⚠️ 以下所有内容总结都来自于 大语言模型的能力,如有错误,仅供参考,谨慎使用
🔴 请注意:千万不要用于严肃的学术场景,只能用于论文阅读前的初筛!
💗 如果您觉得我们的项目对您有帮助 ChatPaperFree ,还请您给我们一些鼓励!⭐️ HuggingFace免费体验
2025-05-03 更新
Perceptual Implications of Automatic Anonymization in Pathological Speech
Authors:Soroosh Tayebi Arasteh, Saba Afza, Tri-Thien Nguyen, Lukas Buess, Maryam Parvin, Tomas Arias-Vergara, Paula Andrea Perez-Toro, Hiu Ching Hung, Mahshad Lotfinia, Thomas Gorges, Elmar Noeth, Maria Schuster, Seung Hee Yang, Andreas Maier
Automatic anonymization techniques are essential for ethical sharing of pathological speech data, yet their perceptual consequences remain understudied. This study presents the first comprehensive human-centered analysis of anonymized pathological speech, using a structured perceptual protocol involving ten native and non-native German listeners with diverse linguistic, clinical, and technical backgrounds. Listeners evaluated anonymized-original utterance pairs from 180 speakers spanning Cleft Lip and Palate, Dysarthria, Dysglossia, Dysphonia, and age-matched healthy controls. Speech was anonymized using state-of-the-art automatic methods (equal error rates in the range of 30-40%). Listeners completed Turing-style discrimination and quality rating tasks under zero-shot (single-exposure) and few-shot (repeated-exposure) conditions. Discrimination accuracy was high overall (91% zero-shot; 93% few-shot), but varied by disorder (repeated-measures ANOVA: p=0.007), ranging from 96% (Dysarthria) to 86% (Dysphonia). Anonymization consistently reduced perceived quality (from 83% to 59%, p<0.001), with pathology-specific degradation patterns (one-way ANOVA: p=0.005). Native listeners rated original speech slightly higher than non-native listeners (Delta=4%, p=0.199), but this difference nearly disappeared after anonymization (Delta=1%, p=0.724). No significant gender-based bias was observed. Critically, human perceptual outcomes did not correlate with automatic privacy or clinical utility metrics. These results underscore the need for listener-informed, disorder- and context-specific anonymization strategies that preserve privacy while maintaining interpretability, communicative functions, and diagnostic utility, especially for vulnerable populations such as children.
自动匿名化技术在伦理共享病理性语音数据方面至关重要,但其感知影响仍缺乏研究。本研究采用以人为中心的结构化感知协议,首次全面分析了匿名化病理性语音,协议涉及具有不同语言、临床和技术背景的10名德语母语和非母语听众。听众评估了来自180名演讲者的匿名化原始语句对,包括唇裂和腭裂、言语障碍、发音障碍、嗓音障碍以及年龄匹配的对照组。语音使用最先进的自动方法进行匿名化处理(误差率范围在30%~40%之间)。听众在零样本(单次曝光)和少样本(多次曝光)条件下完成了图灵风格的辨别和质量评分任务。总体而言,辨别准确率较高(零样本91%;少样本93%),但不同疾病之间存在差异(重复测量方差分析:p=0.007),范围从96%(言语障碍)到86%(嗓音障碍)。匿名化处理一致降低了感知质量(从83%降至59%,p<0.001),且存在特定病理的退化模式(单向方差分析:p=0.005)。母语听众对原始语音的评分略高于非母语听众(差异值为4%,p=0.199),但在匿名处理后这种差异几乎消失(差异值为1%,p=0.724)。未观察到明显的性别偏见。关键的是,人类感知结果与自动隐私或临床实用指标无相关性。这些结果强调,需要基于听众信息、特定疾病和特定语境的匿名化策略,既保护隐私又保持解释性、交际功能和诊断效用,特别是对于儿童等脆弱人群。
论文及项目相关链接
Summary
本文研究了自动匿名化技术在病理语音数据共享中的重要作用,并通过以人为中心的结构化感知协议对匿名化后的病理语音进行了综合分析。结果显示,尽管匿名化技术的歧视准确性高,但会对语音质量产生一定影响,不同病理类型的语音受影响程度不同。同时,原语音与匿名化后语音之间的差异在不同语言背景的听众间也略有不同。本研究强调了需要开发能够根据病理类型、背景和诊断用途制定个性化策略的听众知情匿名化技术,以在保护隐私的同时保持解释性、沟通功能和诊断效用。
Key Takeaways
- 自动匿名化技术在伦理共享病理语音数据方面至关重要。
- 采用结构化的感知协议进行以人为中心的分析。
- 匿名化技术的高歧视准确性同时伴随着语音质量的降低。
- 不同病理类型的语音在匿名化后受到的感知影响不同。
- 原语音与匿名化语音之间的差异在不同背景的听众间存在差异。
- 原语听众和非原语听众对语音评价的差异在匿名化后变得不明显。
- 需要开发能够适应不同病理类型、背景和诊断用途的个性化匿名化策略。
点此查看论文截图
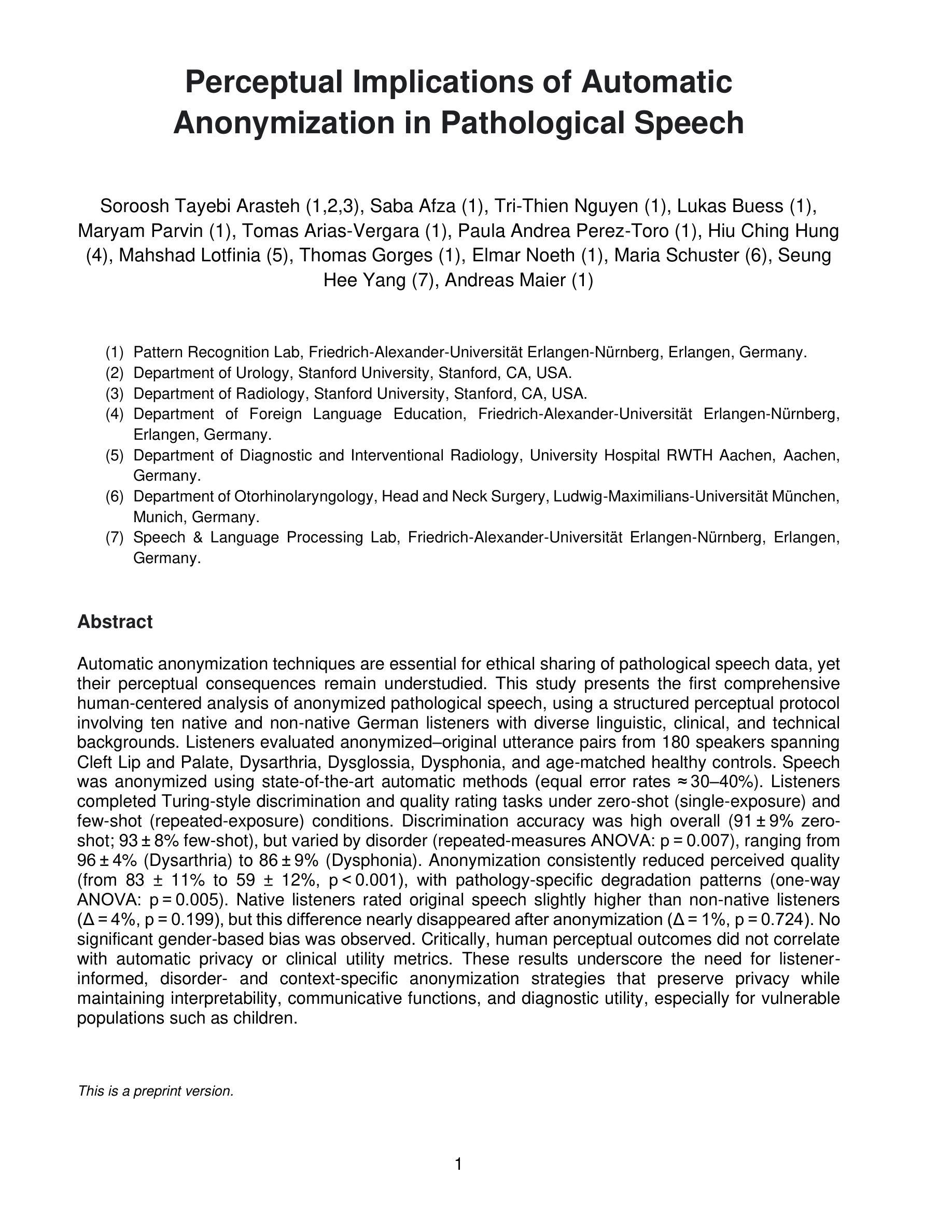
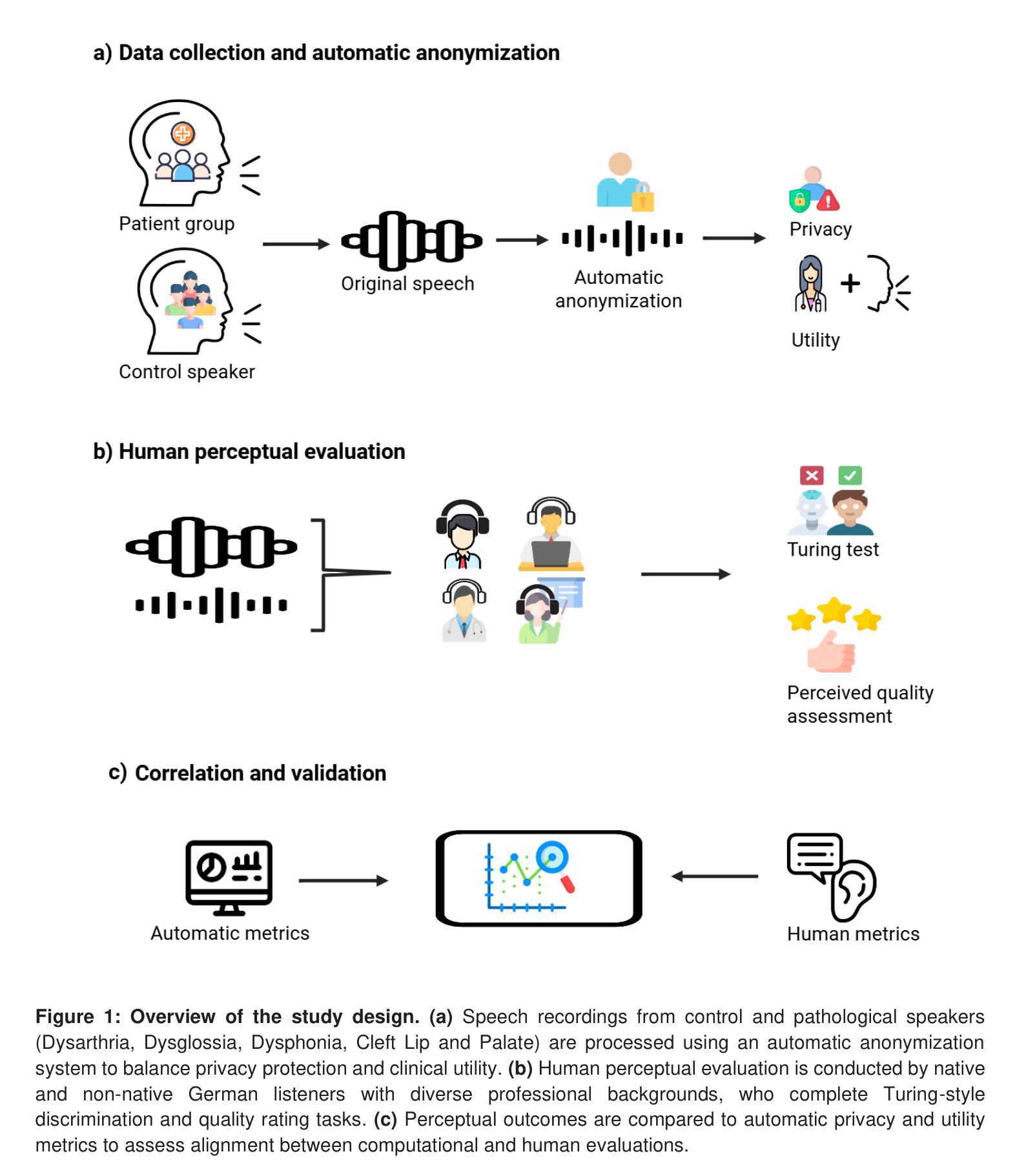
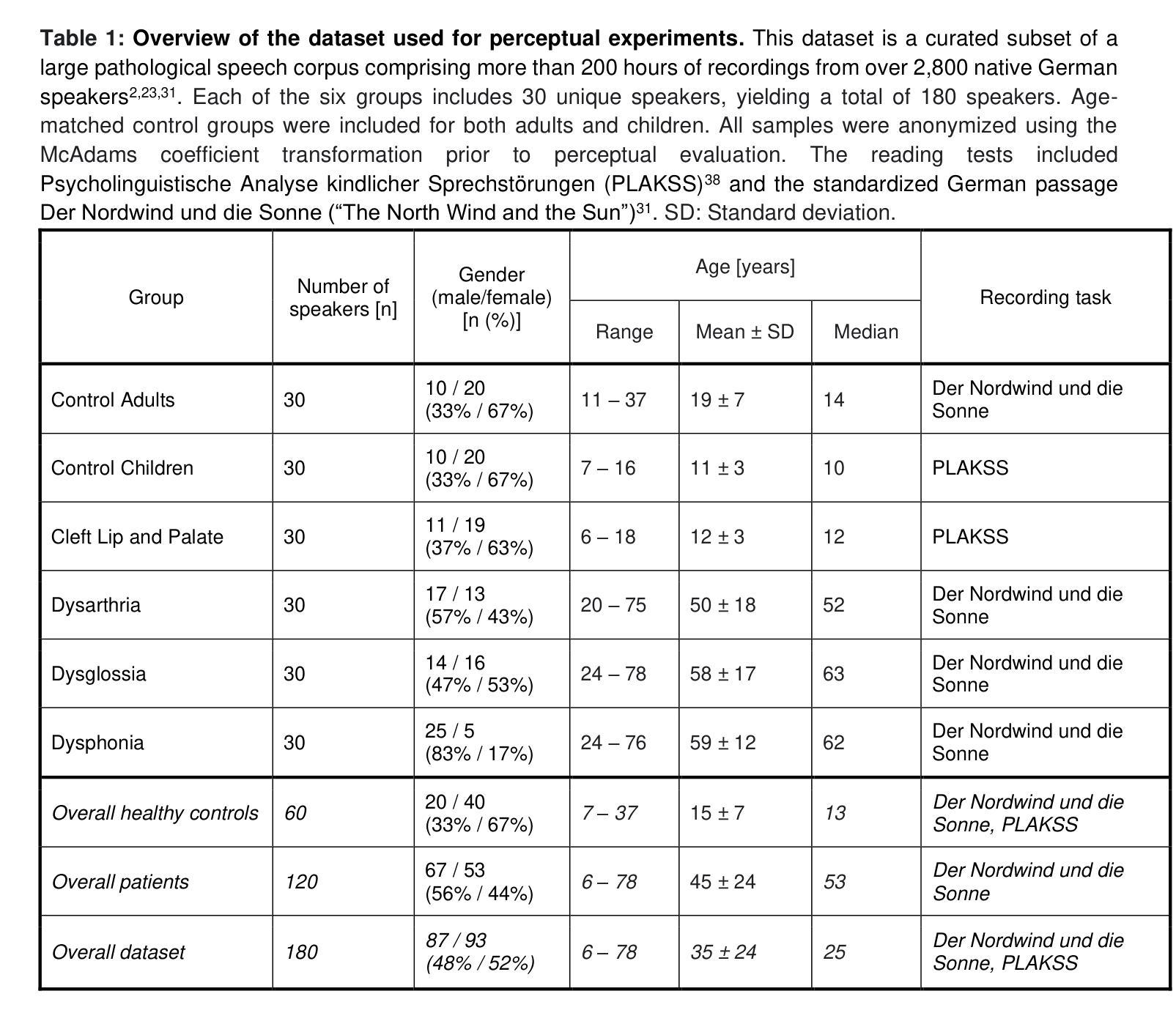
HyPerAlign: Hypotheses-driven Personalized Alignment
Authors:Cristina Garbacea, Chenhao Tan
Alignment algorithms are widely used to align large language models (LLMs) to human users based on preference annotations that reflect their intended real-world use cases. Typically these (often divergent) preferences are aggregated over a diverse set of users, resulting in fine-tuned models that are aligned to the ``average-user’’ preference. Nevertheless, current models are used by individual users in very specific contexts and situations, emphasizing the need for user-dependent preference control. In this work we address the problem of personalizing LLM outputs to their users, aiming to generate customized responses tailored to individual users, instead of generic outputs that emulate the collective voices of diverse populations. We propose a novel interpretable and sample-efficient hypotheses-driven personalization approach (HyPerAlign) where given few-shot examples written by a particular user, we first infer hypotheses about their communication strategies, personality and writing style, then prompt LLM models with these hypotheses and user specific attributes to generate customized outputs. We conduct experiments on two different personalization tasks, authorship attribution and deliberative alignment, with datasets from diverse domains (news articles, blog posts, emails, jailbreaking benchmarks), and demonstrate the superiority of hypotheses-driven personalization approach when compared to preference-based fine-tuning methods. For deliberative alignment, the helpfulness of LLM models is improved by up to $70%$ on average. For authorship attribution, results indicate consistently high win-rates (commonly $>90%$) against state-of-the-art preference fine-tuning approaches for LLM personalization across diverse user profiles and LLM models. Overall, our approach represents an interpretable and sample-efficient strategy for the personalization of LLM models to individual users.
对齐算法广泛应用于根据偏好注释将大型语言模型(LLM)与人类用户进行对齐,这些偏好注释反映了它们预定的现实世界用例。通常这些(经常不同的)偏好会在一组多样化的用户中进行汇总,从而产生与“平均用户”偏好对齐的微调模型。然而,当前模型是由单个用户在特定上下文和情境中使用的,这强调了用户偏好控制的必要性。在这项工作中,我们解决了将LLM输出个性化以适应其用户的问题,旨在生成针对个别用户的定制响应,而不是模拟不同人群集体声音的通用输出。我们提出了一种新颖的可解释性和样本效率高的假设驱动个性化方法(HyPerAlign),给定特定用户编写的少数示例,我们首先推断他们的沟通策略、个性和写作风格方面的假设,然后提示LLM模型结合这些假设和用户特定属性来生成定制输出。我们在两个不同的个性化任务(作者身份识别和审慎对齐)上进行了实验,使用了来自不同领域的数据集(新闻文章、博客文章、电子邮件、越狱基准测试),并证明了假设驱动个性化方法在对比基于偏好的微调方法时的优越性。对于审慎对齐,LLM模型的实用性平均提高了高达70%。对于作者身份识别,结果表明,在多种用户配置文件和LLM模型的情况下,与最新的LLM个性化偏好微调方法相比,我们的方法胜率一直很高(通常超过90%)。总的来说,我们的方法代表了可解释性和样本效率高的策略,用于将LLM模型个性化适应于单个用户。
论文及项目相关链接
Summary
本文提出一种针对大型语言模型(LLM)的个人化对齐方法。通过用户特定的少数样本例子,推断用户的沟通策略、个性和写作风格,进而生成个性化的输出响应,而非通用输出。实验结果表明,该假设驱动的个人化对齐方法在两种不同的个人化任务上优于基于偏好的微调方法。
Key Takeaways
- 大型语言模型(LLM)通常基于反映真实使用情况的偏好注释进行对齐。
- 目前模型在处理特定用户时,无法很好地进行个性化输出响应。这导致生成的响应通常更像集体的声音而非个性化。
- 提出了一种新的假设驱动的个人化对齐方法(HyPerAlign)。通过少数用户样本,推断其沟通策略、个性和写作风格,并将这些假设与用户的特定属性一起输入LLM模型来生成定制的输出。
- 实验表明该方法在个人化任务(如作者归属和审慎对齐)上的表现优于基于偏好的微调方法。
- 在审慎对齐任务中,该方法平均提高了LLM模型的实用性高达70%。在作者归属任务中,与最新的偏好微调方法相比,该方法在所有用户配置文件和LLM模型中均表现出较高胜率(通常超过90%)。
- 该方法提供了一个可解释性和样本效率高的策略来实现LLM模型的个人化。这意味着使用更少的数据和计算资源就可以实现模型的个性化调整。
点此查看论文截图
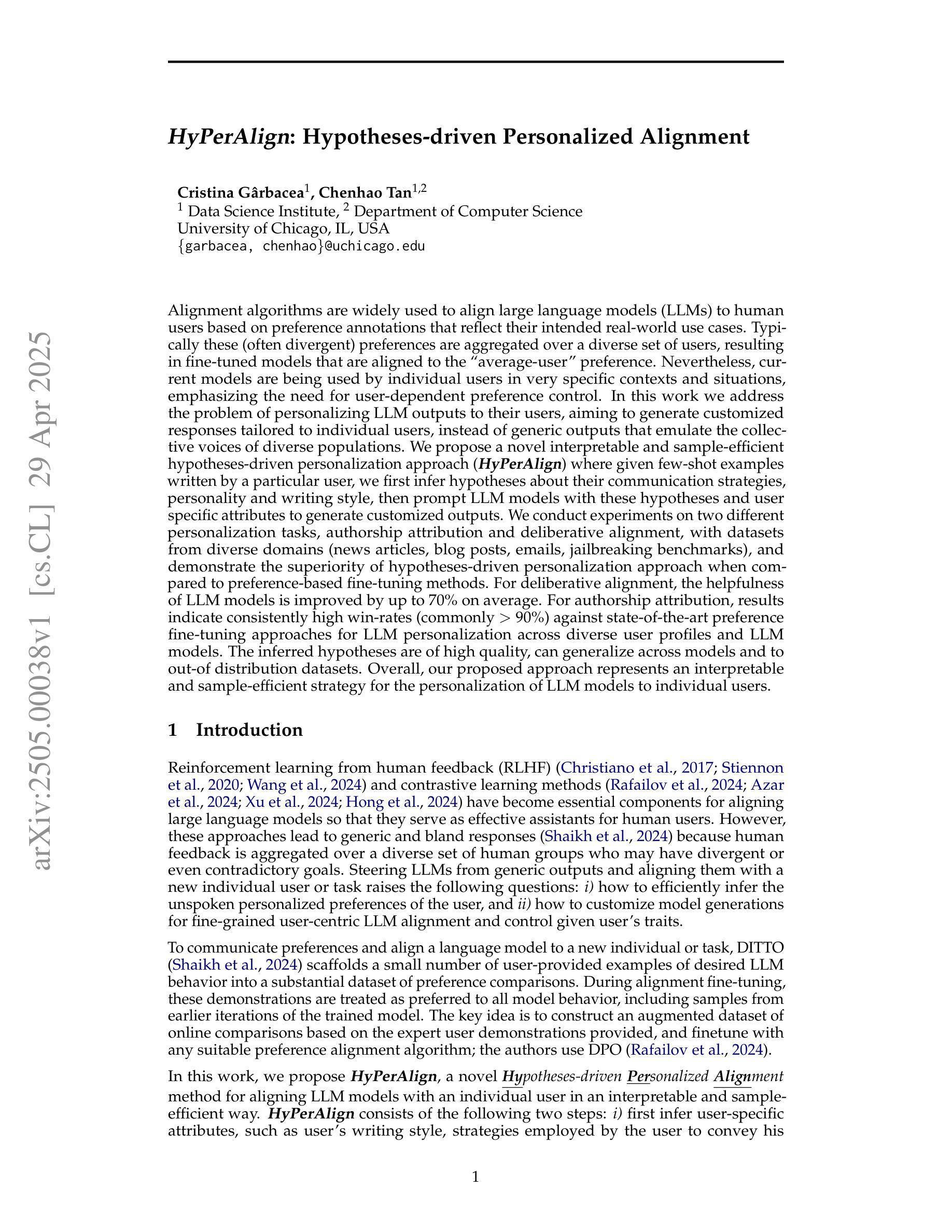
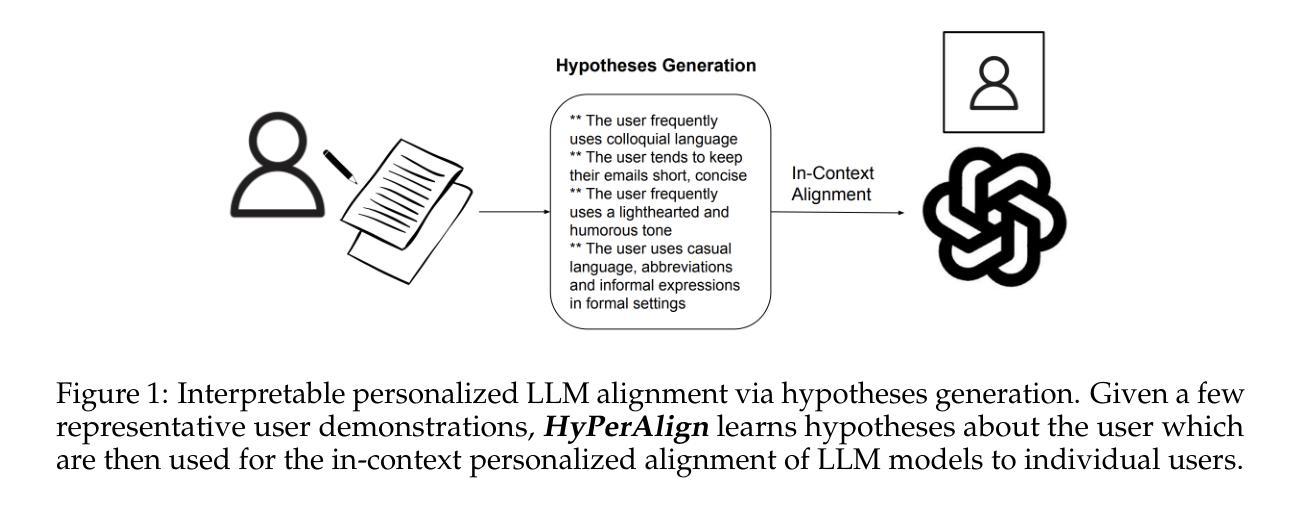
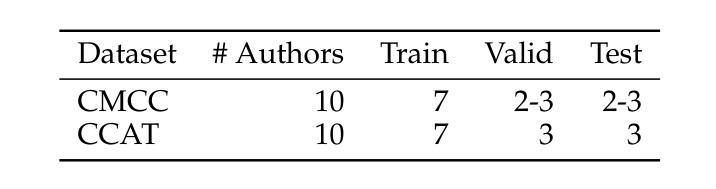