⚠️ 以下所有内容总结都来自于 大语言模型的能力,如有错误,仅供参考,谨慎使用
🔴 请注意:千万不要用于严肃的学术场景,只能用于论文阅读前的初筛!
💗 如果您觉得我们的项目对您有帮助 ChatPaperFree ,还请您给我们一些鼓励!⭐️ HuggingFace免费体验
2025-05-06 更新
T-Graph: Enhancing Sparse-view Camera Pose Estimation by Pairwise Translation Graph
Authors:Qingyu Xian, Weiqin Jiao, Hao Cheng, Berend Jan van der Zwaag, Yanqiu Huang
Sparse-view camera pose estimation, which aims to estimate the 6-Degree-of-Freedom (6-DoF) poses from a limited number of images captured from different viewpoints, is a fundamental yet challenging problem in remote sensing applications. Existing methods often overlook the translation information between each pair of viewpoints, leading to suboptimal performance in sparse-view scenarios. To address this limitation, we introduce T-Graph, a lightweight, plug-and-play module to enhance camera pose estimation in sparse-view settings. T-graph takes paired image features as input and maps them through a Multilayer Perceptron (MLP). It then constructs a fully connected translation graph, where nodes represent cameras and edges encode their translation relationships. It can be seamlessly integrated into existing models as an additional branch in parallel with the original prediction, maintaining efficiency and ease of use. Furthermore, we introduce two pairwise translation representations, relative-t and pair-t, formulated under different local coordinate systems. While relative-t captures intuitive spatial relationships, pair-t offers a rotation-disentangled alternative. The two representations contribute to enhanced adaptability across diverse application scenarios, further improving our module’s robustness. Extensive experiments on two state-of-the-art methods (RelPose++ and Forge) using public datasets (C03D and IMC PhotoTourism) validate both the effectiveness and generalizability of T-Graph. The results demonstrate consistent improvements across various metrics, notably camera center accuracy, which improves by 1% to 6% from 2 to 8 viewpoints.
稀疏视角相机姿态估计旨在从有限的从不同视角捕获的图像中估计出相机的六自由度(6-DoF)姿态,是遥感应用中的一个基本且具有挑战性的问题。现有方法常常忽略各对视角之间的平移信息,导致在稀疏视角场景中的性能不佳。为了解决这个问题,我们引入了T-Graph,这是一个轻量级、即插即用的模块,用于增强稀疏视角下的相机姿态估计。T-Graph以成对的图像特征作为输入,通过多层感知器(MLP)进行映射,然后构建一个全连接的平移图,其中节点代表相机,边编码它们的平移关系。它可以无缝集成到现有模型中,作为与原始预测并行存在的附加分支,保持高效和易用性。此外,我们引入了两种成对平移表示方法,即相对t和配对t,它们在不同的局部坐标系下制定。相对t捕捉直观的空间关系,而配对t则提供一种旋转解耦的替代方案。这两种表示方法有助于提高在不同应用场景中的适应性,进一步增强了模块的稳健性。在两种最新方法(RelPose++和Forge)上使用公开数据集(C03D和IMC PhotoTourism)进行的广泛实验验证了T-Graph的有效性和通用性。结果表明,在各种指标上均实现了持续的改进,尤其是相机中心精度提高了1%至6%,从2个视角到8个视角。
论文及项目相关链接
Summary
本文介绍了针对稀疏视角相机姿态估计的问题,提出了一种名为T-Graph的轻量级、即插即用的模块。该模块利用多层感知器构建全连接的翻译图,节点代表相机,边编码它们的翻译关系。T-Graph可无缝集成到现有模型中,作为与原始预测并行的额外分支,同时保持效率和易用性。此外,还引入了两个配对翻译表示,相对t和配对t,它们在不同的局部坐标系下制定。这两个表示提高了模块在不同应用场景中的适应性,并增强了其稳健性。在公共数据集上的实验验证了T-Graph的有效性和通用性。
Key Takeaways
- 稀疏视角相机姿态估计是一个重要且具挑战性的问题,需要估计从有限图像中获取的6自由度姿态。
- 现有方法忽视了不同视角间的翻译信息,导致在稀疏视角下的性能不佳。
- T-Graph模块利用多层感知器构建全连接的翻译图,以改进稀疏视角下的相机姿态估计。
- T-Graph可轻松集成到现有模型中,作为一个额外的并行分支,同时保持效率和易用性。
- 引入两种配对翻译表示,相对t和配对t,以提高模块在不同应用场景中的适应性。
- 在公共数据集上的实验验证了T-Graph的有效性和通用性,改善了摄像机中心准确度的各项指标。
点此查看论文截图
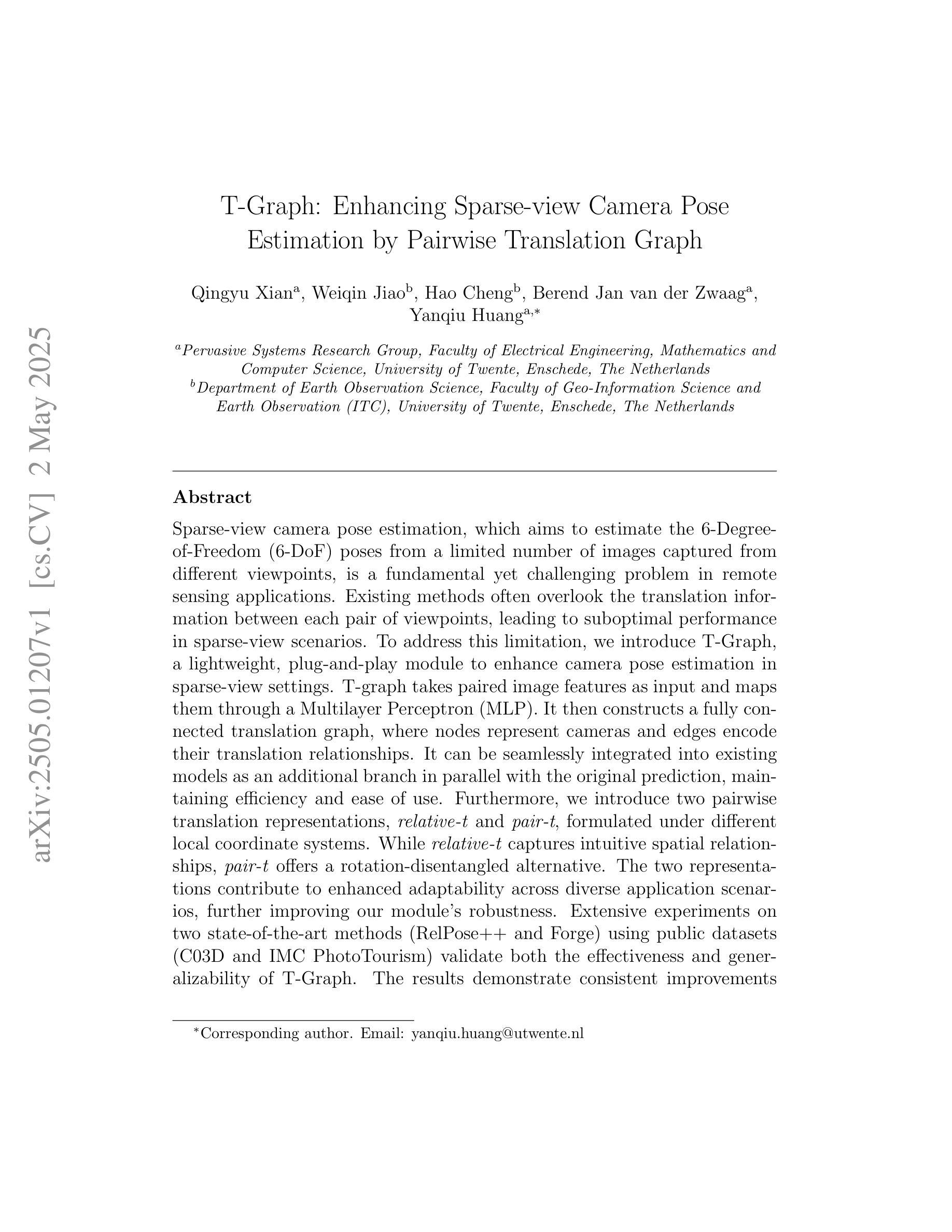
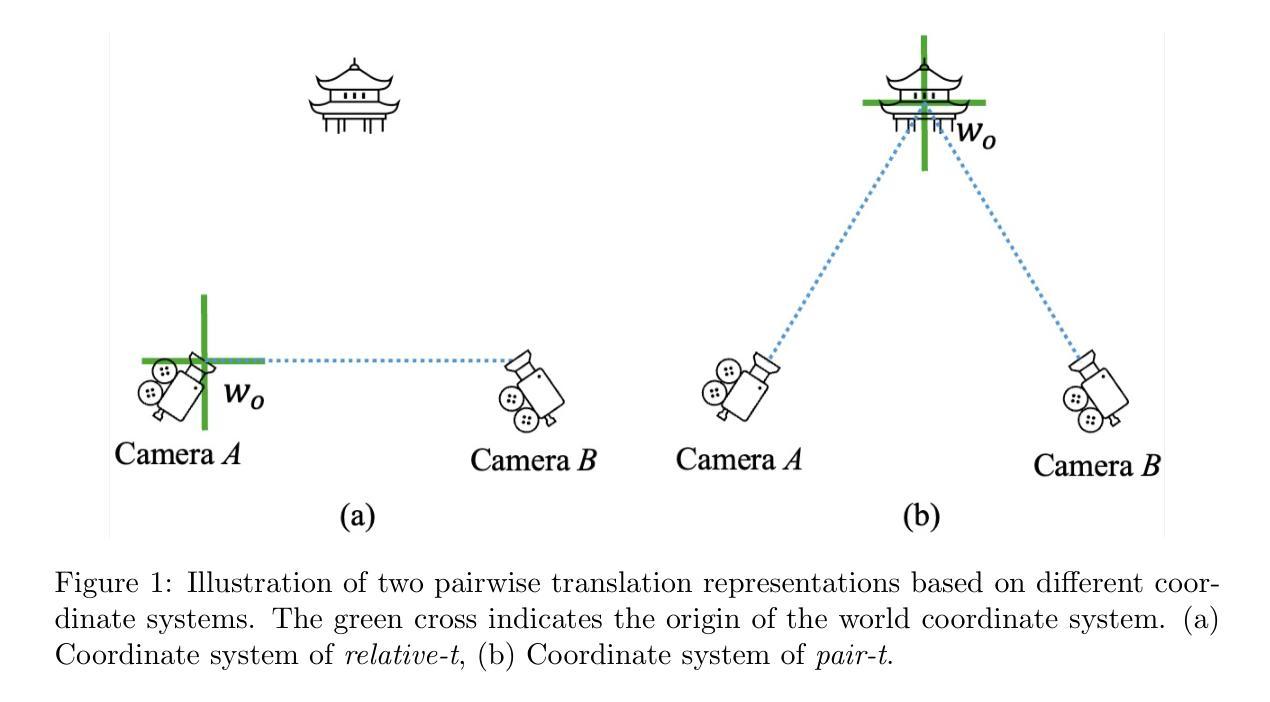