⚠️ 以下所有内容总结都来自于 大语言模型的能力,如有错误,仅供参考,谨慎使用
🔴 请注意:千万不要用于严肃的学术场景,只能用于论文阅读前的初筛!
💗 如果您觉得我们的项目对您有帮助 ChatPaperFree ,还请您给我们一些鼓励!⭐️ HuggingFace免费体验
2025-05-06 更新
Monitoring morphometric drift in lifelong learning segmentation of the spinal cord
Authors:Enamundram Naga Karthik, Sandrine Bédard, Jan Valošek, Christoph S. Aigner, Elise Bannier, Josef Bednařík, Virginie Callot, Anna Combes, Armin Curt, Gergely David, Falk Eippert, Lynn Farner, Michael G Fehlings, Patrick Freund, Tobias Granberg, Cristina Granziera, RHSCIR Network Imaging Group, Ulrike Horn, Tomáš Horák, Suzanne Humphreys, Markus Hupp, Anne Kerbrat, Nawal Kinany, Shannon Kolind, Petr Kudlička, Anna Lebret, Lisa Eunyoung Lee, Caterina Mainero, Allan R. Martin, Megan McGrath, Govind Nair, Kristin P. O’Grady, Jiwon Oh, Russell Ouellette, Nikolai Pfender, Dario Pfyffer, Pierre-François Pradat, Alexandre Prat, Emanuele Pravatà, Daniel S. Reich, Ilaria Ricchi, Naama Rotem-Kohavi, Simon Schading-Sassenhausen, Maryam Seif, Andrew Smith, Seth A Smith, Grace Sweeney, Roger Tam, Anthony Traboulsee, Constantina Andrada Treaba, Charidimos Tsagkas, Zachary Vavasour, Dimitri Van De Ville, Kenneth Arnold Weber II, Sarath Chandar, Julien Cohen-Adad
Morphometric measures derived from spinal cord segmentations can serve as diagnostic and prognostic biomarkers in neurological diseases and injuries affecting the spinal cord. While robust, automatic segmentation methods to a wide variety of contrasts and pathologies have been developed over the past few years, whether their predictions are stable as the model is updated using new datasets has not been assessed. This is particularly important for deriving normative values from healthy participants. In this study, we present a spinal cord segmentation model trained on a multisite $(n=75)$ dataset, including 9 different MRI contrasts and several spinal cord pathologies. We also introduce a lifelong learning framework to automatically monitor the morphometric drift as the model is updated using additional datasets. The framework is triggered by an automatic GitHub Actions workflow every time a new model is created, recording the morphometric values derived from the model’s predictions over time. As a real-world application of the proposed framework, we employed the spinal cord segmentation model to update a recently-introduced normative database of healthy participants containing commonly used measures of spinal cord morphometry. Results showed that: (i) our model outperforms previous versions and pathology-specific models on challenging lumbar spinal cord cases, achieving an average Dice score of $0.95 \pm 0.03$; (ii) the automatic workflow for monitoring morphometric drift provides a quick feedback loop for developing future segmentation models; and (iii) the scaling factor required to update the database of morphometric measures is nearly constant among slices across the given vertebral levels, showing minimum drift between the current and previous versions of the model monitored by the framework. The model is freely available in Spinal Cord Toolbox v7.0.
从脊髓分割得到的形态学测量值可以作为影响脊髓的神经系统疾病和损伤的诊断和预后生物标志物。虽然过去几年已经开发出了针对各种对比度和病理的稳健、自动的分割方法,但尚未评估随着新数据集的使用模型更新时,其预测的稳定性。这对于从健康参与者中得出规范值尤为重要。在这项研究中,我们展示了一个在多点(n=75)数据集上训练的脊髓分割模型,包括9种不同的MRI对比度和几种脊髓病理。我们还介绍了一个终身学习框架,以自动监控随着模型使用附加数据集进行更新时的形态学漂移。每当创建新模型时,该框架都会由GitHub Actions工作流自动触发,记录随时间推移从模型预测中得出的形态测量值。作为所提出的框架的现实应用,我们采用脊髓分割模型来更新最近引入的健康参与者规范数据库,其中包含常用的脊髓形态测量指标。结果显示:(i)我们的模型在具有挑战性的腰椎脊髓病例上的表现优于以前版本和特定病理模型,平均Dice得分为$0.95 \pm 0.03$;(ii)用于监控形态学漂移的自动工作流程为开发未来的分割模型提供了快速反馈循环;(iii)用于更新数据库形态测量指标的缩放因子在给定的椎体水平之间几乎恒定,显示出当前版本和由框架监控的先前版本之间的最小漂移。该模型可在Spinal Cord Toolbox v7.0中免费使用。
论文及项目相关链接
摘要
脊髓分割的形态学测量值可作为神经系统疾病和脊髓损伤的诊断和预后生物标志物。尽管过去几年已经开发出了针对各种对比度和病理的稳健的自动分割方法,但关于使用新数据集更新模型时其预测的稳定性尚未进行评估。这对于从健康参与者中得出规范值尤为重要。本研究中,我们展示了一个在多个站点(n=75)的数据集上训练的脊髓分割模型,包括9种不同的MRI对比度和多种脊髓病理。我们还引入了一种终身学习框架,以自动监视模型使用附加数据集进行更新时的形态学漂移。该框架每次创建新模型时都会由GitHub Actions工作流程自动触发,记录模型预测得出的形态学值随时间的变化。作为所提出框架的实际应用,我们采用了脊髓分割模型来更新最近引入的健康参与者的规范数据库,该数据库包含常用的脊髓形态学测量值。结果表明:(i)我们的模型在具有挑战性的腰椎脊髓病例上的表现优于以前版本和特定病理模型,平均Dice得分为0.95±0.03;(ii)用于监视形态学漂移的自动工作流程为开发未来的分割模型提供了快速反馈循环;(iii)用于更新数据库的形态学测量值的缩放因子在给定的椎体水平之间几乎恒定,显示出模型和当前版本之间最小的漂移。该模型可在Spinal Cord Toolbox v7.0中免费获得。
要点
- 脊髓分割的形态学测量值在神经系统疾病和脊髓损伤中具有诊断和预后的生物标志物价值。
- 研究提出了一种在多站点数据集上训练的脊髓分割模型,表现优异,特别是在具有挑战性的病例上。
- 引入了一种终身学习框架,用于自动监视模型更新时的形态学漂移。
- 框架通过自动工作流程实现快速反馈,有助于未来分割模型的开发。
- 更新数据库所需的缩放因子在椎体水平间近乎恒定,显示模型稳定性。
- 模型具备高度的实用价值,可在Spinal Cord Toolbox v7.0中免费获取。
点此查看论文截图
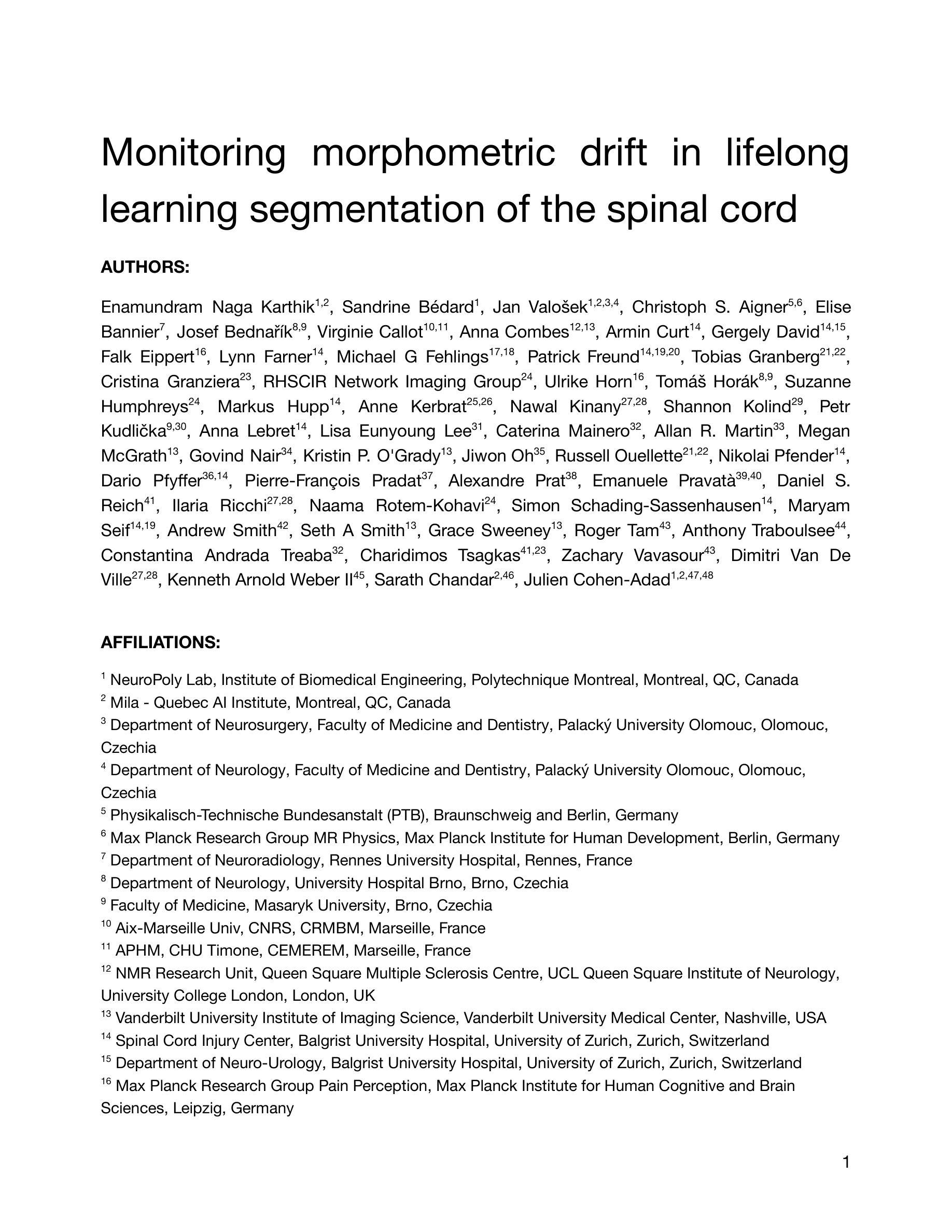
Core-Set Selection for Data-efficient Land Cover Segmentation
Authors:Keiller Nogueira, Akram Zaytar, Wanli Ma, Ribana Roscher, Ronny Hänsch, Caleb Robinson, Anthony Ortiz, Simone Nsutezo, Rahul Dodhia, Juan M. Lavista Ferres, Oktay Karakuş, Paul L. Rosin
The increasing accessibility of remotely sensed data and the potential of such data to inform large-scale decision-making has driven the development of deep learning models for many Earth Observation tasks. Traditionally, such models must be trained on large datasets. However, the common assumption that broadly larger datasets lead to better outcomes tends to overlook the complexities of the data distribution, the potential for introducing biases and noise, and the computational resources required for processing and storing vast datasets. Therefore, effective solutions should consider both the quantity and quality of data. In this paper, we propose six novel core-set selection methods for selecting important subsets of samples from remote sensing image segmentation datasets that rely on imagery only, labels only, and a combination of each. We benchmark these approaches against a random-selection baseline on three commonly used land cover classification datasets: DFC2022, Vaihingen, and Potsdam. In each of the datasets, we demonstrate that training on a subset of samples outperforms the random baseline, and some approaches outperform training on all available data. This result shows the importance and potential of data-centric learning for the remote sensing domain. The code is available at https://github.com/keillernogueira/data-centric-rs-classification/.
遥感数据的日益普及及其为大规模决策提供信息的潜力,推动了深度学习模型在地球观测任务中的发展。传统上,此类模型必须在大型数据集上进行训练。然而,普遍认为更大的数据集会带来更好的结果往往忽略了数据分布的复杂性、引入偏见和噪声的可能性以及处理存储大量数据所需的计算资源。因此,有效的解决方案应同时考虑数据的质量和数量。在本文中,我们提出了六种新颖的核集选择方法,用于从遥感图像分割数据集中选择重要的样本子集,这些选择方法依赖于仅使用图像、仅使用标签以及两者的组合。我们在三个常用的土地覆盖分类数据集:DFC2022、瓦伊欣根和波茨坦上,以随机选择基线为基准对这些方法进行了评估。在每个数据集中,我们都证明了在样本子集上进行训练优于随机基线,有些方法的性能优于在所有可用数据上进行训练。这一结果展示了以数据为中心的学习在遥感领域的重要性和潜力。代码可在https://github.com/keillernogueira/data-centric-rs-classification/找到。
论文及项目相关链接
Summary
遥感数据的日益可获取性及其在大规模决策中的潜力推动了深度学习模型在地球观测任务中的应用发展。传统上,此类模型需要在大数据集上进行训练。然而,普遍认为更大的数据集会带来更好的结果,这往往忽视了数据分布的复杂性、引入偏见和噪声的潜力以及处理存储大量数据所需的计算资源。因此,有效的解决方案应同时考虑数据数量和质量。本文提出了六种新型核心集选择方法,可从遥感图像分割数据集中选择重要样本子集,这些方法依赖于仅使用图像、仅使用标签以及两者的组合。我们在三个常用的土地覆盖分类数据集上对这些方法进行了与随机选择基准的基准测试:DFC2022、瓦伊宁根和波茨坦。在每个数据集中,我们证明了在样本子集上进行训练优于随机基线,某些方法的表现优于在所有可用数据上的训练。这一结果展示了数据密集型学习在遥感领域的重要性和潜力。
Key Takeaways
- 遥感数据的可获取性增加,驱动了深度学习模型在地球观测任务中的应用。
- 传统上,遥感相关深度学习模型需要在大数据集上进行训练。
- 单纯追求大数据可能忽视数据分布的复杂性、引入偏见和噪声,以及计算资源需求。
- 本文提出了六种核心集选择方法,用于从遥感图像分割数据中选取重要样本子集。
- 在三个土地覆盖分类数据集上的实验表明,在样本子集上训练模型表现优于随机选择,部分方法表现优于全数据训练。
- 结果强调了数据密集型学习在遥感领域的重要性和潜力。
点此查看论文截图
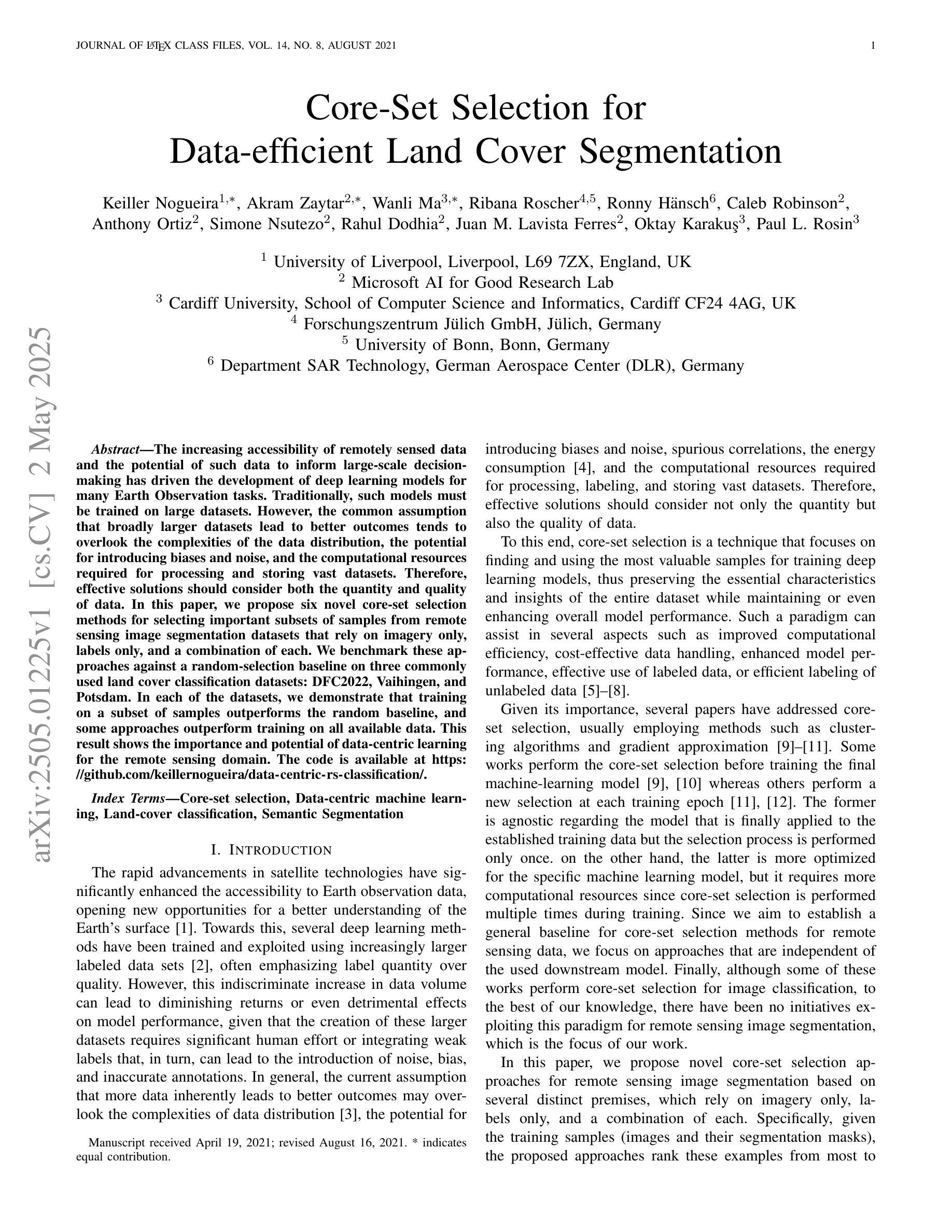
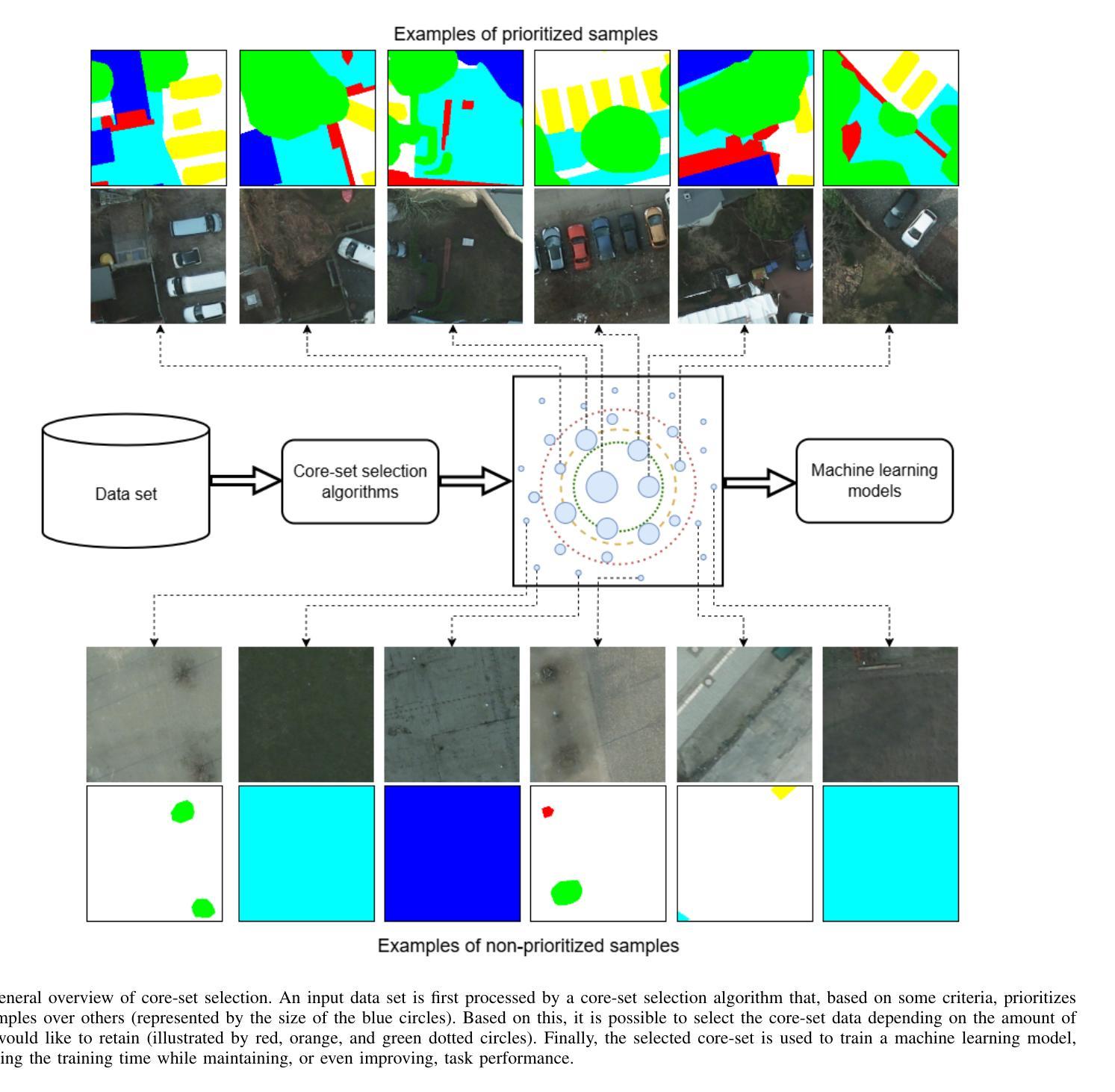
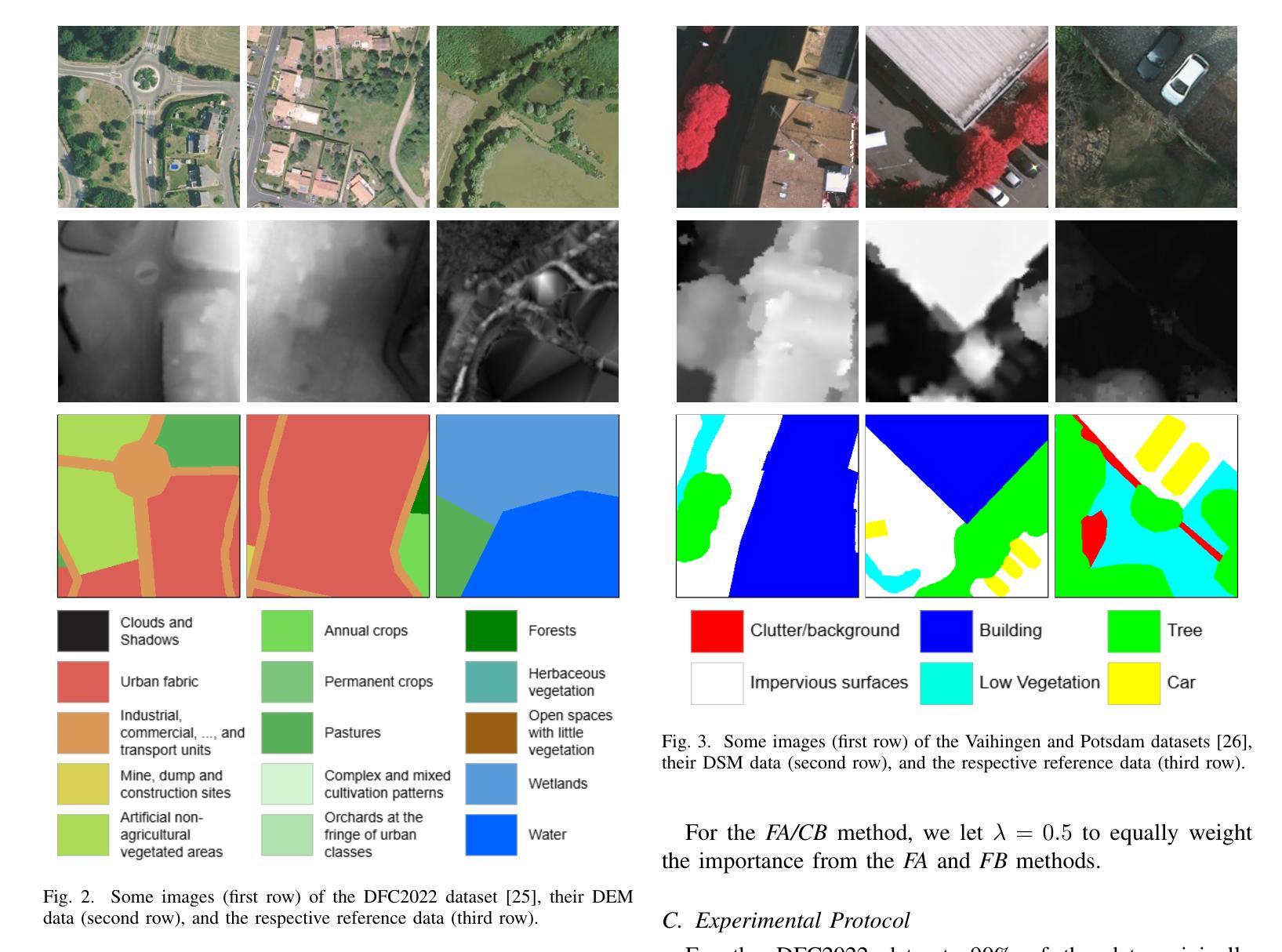
GeloVec: Higher Dimensional Geometric Smoothing for Coherent Visual Feature Extraction in Image Segmentation
Authors:Boris Kriuk, Matey Yordanov
This paper introduces GeloVec, a new CNN-based attention smoothing framework for semantic segmentation that addresses critical limitations in conventional approaches. While existing attention-backed segmentation methods suffer from boundary instability and contextual discontinuities during feature mapping, our framework implements a higher-dimensional geometric smoothing method to establish a robust manifold relationships between visually coherent regions. GeloVec combines modified Chebyshev distance metrics with multispatial transformations to enhance segmentation accuracy through stabilized feature extraction. The core innovation lies in the adaptive sampling weights system that calculates geometric distances in n-dimensional feature space, achieving superior edge preservation while maintaining intra-class homogeneity. The multispatial transformation matrix incorporates tensorial projections with orthogonal basis vectors, creating more discriminative feature representations without sacrificing computational efficiency. Experimental validation across multiple benchmark datasets demonstrates significant improvements in segmentation performance, with mean Intersection over Union (mIoU) gains of 2.1%, 2.7%, and 2.4% on Caltech Birds-200, LSDSC, and FSSD datasets respectively compared to state-of-the-art methods. GeloVec’s mathematical foundation in Riemannian geometry provides theoretical guarantees on segmentation stability. Importantly, our framework maintains computational efficiency through parallelized implementation of geodesic transformations and exhibits strong generalization capabilities across disciplines due to the absence of information loss during transformations.
本文介绍了GeloVec,这是一种基于CNN的新型注意力平滑框架,用于语义分割,解决了传统方法的关键局限性。虽然现有的基于注意力的分割方法受到特征映射期间边界不稳定和上下文不连续的影响,但我们的框架实现了高维几何平滑方法,以在视觉上连贯的区域之间建立稳健的流形关系。GeloVec结合了修改后的切比雪夫距离度量与多空间变换,通过稳定的特征提取来提高分割精度。核心创新之处在于自适应采样权重系统,该系统在n维特征空间中计算几何距离,在保持类内同质性的同时实现优越的边缘保留。多空间变换矩阵将张量投影与正交基向量相结合,创建更具辨别力的特征表示,而不牺牲计算效率。在多个基准数据集上的实验验证表明,与最新方法相比,分割性能得到了显着提高,在Caltech Birds-200、LSDSC和FSSD数据集上的平均交并比(mIoU)分别提高了2.1%、2.7%和2.4%。GeloVec在黎曼几何中的数学基础为分割稳定性提供了理论保证。重要的是,我们的框架通过并行实施测地线变换而保持计算效率,并由于在变换过程中没有信息损失而表现出跨学科的强大泛化能力。
论文及项目相关链接
PDF 13 pages, 3 figures, 3 tables
Summary
本文提出一种基于CNN的新框架GeloVec,用于语义分割的注意力平滑。该框架解决了传统方法中存在的边界不稳定和特征映射中的上下文不连续问题。通过高维几何平滑方法建立视觉连贯区域之间的稳健流形关系,并结合修改后的切比雪夫距离度量与多空间变换,提高分割精度。核心创新在于自适应采样权重系统,在n维特征空间中计算几何距离,实现边缘保护的同时保持类内同质性。多空间变换矩阵结合张量投影和正交基向量,创建更具判别性的特征表示,且不失计算效率。在多个基准数据集上的实验验证显示,与最新方法相比,分割性能有显著改善,在Caltech Birds-200、LSDSC和FSSD数据集上的平均交并比(mIoU)分别提高了2.1%、2.7%和2.4%。GeloVec的黎曼几何数学基础为分割稳定性提供理论保证。
Key Takeaways
- GeloVec是一个新的CNN框架,用于语义分割的注意力平滑。
- 解决了传统方法中的边界不稳定和上下文不连续问题。
- 通过高维几何平滑方法建立视觉连贯区域之间的稳健流形关系。
- 结合修改后的切比雪夫距离度量与多空间变换,提高分割精度。
- 自适应采样权重系统在n维特征空间中计算几何距离,实现边缘保护并维持类内同质性。
- 多空间变换矩阵提高特征表示的判别性,同时保持计算效率。
- 在多个数据集上的实验验证显示GeloVec的分割性能显著优于其他方法。
点此查看论文截图
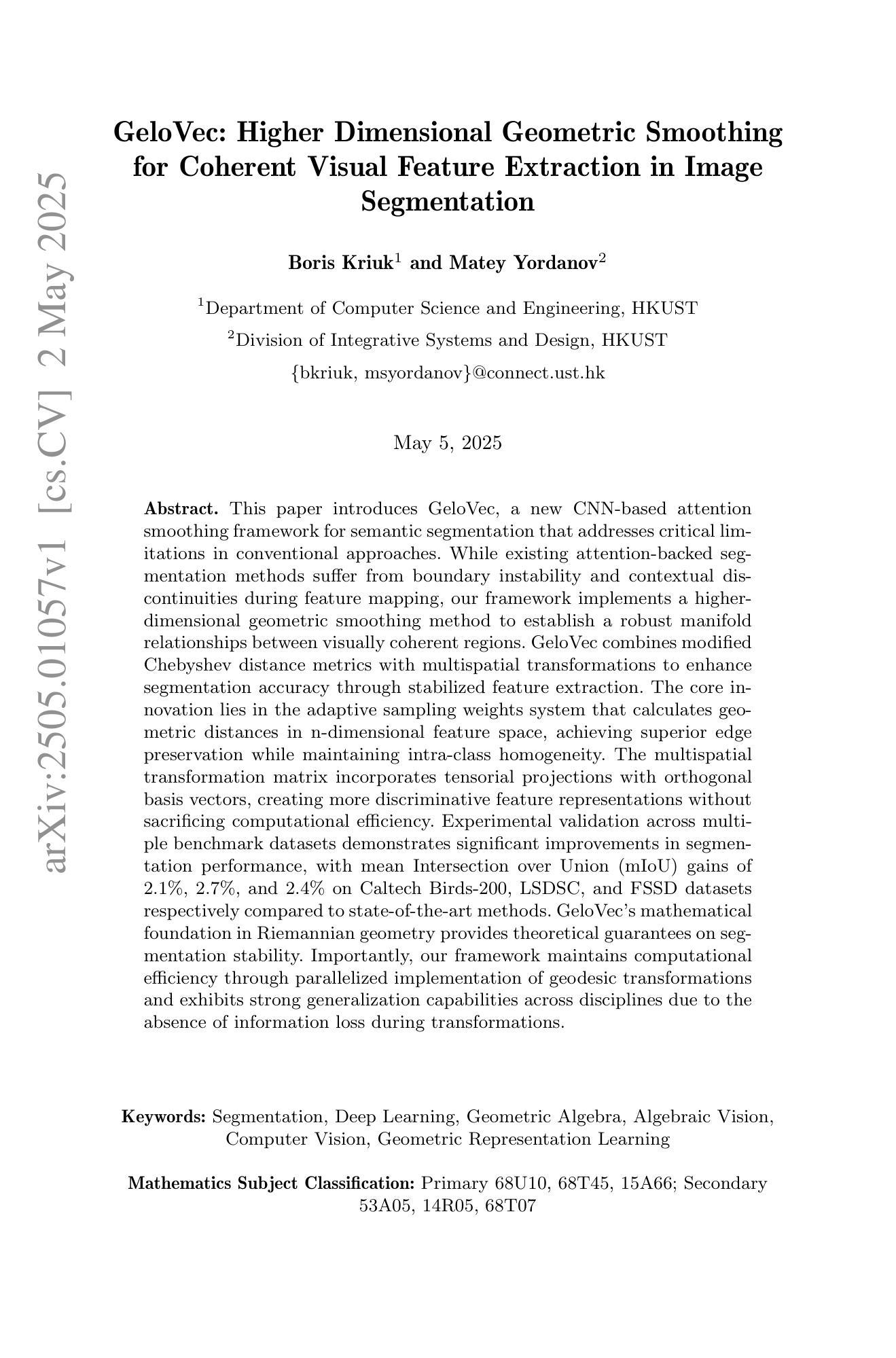
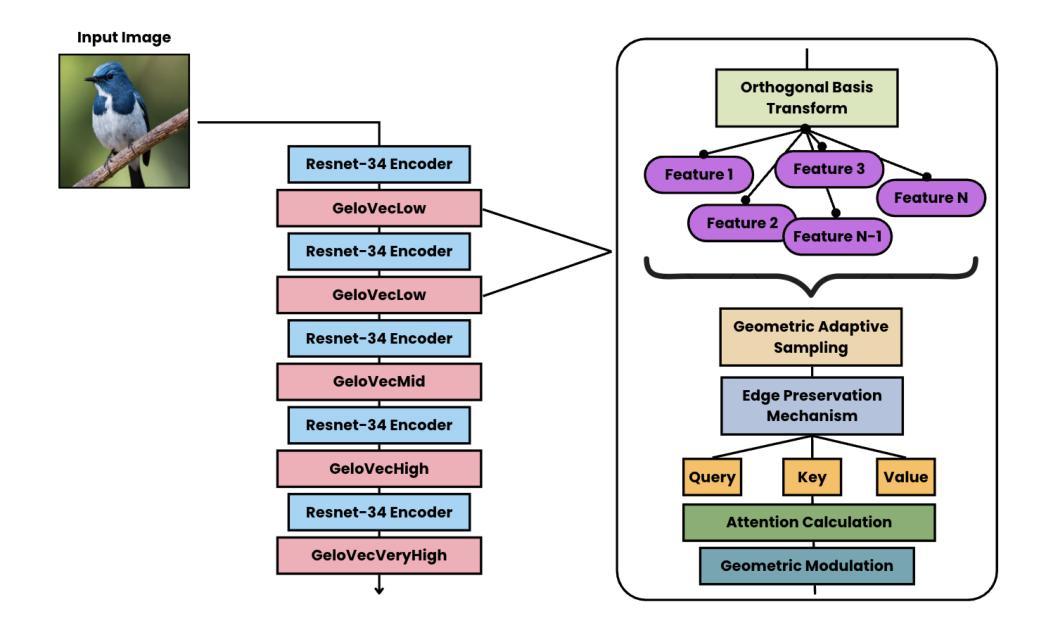
On recovering intragranular strain fields from grain-averaged strains obtained by high-energy X-ray diffraction microscopy
Authors:C. K. Cocke, A. Akerson, S. F. Gorske, K. T. Faber, K. Bhattacharya
We address an unusual problem in the theory of elasticity motivated by the problem of reconstructing the strain field from partial information obtained using X-ray diffraction. Referred to as either high-energy X-ray diffraction microscopy(HEDM) or three-dimensional X-ray diffraction microscopy(3DXRD), these methods provide diffraction images that, once processed, commonly yield detailed grain structure of polycrystalline materials, as well as grain-averaged elastic strains. However, it is desirable to have the entire (point-wise) strain field. So we address the question of recovering the entire strain field from grain-averaged values in an elastic polycrystalline material. The key idea is that grain-averaged strains must be the result of a solution to the equations of elasticity and the overall imposed loads. In this light, the recovery problem becomes the following: find the boundary traction distribution that induces the measured grain-averaged strains under the equations of elasticity. We show that there are either zero or infinite solutions to this problem, and more specifically, that there exist an infinite number of kernel fields, or non-trivial solutions to the equations of elasticity that have zero overall boundary loads and zero grain-averaged strains. We define a best-approximate reconstruction to address this non-uniqueness. We then show that, consistent with Saint-Venant’s principle, in experimentally relevant cylindrical specimens, the uncertainty due to non-uniqueness in recovered strain fields decays exponentially with distance from the ends of the interrogated volume. Thus, one can obtain useful information despite the non-uniqueness. We apply these results to a numerical example and experimental observations on a transparent aluminum oxynitride (AlON) sample.
我们解决弹性理论中一个特殊问题,其动机来源于从使用X射线衍射获得的部分信息重建应变场的问题。这些方法被称为高能X射线衍射显微镜(HEDM)或三维X射线衍射显微镜(3DXRD),它们提供的衍射图像经过处理后通常会产生多晶材料的详细晶粒结构以及晶粒平均弹性应变。然而,拥有整个(点对点)应变场是必要的。因此,我们解决从弹性多晶材料的晶粒平均值恢复整个应变场的问题。关键的想法是,晶粒平均应变必须是弹性方程和总体施加负载的解的结果。在这种情况下,恢复问题变成以下形式:找到边界牵引分布,在弹性方程下,它引起测量的晶粒平均应变。我们表明这个问题存在零个或无限个解,更具体地说,存在无数个核场,或者是弹性方程的平凡解,其具有零总体边界载荷和零晶粒平均应变。为了解决这种唯一性问题,我们定义了一个最佳近似重建。然后我们表明,与圣维南原理一致,在实验中相关的圆柱形样本中,由于恢复的应变场的唯一性不确定性随着距离所询问体积的端部而呈指数衰减。因此,尽管存在唯一性问题,人们仍然可以获得有用的信息。我们将这些结果应用于透明铝氧氮化物(AlON)样本的数值例子和实验观察结果上。
论文及项目相关链接
Summary
本文解决弹性理论中一个由高能量X射线衍射显微镜(HEDM)或三维X射线衍射显微镜(3DXRD)获得部分信息重建应变场的问题。文章探讨了从多晶材料的平均应变值恢复整个应变场的难题,并提出一种最佳近似重建方法来解决这一非唯一性问题。结果显示,非唯一性带来的不确定性会随着与探测体积边界的距离的增加而指数衰减。最后将理论应用于数值示例和透明铝氧氮化物(AlON)样品的实验观察。
Key Takeaways
- 文章探讨了从高能量X射线衍射显微镜(HEDM)或三维X射线衍射显微镜(3DXRD)获得的平均应变值恢复整个应变场的问题。
- 文章指出从多晶材料的平均应变值恢复整个应变场需要解决的是寻找边界牵引分布的问题,该分布需要在弹性方程下产生测量的平均应变。
- 文章表明该问题存在零解或无限解,且存在无穷多的核场或非平凡解,这些解满足弹性方程且总体边界载荷和平均应变均为零。
- 为解决这种非唯一性问题,文章提出了一种最佳近似重建方法。
- 根据圣维南原理,非唯一性带来的不确定性会随着与探测体积边界的距离的增加而指数衰减。
- 文章通过数值示例展示了理论的应用。
点此查看论文截图
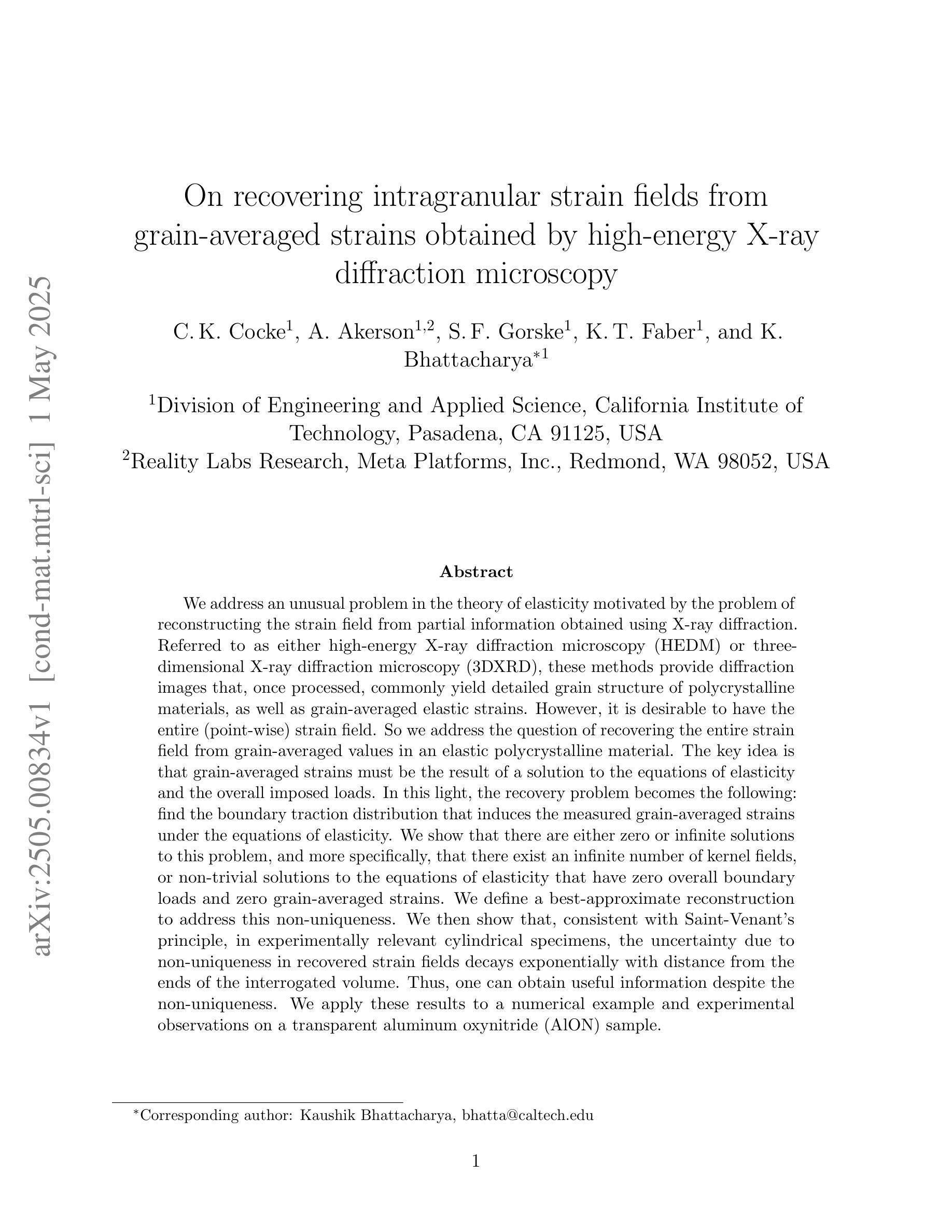
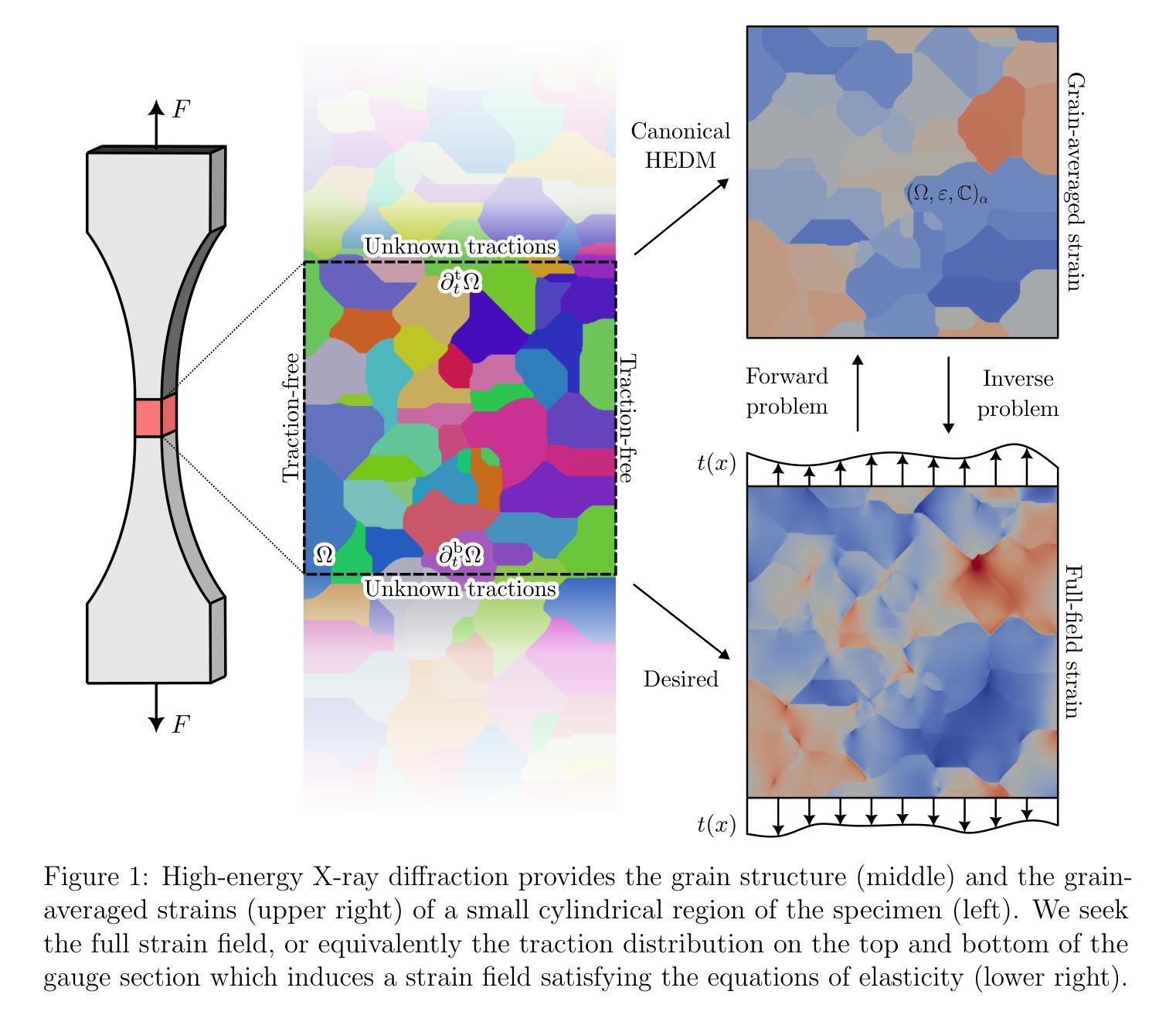
Multimodal Masked Autoencoder Pre-training for 3D MRI-Based Brain Tumor Analysis with Missing Modalities
Authors:Lucas Robinet, Ahmad Berjaoui, Elizabeth Cohen-Jonathan Moyal
Multimodal magnetic resonance imaging (MRI) constitutes the first line of investigation for clinicians in the care of brain tumors, providing crucial insights for surgery planning, treatment monitoring, and biomarker identification. Pre-training on large datasets have been shown to help models learn transferable representations and adapt with minimal labeled data. This behavior is especially valuable in medical imaging, where annotations are often scarce. However, applying this paradigm to multimodal medical data introduces a challenge: most existing approaches assume that all imaging modalities are available during both pre-training and fine-tuning. In practice, missing modalities often occur due to acquisition issues, specialist unavailability, or specific experimental designs on small in-house datasets. Consequently, a common approach involves training a separate model for each desired modality combination, making the process both resource-intensive and impractical for clinical use. Therefore, we introduce BM-MAE, a masked image modeling pre-training strategy tailored for multimodal MRI data. The same pre-trained model seamlessly adapts to any combination of available modalities, extracting rich representations that capture both intra- and inter-modal information. This allows fine-tuning on any subset of modalities without requiring architectural changes, while still benefiting from a model pre-trained on the full set of modalities. Extensive experiments show that the proposed pre-training strategy outperforms or remains competitive with baselines that require separate pre-training for each modality subset, while substantially surpassing training from scratch on several downstream tasks. Additionally, it can quickly and efficiently reconstruct missing modalities, highlighting its practical value. Code and trained models are available at: https://github.com/Lucas-rbnt/BM-MAE
多模态磁共振成像(MRI)是临床医生在脑肿瘤治疗中首选的调查手段,为手术规划、治疗监测和生物标志物识别提供关键见解。在大规模数据集上进行预训练已被证明有助于模型学习可迁移的表示,并可在最小标注数据的情况下进行适应。这种行为在医疗成像中尤其有价值,因为注解通常很稀缺。然而,将这种范式应用于多模态医疗数据带来了一项挑战:大多数现有方法假设在预训练和微调期间都可获得所有成像模式。实际上,由于采集问题、专家不可用或在内部数据集上的特定实验设计,经常会出现缺失的模式。因此,一种常见的方法是为每种所需的模式组合训练一个单独的模型,这不仅资源密集,而且在实际临床使用中不切实际。因此,我们引入了BM-MAE,这是一种针对多模态MRI数据定制的掩模图像建模预训练策略。同一预训练模型无缝适应任何可用的模式组合,提取丰富的表示,这些表示捕获了模式和跨模式的信息。这使得可以在任何模式的子集上进行微调,而无需进行架构更改,同时仍然受益于在完整模式集上进行预训练的模型。大量实验表明,所提出的预训练策略优于或具有竞争力基线,基线需要对每个模式子集进行单独的预训练,同时在几个下游任务上大大超越从头开始训练。此外,它可以快速有效地重建缺失的模式,突显其实用价值。代码和已训练的模型可在[https://github.com/Lucas-rbnt/BM-MAE找到。](注:此处应为原文中的链接地址)
论文及项目相关链接
Summary
多模态磁共振成像(MRI)是临床医生在脑肿瘤诊治中的首选检查手段,可为手术规划、治疗监测和生物标志物识别提供关键信息。预训练大型数据集有助于模型学习可迁移表示,并在医疗影像中,在标注数据稀缺的情况下快速适应。然而,当将这一方法应用于多模态医疗数据时,面临的问题是现有方法大多假设预训练和微调阶段都有所有成像模式的数据可用。实际应用中,由于采集问题、专家不可用或特定内部数据集的实验设计等原因,缺失模式经常发生。因此,我们引入了BM-MAE,一种针对多模态MRI数据的掩膜图像建模预训练策略。同一预训练模型可灵活适应任何可用的模式组合,提取丰富的表示,这些表示可以捕获模式和跨模式的信息。在多个下游任务上,所提出的预训练策略表现出超越或竞争基线的能力,其中基线需要对每个模式子集进行单独的预训练。此外,它还可以快速有效地重建缺失的模式,凸显其实用价值。
Key Takeaways
- 多模态MRI在脑肿瘤诊治中扮演重要角色,为手术规划等提供关键信息。
- 预训练大型数据集有助于模型适应医疗影像领域,尤其在不缺乏标注数据的情况下。
- 将预训练应用于多模态医疗数据面临挑战,因为经常缺失某些成像模式。
- 现有方法通常需要针对每种期望的模式组合训练单独模型,造成资源浪费且不适用于临床实践。
- BM-MAE是一种针对多模态MRI数据的预训练策略,能够适应任何可用的模式组合,提取丰富的信息表示。
- BM-MAE在多个下游任务上表现出超越或竞争基线的能力,并能有效重建缺失的模式。
点此查看论文截图
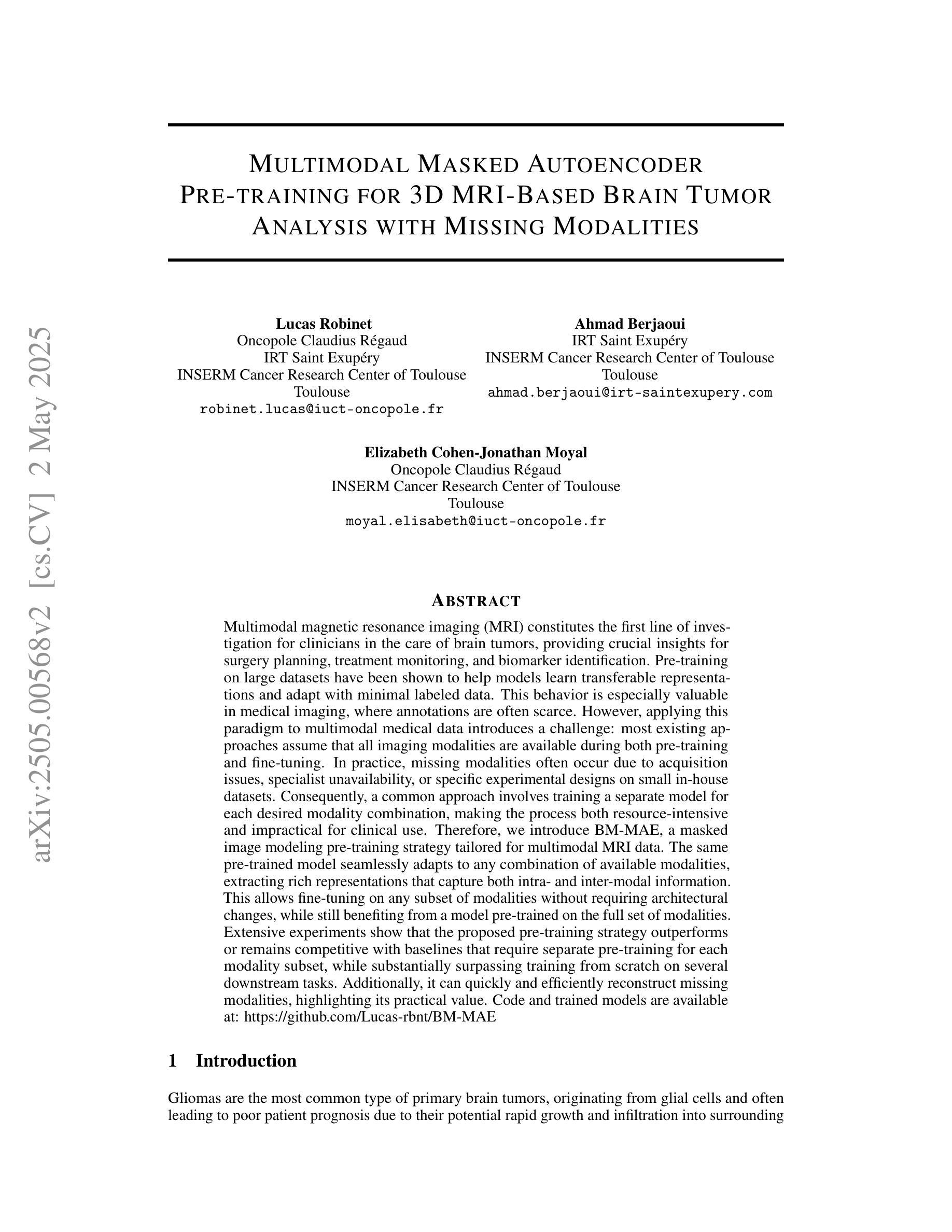
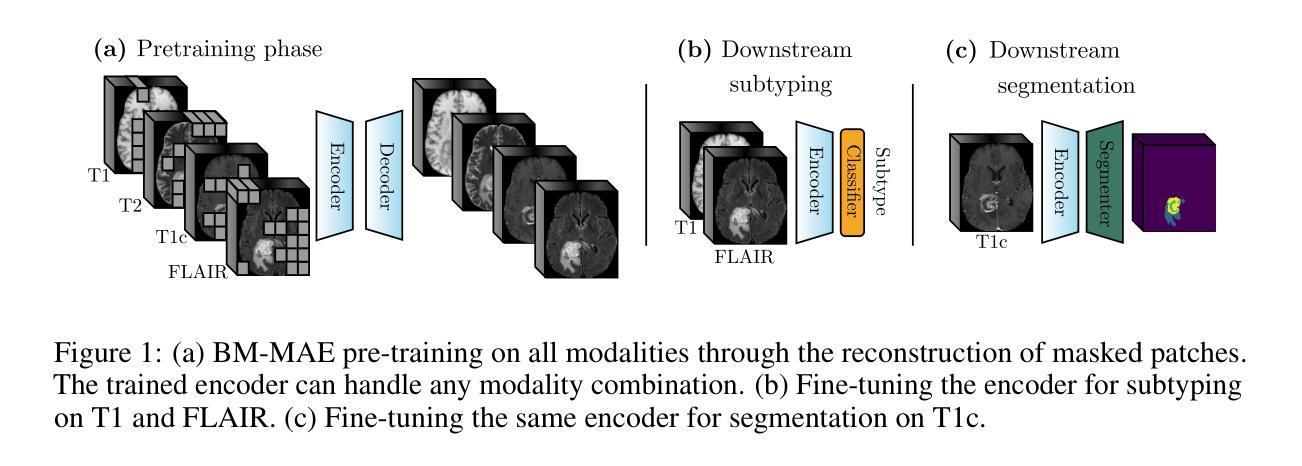
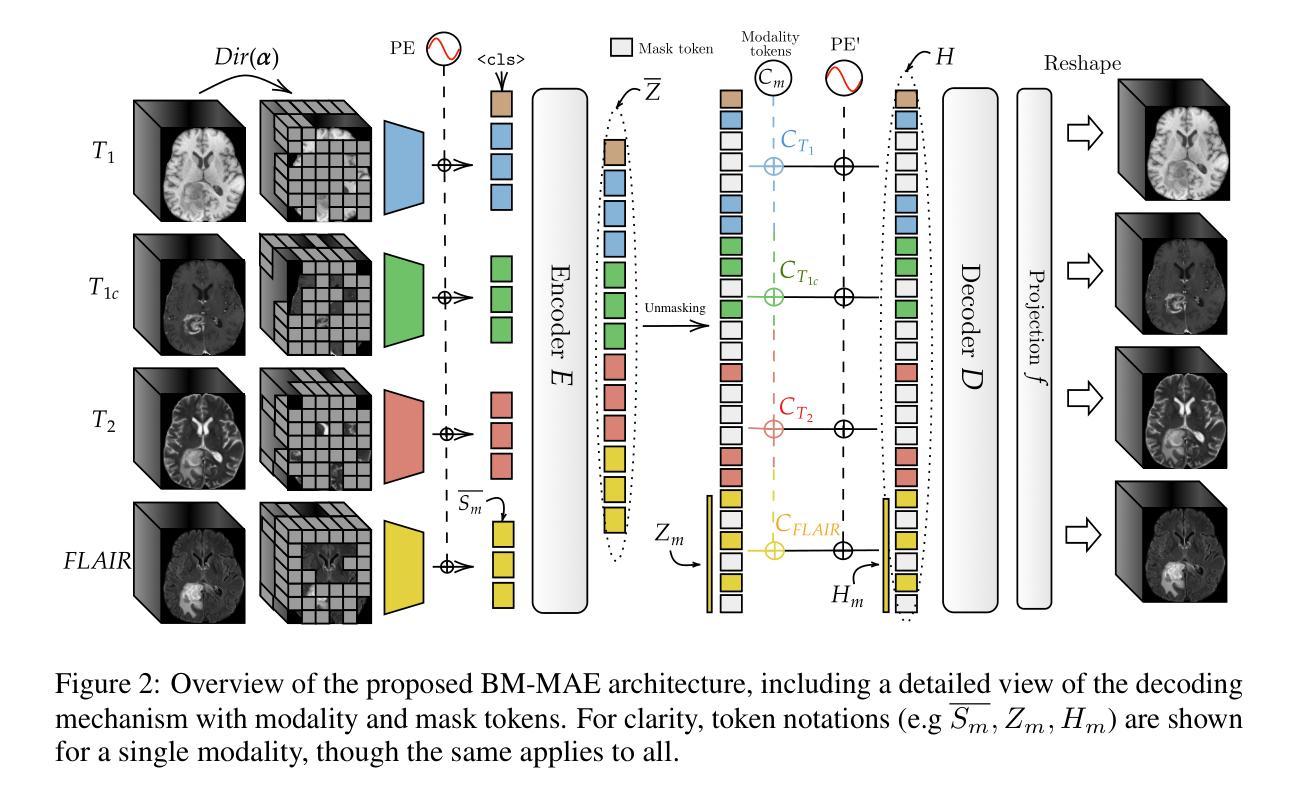
Unveiling Fine Structure and Energy-driven Transition of Photoelectron Kikuchi Diffraction
Authors:Trung-Phuc Vo, Olena Tkach, Aki Pulkkinen, Didier Sebilleau, Aimo Winkelmann, Olena Fedchenko, Yaryna Lytvynenko, Dmitry Vasilyev, Hans-Joachim Elmers, Gerd Schonhense, Jan Minar
The intricate fine structure of Kikuchi diffraction plays a vital role in probing phase transformations and strain distributions in functional materials, particularly in electron microscopy. Beyond these applications, it also proves essential in photoemission spectroscopy (PES) at high photon energies, aiding in the disentanglement of complex angle-resolved PES data and enabling emitter-site-specific studies. However, the detection and analysis of these rich faint structures in photoelectron diffraction (PED), especially in the hard X-ray regime, remain highly challenging, with only a limited number of simulations successfully reproducing these patterns. The strong energy dependence of Kikuchi patterns further complicates their interpretation, necessitating advanced theoretical approaches. To enhance structural analysis, we present a comprehensive theoretical study of fine diffraction patterns and their evolution with energy by simulating core-level emissions from Ge(100) and Si(100). Using multiple-scattering theory and the fully relativistic one-step photoemission model, we simulate faint pattern networks for various core levels across different kinetic energies (106 eV - 4174 eV), avoiding cluster size convergence issues inherent in cluster-based methods. Broadening in patterns is discussed via the inelastic scattering treatment. For the first time, circular dichroism has been observed and successfully reproduced in the angular distribution of Si (100) 1s, revealing detailed features and asymmetries up to 31%. Notably, we successfully replicate experimental bulk and more “surface-sensitivity” diffraction features, further validating the robustness of our simulations. The results show remarkable agreement with the experimental data obtained using circularly polarized radiations, demonstrating the potential of this methodology for advancing high-energy PES investigations.
Kikuchi衍射的精细复杂结构在探测功能材料的相变和应变分布方面起着至关重要的作用,特别是在电子显微镜学中。除了这些应用之外,它在高能光子能量的光发射光谱(PES)中也证明是不可或缺的,有助于解开复杂的角度解析PES数据,并能进行发射器位点的专项研究。然而,在光电子衍射(PED)中检测和解析这些丰富的微弱结构,特别是在硬X射线领域,仍然是一项巨大的挑战,只有少数模拟成功再现了这些图案。Kikuchi图案的强烈能量依赖性进一步使它们的解释复杂化,需要高级理论方法。为了增强结构分析,我们对精细衍射图案及其随能量的演变进行了全面的理论研究,通过对Ge(100)和Si(100)的核心能级发射进行模拟。我们利用多重散射理论和完全相对论的一步光发射模型,模拟了不同动能范围(106电子伏特-4174电子伏特)内各种能级的微弱图案网络,避免了集群大小收敛问题。通过非弹性散射处理讨论了图案的展宽。首次观察到圆二色性并在Si(100)1s的角度分布中成功复制,揭示了高达31%的详细特征和不对称性。值得注意的是,我们成功地复制了实验中的大体和更“表面敏感”的衍射特征,进一步验证了模拟的稳健性。该结果与使用圆偏振辐射获得的实验数据显示出显著的一致性,证明了这种方法在高能PES研究中应用的潜力。
论文及项目相关链接
摘要
本研究探讨了Kikuchi衍射精细结构在功能材料相变和应变分布探测中的重要作用,特别是在电子显微镜中的应用。此外,它还在高能光子光谱中的光发射光谱(PES)中发挥着关键作用,有助于解开复杂的角度解析PES数据,并支持进行发射器位点的特定研究。然而,在光电子衍射(PED)中检测和解析这些丰富的微弱结构仍然极具挑战性,特别是在硬X射线领域,只有少数模拟成功复制了这些模式。本研究对Kikuchi模式的能量依赖性进行了深入的理论研究,模拟了Ge(100)和Si(100)的核心水平发射的精细衍射模式及其随能量的演变。通过多重散射理论和完全相对论的一步光发射模型,我们模拟了不同核心层次在不同动能(106 eV - 4174 eV)下的微弱模式网络,解决了集群大小收敛问题。通过非弹性散射处理讨论了图案的扩展。首次观察到并成功模拟了Si(100)1s的圆二色性在角度分布中的特征,揭示了高达31%的详细特征和不对称性。我们的模拟成功复制了实验中的大体和更“表面敏感性”的衍射特征,验证了模拟的稳健性。该结果与使用圆偏振辐射获得的实验数据显示出良好一致性,表明该方法在高能PES研究中的潜力。
关键见解
- Kikuchi衍射的精细结构在功能材料的相变和应变分布探测中起关键作用,特别是在电子显微镜应用中。
- 在高能光子光谱中的光发射光谱(PES)中,Kikuchi衍射有助于解开复杂的角度解析数据。
- 检测和解析光电子衍射(PED)中的微弱结构,特别是在硬X射线领域,仍然极具挑战。
- 通过多重散射理论和完全相对论的一步光发射模型,成功模拟了Kikuchi衍射的精细模式及其随能量的演变。
- 首次成功模拟并观察到Si(100)1s的圆二色性特征。
- 模拟结果成功复制了实验中的大体和表面敏感性衍射特征,验证了模拟的稳健性。
点此查看论文截图
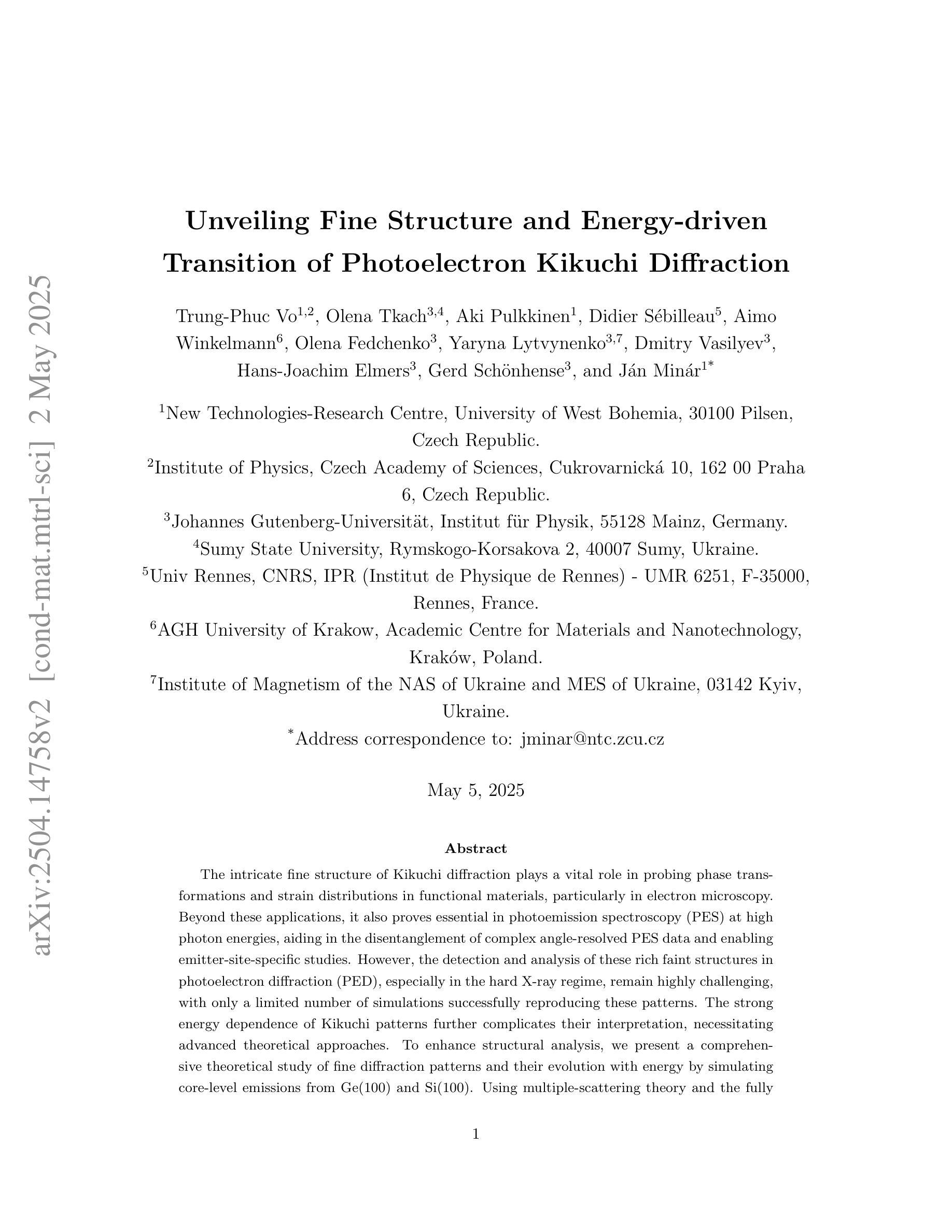
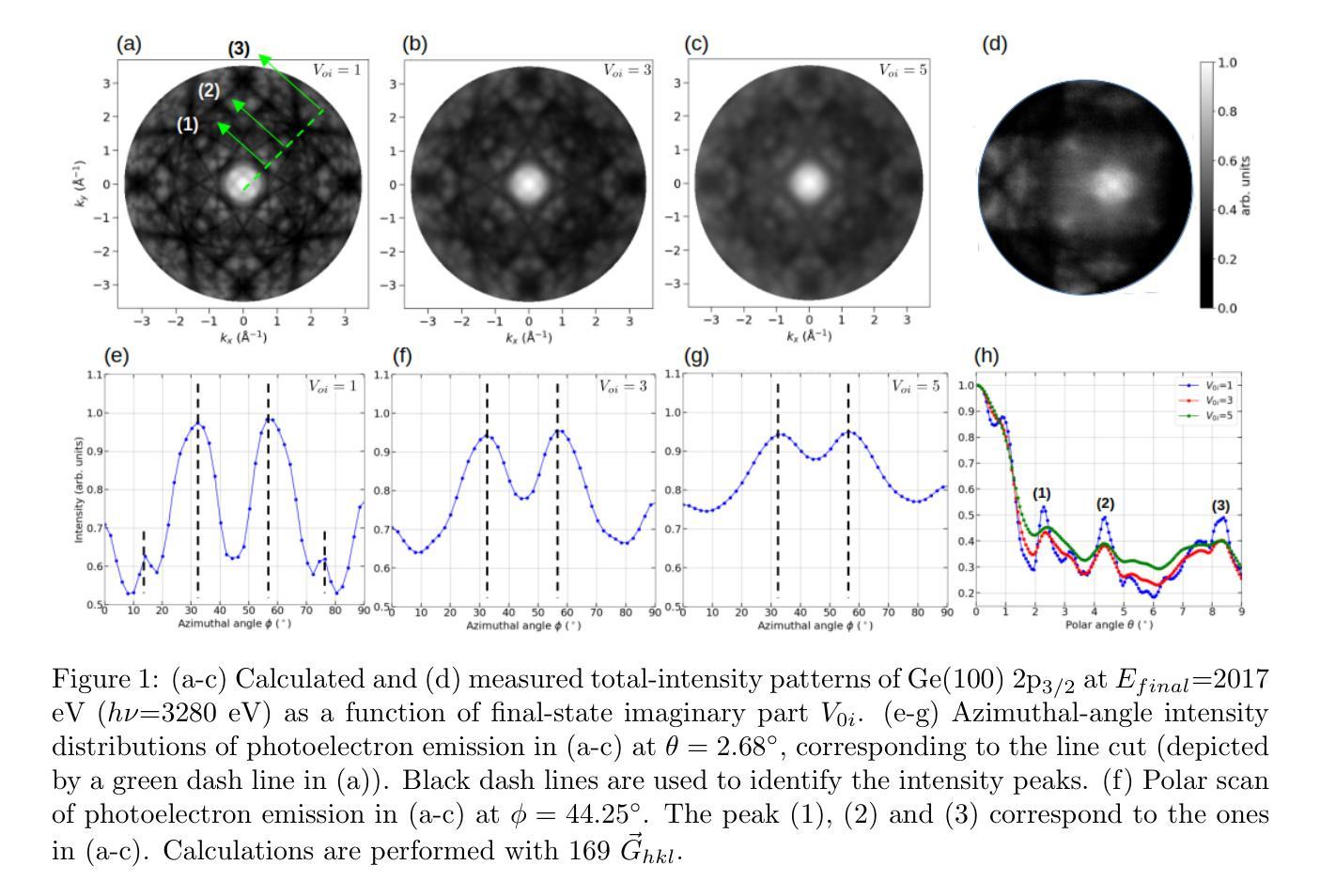
Soybean Disease Detection via Interpretable Hybrid CNN-GNN: Integrating MobileNetV2 and GraphSAGE with Cross-Modal Attention
Authors:Md Abrar Jahin, Soudeep Shahriar, M. F. Mridha, Md. Jakir Hossen, Nilanjan Dey
Soybean leaf disease detection is critical for agricultural productivity but faces challenges due to visually similar symptoms and limited interpretability in conventional methods. While Convolutional Neural Networks (CNNs) excel in spatial feature extraction, they often neglect inter-image relational dependencies, leading to misclassifications. This paper proposes an interpretable hybrid Sequential CNN-Graph Neural Network (GNN) framework that synergizes MobileNetV2 for localized feature extraction and GraphSAGE for relational modeling. The framework constructs a graph where nodes represent leaf images, with edges defined by cosine similarity-based adjacency matrices and adaptive neighborhood sampling. This design captures fine-grained lesion features and global symptom patterns, addressing inter-class similarity challenges. Cross-modal interpretability is achieved via Grad-CAM and Eigen-CAM visualizations, generating heatmaps to highlight disease-influential regions. Evaluated on a dataset of ten soybean leaf diseases, the model achieves $97.16%$ accuracy, surpassing standalone CNNs ($\le95.04%$) and traditional machine learning models ($\le77.05%$). Ablation studies validate the sequential architecture’s superiority over parallel or single-model configurations. With only 2.3 million parameters, the lightweight MobileNetV2-GraphSAGE combination ensures computational efficiency, enabling real-time deployment in resource-constrained environments. The proposed approach bridges the gap between accurate classification and practical applicability, offering a robust, interpretable tool for agricultural diagnostics while advancing CNN-GNN integration in plant pathology research.
大豆叶病检测对农业生产力至关重要,但由于症状视觉相似性和传统方法的有限解释性,它面临着挑战。尽管卷积神经网络(CNN)在空间特征提取方面表现出色,但它们往往忽略了图像间的关系依赖性,从而导致误分类。本文提出了一种可解释的混合序贯CNN-图神经网络(GNN)框架,该框架协同MobileNetV2进行局部特征提取和GraphSAGE进行关系建模。该框架构建了一个图,其中节点代表叶图像,边由基于余弦相似性的邻接矩阵和自适应邻域采样定义。这种设计捕捉了精细的病变特征和全局症状模式,解决了类间相似性的挑战。通过Grad-CAM和Eigen-CAM可视化实现跨模态解释性,生成热图以突出显示影响疾病的区域。在包含十种大豆叶病的数据集上进行评估,该模型达到了97.16%的准确率,超过了单独的CNN(≤95.04%)和传统机器学习模型(≤77.05%)。消融研究验证了序贯架构优于并行或单一模型配置。仅有230万参数的轻量化MobileNetV2-GraphSAGE组合确保了计算效率,可在资源受限的环境中实现实时部署。所提出的方法缩小了准确分类和实际应用之间的差距,为农业诊断提供了一个稳健、可解释的工具,同时推动了CNN-GNN在植物病理学研究中的融合。
论文及项目相关链接
摘要
本文提出一种可解释的混合Sequential CNN-Graph Neural Network(GNN)框架,用于大豆叶病检测。该框架结合MobileNetV2进行局部特征提取和GraphSAGE进行关系建模,以解决传统方法中视觉相似症状和有限解释性所带来的挑战。通过构建图像节点和基于余弦相似性的邻接矩阵以及自适应邻域采样定义的边缘,该设计能够捕捉精细的病变特征和全局症状模式。在十种大豆叶病数据集上进行评估,该模型达到97.16%的准确率,超越单独的CNN(≤95.04%)和传统机器学习模型(≤77.05%)。通过Grad-CAM和Eigen-CAM可视化实现跨模态解释性,生成热图以突出疾病影响区域。该轻型模型仅有2.3百万参数,可在资源受限环境中实时部署。所提出的方法在准确分类和实际适用之间搭建了桥梁,为农业诊断提供了稳健、可解释的工具,推动了植物病理学研究中CNN-GNN的融合。
关键见解
- 大豆叶病检测对农业生产力至关重要,面临视觉相似症状和解释性有限的挑战。
- 传统方法在大豆叶病检测中存在局限性,需要更先进的技术来提高准确率和解释性。
- 本文提出了一种混合Sequential CNN-Graph Neural Network(GNN)框架,结合了MobileNetV2和GraphSAGE的优势。
- 该框架通过构建图像节点和基于余弦相似性的邻接矩阵,能够捕捉精细的病变特征和全局症状模式。
- 模型在十种大豆叶病数据集上实现了高达97.16%的准确率,优于传统机器学习和单独使用CNN的方法。
- 通过Grad-CAM和Eigen-CAM可视化,模型提供了跨模态解释性,有助于理解模型决策过程。
- 轻型模型具有计算效率,可在资源受限环境中实时部署,为农业诊断提供了实用的工具。
点此查看论文截图
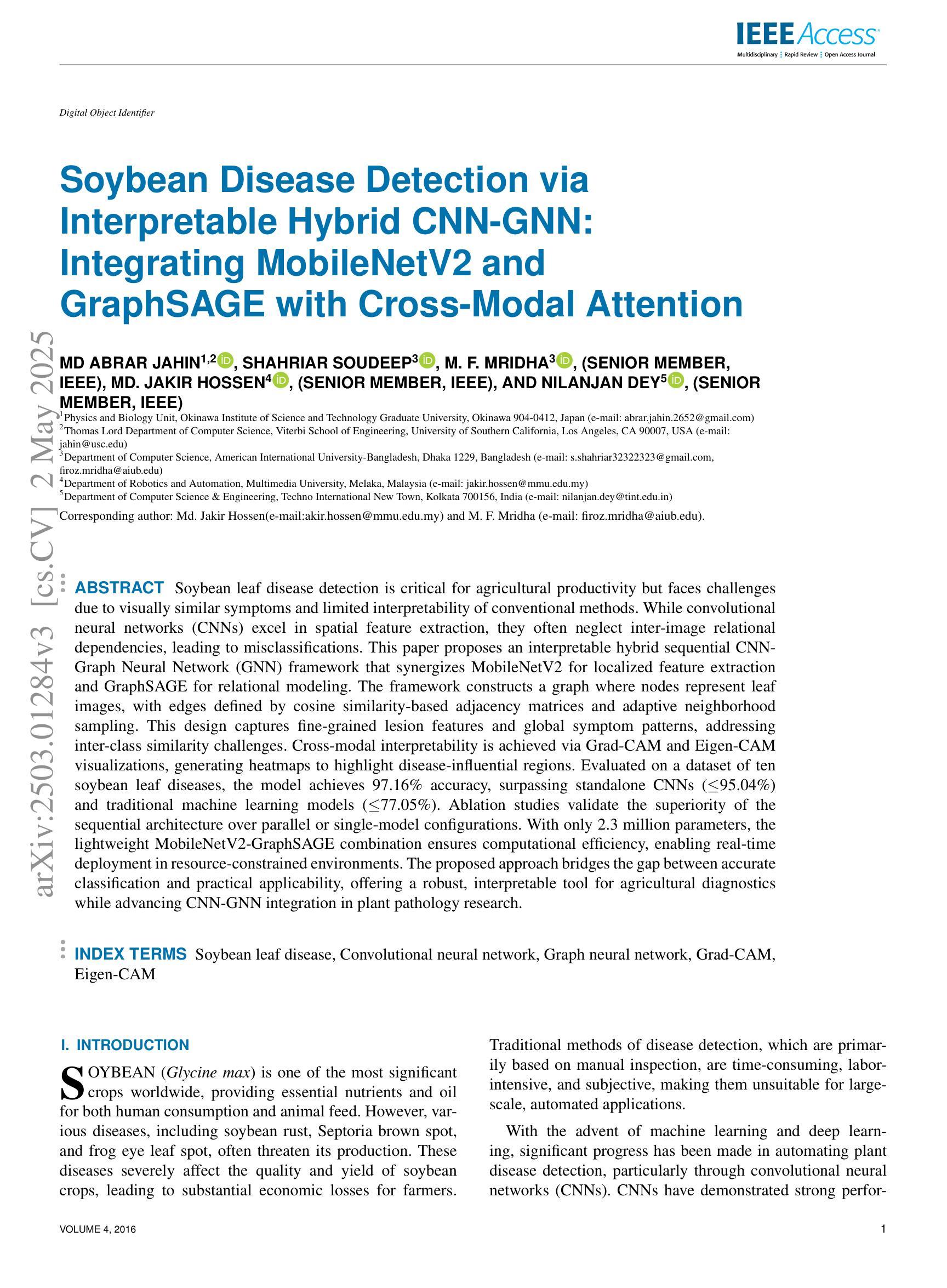
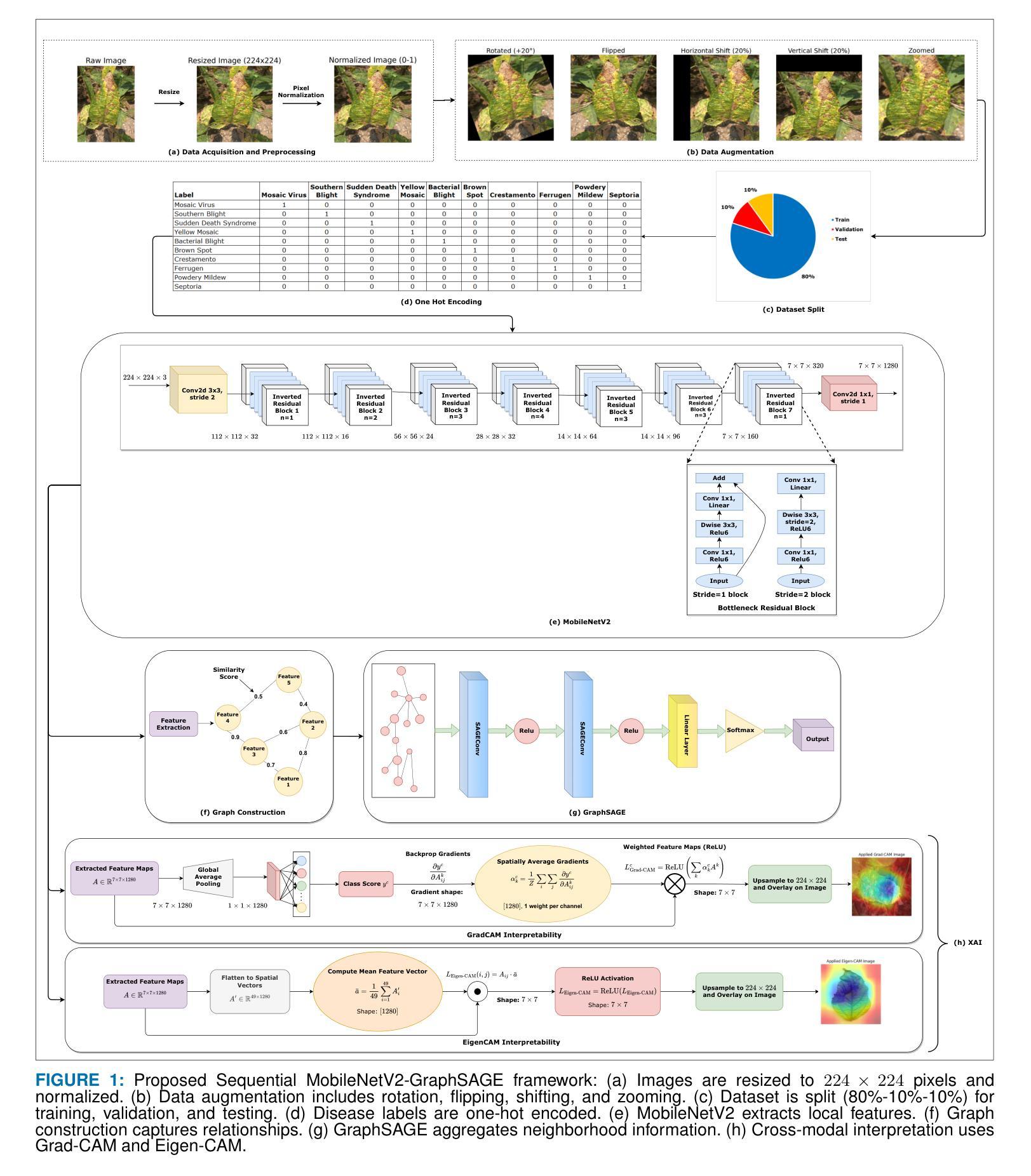
Volumetric medical image segmentation through dual self-distillation in U-shaped networks
Authors:Soumyanil Banerjee, Nicholas Summerfield, Ming Dong, Carri Glide-Hurst
U-shaped networks and its variants have demonstrated exceptional results for medical image segmentation. In this paper, we propose a novel dual self-distillation (DSD) framework in U-shaped networks for volumetric medical image segmentation. DSD distills knowledge from the ground-truth segmentation labels to the decoder layers. Additionally, DSD also distills knowledge from the deepest decoder and encoder layer to the shallower decoder and encoder layers respectively of a single U-shaped network. DSD is a general training strategy that could be attached to the backbone architecture of any U-shaped network to further improve its segmentation performance. We attached DSD on several state-of-the-art U-shaped backbones, and extensive experiments on various public 3D medical image segmentation datasets (cardiac substructure, brain tumor and Hippocampus) demonstrated significant improvement over the same backbones without DSD. On average, after attaching DSD to the U-shaped backbones, we observed an increase of 2.82%, 4.53% and 1.3% in Dice similarity score, a decrease of 7.15 mm, 6.48 mm and 0.76 mm in the Hausdorff distance, for cardiac substructure, brain tumor and Hippocampus segmentation, respectively. These improvements were achieved with negligible increase in the number of trainable parameters and training time. Our proposed DSD framework also led to significant qualitative improvements for cardiac substructure, brain tumor and Hippocampus segmentation over the U-shaped backbones. The source code is publicly available at https://github.com/soumbane/DualSelfDistillation.
U形网络及其变体在医学图像分割方面表现出卓越的效果。在本文中,我们在U形网络中提出了一种新型的双自蒸馏(DSD)框架,用于体积医学图像分割。DSD将从真实分割标签中提炼的知识传递给解码器层。此外,DSD还将从最深解码器和编码器层提炼的知识分别传递给较浅的解码器和编码器层,这些都属于单个U形网络。DSD是一种通用训练策略,可以附加到任何U形网络的主架构上,以进一步提高其分割性能。我们在几种最先进的U形主干网络上附加了DSD,并在各种公共3D医学图像分割数据集(心脏子结构、脑肿瘤和海马体)上进行了广泛实验,与没有DSD的相同主干网络相比,我们的方法取得了显著的改进。在U形主干网络上附加DSD后,我们观察到Dice相似度得分平均提高了2.82%、4.53%和1.3%,Hausdorff距离平均减少了7.15mm、6.48mm和0.76mm,分别用于心脏子结构、脑肿瘤和海马体分割。这些改进是在增加可训练参数和训练时间微乎其微的情况下实现的。我们提出的DSD框架还导致心脏子结构、脑肿瘤和海马体分割的定性显著改善,优于U形主干网络。源代码可在https://github.com/soumbane/DualSelfDistillation公开获取。
论文及项目相关链接
PDF 28 pages, 5 figures, 7 tables, accepted at IEEE Transactions on Biomedical Engineering, 2025
摘要
本文提出了一种新型的双重自蒸馏(DSD)框架,应用于U型网络及其变体,用于体积医学图像分割。DSD从地面真实分割标签中提炼知识并传递给解码器层,同时也从最深解码器和编码器层提炼知识并传递给较浅的解码器和编码器层。DSD是一种通用训练策略,可以附加到任何U型网络的骨干架构上,以进一步提高其分割性能。在多个先进的U型骨干上应用DSD,并在多个公共3D医学图像分割数据集(心脏子结构、脑肿瘤和海马体)上进行广泛实验,证明DSD可以显著提高分割性能。平均而言,在U型骨干上附加DSD后,Dice相似度得分提高了2.82%、4.53%和1.3%,Hausdorff距离分别减少了7.15mm、6.48mm和0.76mm。这些改进是在增加可训练参数和训练时间的数量微乎其微的情况下实现的。所提出的DSD框架还实现了心脏子结构、脑肿瘤和海马体分割的定性显著改善。源代码可在https://github.com/soumbane/DualSelfDistillation上公开获得。
关键见解
- 论文提出了双重自蒸馏(DSD)框架,应用于U型网络,用于医学图像分割。
- DSD利用地面真实分割标签和网络的内部层次结构进行知识提炼和传递。
- DSD可广泛应用于各种U型网络架构,可进一步提高分割性能。
- 在多个数据集上的实验表明,DSD显著提高了医学图像分割的性能指标,包括Dice相似度得分和Hausdorff距离。
- DSD改进了心脏子结构、脑肿瘤和海马体分割的视觉效果。
- DSD的附加对可训练参数和训练时间的增加微乎其微。
点此查看论文截图
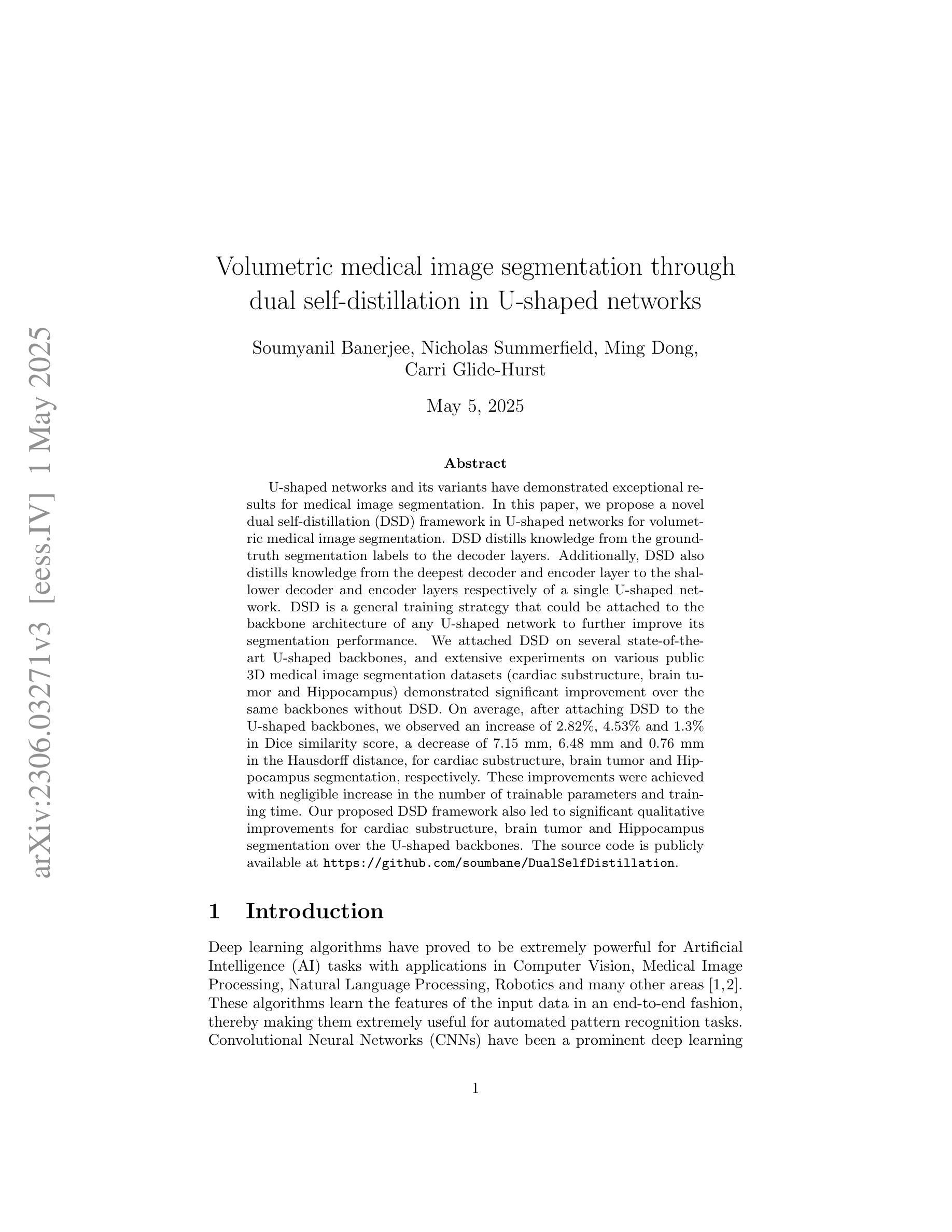
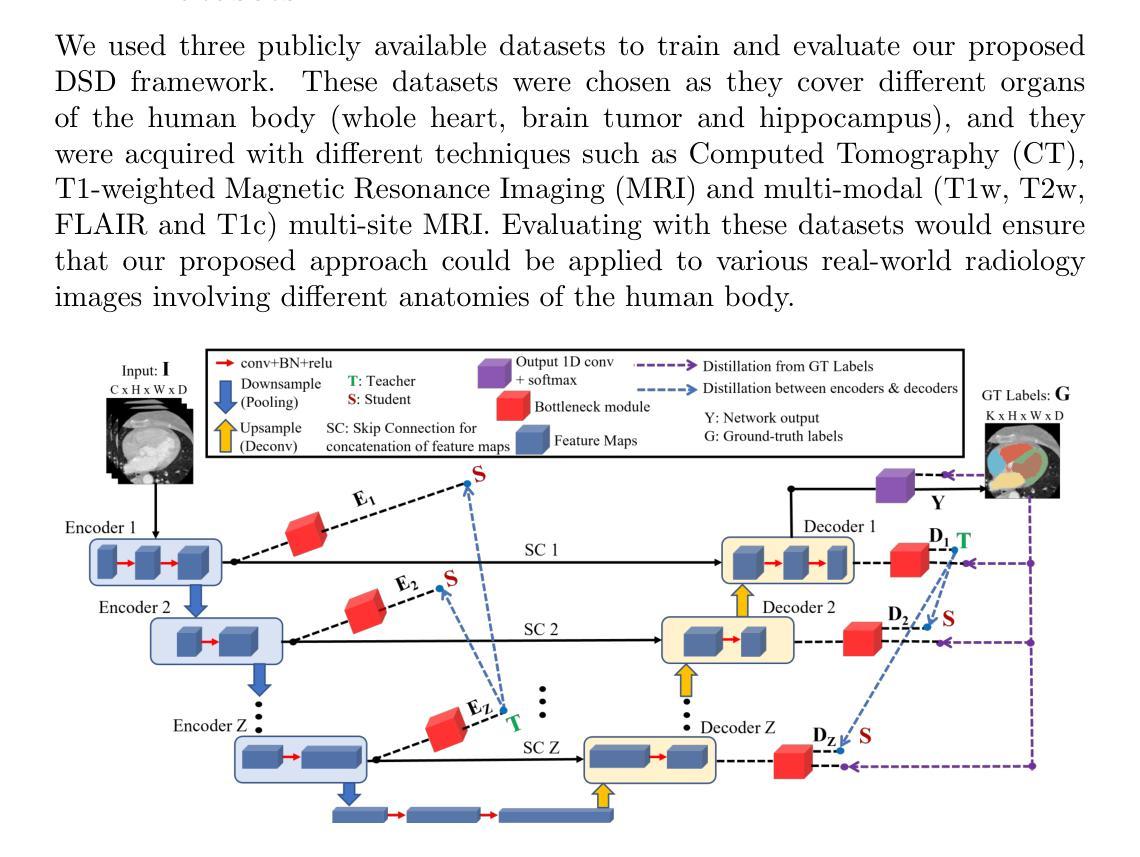