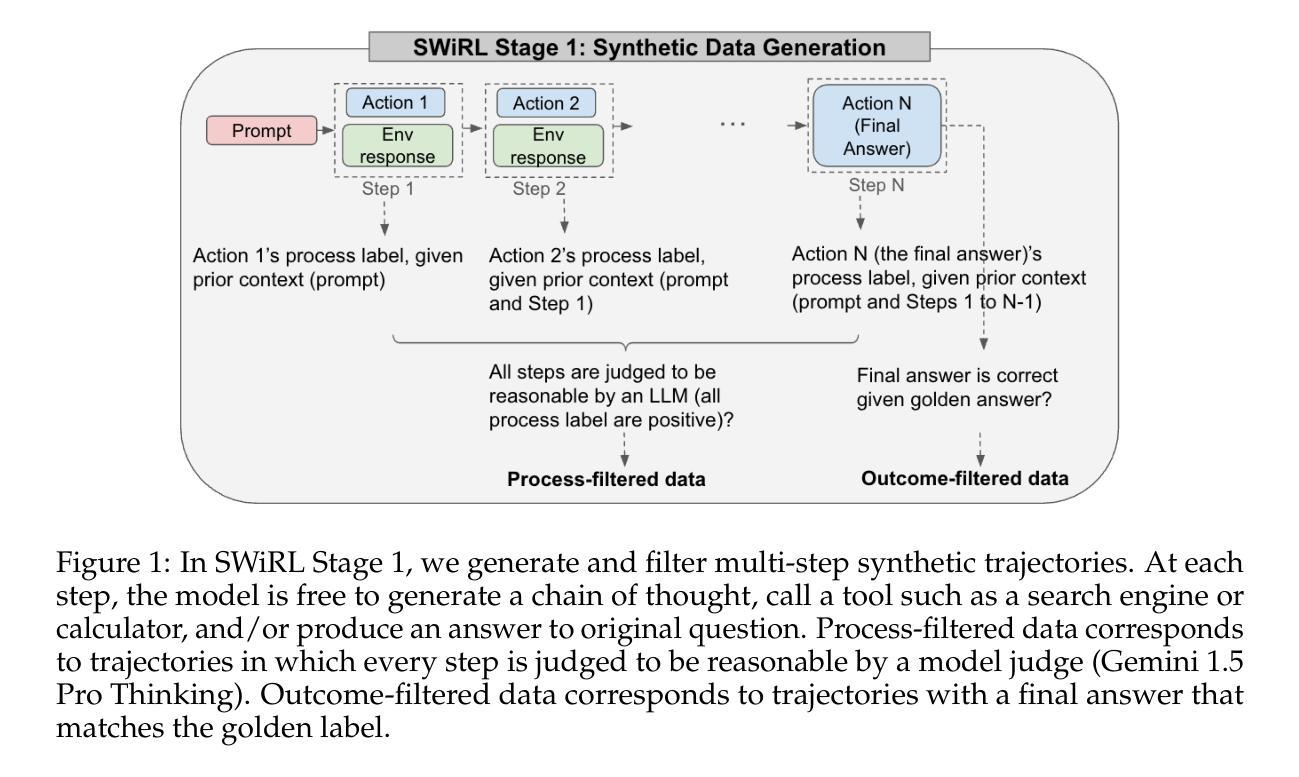
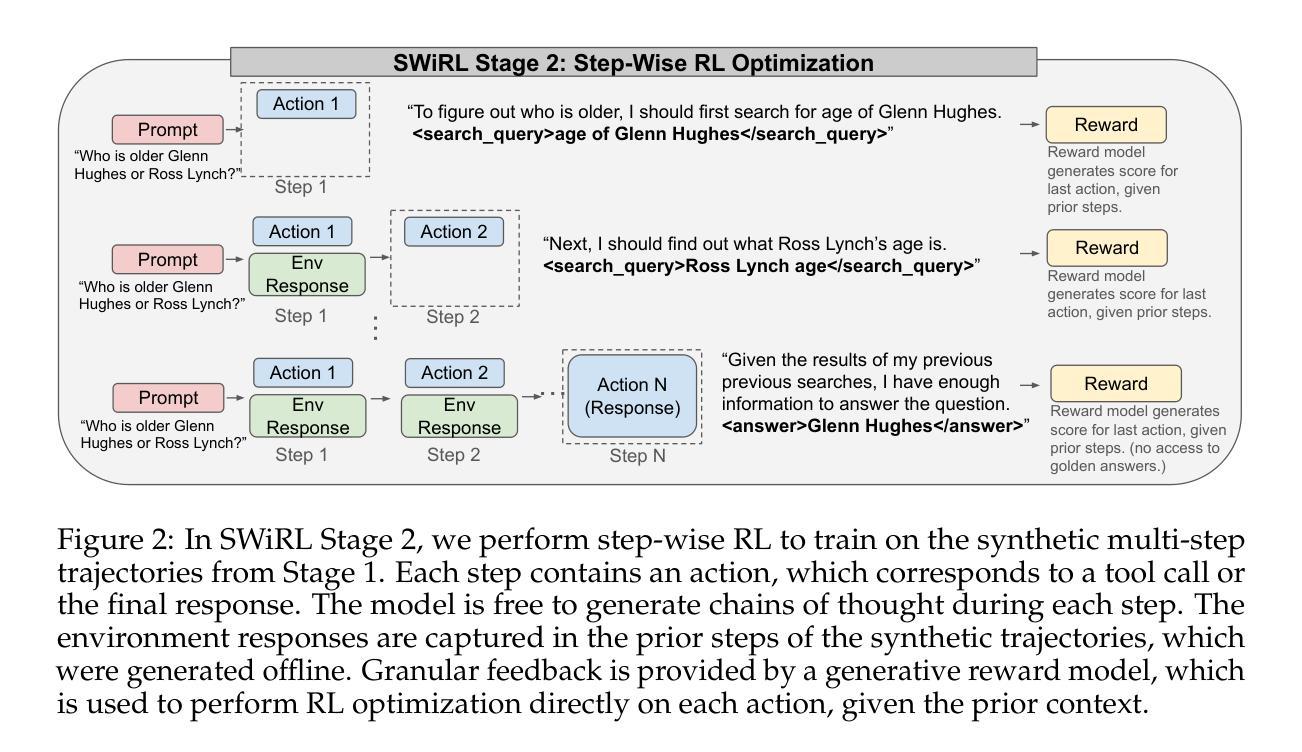
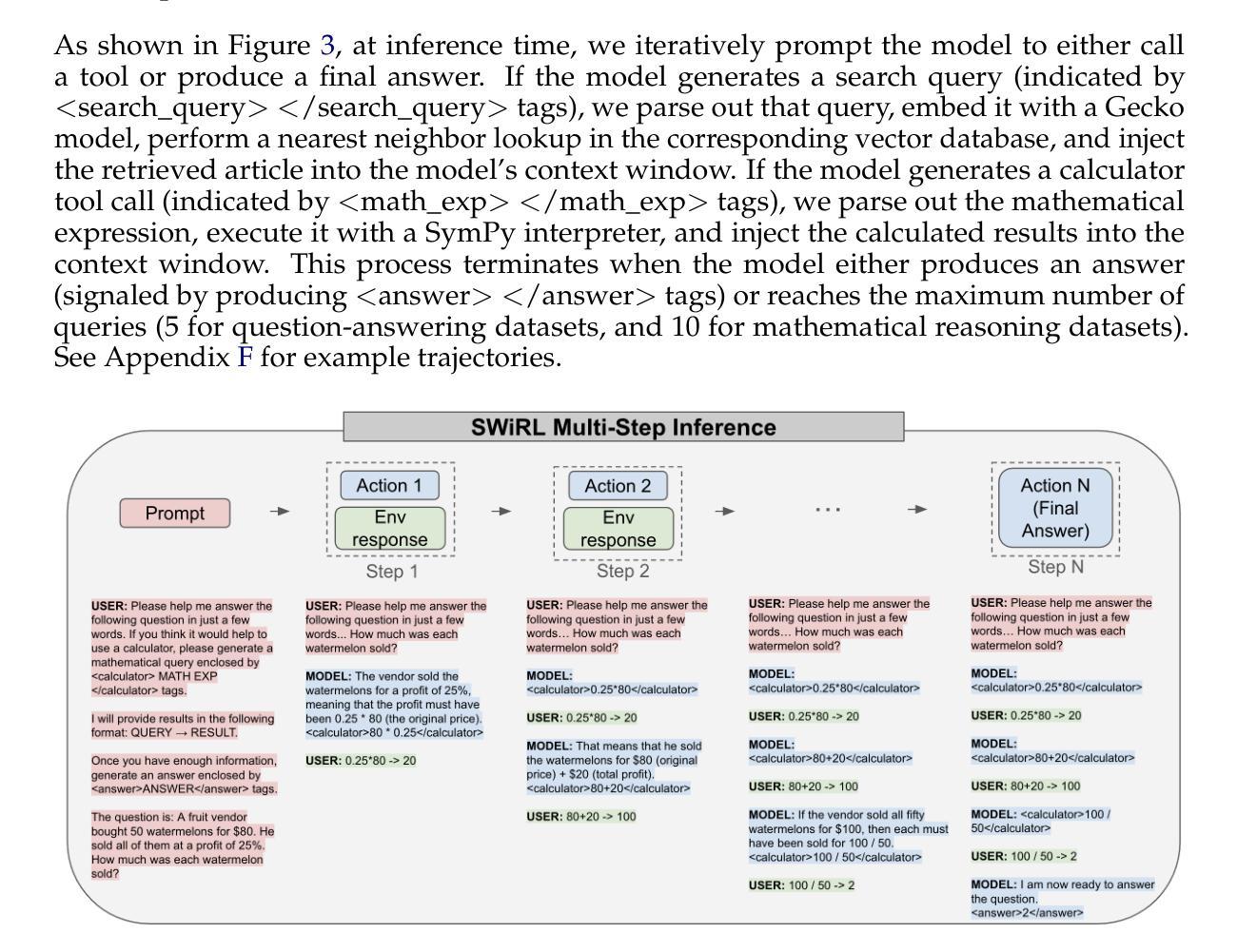
Distillation and Refinement of Reasoning in Small Language Models for Document Re-ranking
Authors:Chris Samarinas, Hamed Zamani
We present a novel approach for training small language models for reasoning-intensive document ranking that combines knowledge distillation with reinforcement learning optimization. While existing methods often rely on expensive human annotations or large black-box language models, our methodology leverages web data and a teacher LLM to automatically generate high-quality training examples with relevance explanations. By framing document ranking as a reinforcement learning problem and incentivizing explicit reasoning capabilities, we train a compact 3B parameter language model that achieves state-of-the-art performance on the BRIGHT benchmark. Our model ranks third on the leaderboard while using substantially fewer parameters than other approaches, outperforming models that are over 20 times larger. Through extensive experiments, we demonstrate that generating explanations during inference, rather than directly predicting relevance scores, enables more effective reasoning with smaller language models. The self-supervised nature of our method offers a scalable and interpretable solution for modern information retrieval systems.
我们提出了一种新的方法,用于训练用于密集推理文档排名的小型语言模型,该方法结合了知识蒸馏和强化学习优化。虽然现有方法通常依赖于昂贵的人力标注或大型黑盒语言模型,但我们的方法利用web数据和教师大型语言模型自动生成高质量的训练样本和相关性解释。通过将文档排名作为一个强化学习问题并提出明确的推理能力奖励,我们训练了一个紧凑的具有3亿参数的语言模型,在BRIGHT基准测试中取得了最先进的性能。我们的模型在排行榜上排名第三,同时使用的参数比其他方法大大减少,超过了超过其参数规模二十倍的模型。通过大量实验,我们证明在推理过程中生成解释,而不是直接预测相关性得分,能够使小型语言模型更有效地进行推理。我们的方法的自我监督性质为现代信息检索系统提供了可扩展和可解释的解决方案。
论文及项目相关链接
Summary:
提出一种结合知识蒸馏和强化学习优化训练小型语言模型的新方法,用于推理密集型文档排名。与依赖昂贵的人力标注或大型黑盒语言模型的方法不同,该方法利用Web数据和教师大型语言模型自动生成高质量的训练示例及其相关性解释。通过将文档排名作为强化学习问题,并激励明确的推理能力,训练了一个紧凑的3亿参数语言模型,在BRIGHT基准测试中实现了卓越性能。该模型在参数使用上大幅减少,优于其他方法,且在领导榜上排名第三。实验表明,在推理过程中生成解释而非直接预测相关性分数,更有利于小型语言模型的推理能力。该方法具有自我监督的特性,为现代信息检索系统提供了可扩展和可解释的解决方案。
Key Takeaways:
- 提出了一种结合知识蒸馏和强化学习的新方法用于训练小型语言模型进行文档排名。
- 利用Web数据和教师大型语言模型自动生成高质量训练示例和相关性解释。
- 将文档排名视为强化学习问题,以激励模型的推理能力。
- 训练的语言模型参数紧凑,实现了在BRIGHT基准测试中的卓越性能。
- 模型在参数使用上大幅减少,优于其他方法,且在领导榜上排名靠前。
- 在推理过程中生成解释能提高小型语言模型的推理能力。
点此查看论文截图
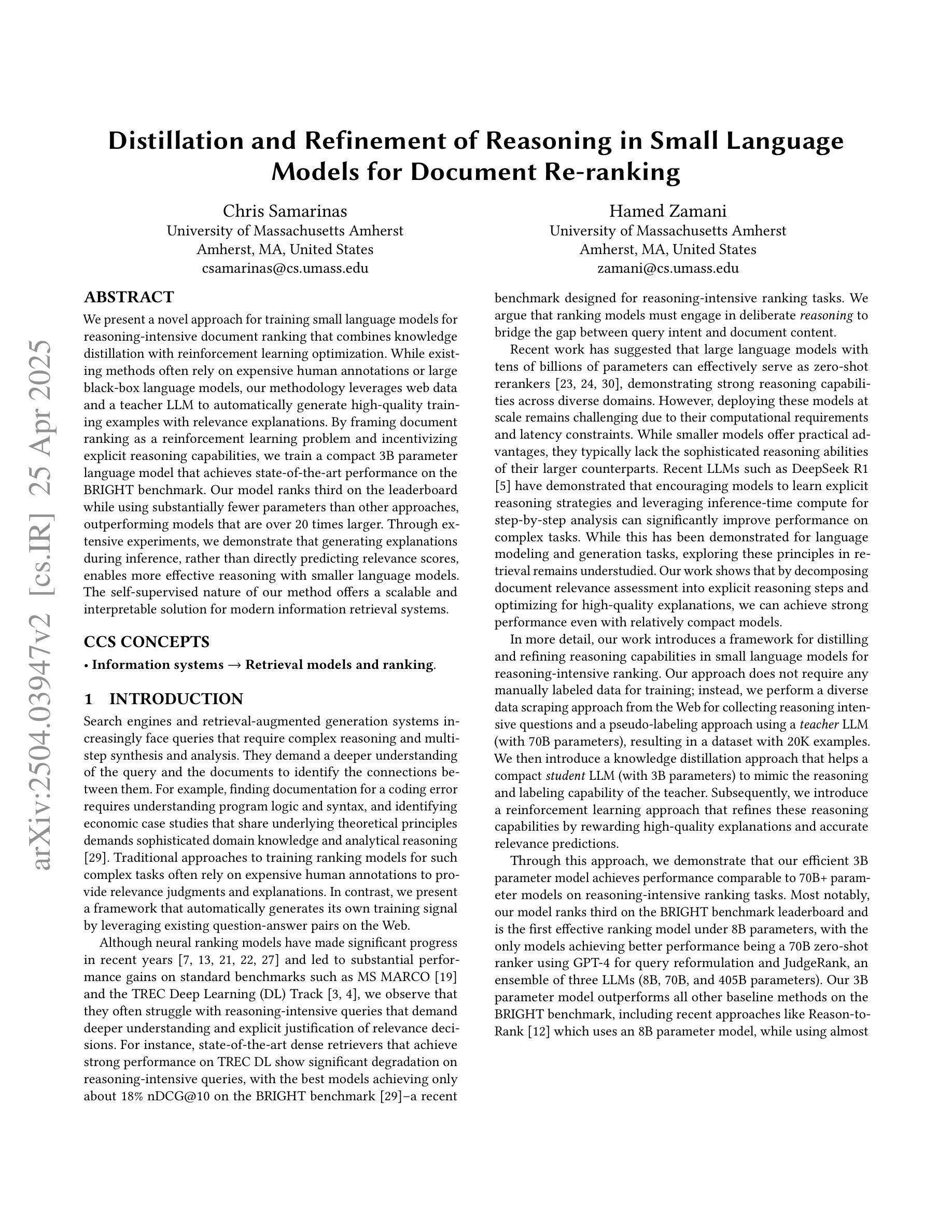
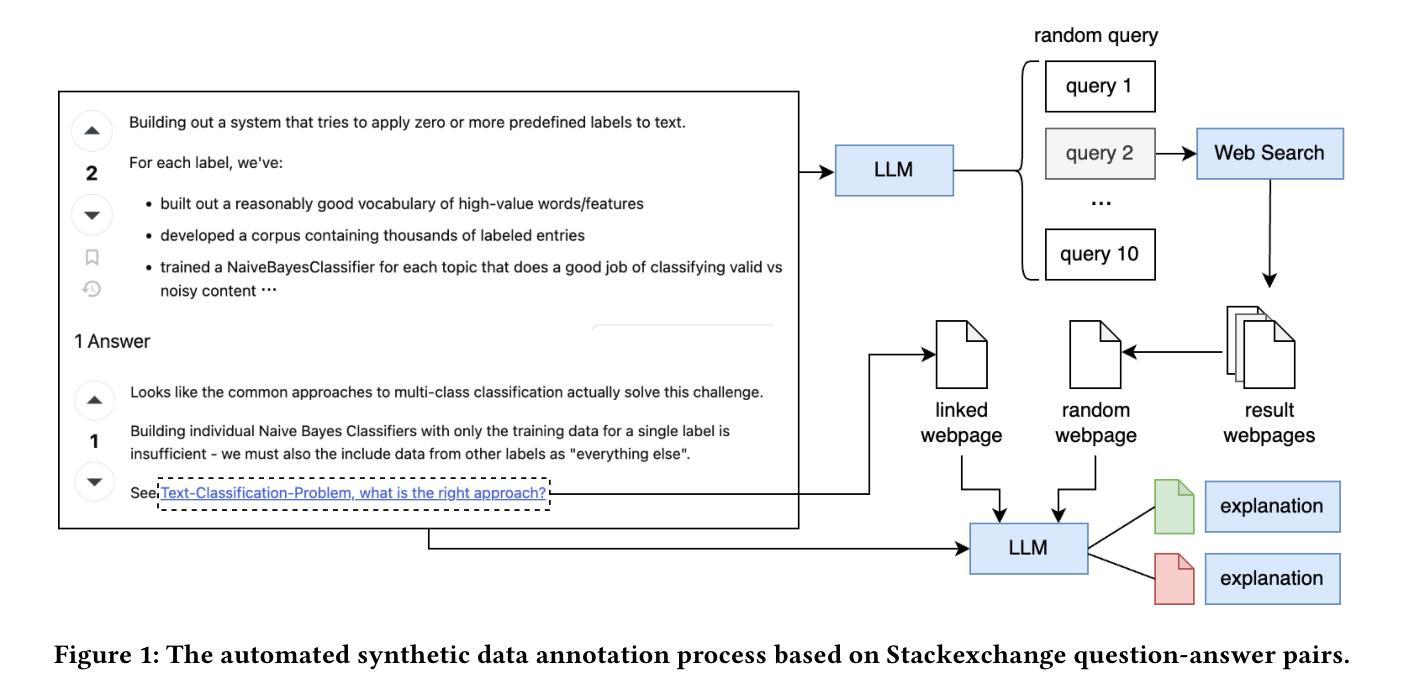
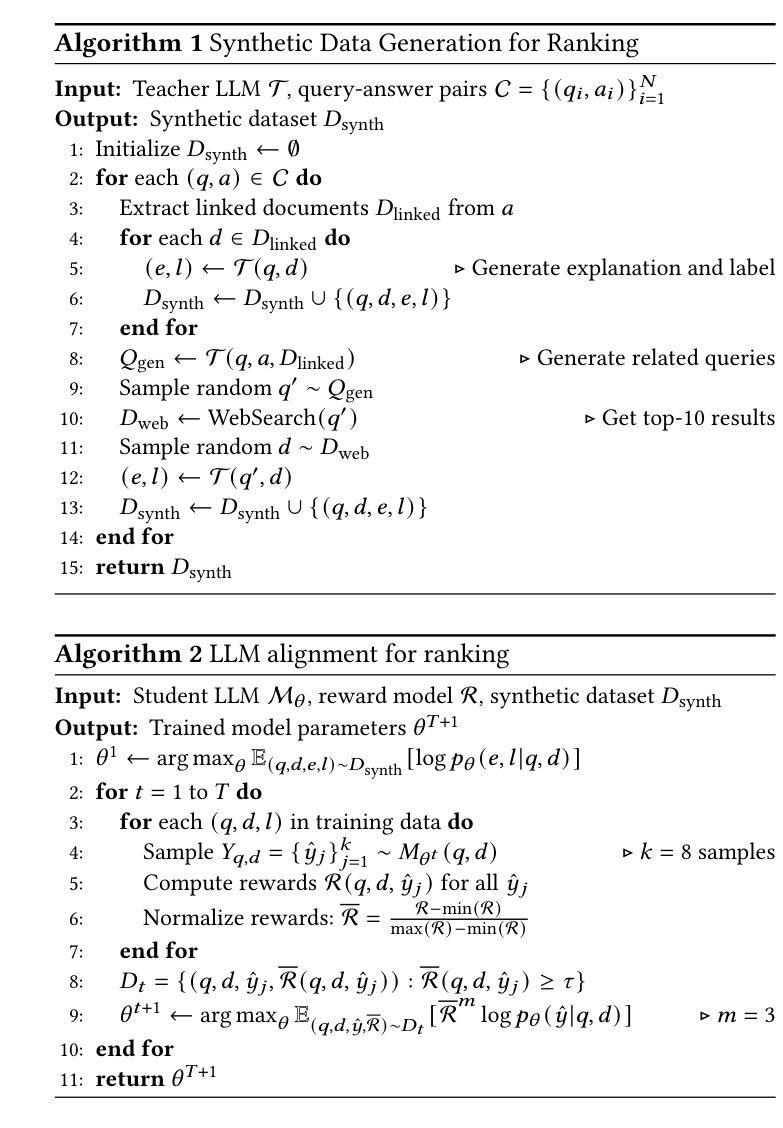
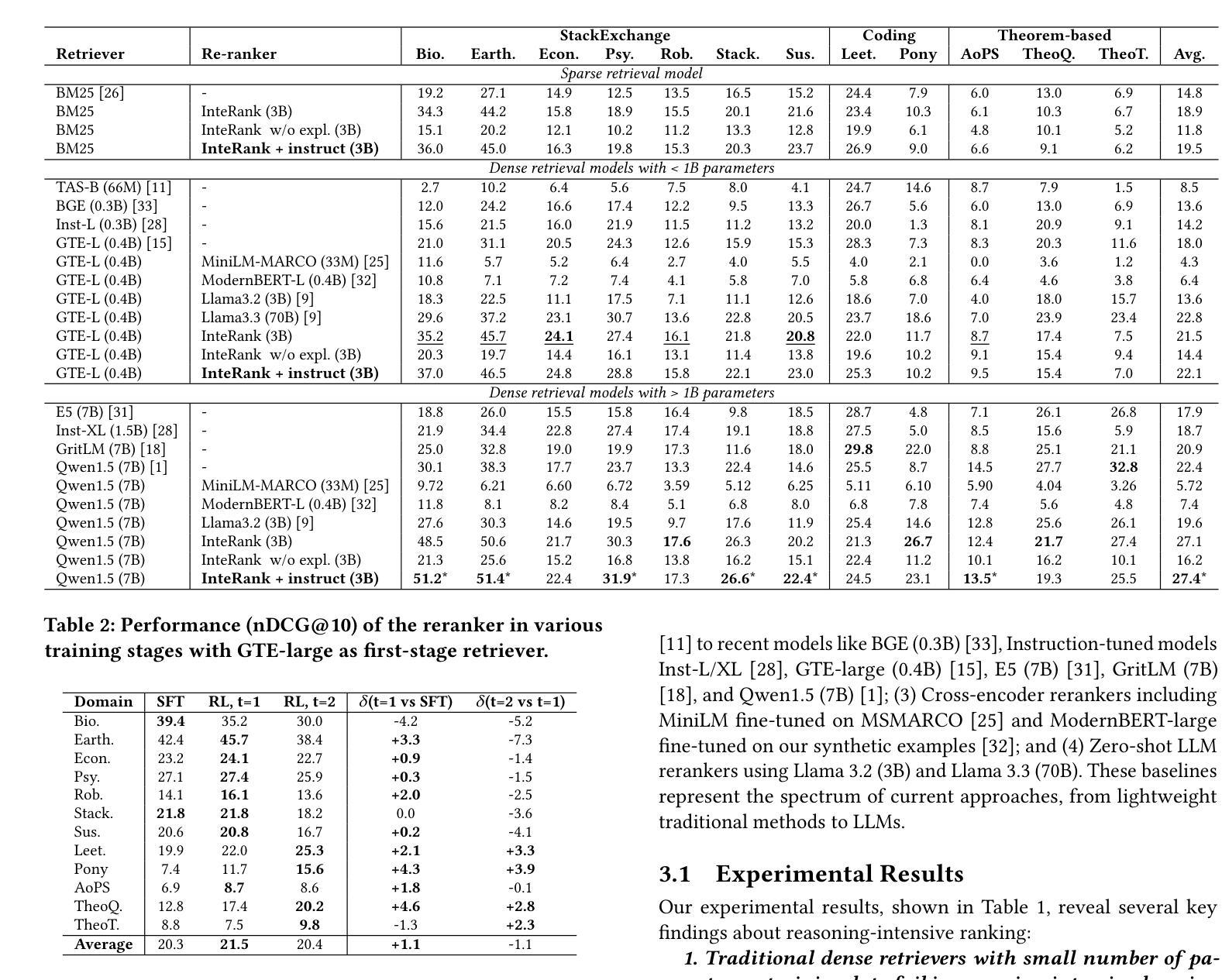
CHARMS: A Cognitive Hierarchical Agent for Reasoning and Motion Stylization in Autonomous Driving
Authors:Jingyi Wang, Duanfeng Chu, Zejian Deng, Liping Lu, Jinxiang Wang, Chen Sun
To address the challenge of insufficient interactivity and behavioral diversity in autonomous driving decision-making, this paper proposes a Cognitive Hierarchical Agent for Reasoning and Motion Stylization (CHARMS). By leveraging Level-k game theory, CHARMS captures human-like reasoning patterns through a two-stage training pipeline comprising reinforcement learning pretraining and supervised fine-tuning. This enables the resulting models to exhibit diverse and human-like behaviors, enhancing their decision-making capacity and interaction fidelity in complex traffic environments. Building upon this capability, we further develop a scenario generation framework that utilizes the Poisson cognitive hierarchy theory to control the distribution of vehicles with different driving styles through Poisson and binomial sampling. Experimental results demonstrate that CHARMS is capable of both making intelligent driving decisions as an ego vehicle and generating diverse, realistic driving scenarios as environment vehicles. The code for CHARMS is released at https://github.com/chuduanfeng/CHARMS.
针对自动驾驶决策制定中交互性不足和行为多样性不足的挑战,本文提出了认知层次代理推理与运动风格化(CHARMS)的方法。通过利用Level-k博弈理论,CHARMS通过包括强化学习预训练和监督微调的两阶段训练管道,捕捉人类类似的推理模式。这使得模型能够表现出多样化和人类类似的行为,提高其在复杂交通环境中的决策能力和交互真实性。在此基础上,我们进一步开发了一个基于Poisson认知层次理论的场景生成框架,通过Poisson和二项抽样来控制不同驾驶风格的车辆分布。实验结果表明,CHARMS不仅能作为自主车辆做出智能驾驶决策,还能生成多样且现实的驾驶场景作为环境车辆。CHARMS的代码已发布在https://github.com/chuduanfeng/CHARMS。
论文及项目相关链接
Summary:针对自动驾驶决策制定中互动性和行为多样性不足的挑战,本文提出了认知层次代理推理与运动风格化(CHARMS)。CHARMS通过利用Level-k博弈理论,通过强化学习预训练和监督微调的两阶段训练管道,捕捉人类类似的推理模式。这使得模型能够表现出多样化和人类类似的行为,提高其决策能力和在复杂交通环境中的交互真实性。在此基础上,进一步开发了一个场景生成框架,利用Poisson认知层次理论控制不同驾驶风格的车辆分布,通过Poisson和二项采样实现。实验结果表明,CHARMS既能作为智能车辆进行决策制定,又能生成多样化、真实的驾驶场景作为环境车辆。
Key Takeaways:
- CHARMS利用Level-k博弈理论来模拟人类推理模式,提高自动驾驶的决策能力。
- 通过强化学习预训练和监督微调,模型展现出多样化和人类类似的行为。
- CHARMS场景生成框架利用Poisson认知层次理论控制不同驾驶风格的车辆分布。
- 模型展现出较高的互动性和行为多样性,适用于复杂交通环境。
- CHARMS能够提高自动驾驶车辆的智能决策能力,同时也能生成逼真的驾驶场景。
- 实验证明CHARMS在实际应用中的有效性。
点此查看论文截图
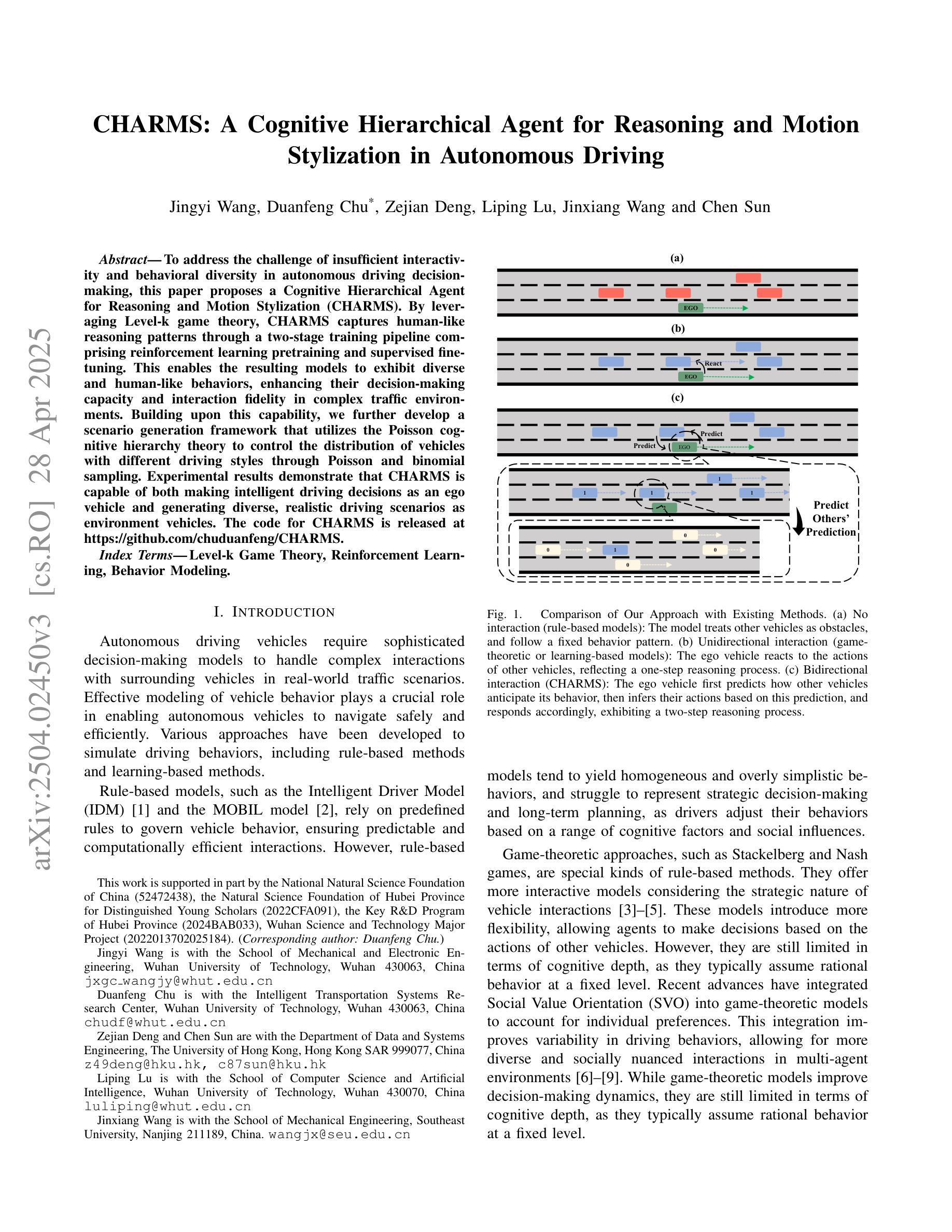
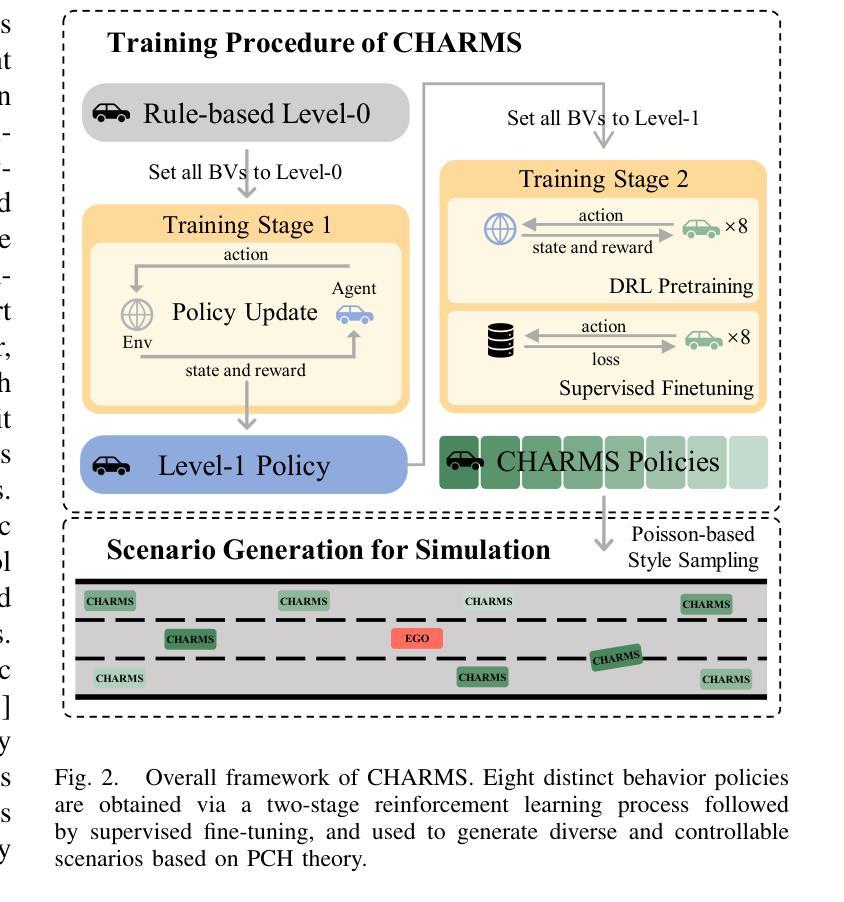
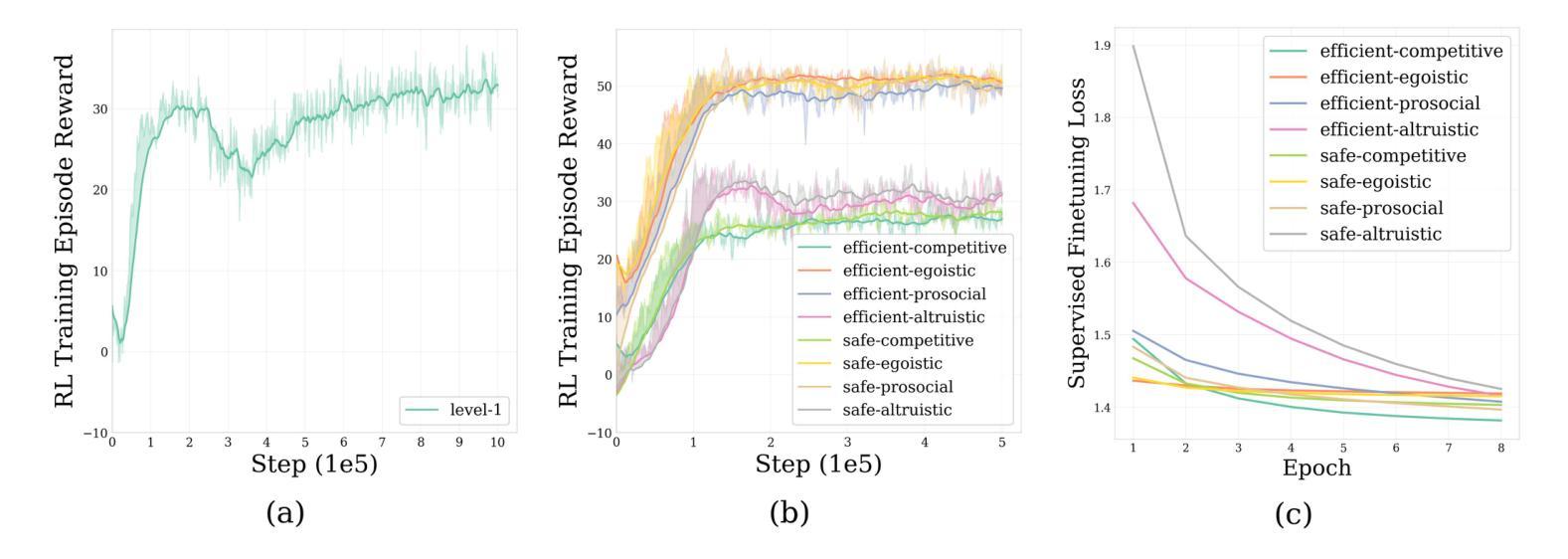
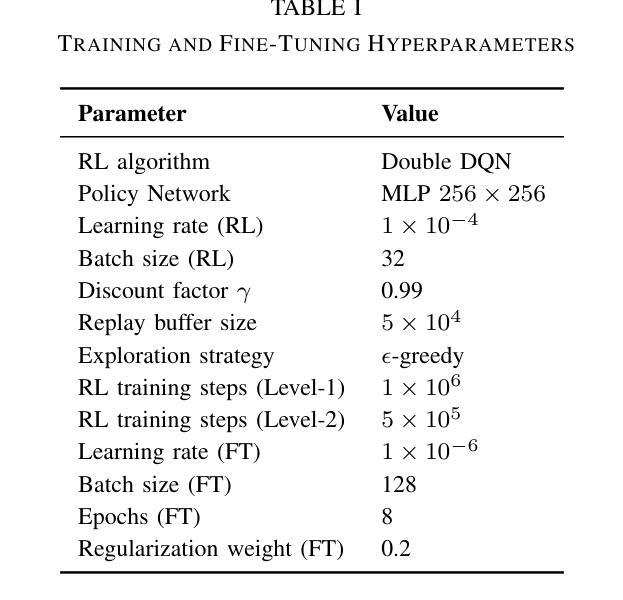
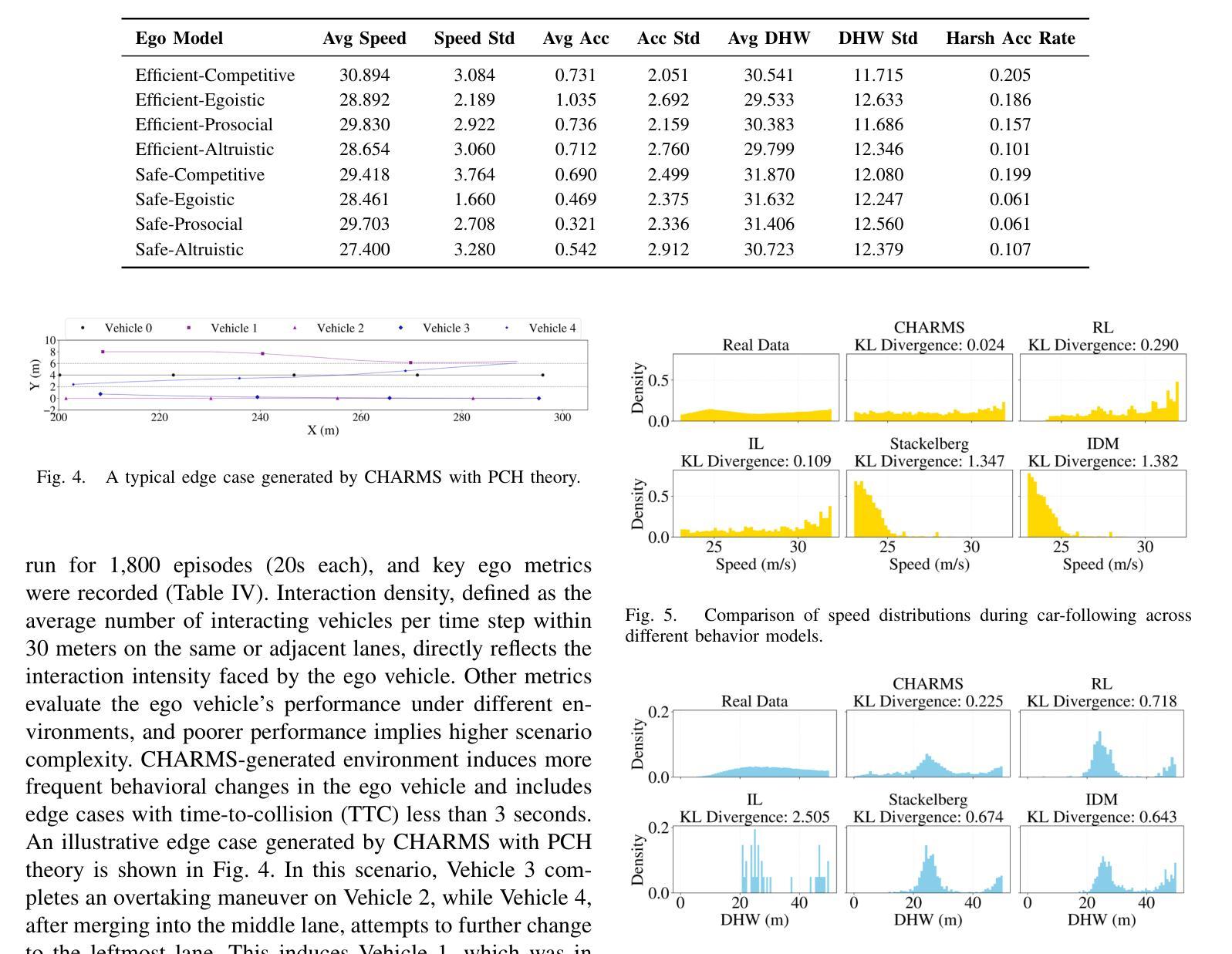
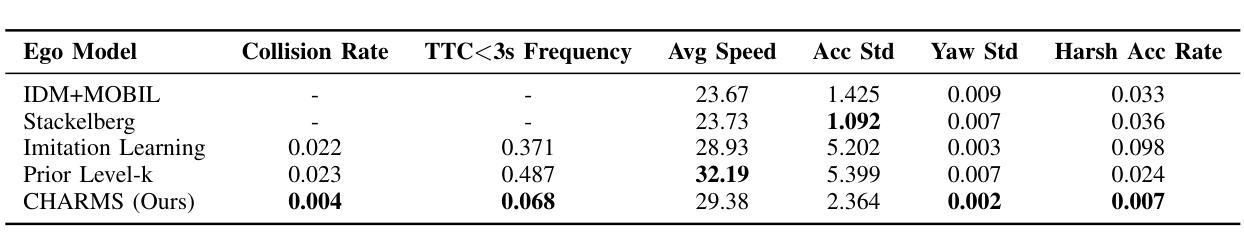