⚠️ 以下所有内容总结都来自于 大语言模型的能力,如有错误,仅供参考,谨慎使用
🔴 请注意:千万不要用于严肃的学术场景,只能用于论文阅读前的初筛!
💗 如果您觉得我们的项目对您有帮助 ChatPaperFree ,还请您给我们一些鼓励!⭐️ HuggingFace免费体验
2025-05-03 更新
Cues3D: Unleashing the Power of Sole NeRF for Consistent and Unique Instances in Open-Vocabulary 3D Panoptic Segmentation
Authors:Feng Xue, Wenzhuang Xu, Guofeng Zhong, Anlong Minga, Nicu Sebe
Open-vocabulary 3D panoptic segmentation has recently emerged as a significant trend. Top-performing methods currently integrate 2D segmentation with geometry-aware 3D primitives. However, the advantage would be lost without high-fidelity 3D point clouds, such as methods based on Neural Radiance Field (NeRF). These methods are limited by the insufficient capacity to maintain consistency across partial observations. To address this, recent works have utilized contrastive loss or cross-view association pre-processing for view consensus. In contrast to them, we present Cues3D, a compact approach that relies solely on NeRF instead of pre-associations. The core idea is that NeRF’s implicit 3D field inherently establishes a globally consistent geometry, enabling effective object distinction without explicit cross-view supervision. We propose a three-phase training framework for NeRF, initialization-disambiguation-refinement, whereby the instance IDs are corrected using the initially-learned knowledge. Additionally, an instance disambiguation method is proposed to match NeRF-rendered 3D masks and ensure globally unique 3D instance identities. With the aid of Cues3D, we obtain highly consistent and unique 3D instance ID for each object across views with a balanced version of NeRF. Our experiments are conducted on ScanNet v2, ScanNet200, ScanNet++, and Replica datasets for 3D instance, panoptic, and semantic segmentation tasks. Cues3D outperforms other 2D image-based methods and competes with the latest 2D-3D merging based methods, while even surpassing them when using additional 3D point clouds. The code link could be found in the appendix and will be released on \href{https://github.com/mRobotit/Cues3D}{github}
开放词汇表的3D全景分割最近成为了一个重要的趋势。目前表现最佳的方法将2D分割与具有几何意识的3D基本体相结合。然而,如果没有高保真3D点云,优势将会丧失,例如基于神经辐射场(NeRF)的方法。这些方法受限于在部分观察结果之间保持一致性的能力不足。为了解决这一问题,近期的研究工作采用了对比损失或跨视图关联预处理来实现视图共识。与之相反,我们提出了Cues3D,这是一种仅依赖NeRF而不依赖预先关联的紧凑方法。核心思想是NeRF的隐式3D场内在地建立了全局一致的几何结构,从而能够在没有明确的跨视图监督的情况下实现有效的对象区分。我们为NeRF提出了一个三阶段训练框架,即初始化-去模糊-细化,通过利用初始学习到的知识来纠正实例ID。此外,还提出了一种实例去模糊方法来匹配NeRF呈现的3D掩膜,并确保全局唯一的3D实例身份。借助Cues3D,我们获得了每个对象在视图之间的高度一致和唯一的3D实例ID,同时采用了平衡的NeRF版本。我们的实验是在ScanNet v2、ScanNet200、ScanNet++和Replica数据集上针对3D实例、全景和语义分割任务进行的。Cues3D在基于其他二维图像的方法中表现优异,并且在与最新的二维至三维合并方法相竞争的同时甚至还能在附加的3D点云的情况下超越它们。代码链接可在附录中找到,并且将在\href{https://github.com/mRobotit/Cues3D}{github}上发布。
论文及项目相关链接
PDF Accepted by Information Fusion
摘要
基于NeRF的开放词汇3D全景分割方法突破现有技术限制,结合二维分割与三维几何感知元素。本文提出Cues3D方法,仅靠NeRF建模而不依赖预先关联技术即可实现不同视角下的全局一致几何特性及物体辨别能力。采用三阶段NeRF训练框架,即初始化-消歧-细化过程,利用初始学习到的知识修正实例ID。提出实例消歧方法,确保NeRF渲染的三维掩膜匹配并获取全局唯一的三维实例ID。在ScanNet v2、ScanNet200、ScanNet++和Replica数据集上进行的实验表明,Cues3D在三维实例、全景和语义分割任务上优于其他基于二维图像的方法,与最新的二维至三维融合方法竞争并在使用额外三维点云数据时更胜一筹。代码详见附录并将在github上发布。
关键见解
- Cues3D是一种基于NeRF的开放词汇3D全景分割方法,无需高保真三维点云即可实现全局一致的几何特性。
- Cues3D采用三阶段NeRF训练框架,通过初始化、消歧和细化过程修正实例ID,确保在不同视角下的有效物体识别。
- Cues3D提出一种实例消歧方法,匹配NeRF渲染的三维掩膜并确保全局唯一的三维实例ID。
- Cues3D在多个数据集上的实验结果表明,其在三维实例、全景和语义分割任务上表现优异。
- Cues3D优于其他基于二维图像的方法,并与最新的二维至三维融合方法竞争。
- Cues3D可在使用额外三维点云数据时表现更优秀。
点此查看论文截图
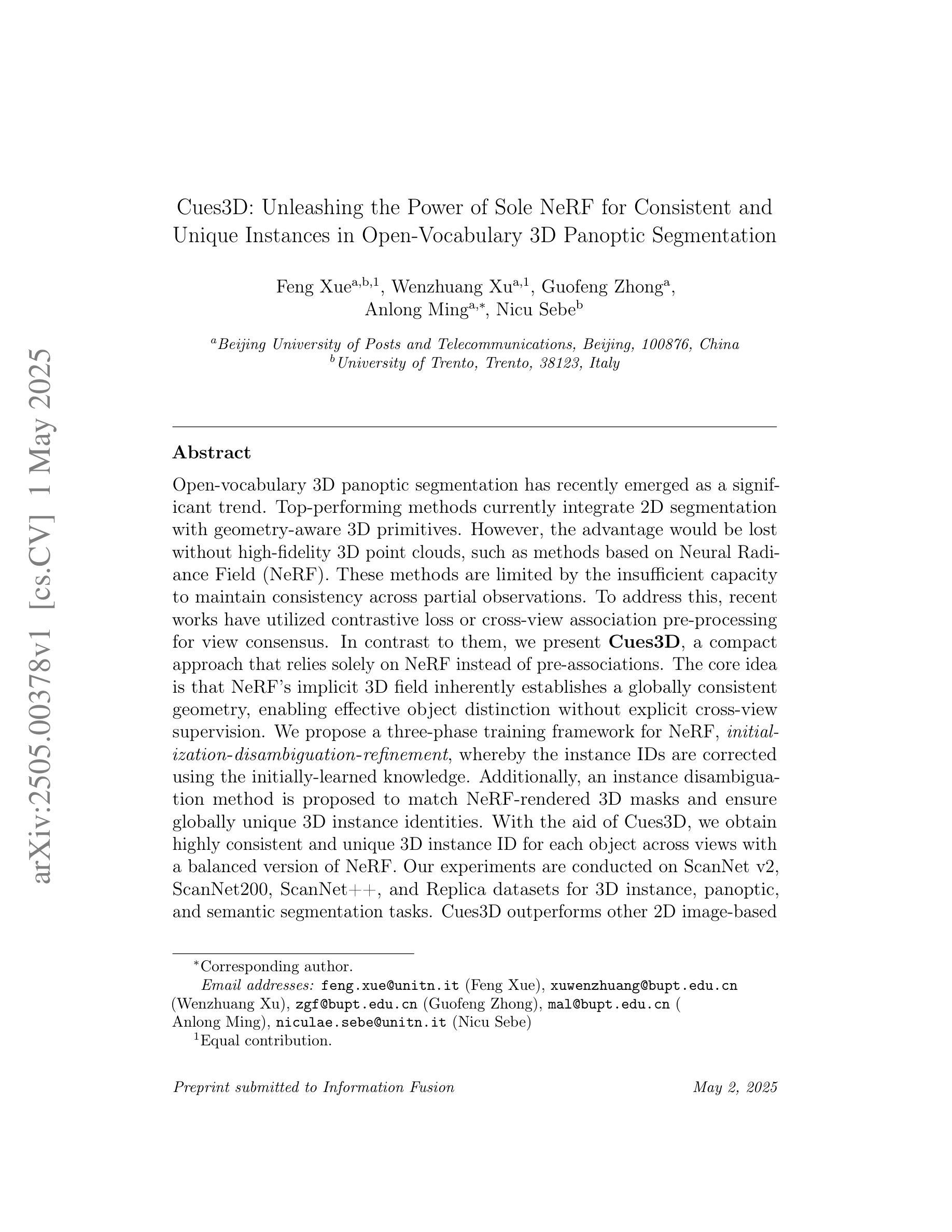
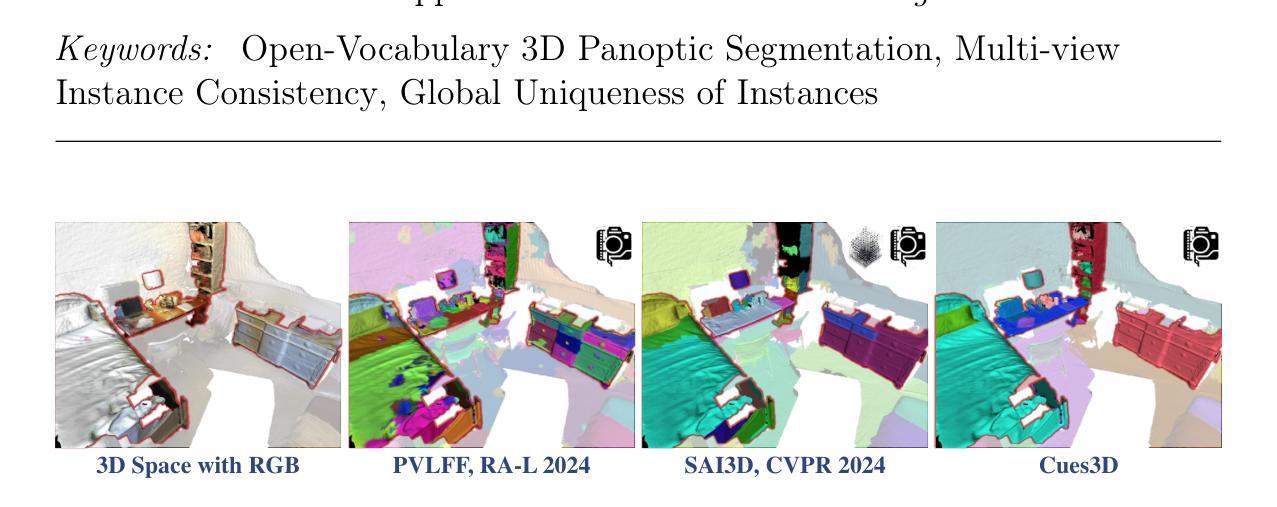
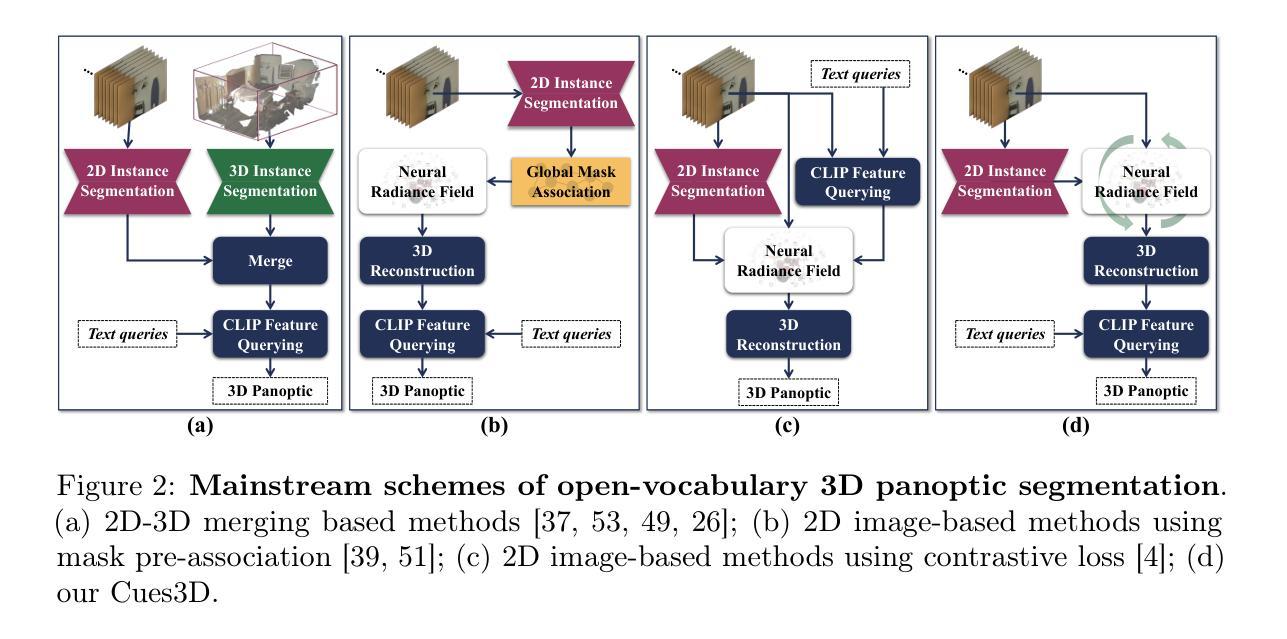