⚠️ 以下所有内容总结都来自于 大语言模型的能力,如有错误,仅供参考,谨慎使用
🔴 请注意:千万不要用于严肃的学术场景,只能用于论文阅读前的初筛!
💗 如果您觉得我们的项目对您有帮助 ChatPaperFree ,还请您给我们一些鼓励!⭐️ HuggingFace免费体验
2025-05-02 更新
Anomaly-Driven Approach for Enhanced Prostate Cancer Segmentation
Authors:Alessia Hu, Regina Beets-Tan, Lishan Cai, Eduardo Pooch
Magnetic Resonance Imaging (MRI) plays an important role in identifying clinically significant prostate cancer (csPCa), yet automated methods face challenges such as data imbalance, variable tumor sizes, and a lack of annotated data. This study introduces Anomaly-Driven U-Net (adU-Net), which incorporates anomaly maps derived from biparametric MRI sequences into a deep learning-based segmentation framework to improve csPCa identification. We conduct a comparative analysis of anomaly detection methods and evaluate the integration of anomaly maps into the segmentation pipeline. Anomaly maps, generated using Fixed-Point GAN reconstruction, highlight deviations from normal prostate tissue, guiding the segmentation model to potential cancerous regions. We compare the performance by using the average score, computed as the mean of the AUROC and Average Precision (AP). On the external test set, adU-Net achieves the best average score of 0.618, outperforming the baseline nnU-Net model (0.605). The results demonstrate that incorporating anomaly detection into segmentation improves generalization and performance, particularly with ADC-based anomaly maps, offering a promising direction for automated csPCa identification.
磁共振成像(MRI)在识别临床重要前列腺癌(csPCa)方面发挥着重要作用,但自动化方法面临数据不平衡、肿瘤大小可变和缺乏注释数据等挑战。本研究介绍了Anomaly-Driven U-Net(adU-Net),它将来自双参数MRI序列的异常映射引入到基于深度学习的分割框架中,以提高csPCa的识别。我们对异常检测方法进行了比较分析,并评估了异常映射在分割管道中的集成。使用Fixed-Point GAN重建技术生成的异常映射突出了前列腺正常组织中的偏差,引导分割模型定位潜在癌变区域。我们通过使用平均得分进行比较,该得分是AUROC和平均精度(AP)的平均值。在外部测试集上,adU-Net获得了最佳平均得分0.618,超过了基线nnU-Net模型(0.605)。结果表明,将异常检测纳入分割可以提高泛化和性能,特别是基于ADC的异常映射,为自动csPCa识别提供了有前景的方向。
论文及项目相关链接
PDF Paper accepted for publication at 2025 47th Annual International Conference of the IEEE Engineering in Medicine and Biology Society (EMBC) Copyright 2025 IEEE. Personal use of this material is permitted. Permission from IEEE must be obtained for all other uses, in any current or future media
Summary
本文介绍了一种基于深度学习的前列腺癌症识别新方法——Anomaly-Driven U-Net(adU-Net)。该方法结合双参数磁共振成像(MRI)序列生成的异常图,以提高对临床显著前列腺癌(csPCa)的识别能力。通过对比不同异常检测方法,并评估异常图在分割管道中的集成效果,实验结果显示,采用Fixed-Point GAN重建生成的异常图能突出正常前列腺组织的偏差,引导分割模型识别潜在癌变区域。在外部测试集上,adU-Net的平均得分最高,达到0.618,优于基线nnU-Net模型(0.605),表明将异常检测融入分割过程能提高模型的泛化能力和性能,特别是基于ADC的异常图,为自动csPCa识别提供了有前景的方向。
Key Takeaways
- Anomaly-Driven U-Net(adU-Net)结合了双参数MRI序列生成的异常图,提高了对临床显著前列腺癌(csPCa)的识别能力。
- 通过Fixed-Point GAN重建生成异常图,能突出正常前列腺组织的偏差,引导分割模型识别潜在癌变区域。
- 深度学习方法在前列腺癌症识别中面临数据不平衡、肿瘤大小可变和缺乏标注数据等挑战。
- adU-Net在外部测试集上的平均得分达到0.618,优于基线nnU-Net模型。
- 结合异常检测能提高模型的泛化能力和性能。
- 基于ADC的异常图在自动csPCa识别方面表现出有前景的方向。
点此查看论文截图
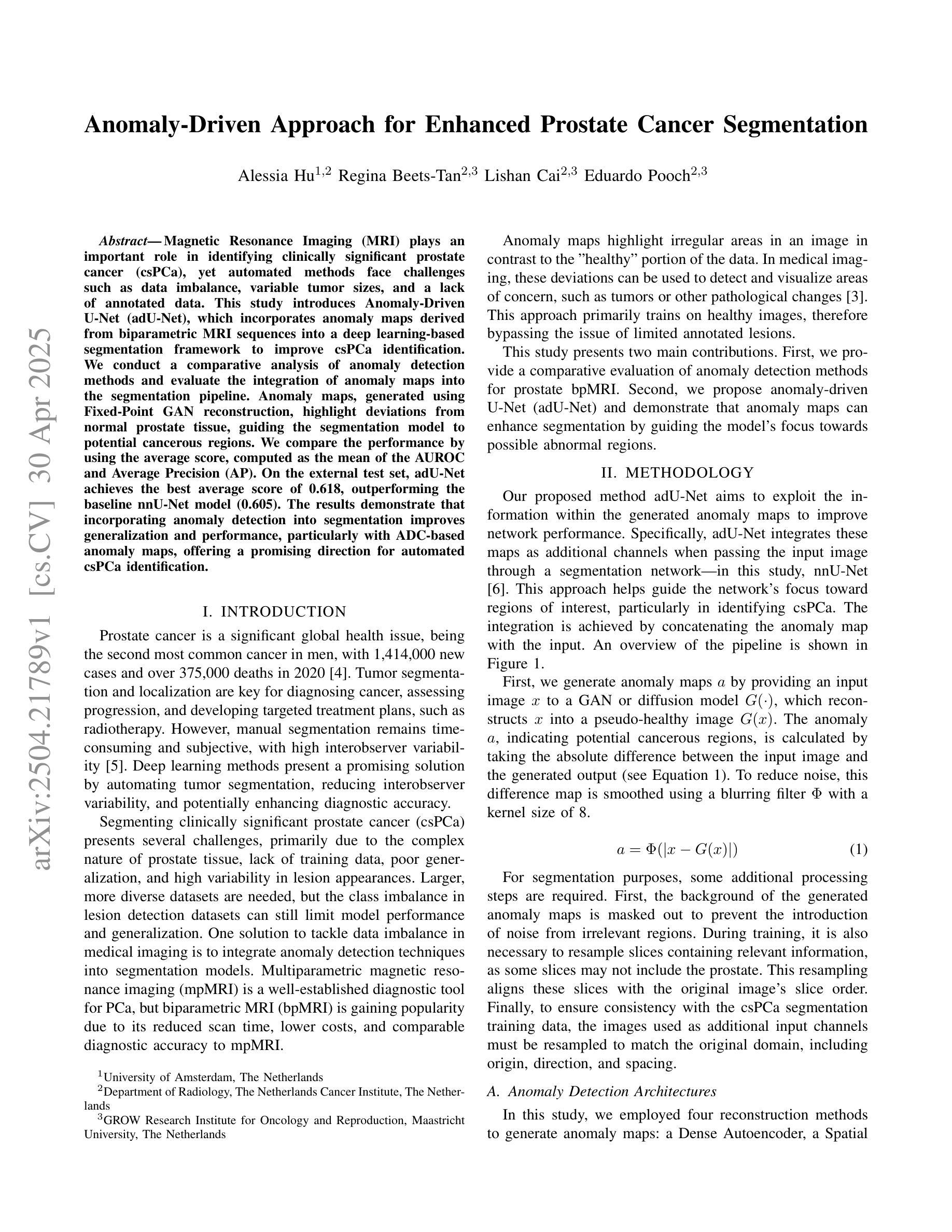
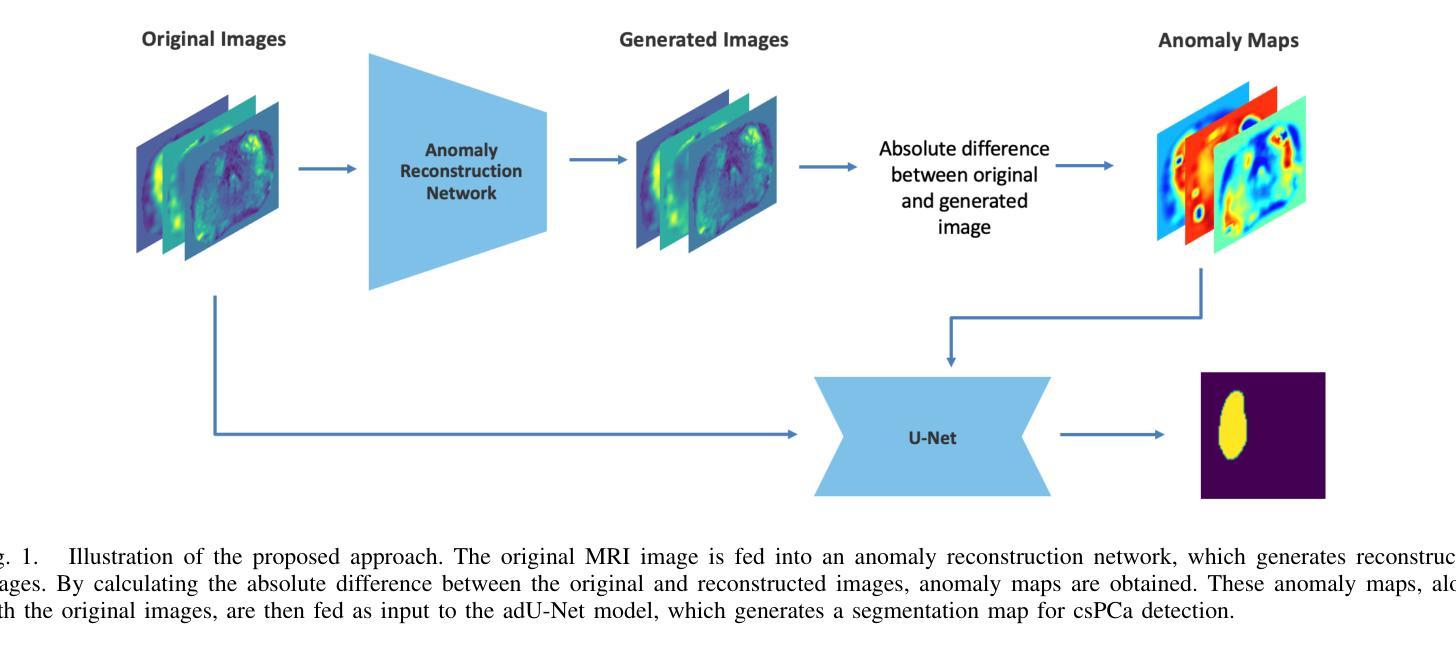
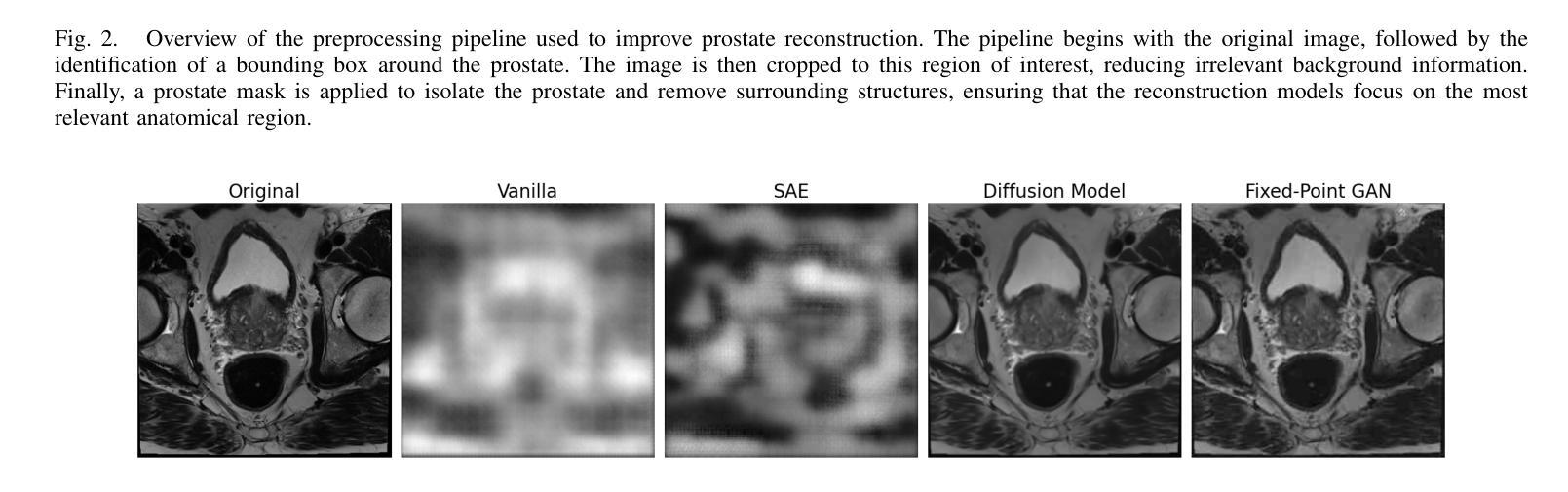
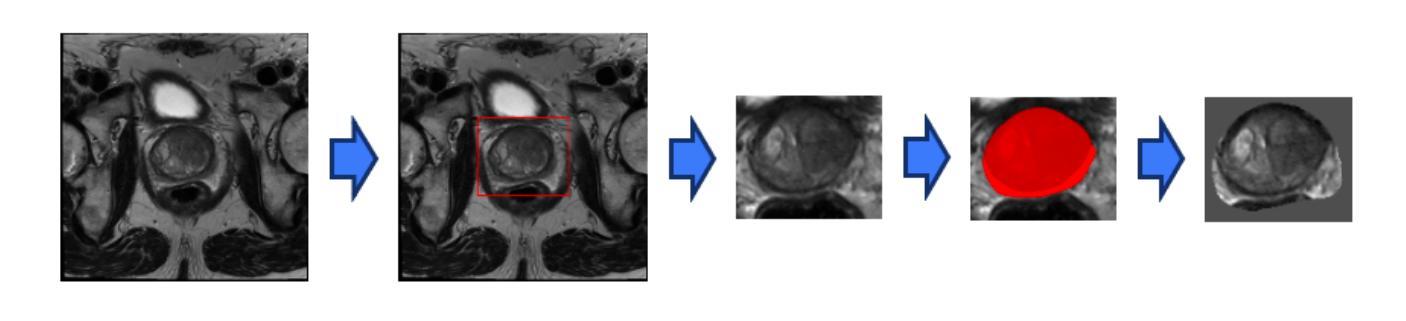
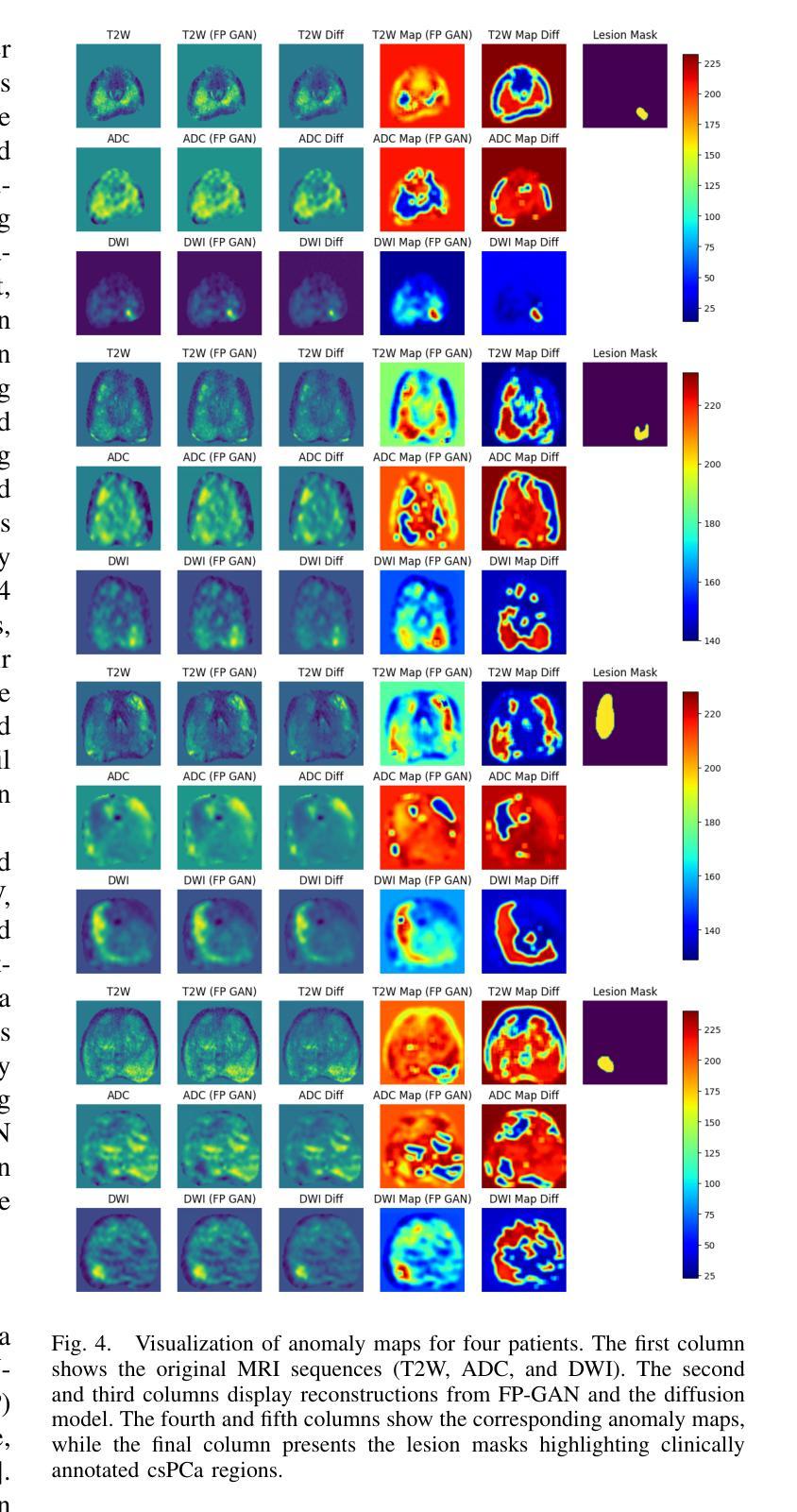
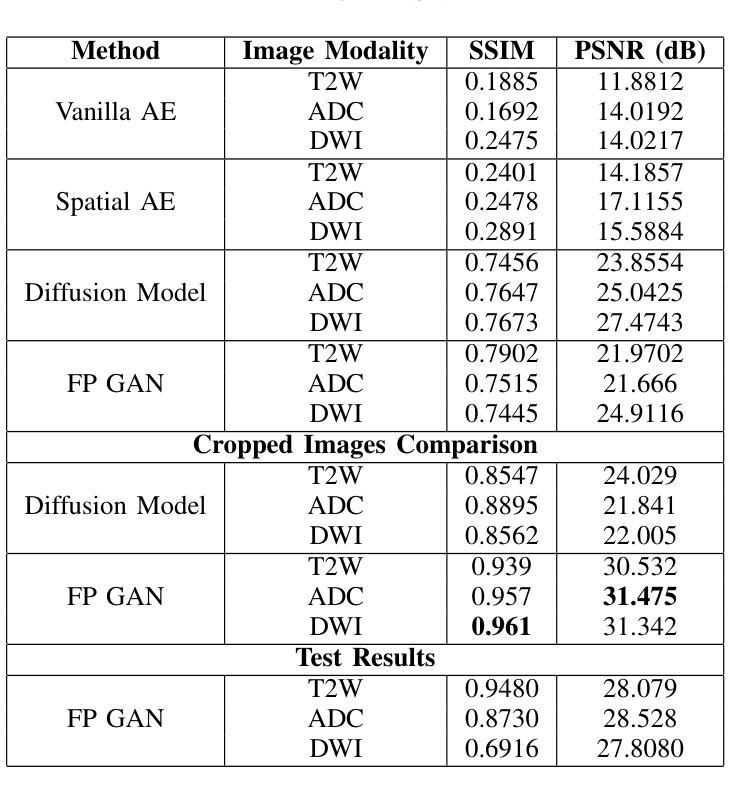
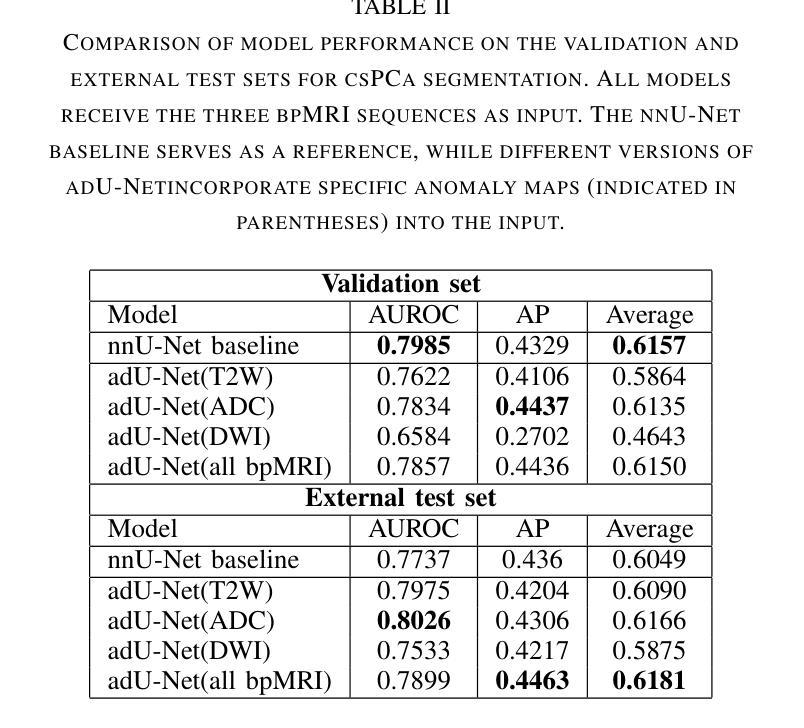
Text-Conditioned Diffusion Model for High-Fidelity Korean Font Generation
Authors:Abdul Sami, Avinash Kumar, Irfanullah Memon, Youngwon Jo, Muhammad Rizwan, Jaeyoung Choi
Automatic font generation (AFG) is the process of creating a new font using only a few examples of the style images. Generating fonts for complex languages like Korean and Chinese, particularly in handwritten styles, presents significant challenges. Traditional AFGs, like Generative adversarial networks (GANs) and Variational Auto-Encoders (VAEs), are usually unstable during training and often face mode collapse problems. They also struggle to capture fine details within font images. To address these problems, we present a diffusion-based AFG method which generates high-quality, diverse Korean font images using only a single reference image, focusing on handwritten and printed styles. Our approach refines noisy images incrementally, ensuring stable training and visually appealing results. A key innovation is our text encoder, which processes phonetic representations to generate accurate and contextually correct characters, even for unseen characters. We used a pre-trained style encoder from DG FONT to effectively and accurately encode the style images. To further enhance the generation quality, we used perceptual loss that guides the model to focus on the global style of generated images. Experimental results on over 2000 Korean characters demonstrate that our model consistently generates accurate and detailed font images and outperforms benchmark methods, making it a reliable tool for generating authentic Korean fonts across different styles.
自动字体生成(AFG)是利用少量风格图像创建新字体的过程。对于韩语和中文等复杂语言的字体生成,尤其是手写风格,存在重大挑战。传统的AFG技术,如生成对抗网络(GANs)和变分自动编码器(VAEs),在训练过程中通常不稳定,经常面临模式崩溃问题。它们也很难捕捉字体图像中的细节。为了解决这些问题,我们提出了一种基于扩散的AFG方法,该方法仅使用单个参考图像生成高质量、多样化的韩文字体图像,重点关注手写和打印风格。我们的方法逐步细化噪声图像,确保训练稳定且结果视觉上令人愉悦。一个关键的创新之处是我们的文本编码器,它处理语音表示以生成准确且上下文正确的字符,即使对于未见过的字符也是如此。我们使用DG FONT的预训练风格编码器来有效且准确地编码风格图像。为了进一步提高生成质量,我们使用了感知损失,引导模型关注生成图像的整体风格。对超过2000个韩国字符的实验结果表明,我们的模型持续生成准确且详细的字体图像,并优于基准方法,成为生成不同风格的真实韩国字体的可靠工具。
论文及项目相关链接
PDF 6 pages, 4 figures, Accepted at ICOIN 2025
Summary
基于扩散模型的自动字体生成方法,利用单一参考图像生成高质量、多样化的韩文手写和打印风格字体。通过逐步去噪图像,实现稳定训练和视觉效果好。采用文本编码器处理语音表示,生成准确且上下文正确的字符,甚至适用于未见字符。利用预训练的样式编码器和感知损失进一步提高生成质量,使模型专注于全局风格。实验结果显示,该模型在超过2000个韩文字符上表现优越,可信赖地生成各种风格的韩国字体。
Key Takeaways
- 扩散模型用于自动字体生成(AFG),仅使用单一参考图像即可生成高质量、多样化的韩文手写和打印风格字体。
- 通过逐步去噪图像实现精细化的字体生成,确保稳定训练和良好的视觉效果。
- 创新使用文本编码器处理语音表示,可以生成准确且上下文正确的字符,包括对于未见字符的处理。
- 利用预训练的样式编码器和感知损失来提高生成质量,使模型关注全局风格。
- 模型在超过2000个韩文角色上的实验表现优越,证明其有效性和可靠性。
- 该模型适用于不同风格的韩国字体生成。
点此查看论文截图
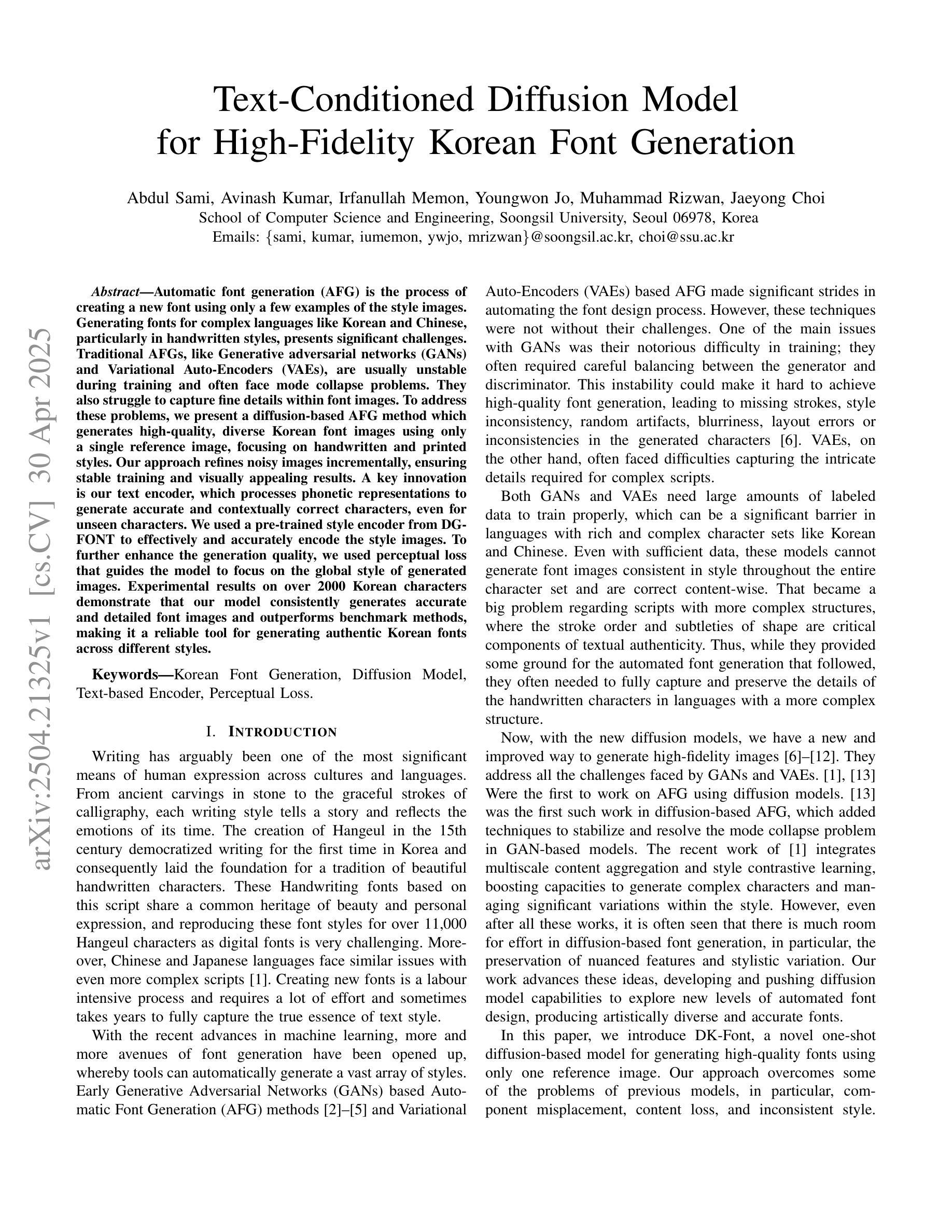
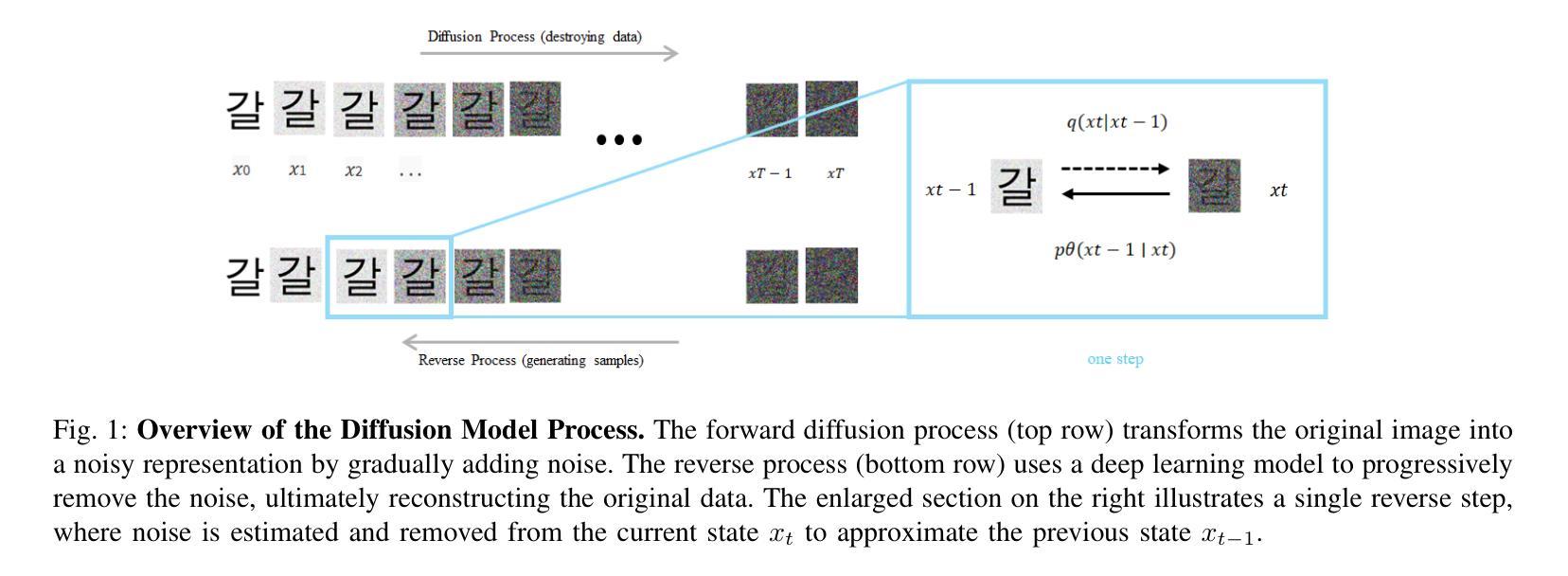
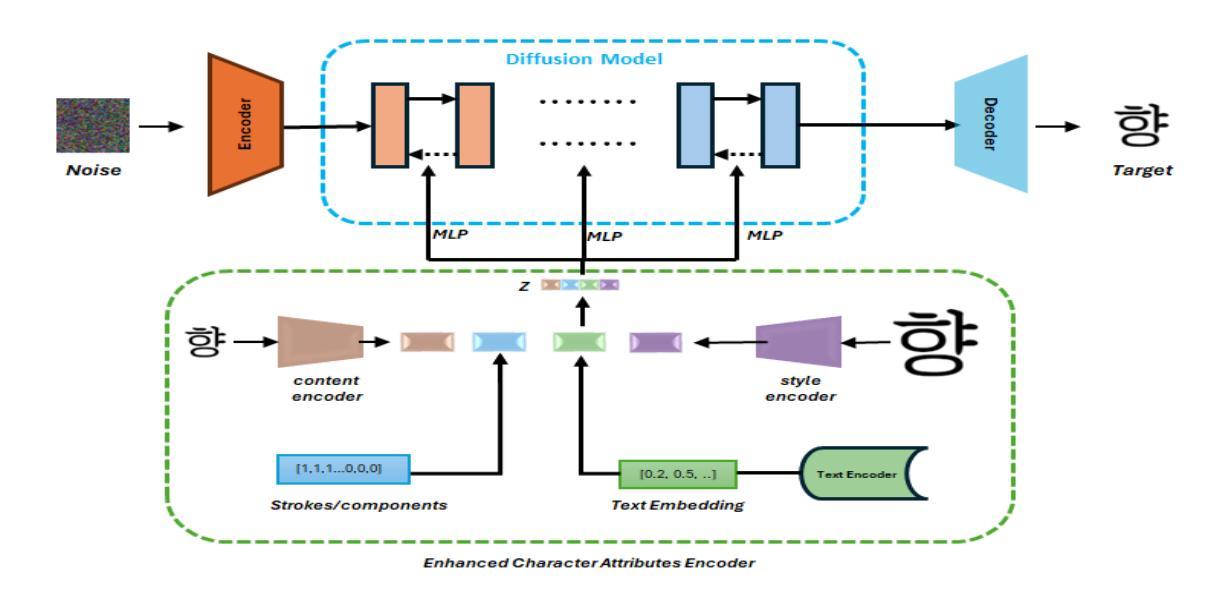
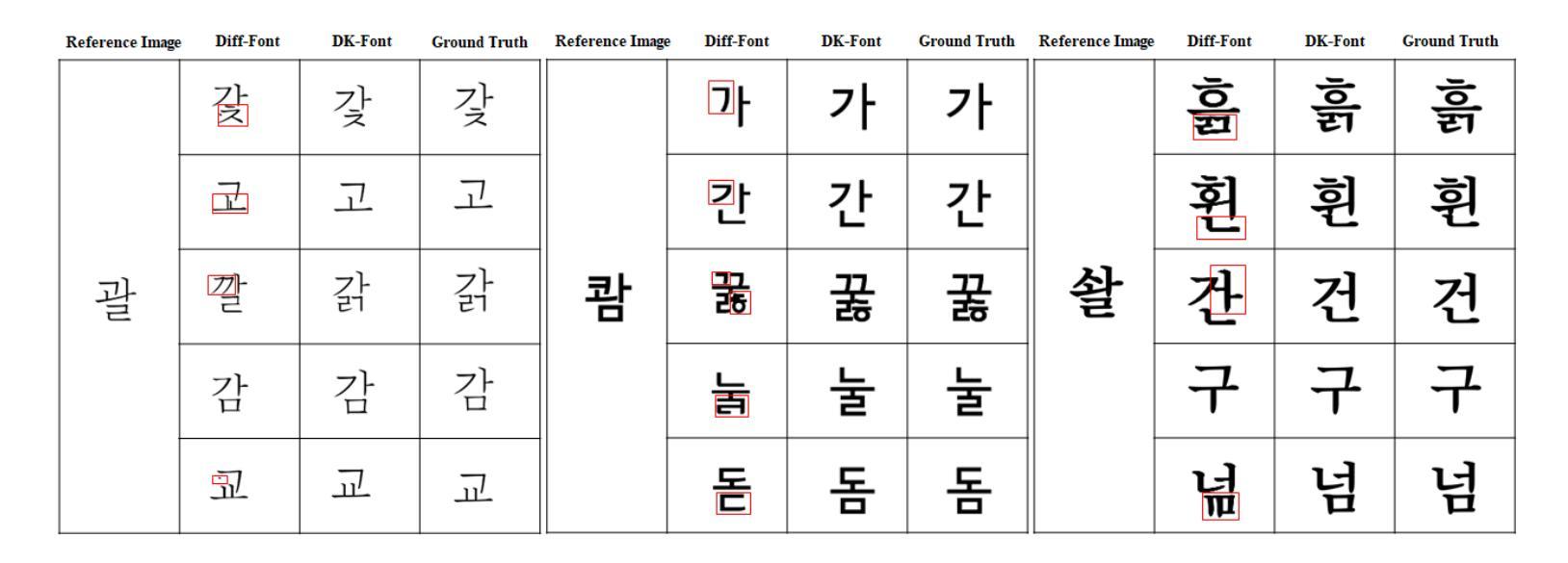
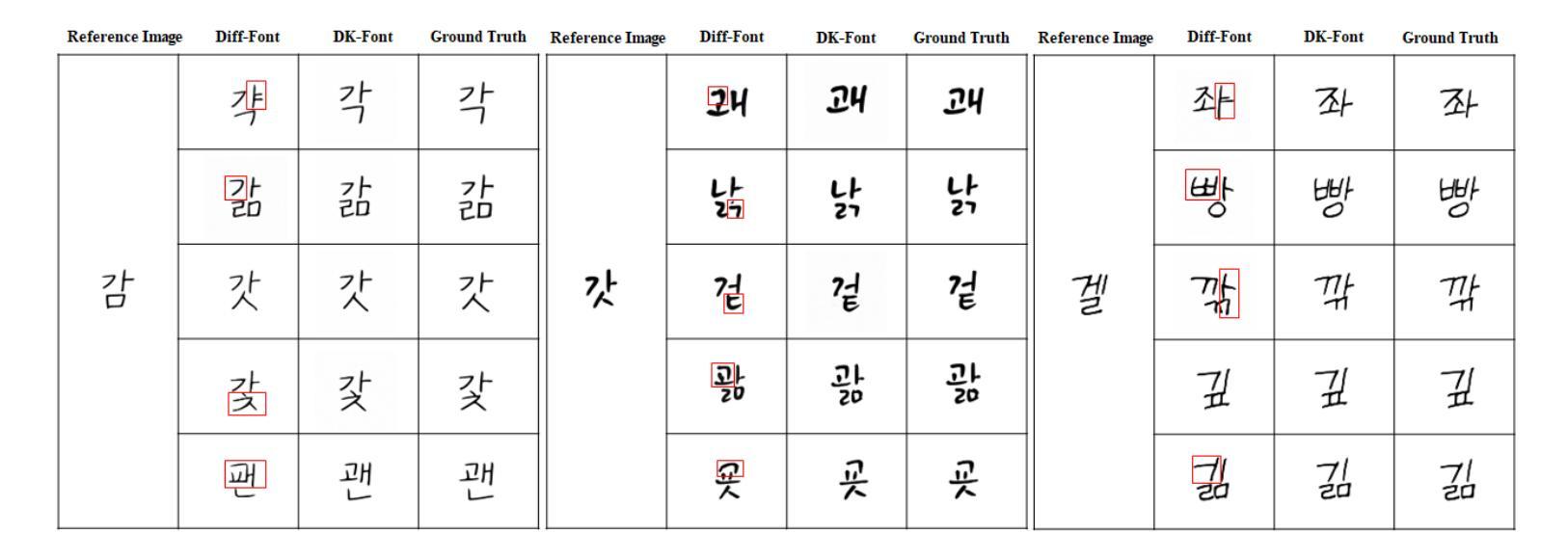
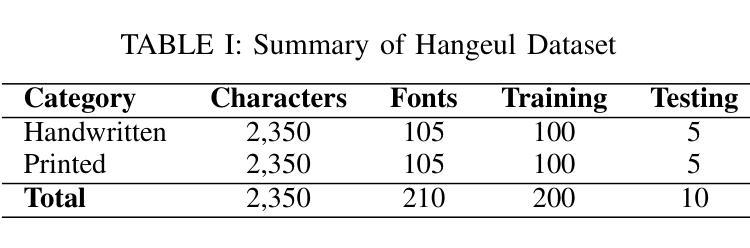
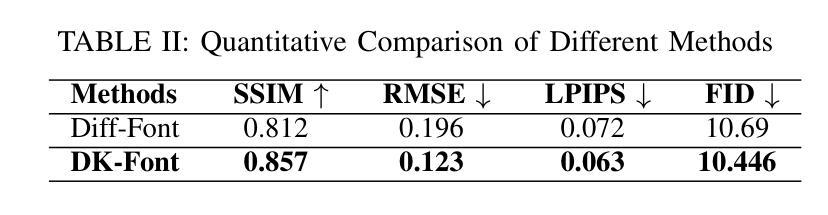
Generalizable Synthetic Image Detection via Language-guided Contrastive Learning
Authors:Haiwei Wu, Jiantao Zhou, Shile Zhang
The heightened realism of AI-generated images can be attributed to the rapid development of synthetic models, including generative adversarial networks (GANs) and diffusion models (DMs). The malevolent use of synthetic images, such as the dissemination of fake news or the creation of fake profiles, however, raises significant concerns regarding the authenticity of images. Though many forensic algorithms have been developed for detecting synthetic images, their performance, especially the generalization capability, is still far from being adequate to cope with the increasing number of synthetic models. In this work, we propose a simple yet very effective synthetic image detection method via a language-guided contrastive learning. Specifically, we augment the training images with carefully-designed textual labels, enabling us to use a joint visual-language contrastive supervision for learning a forensic feature space with better generalization. It is shown that our proposed LanguAge-guided SynThEsis Detection (LASTED) model achieves much improved generalizability to unseen image generation models and delivers promising performance that far exceeds state-of-the-art competitors over four datasets. The code is available at https://github.com/HighwayWu/LASTED.
人工智能生成的图像的真实性增强可归因于合成模型的快速发展,包括生成对抗网络(GANs)和扩散模型(DMs)。然而,合成图像被恶意使用的现象,如传播假新闻或创建虚假个人资料,引发了人们对图像真实性的重大担忧。虽然已开发出许多用于检测合成图像的前瞻性算法,但它们的表现,特别是其泛化能力,仍然远远不足以应对日益增多的合成模型。在本文中,我们提出了一种简单而有效的合成图像检测方法,即通过语言引导对比学习的方法。具体来说,我们用精心设计的文本标签增强训练图像,使我们能够使用联合视觉语言对比监督来学习一个具有更好泛化的法医特征空间。结果表明,我们提出的基于语言的合成检测(LASTED)模型对未见过的图像生成模型具有更强的泛化能力,并且在四个数据集上的性能均优于最先进的竞争对手。代码可在 https://github.com/HighwayWu/LASTED 找到。
论文及项目相关链接
Summary
本文关注AI生成的图像的真实性问题,阐述了随着合成模型(包括生成对抗网络(GANs)和扩散模型(DMs))的快速发展,AI生成的图像越来越逼真,但这也被恶意用于传播虚假信息或创建虚假个人资料。虽然已有许多用于检测合成图像的前端算法,但其性能尤其是泛化能力仍不足以应对不断增多的合成模型。本研究提出了一种简单而有效的合成图像检测方法,通过语言引导对比学习,使用精心设计的文本标签增强训练图像,以学习具有更好泛化能力的法医特征空间。所提出的LanguAge-guided SynThEsis Detection (LASTED)模型在未见过的图像生成模型上表现出优异的一般化能力,并在四个数据集上的性能远超现有竞争者。
Key Takeaways
- AI生成的图像真实性日益增强,引发了关于图像真实性的关注,尤其是其被恶意用于传播虚假信息的问题。
- 虽然已有许多用于检测合成图像的前端算法,但其泛化能力尚待提升。
- 本研究提出了一种新的合成图像检测方法——LanguAge-guided SynThEsis Detection (LASTED)模型。
- LASTED模型通过语言引导对比学习,使用文本标签增强训练图像。
- LASTED模型在未见过的图像生成模型上表现出优异的一般化能力。
- LASTED模型在四个数据集上的性能远超现有竞争者。
点此查看论文截图
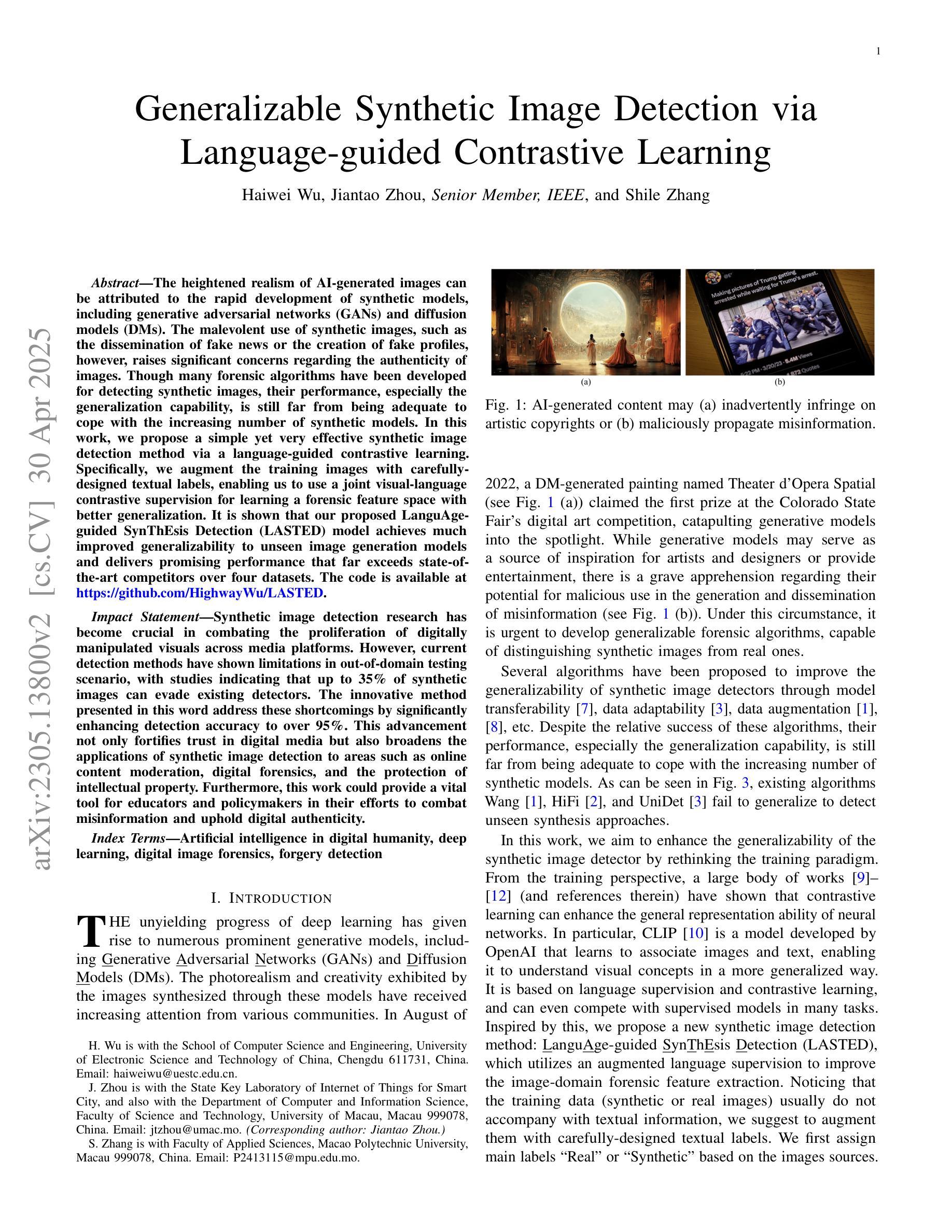
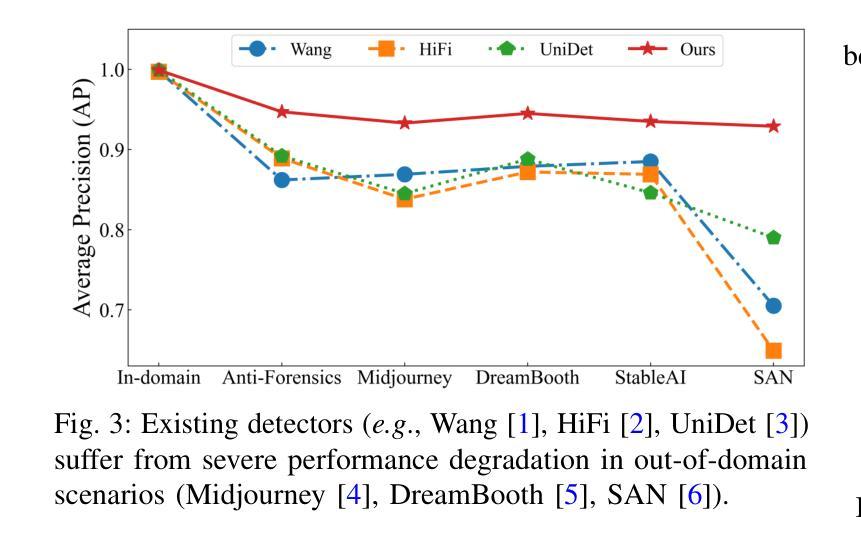
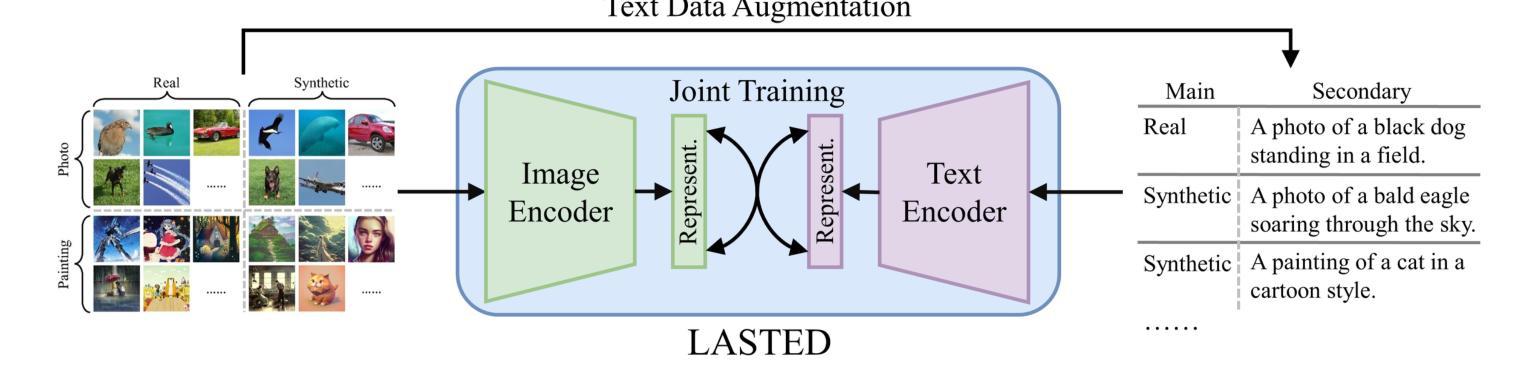
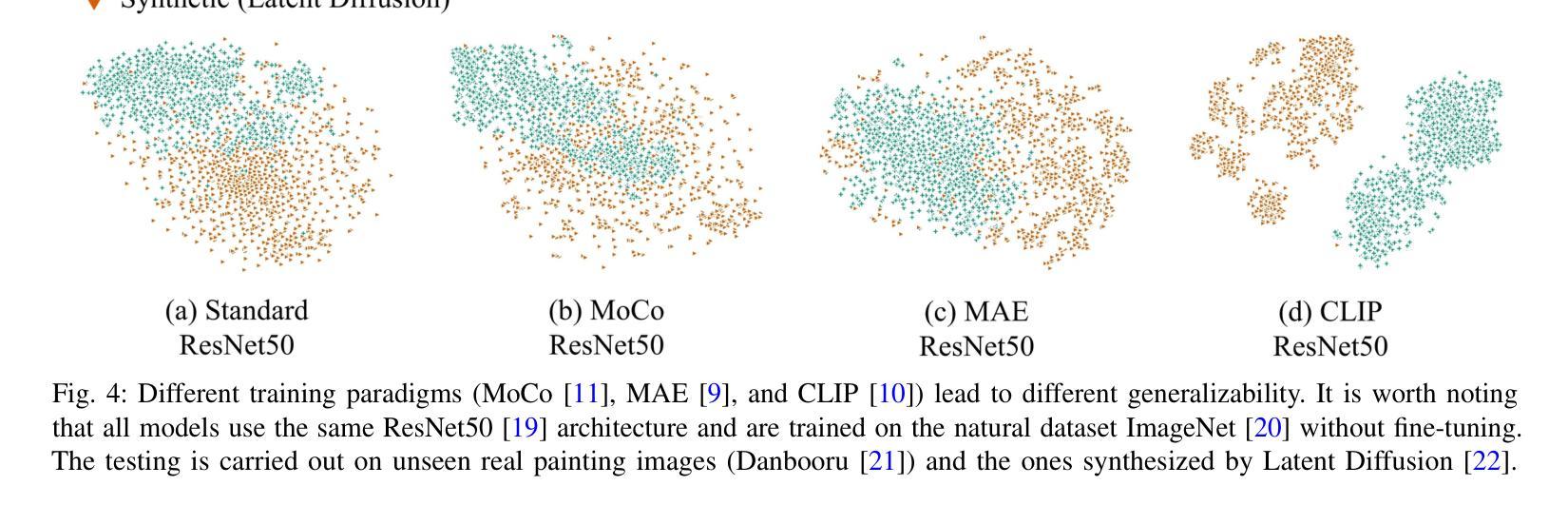
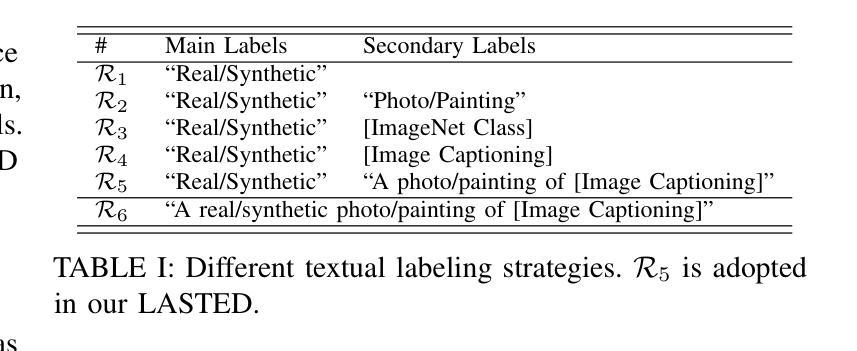
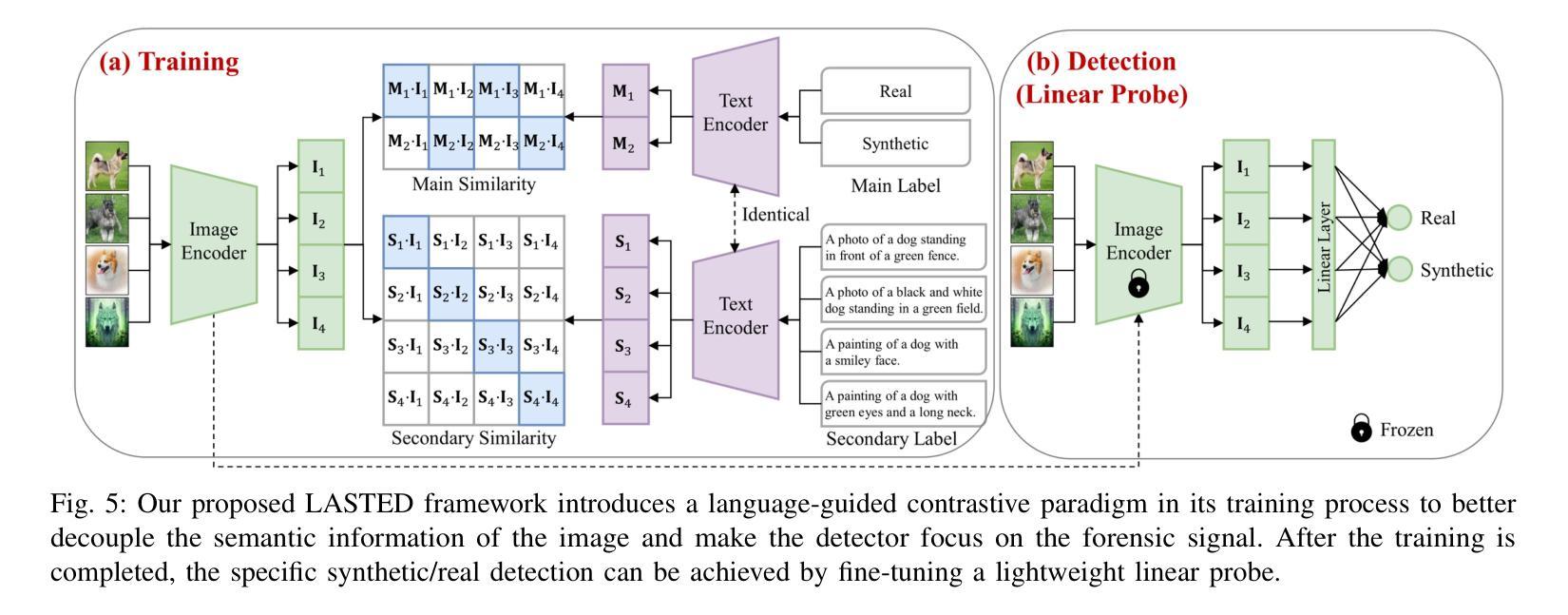
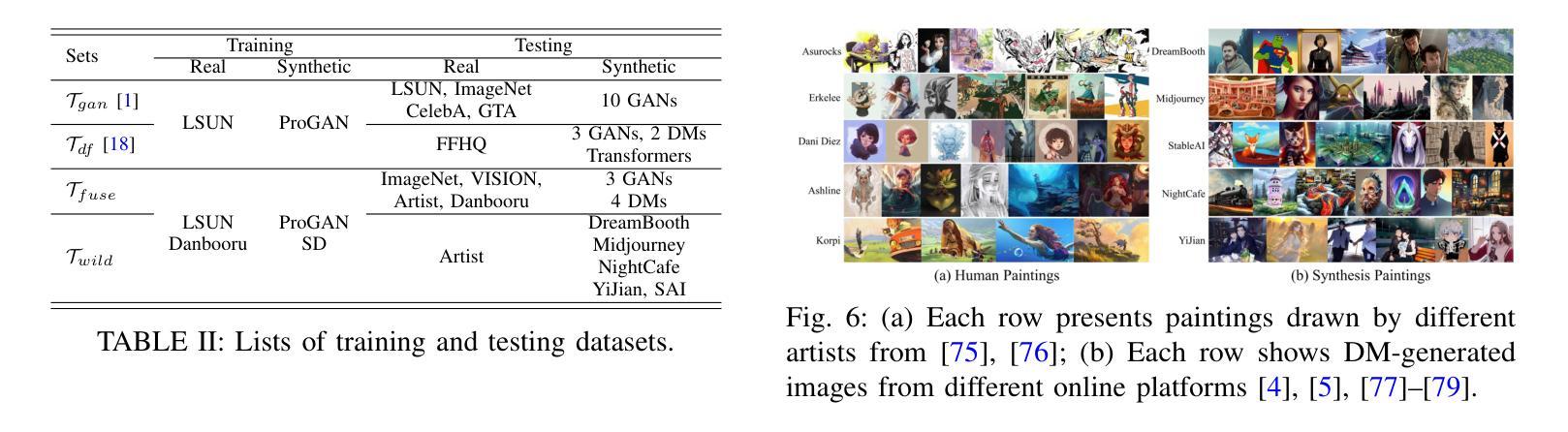
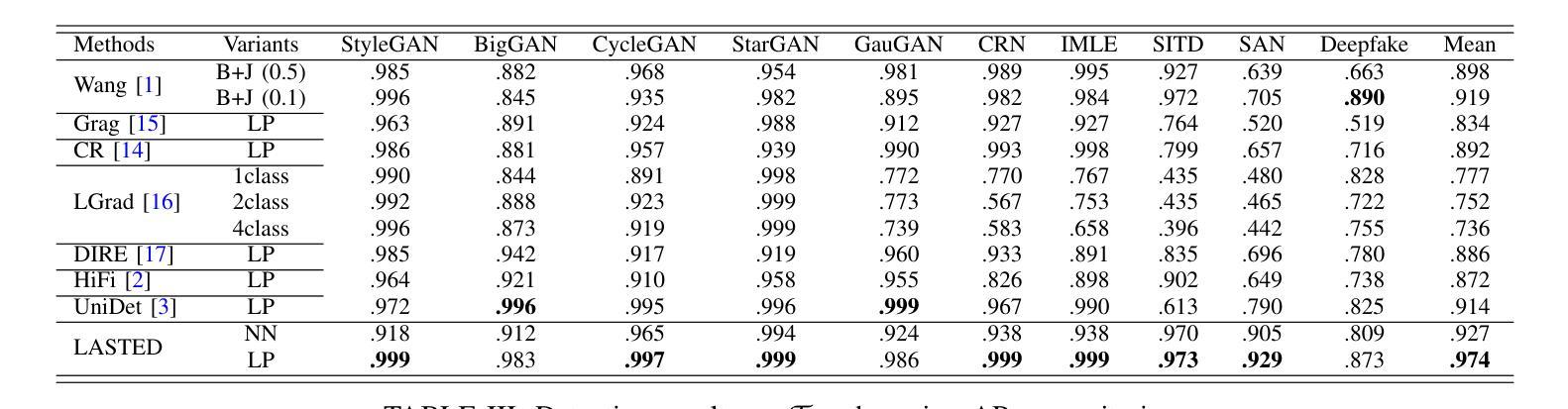
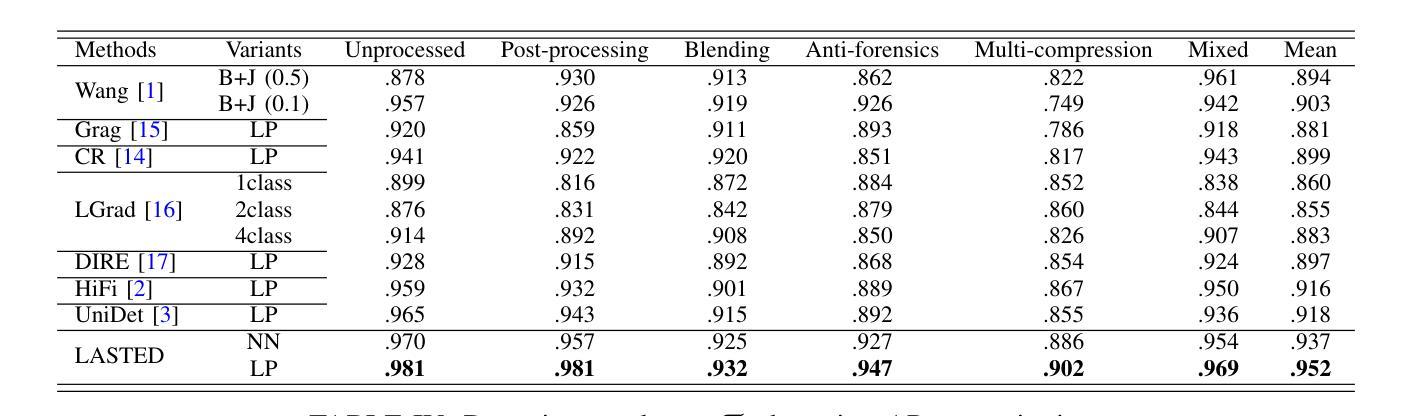