⚠️ 以下所有内容总结都来自于 大语言模型的能力,如有错误,仅供参考,谨慎使用
🔴 请注意:千万不要用于严肃的学术场景,只能用于论文阅读前的初筛!
💗 如果您觉得我们的项目对您有帮助 ChatPaperFree ,还请您给我们一些鼓励!⭐️ HuggingFace免费体验
2025-05-03 更新
Real-Time Animatable 2DGS-Avatars with Detail Enhancement from Monocular Videos
Authors:Xia Yuan, Hai Yuan, Wenyi Ge, Ying Fu, Xi Wu, Guanyu Xing
High-quality, animatable 3D human avatar reconstruction from monocular videos offers significant potential for reducing reliance on complex hardware, making it highly practical for applications in game development, augmented reality, and social media. However, existing methods still face substantial challenges in capturing fine geometric details and maintaining animation stability, particularly under dynamic or complex poses. To address these issues, we propose a novel real-time framework for animatable human avatar reconstruction based on 2D Gaussian Splatting (2DGS). By leveraging 2DGS and global SMPL pose parameters, our framework not only aligns positional and rotational discrepancies but also enables robust and natural pose-driven animation of the reconstructed avatars. Furthermore, we introduce a Rotation Compensation Network (RCN) that learns rotation residuals by integrating local geometric features with global pose parameters. This network significantly improves the handling of non-rigid deformations and ensures smooth, artifact-free pose transitions during animation. Experimental results demonstrate that our method successfully reconstructs realistic and highly animatable human avatars from monocular videos, effectively preserving fine-grained details while ensuring stable and natural pose variation. Our approach surpasses current state-of-the-art methods in both reconstruction quality and animation robustness on public benchmarks.
从单目视频中重建高质量、可动画的3D人类化身,对于减少复杂硬件的依赖具有显著潜力,在游戏开发、增强现实和社交媒体等应用中具有很高的实用性。然而,现有方法仍然面临着捕捉精细几何细节和保持动画稳定性的挑战,特别是在动态或复杂姿势下。为了解决这些问题,我们提出了一种基于二维高斯平铺(2DGS)的可动画人类化身重建的实时框架。通过利用二维高斯平铺和全局SMPL姿势参数,我们的框架不仅解决了位置和旋转误差的问题,还能够对重建的化身进行稳健且自然的姿态驱动动画。此外,我们引入了一个旋转补偿网络(RCN),该网络通过整合局部几何特征与全局姿态参数来学习旋转残差。该网络极大地提高了对非刚体变形的处理能力,确保了动画过程中姿态转换的流畅性且无伪影。实验结果表明,我们的方法成功地从单目视频中重建出逼真且高度可动画的人类化身,在保持精细细节的同时,确保了稳定的自然姿态变化。我们的方法在公共基准测试上的重建质量和动画稳定性均超过了当前最先进的方法。
论文及项目相关链接
Summary
本文提出了基于二维高斯投影实时框架的可动画人类角色重建方法,使用全球SMPL姿势参数与局部几何特征整合旋转补偿网络,旨在提高动态重建中的人形精度与动画稳定性。通过实现姿态驱动的动画并捕捉细致几何细节,该技术可广泛应用于游戏开发、增强现实和社会媒体等领域。相较于当前最前沿的方法,该技术在公开基准测试中实现了重建质量与动画稳定性的超越。
Key Takeaways
- 提出了基于二维高斯投影的实时框架进行可动画人类角色重建。
- 利用全球SMPL姿势参数,实现了姿态与旋转偏差的对齐。
- 引入旋转补偿网络(RCN),通过整合局部几何特征与全球姿势参数,提高了对非刚性变形的处理能力。
- 方法能有效捕捉精细几何细节并维持动画时的稳定性与自然姿态变化。
- 技术可广泛应用于游戏开发、增强现实和社会媒体等领域。
- 与当前最前沿的方法相比,该技术在公开基准测试中表现更优秀。
点此查看论文截图
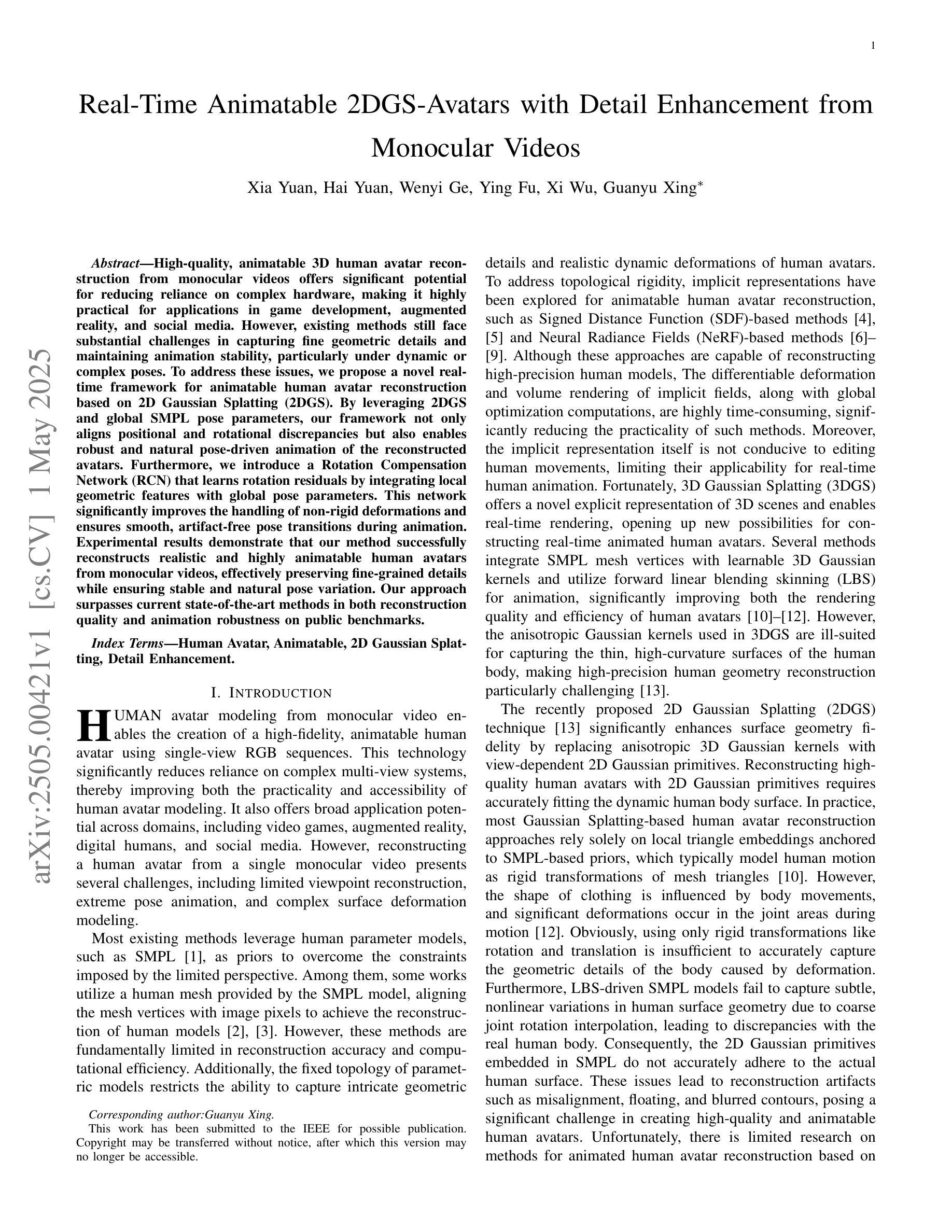
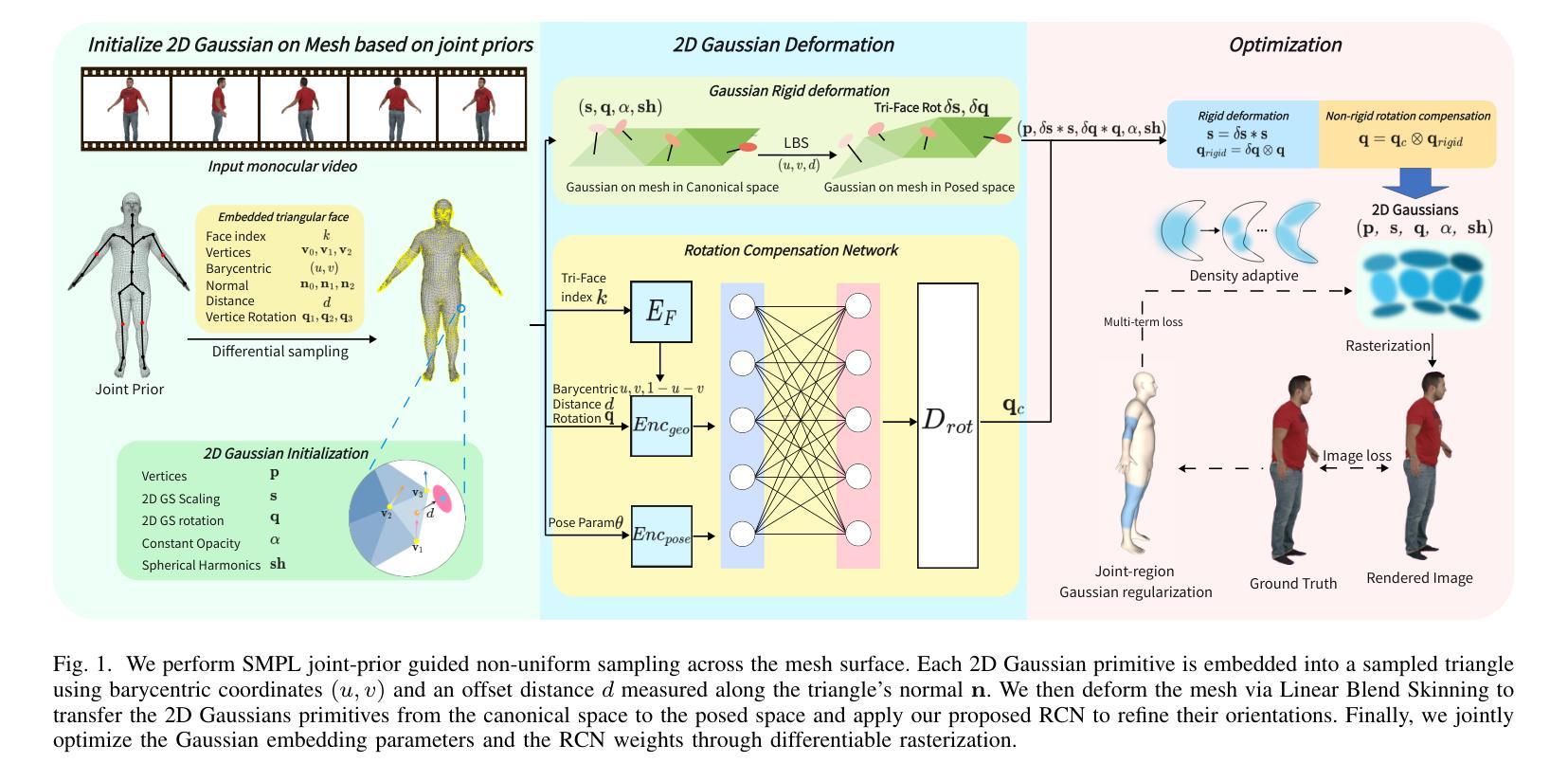
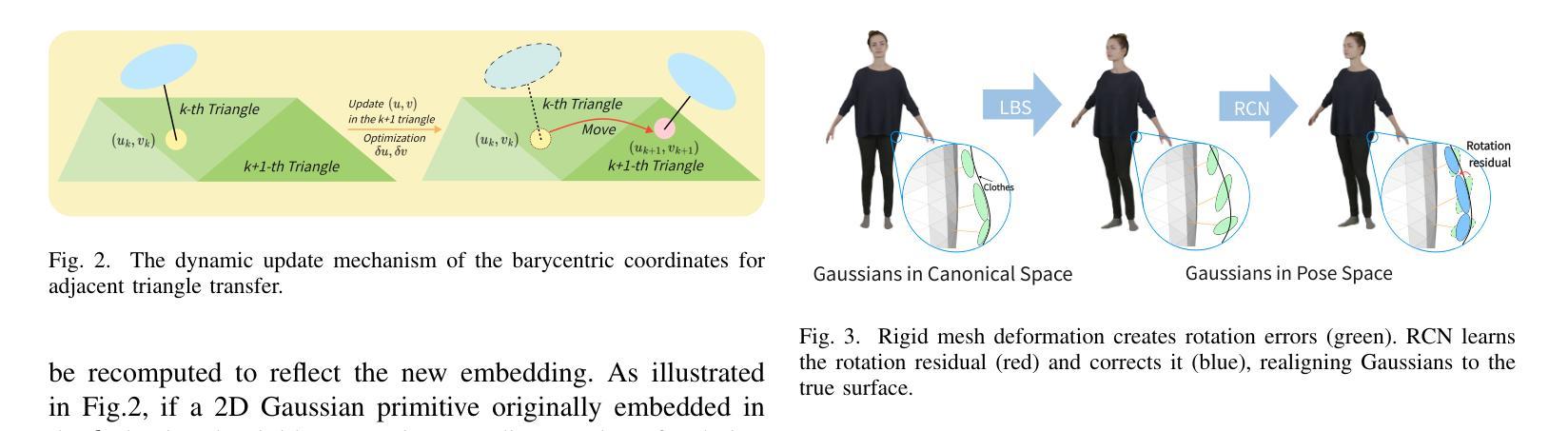
TransparentGS: Fast Inverse Rendering of Transparent Objects with Gaussians
Authors:Letian Huang, Dongwei Ye, Jialin Dan, Chengzhi Tao, Huiwen Liu, Kun Zhou, Bo Ren, Yuanqi Li, Yanwen Guo, Jie Guo
The emergence of neural and Gaussian-based radiance field methods has led to considerable advancements in novel view synthesis and 3D object reconstruction. Nonetheless, specular reflection and refraction continue to pose significant challenges due to the instability and incorrect overfitting of radiance fields to high-frequency light variations. Currently, even 3D Gaussian Splatting (3D-GS), as a powerful and efficient tool, falls short in recovering transparent objects with nearby contents due to the existence of apparent secondary ray effects. To address this issue, we propose TransparentGS, a fast inverse rendering pipeline for transparent objects based on 3D-GS. The main contributions are three-fold. Firstly, an efficient representation of transparent objects, transparent Gaussian primitives, is designed to enable specular refraction through a deferred refraction strategy. Secondly, we leverage Gaussian light field probes (GaussProbe) to encode both ambient light and nearby contents in a unified framework. Thirdly, a depth-based iterative probes query (IterQuery) algorithm is proposed to reduce the parallax errors in our probe-based framework. Experiments demonstrate the speed and accuracy of our approach in recovering transparent objects from complex environments, as well as several applications in computer graphics and vision.
神经和高斯基辐射场方法的出现为新视角合成和3D对象重建带来了重大进展。然而,由于辐射场对高频光变化的不稳定性和不正确的过度拟合,镜面反射和折射仍然构成重大挑战。目前,即使是强大且高效的3D高斯喷射(3D-GS)工具,在处理邻近内容的透明对象恢复时,也存在明显的二次射线效应问题。为了解决这一问题,我们提出了基于3D-GS的透明对象快速逆向渲染管道TransparentGS。主要贡献有三点。首先,设计了一种透明对象的高效表示方法,即透明高斯基元,以实现通过延迟折射策略的镜面折射。其次,我们利用高斯光场探针(GaussProbe)在统一框架中编码环境光和邻近内容。第三,提出了一种基于深度的迭代探针查询(IterQuery)算法,以减少我们基于探针的框架中的视差误差。实验证明,我们的方法在复杂环境中恢复透明对象的速度和准确性,以及在计算机图形和视觉中的一些应用表现良好。
论文及项目相关链接
PDF accepted by SIGGRAPH 2025; https://letianhuang.github.io/transparentgs/
Summary
神经网络和基于高斯的光场方法的发展极大地推动了新型视图合成和三维物体重建的进步。然而,由于高频光照变化下的辐射场不稳定和过度拟合问题,镜面反射和折射仍是重大挑战。针对透明物体附近内容的恢复问题,提出了基于三维高斯溅射(3D-GS)的快速逆向渲染管道TransparentGS。主要贡献包括:设计透明高斯原始模型,实现镜面折射的延迟折射策略;利用高斯光场探针(GaussProbe)统一编码环境光和附近内容;提出基于深度的迭代探针查询(IterQuery)算法,减少探针查询中的视差误差。实验证明,该方法在复杂环境下恢复透明物体速度更快、更准确,在计算机图形学和视觉领域有广泛应用。
Key Takeaways
- 神经网络和基于高斯的光场方法推动了三维重建和视图合成的进步。
- 镜面反射和折射仍是当前研究的重大挑战,尤其在高频光照变化下。
- 现有技术如3D高斯溅射(3D-GS)在恢复透明物体附近内容时存在局限。
- TransparentGS方法通过透明高斯原始模型、高斯光场探针和迭代查询算法解决了这一问题。
点此查看论文截图
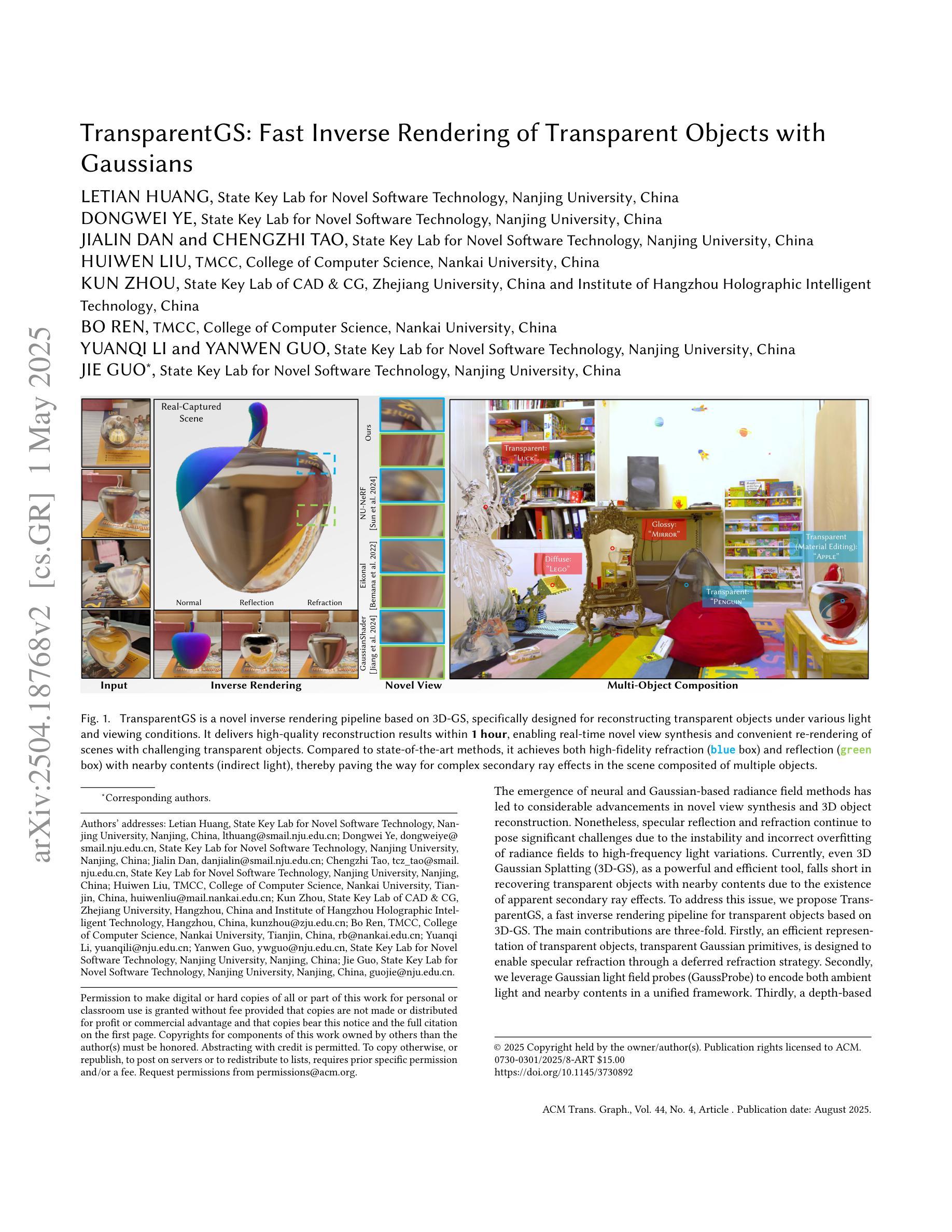
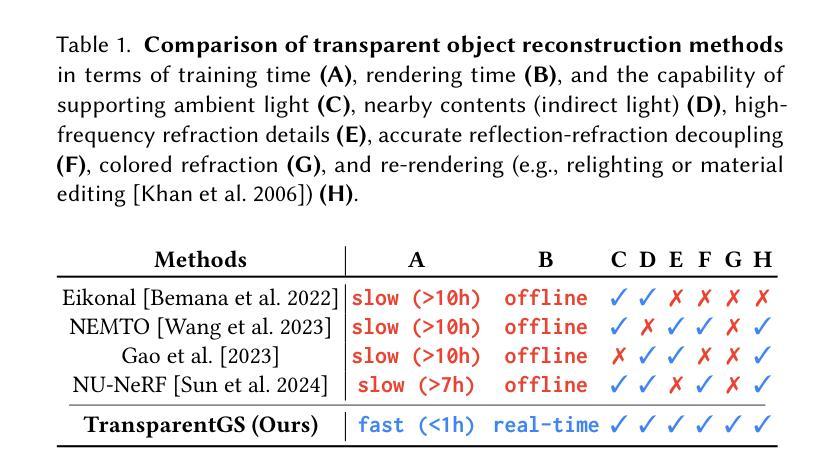
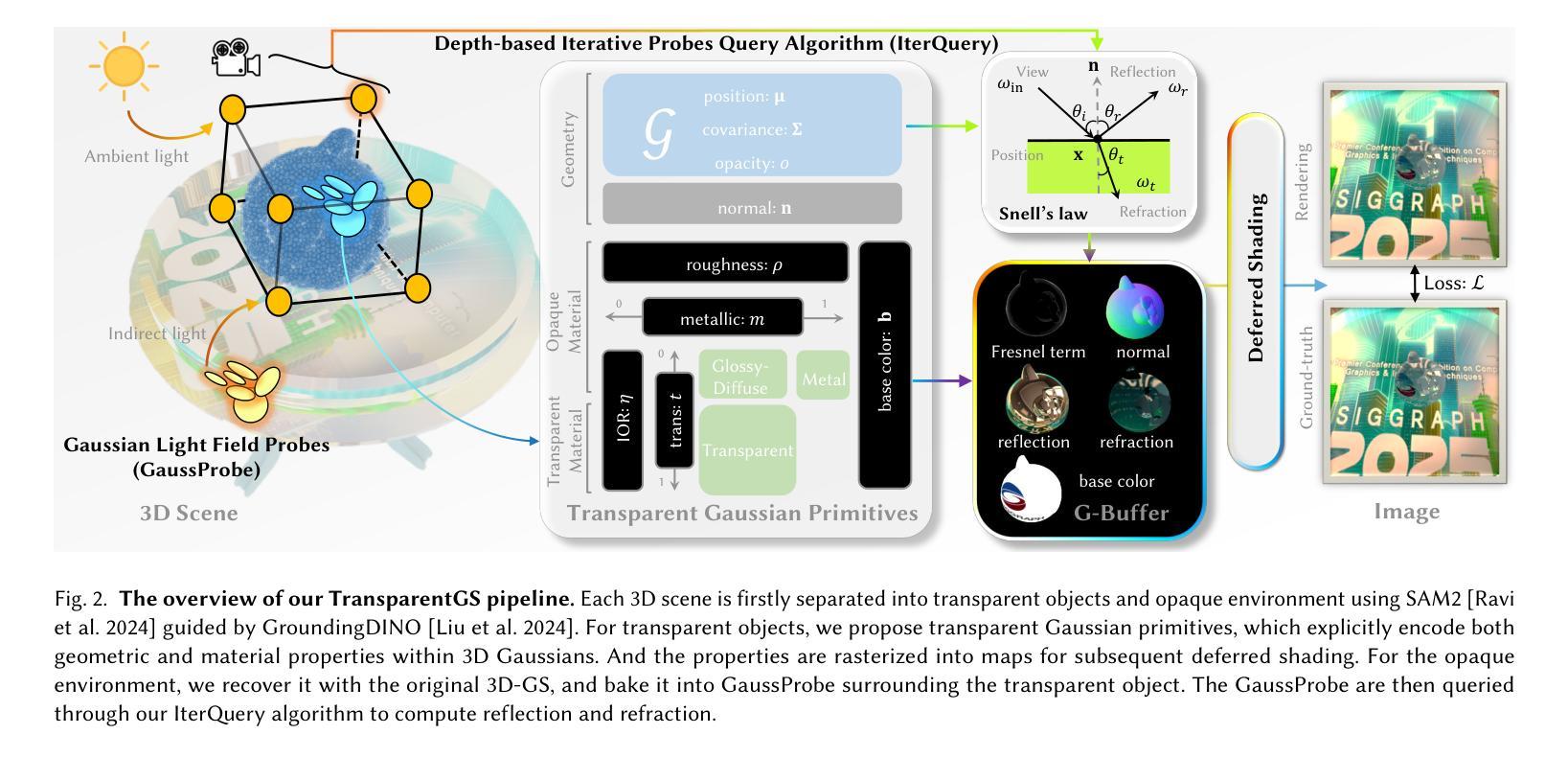
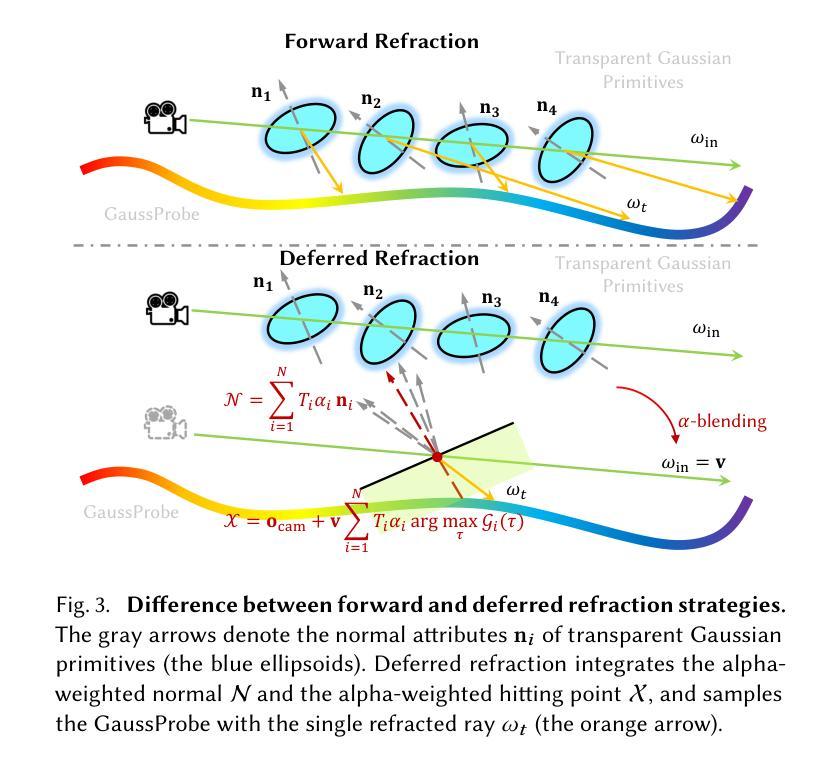
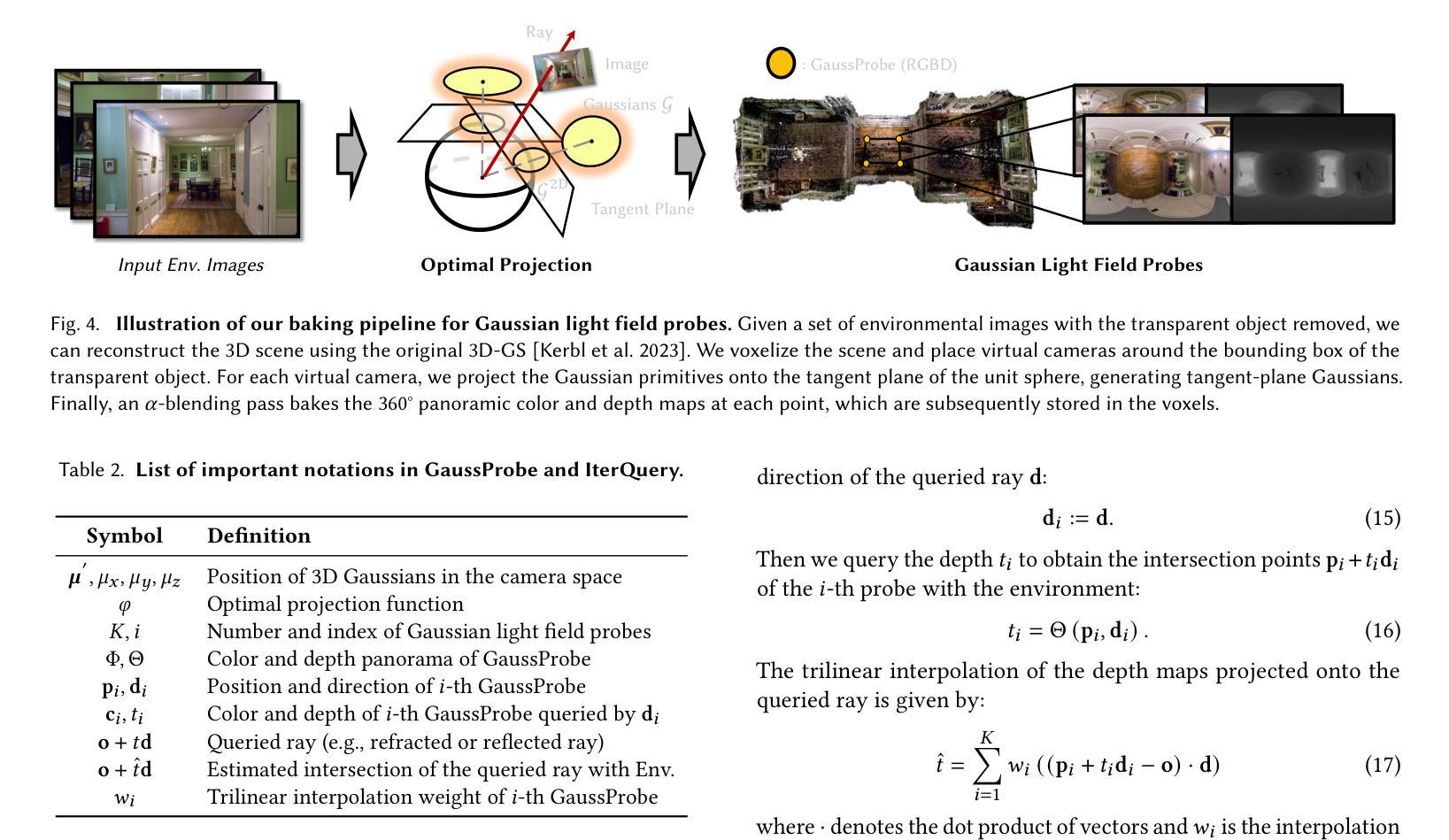
3D StreetUnveiler with Semantic-aware 2DGS – a simple baseline
Authors:Jingwei Xu, Yikai Wang, Yiqun Zhao, Yanwei Fu, Shenghua Gao
Unveiling an empty street from crowded observations captured by in-car cameras is crucial for autonomous driving. However, removing all temporarily static objects, such as stopped vehicles and standing pedestrians, presents a significant challenge. Unlike object-centric 3D inpainting, which relies on thorough observation in a small scene, street scene cases involve long trajectories that differ from previous 3D inpainting tasks. The camera-centric moving environment of captured videos further complicates the task due to the limited degree and time duration of object observation. To address these obstacles, we introduce StreetUnveiler to reconstruct an empty street. StreetUnveiler learns a 3D representation of the empty street from crowded observations. Our representation is based on the hard-label semantic 2D Gaussian Splatting (2DGS) for its scalability and ability to identify Gaussians to be removed. We inpaint rendered image after removing unwanted Gaussians to provide pseudo-labels and subsequently re-optimize the 2DGS. Given its temporal continuous movement, we divide the empty street scene into observed, partial-observed, and unobserved regions, which we propose to locate through a rendered alpha map. This decomposition helps us to minimize the regions that need to be inpainted. To enhance the temporal consistency of the inpainting, we introduce a novel time-reversal framework to inpaint frames in reverse order and use later frames as references for earlier frames to fully utilize the long-trajectory observations. Our experiments conducted on the street scene dataset successfully reconstructed a 3D representation of the empty street. The mesh representation of the empty street can be extracted for further applications. The project page and more visualizations can be found at: https://streetunveiler.github.io
从车载摄像头捕捉的拥挤观察中揭示空荡荡的街道对自动驾驶至关重要。然而,移除所有临时静止的物体,如停驶的车辆和站立的行人,是一项重大挑战。与依赖于小场景彻底观察的以物体为中心的3D补全不同,街道场景的情况涉及长期轨迹,与之前的3D补全任务不同。由于物体观察的有限程度和持续时间,捕获视频的以相机为中心的运动环境进一步加剧了任务的复杂性。为了解决这些障碍,我们引入了StreetUnveiler来重建一条空荡荡的街道。StreetUnveiler从拥挤的观察中学习街道的空旷3D表示。我们的表示基于硬标签语义2D高斯拼贴(2DGS),因其可扩展性和识别要移除的高斯的能力。我们在移除不需要的高斯后补全渲染图像以提供伪标签,然后重新优化2DGS。考虑到其时间上的连续运动,我们将空旷的街道场景分为已观察、部分观察和未观察的区域,我们建议通过渲染的alpha地图来定位。这种分解有助于我们最小化需要补全的区域。为了提高补全的时间一致性,我们引入了一个新颖的时间反转框架,按相反顺序补全帧并使用后续的帧作为早期帧的参考,以充分利用长轨迹观察。我们在街道场景数据集上进行的实验成功地重建了街道的空旷3D表示。空荡荡的街道的网格表示可以提取用于进一步应用。项目和更多可视化内容可在以下网址找到:https://streetunveiler.github.io
论文及项目相关链接
PDF Project page: https://streetunveiler.github.io
Summary
本文介绍了在自动驾驶领域中,从车载摄像头捕捉的拥挤街道场景中重建空街道的重要性及挑战。为应对这些挑战,研究团队引入了StreetUnveiler,它能从拥挤的观察中学习空街道的3D表示。通过基于硬标签语义的2D高斯斑点法(2DGS)进行表示,该方法具有良好的可扩展性和去除需移除的高斯斑点的能力。在渲染图像去除不需要的高斯斑点后进行填充,提供伪标签并重新优化2DGS。研究团队还将空街道场景分为观察区、部分观察区和未观察区,并通过渲染的alpha地图进行定位,以减少需要填充的区域。为提高填充的时空一致性,研究团队采用反向时间框架,按反向顺序填充帧并利用后续帧作为早期帧的参考,充分利用长轨迹观察。实验证明,StreetUnveiler成功重建了空街道的3D表示,可为进一步应用提取网格表示。
Key Takeaways
- 自动驾驶中从拥挤的街道场景中重建空街道至关重要,但移除静态物体是一大挑战。
- StreetUnveiler能从拥挤的观察中学习空街道的3D表示。
- 采用基于硬标签语义的2D高斯斑点法(2DGS)进行表示,具有良好的可扩展性和去除特定物体的能力。
- 通过渲染图像去除不需要的高斯斑点后进行填充,并提供伪标签进行优化。
- 将空街道场景分为观察、部分观察和未观察区域,通过渲染的alpha地图定位,减少需填充区域。
- 采用时间反向框架提高填充的时空一致性。
点此查看论文截图
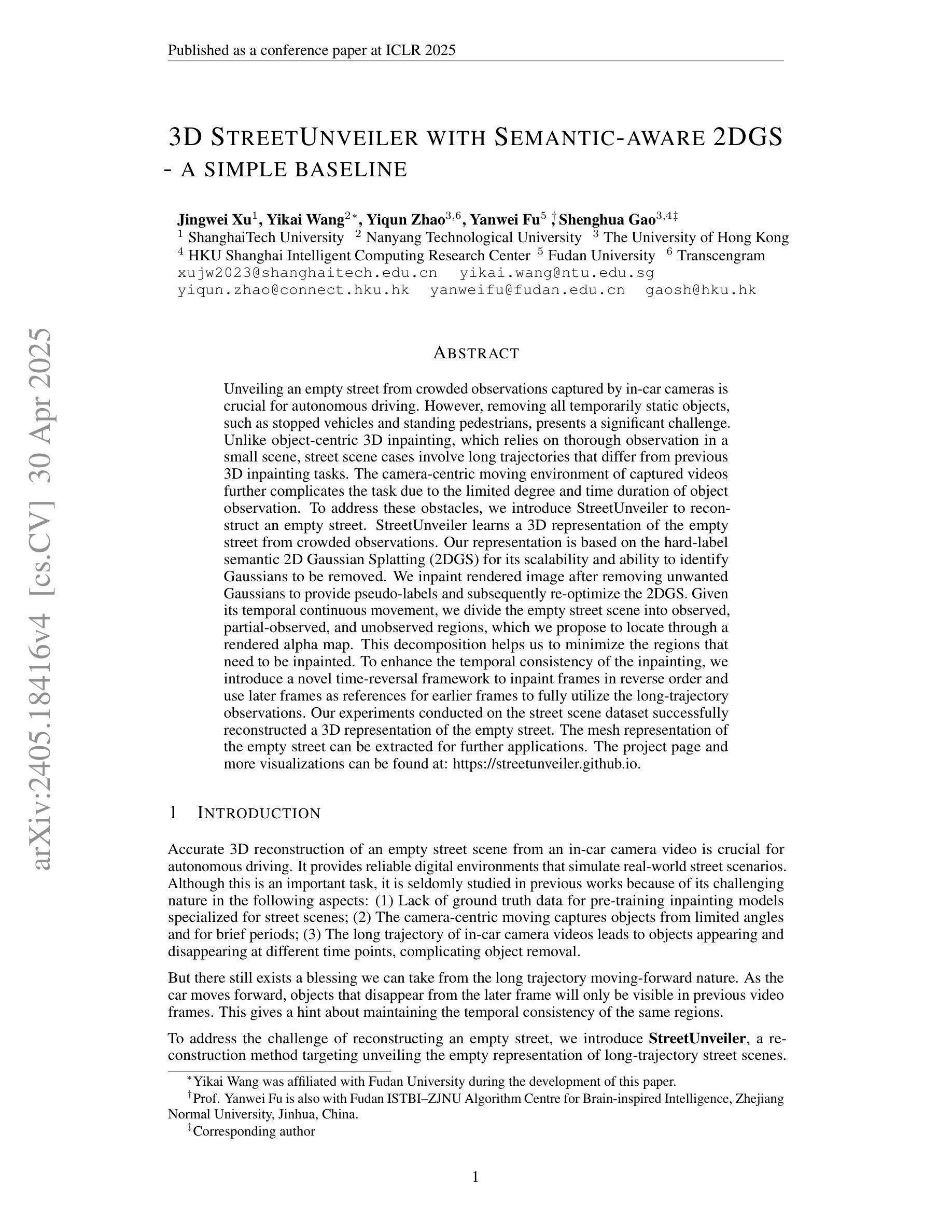
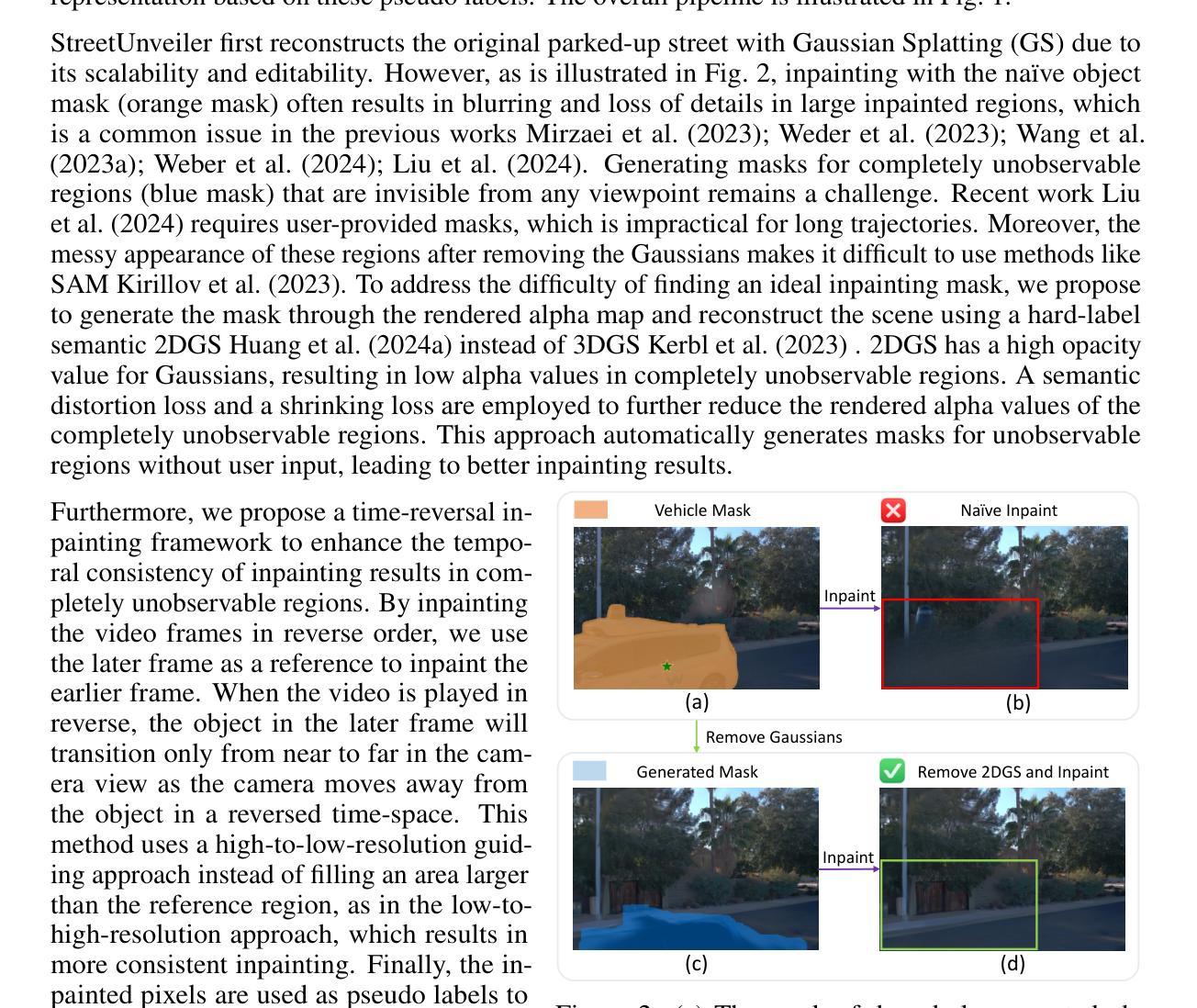
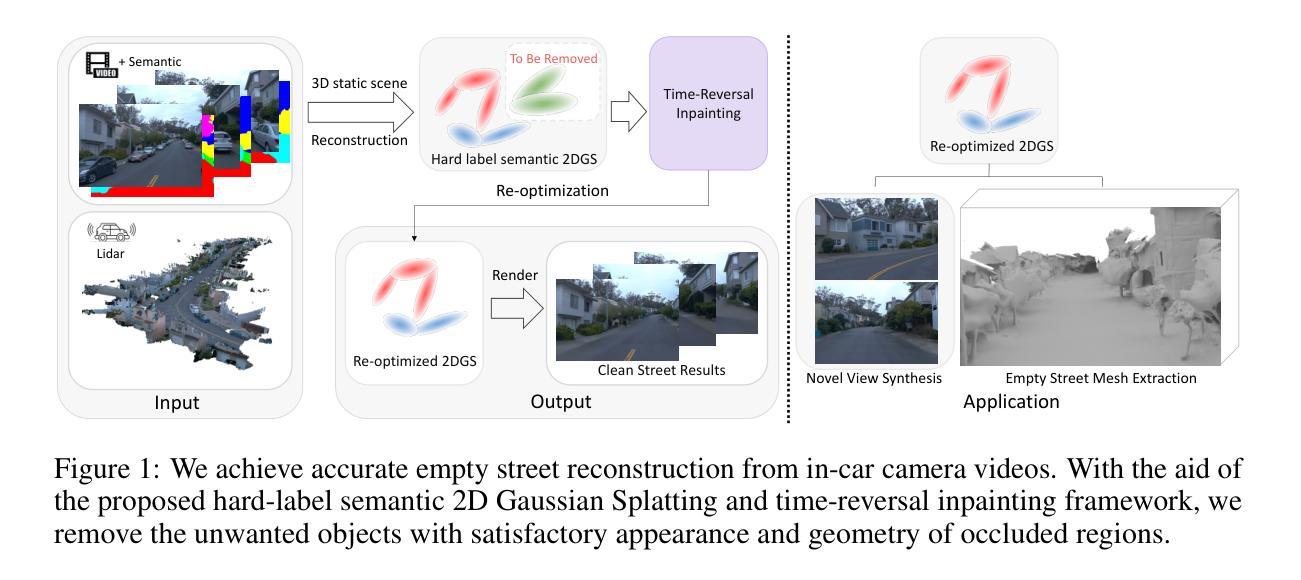