⚠️ 以下所有内容总结都来自于 大语言模型的能力,如有错误,仅供参考,谨慎使用
🔴 请注意:千万不要用于严肃的学术场景,只能用于论文阅读前的初筛!
💗 如果您觉得我们的项目对您有帮助 ChatPaperFree ,还请您给我们一些鼓励!⭐️ HuggingFace免费体验
2025-05-01 更新
AlignDiT: Multimodal Aligned Diffusion Transformer for Synchronized Speech Generation
Authors:Jeongsoo Choi, Ji-Hoon Kim, Kim Sung-Bin, Tae-Hyun Oh, Joon Son Chung
In this paper, we address the task of multimodal-to-speech generation, which aims to synthesize high-quality speech from multiple input modalities: text, video, and reference audio. This task has gained increasing attention due to its wide range of applications, such as film production, dubbing, and virtual avatars. Despite recent progress, existing methods still suffer from limitations in speech intelligibility, audio-video synchronization, speech naturalness, and voice similarity to the reference speaker. To address these challenges, we propose AlignDiT, a multimodal Aligned Diffusion Transformer that generates accurate, synchronized, and natural-sounding speech from aligned multimodal inputs. Built upon the in-context learning capability of the DiT architecture, AlignDiT explores three effective strategies to align multimodal representations. Furthermore, we introduce a novel multimodal classifier-free guidance mechanism that allows the model to adaptively balance information from each modality during speech synthesis. Extensive experiments demonstrate that AlignDiT significantly outperforms existing methods across multiple benchmarks in terms of quality, synchronization, and speaker similarity. Moreover, AlignDiT exhibits strong generalization capability across various multimodal tasks, such as video-to-speech synthesis and visual forced alignment, consistently achieving state-of-the-art performance. The demo page is available at https://mm.kaist.ac.kr/projects/AlignDiT .
本文致力于解决多模态到语音生成的任务,该任务旨在从多种输入模式(文本、视频和参考音频)中合成高质量语音。由于其在电影制作、配音和虚拟角色等领域的广泛应用,该任务已引起广泛关注。尽管最近有所进展,但现有方法仍面临语音清晰度、音视频同步、语音自然度和与参考演讲者的声音相似性等方面的局限性。为了应对这些挑战,我们提出了AlignDiT,这是一种多模态对齐扩散转换器,可以从对齐的多模态输入中生成准确、同步和自然语音。AlignDiT基于DiT架构的上下文学习能力,探索了三种有效的策略来进行多模态表示对齐。此外,我们还引入了一种新型的无分类器引导机制,使模型在语音合成过程中能够自适应地平衡各种模态的信息。大量实验表明,AlignDiT在多个基准测试上显著优于现有方法,在质量、同步和演讲者相似性方面表现出色。此外,AlignDiT在各种多模态任务中表现出强大的泛化能力,如视频到语音合成和视觉强制对齐,始终达到最先进的性能。演示页面可访问:https://mm.kaist.ac.kr/projects/AlignDiT。
论文及项目相关链接
Summary
本文关注多模态到语音的生成任务,旨在从文本、视频和参考音频等多种输入模态中合成高质量语音。针对现有方法的局限性,如语音清晰度、音视频同步、语音自然度和与参考演讲者的声音相似性等问题,提出了AlignDiT方法。该方法基于扩散Transformer架构,通过三种有效策略实现多模态表示对齐。此外,还引入了一种新型的多模态无分类器引导机制,使模型在语音合成过程中能够自适应地平衡各模态的信息。实验表明,AlignDiT在多个基准测试中显著优于现有方法,具有强大的泛化能力。
Key Takeaways
- 本文关注多模态到语音的生成任务,涉及文本、视频和参考音频等多种输入模态。
- 现有方法在语音清晰度、音视频同步、语音自然度和与参考演讲者的声音相似性等方面存在局限性。
- 提出的AlignDiT方法基于扩散Transformer架构,实现多模态表示的有效对齐。
- AlignDiT采用三种策略进行多模态对齐,包括利用上下文学习能力的DiT架构。
- 引入了一种新型的多模态无分类器引导机制,使模型在语音合成过程中能够自适应平衡各模态信息。
- 实验结果表明,AlignDiT在多个基准测试中显著优于现有方法。
点此查看论文截图
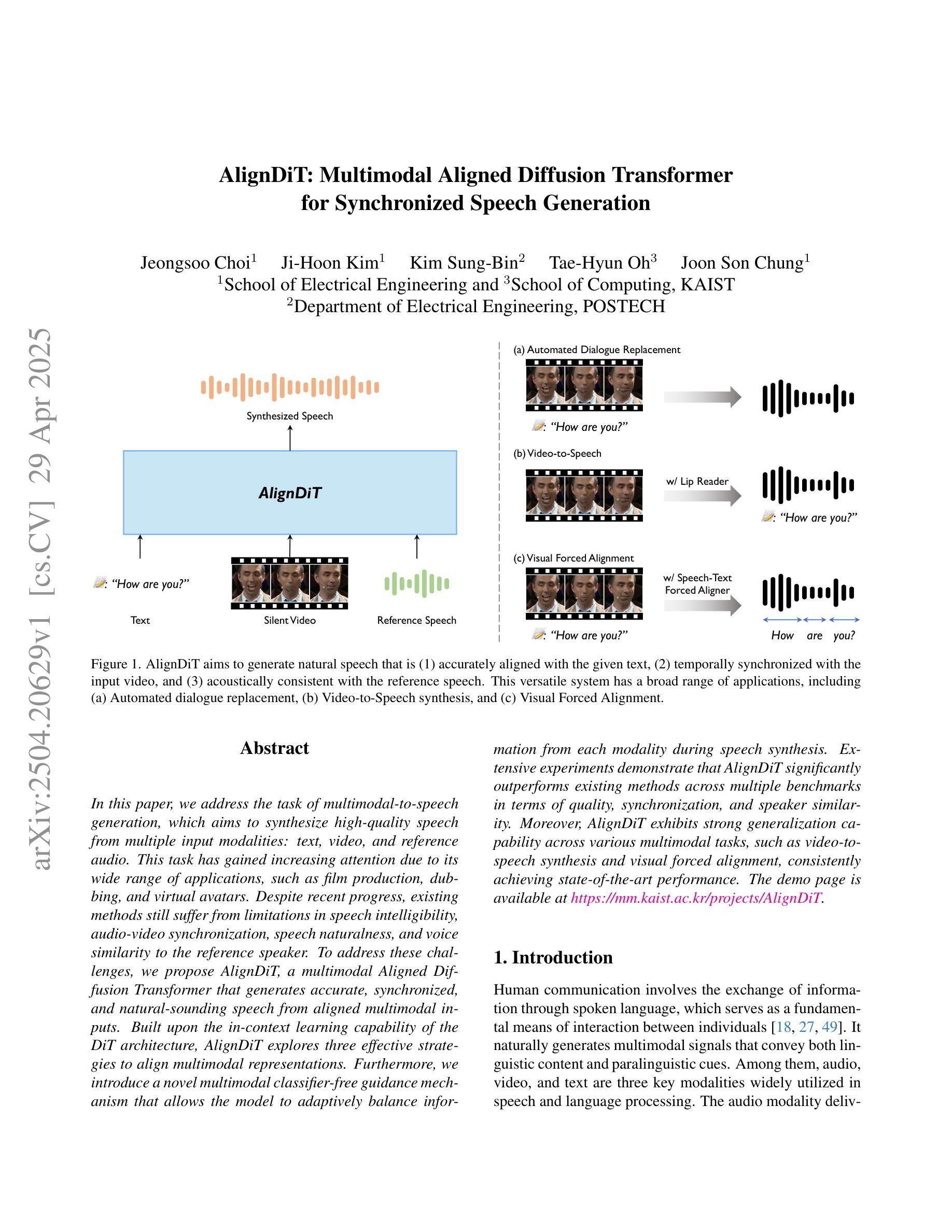
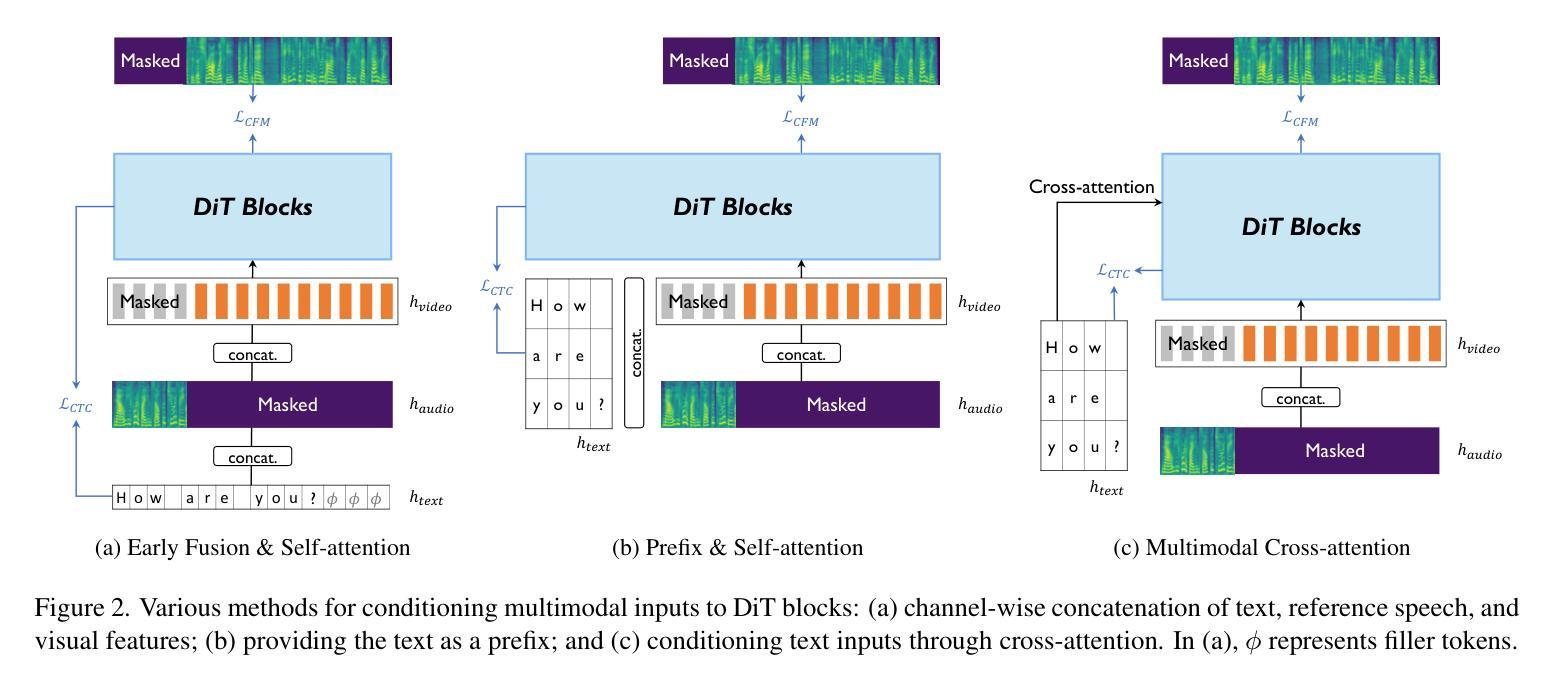