⚠️ 以下所有内容总结都来自于 大语言模型的能力,如有错误,仅供参考,谨慎使用
🔴 请注意:千万不要用于严肃的学术场景,只能用于论文阅读前的初筛!
💗 如果您觉得我们的项目对您有帮助 ChatPaperFree ,还请您给我们一些鼓励!⭐️ HuggingFace免费体验
2025-05-06 更新
How much to Dereverberate? Low-Latency Single-Channel Speech Enhancement in Distant Microphone Scenarios
Authors:Satvik Venkatesh, Philip Coleman, Arthur Benilov, Simon Brown, Selim Sheta, Frederic Roskam
Dereverberation is an important sub-task of Speech Enhancement (SE) to improve the signal’s intelligibility and quality. However, it remains challenging because the reverberation is highly correlated with the signal. Furthermore, the single-channel SE literature has predominantly focused on rooms with short reverb times (typically under 1 second), smaller rooms (under volumes of 1000 cubic meters) and relatively short distances (up to 2 meters). In this paper, we explore real-time low-latency single-channel SE under distant microphone scenarios, such as 5 to 10 meters, and focus on conference rooms and theatres, with larger room dimensions and reverberation times. Such a setup is useful for applications such as lecture demonstrations, drama, and to enhance stage acoustics. First, we show that single-channel SE in such challenging scenarios is feasible. Second, we investigate the relationship between room volume and reverberation time, and demonstrate its importance when randomly simulating room impulse responses. Lastly, we show that for dereverberation with short decay times, preserving early reflections before decaying the transfer function of the room improves overall signal quality.
消混响是语音增强(SE)的一个重要子任务,旨在提高信号的清晰度和质量。然而,消混响仍然是一个挑战,因为混响与信号高度相关。此外,单通道SE文献主要集中在混响时间较短(通常低于1秒)、空间较小(体积小于1000立方米)以及距离相对较近(最多2米)的房间。在本文中,我们研究了远距离麦克风场景下的实时低延迟单通道SE,例如5到10米的距离,并关注于会议室和剧院等具有较大房间尺寸和混响时间的场景。这种设置对于讲座演示、戏剧以及改善舞台声学等应用非常有用。首先,我们证明了在这种具有挑战性的场景中实现单通道SE是可行的。其次,我们研究了房间体积与混响时间之间的关系,并展示了在随机模拟房间冲击响应时其重要性。最后,我们表明,对于具有短衰减时间的消混响,在衰减房间传递函数之前保留早期反射会提高整体信号质量。
论文及项目相关链接
PDF Published in ICASSP 2025
Summary
该文本主要介绍了在语音增强(SE)中消减混响的重要性及其挑战。文中探索了在远距离麦克风场景下的实时低延迟单通道SE,并重点研究了会议室和剧院等大型房间的维度和混响时间较长的场景。研究发现,在具有挑战性的场景中实现单通道SE是可行的;并指出了房间体积与混响时间的关系,以及在模拟房间冲击响应中的重要性;最后指出,对于衰减时间较短的消混响技术,保留早期反射可以改善整体信号质量。
Key Takeaways
- 消减混响是语音增强(SE)的重要子任务,旨在提高信号的清晰度和质量。
- 单通道SE在远距离麦克风场景下面临挑战,特别是大型房间和长混响时间的情况。
- 在具有挑战性的场景中实现单通道SE是可行的。
- 房间体积与混响时间存在关联,这在模拟房间冲击响应时尤为重要。
- 在消混响技术中,对于较短的衰减时间,保留早期反射可以改善信号的整体质量。
- 研究结果对于讲座演示、戏剧、舞台音响增强等应用场景具有实际意义。
点此查看论文截图
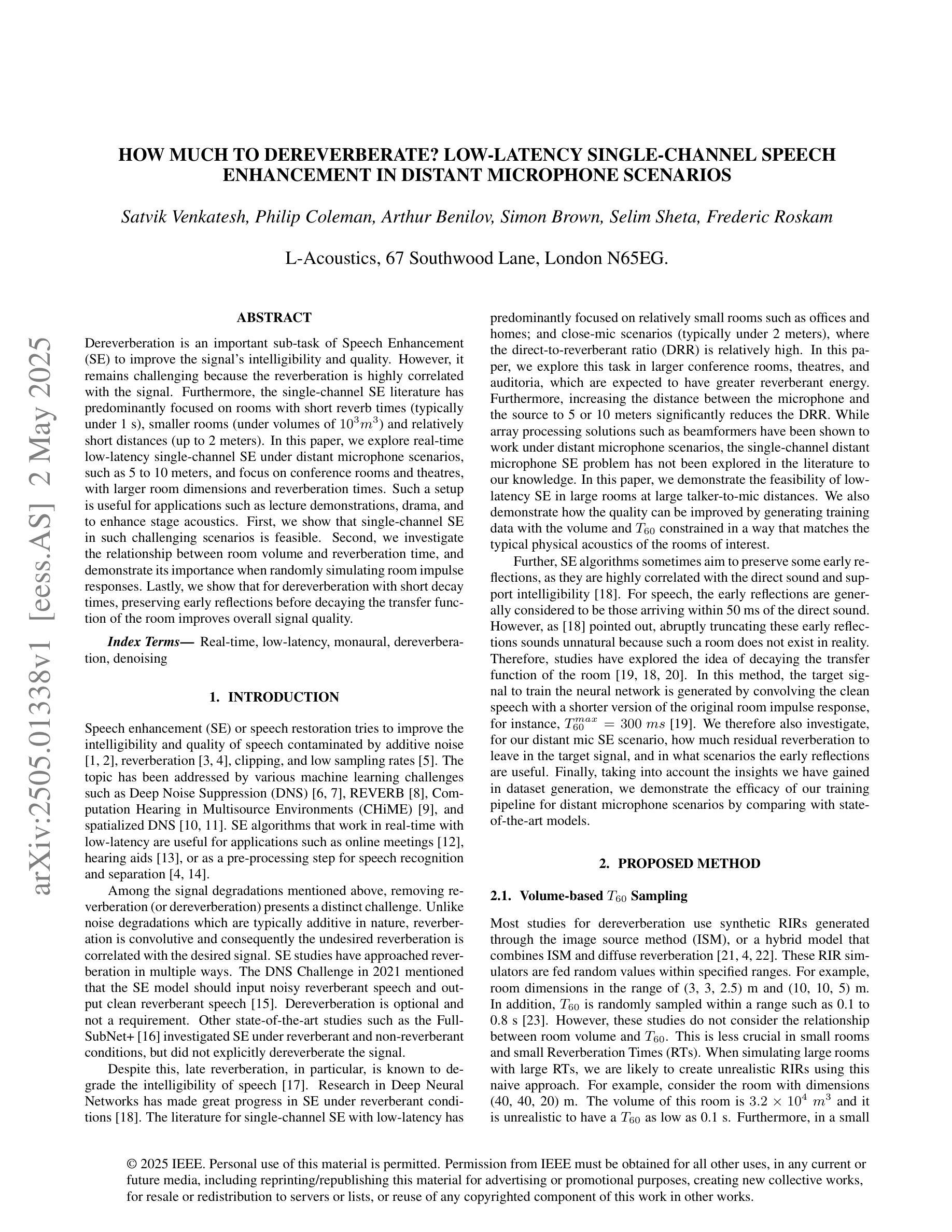
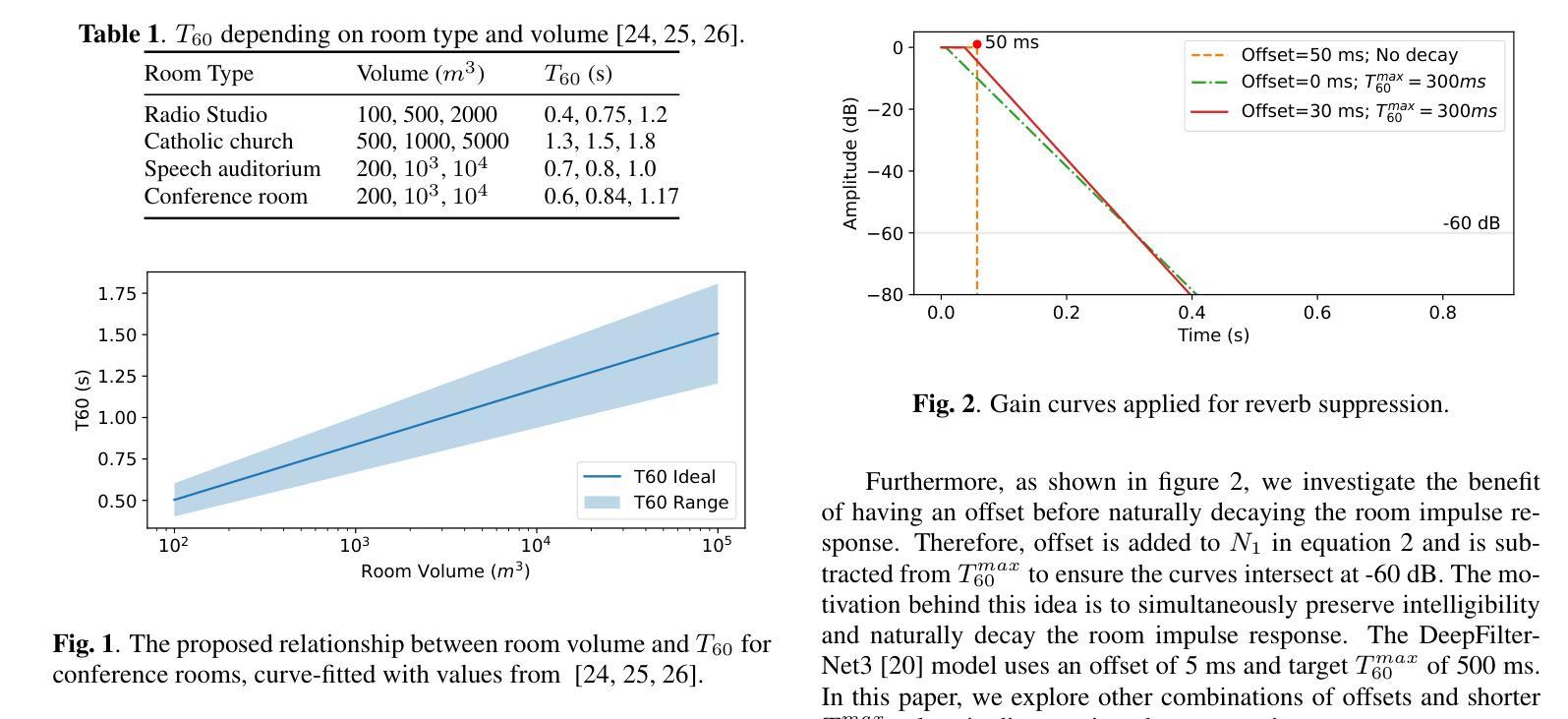
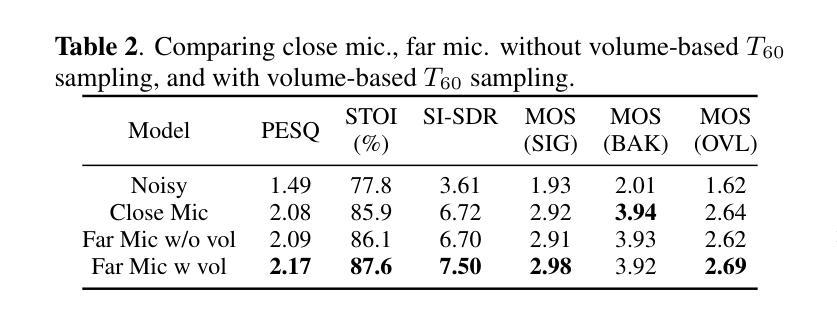
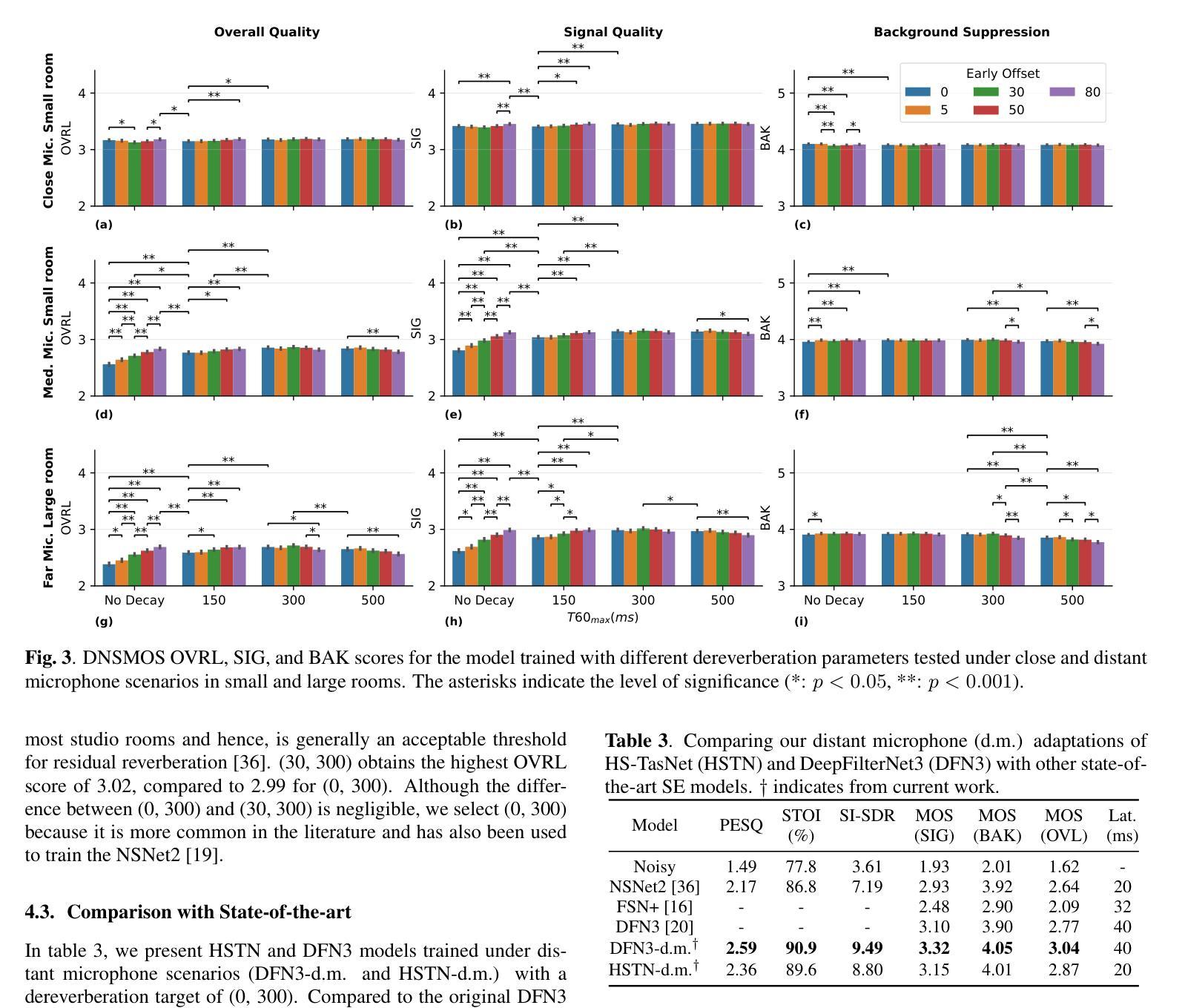
FlowDubber: Movie Dubbing with LLM-based Semantic-aware Learning and Flow Matching based Voice Enhancing
Authors:Gaoxiang Cong, Liang Li, Jiadong Pan, Zhedong Zhang, Amin Beheshti, Anton van den Hengel, Yuankai Qi, Qingming Huang
Movie Dubbing aims to convert scripts into speeches that align with the given movie clip in both temporal and emotional aspects while preserving the vocal timbre of a given brief reference audio. Existing methods focus primarily on reducing the word error rate while ignoring the importance of lip-sync and acoustic quality. To address these issues, we propose a large language model (LLM) based flow matching architecture for dubbing, named FlowDubber, which achieves high-quality audio-visual sync and pronunciation by incorporating a large speech language model and dual contrastive aligning while achieving better acoustic quality via the proposed voice-enhanced flow matching than previous works. First, we introduce Qwen2.5 as the backbone of LLM to learn the in-context sequence from movie scripts and reference audio. Then, the proposed semantic-aware learning focuses on capturing LLM semantic knowledge at the phoneme level. Next, dual contrastive aligning (DCA) boosts mutual alignment with lip movement, reducing ambiguities where similar phonemes might be confused. Finally, the proposed Flow-based Voice Enhancing (FVE) improves acoustic quality in two aspects, which introduces an LLM-based acoustics flow matching guidance to strengthen clarity and uses affine style prior to enhance identity when recovering noise into mel-spectrograms via gradient vector field prediction. Extensive experiments demonstrate that our method outperforms several state-of-the-art methods on two primary benchmarks. The demos are available at {\href{https://galaxycong.github.io/LLM-Flow-Dubber/}{\textcolor{red}{https://galaxycong.github.io/LLM-Flow-Dubber/}}}.
电影配音旨在将剧本转换为与给定电影片段在时间和情感方面对齐的演讲,同时保留给定简短参考音频的音色。现有方法主要关注降低词错误率,而忽视唇形同步和音质的重要性。为了解决这些问题,我们提出了一种基于大型语言模型(LLM)的流式匹配架构用于配音,名为FlowDubber。它通过结合大型语言模型和双对比对齐技术,实现了高质量的声音与影像同步和发音;并且通过提出的语音增强流式匹配技术,在音质方面实现了比之前的工作更好的表现。首先,我们引入Qwen2.5作为LLM的主干,从电影剧本和参考音频中学习上下文序列。然后,提出的语义感知学习侧重于在音素级别捕获LLM语义知识。接下来,双对比对齐(DCA)通过唇部动作加强相互对齐,减少相似音素可能引起的混淆。最后,提出的基于流的语音增强(FVE)从两个方面提高了音质:它引入基于LLM的声学流匹配指导,以增强清晰度,并在通过梯度矢量场预测恢复噪声时,使用仿射风格来增强身份特征。大量实验表明,我们的方法在两个主要基准测试上优于几种最新技术。演示作品请访问:[https://galaxycong.github.io/LLM-Flow-Dubber/]。(红色为超链接)
论文及项目相关链接
Summary
本文介绍了一种基于大型语言模型(LLM)的电影配音方法FlowDubber,旨在将剧本转换为与电影片段在时间和情感方面对齐的语音,同时保留给定简短参考音频的音色。该方法通过引入LLM作为骨干、语义感知学习、双重对比对齐和基于流的语音增强等技术,解决了现有方法忽视唇同步和音质的问题,实现了高质量的音视频同步和发音。
Key Takeaways
- FlowDubber是一种基于大型语言模型(LLM)的电影配音方法,旨在实现剧本与电影片段在时间和情感上的对齐。
- FlowDubber结合了LLM和双重对比对齐技术,提高了音频视觉同步和发音质量。
- FlowDubber通过引入语义感知学习,能够捕捉LLM在音素级别的语义知识。
- 双重对比对齐技术(DCA)增强了与唇部动作的相互对齐,减少了相似音素可能产生的混淆。
- 基于流的语音增强(FVE)技术提高了音频的音质,通过LLM引导的声学流匹配指导和仿射风格先验增强身份识别。
- 实验表明,FlowDubber在两项主要基准测试中优于几种最新方法。
- 演示网站为https://galaxycong.github.io/LLM-Flow-Dubber/。
点此查看论文截图
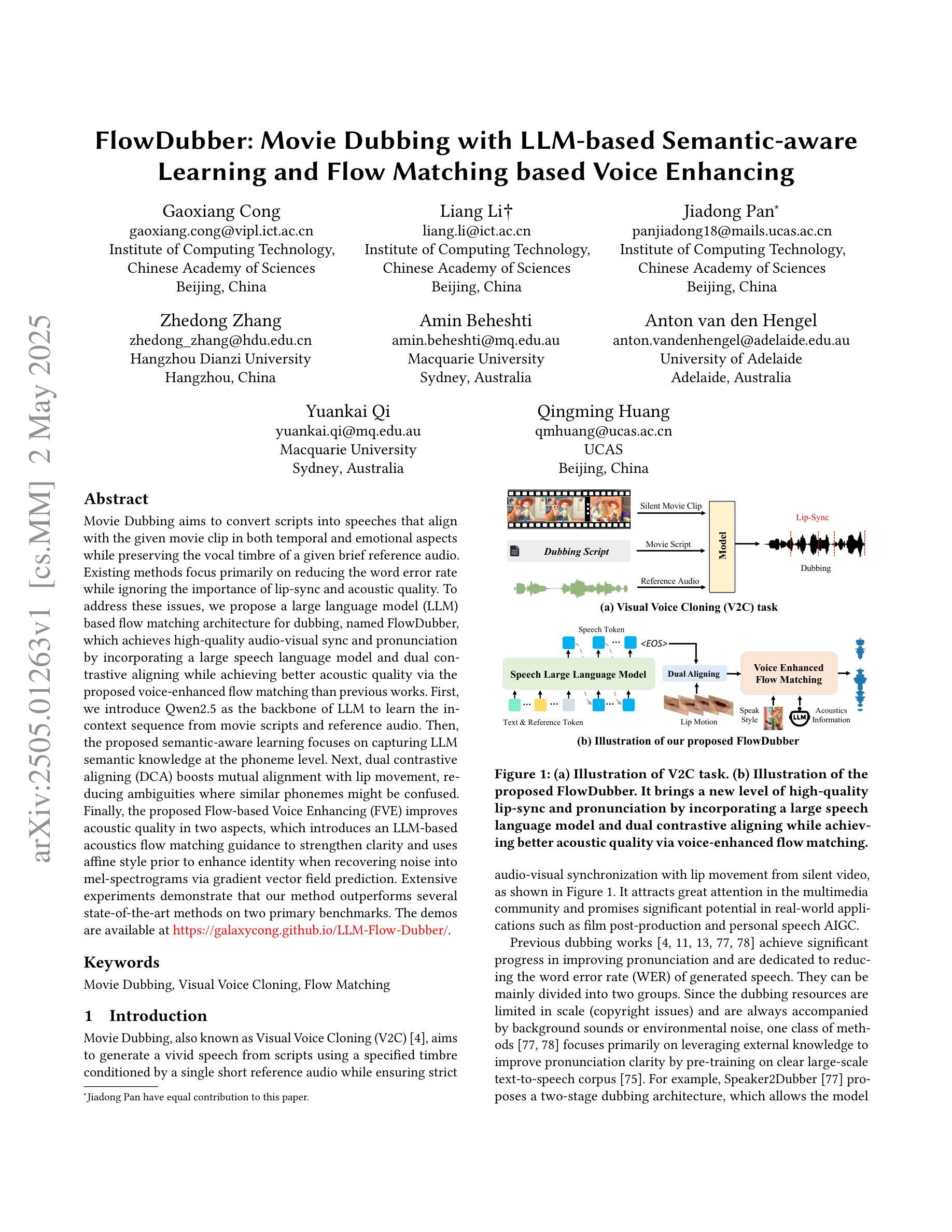
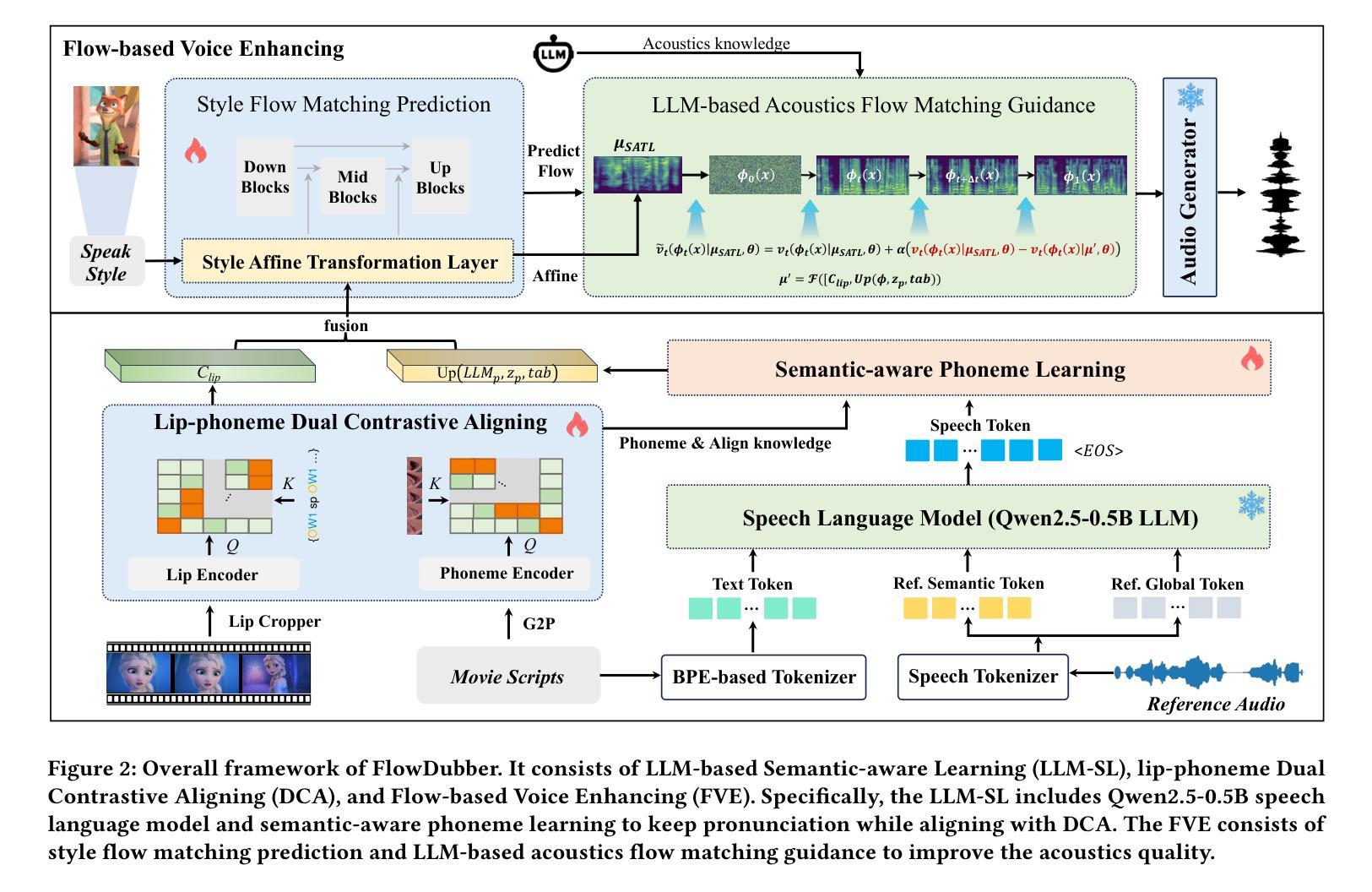
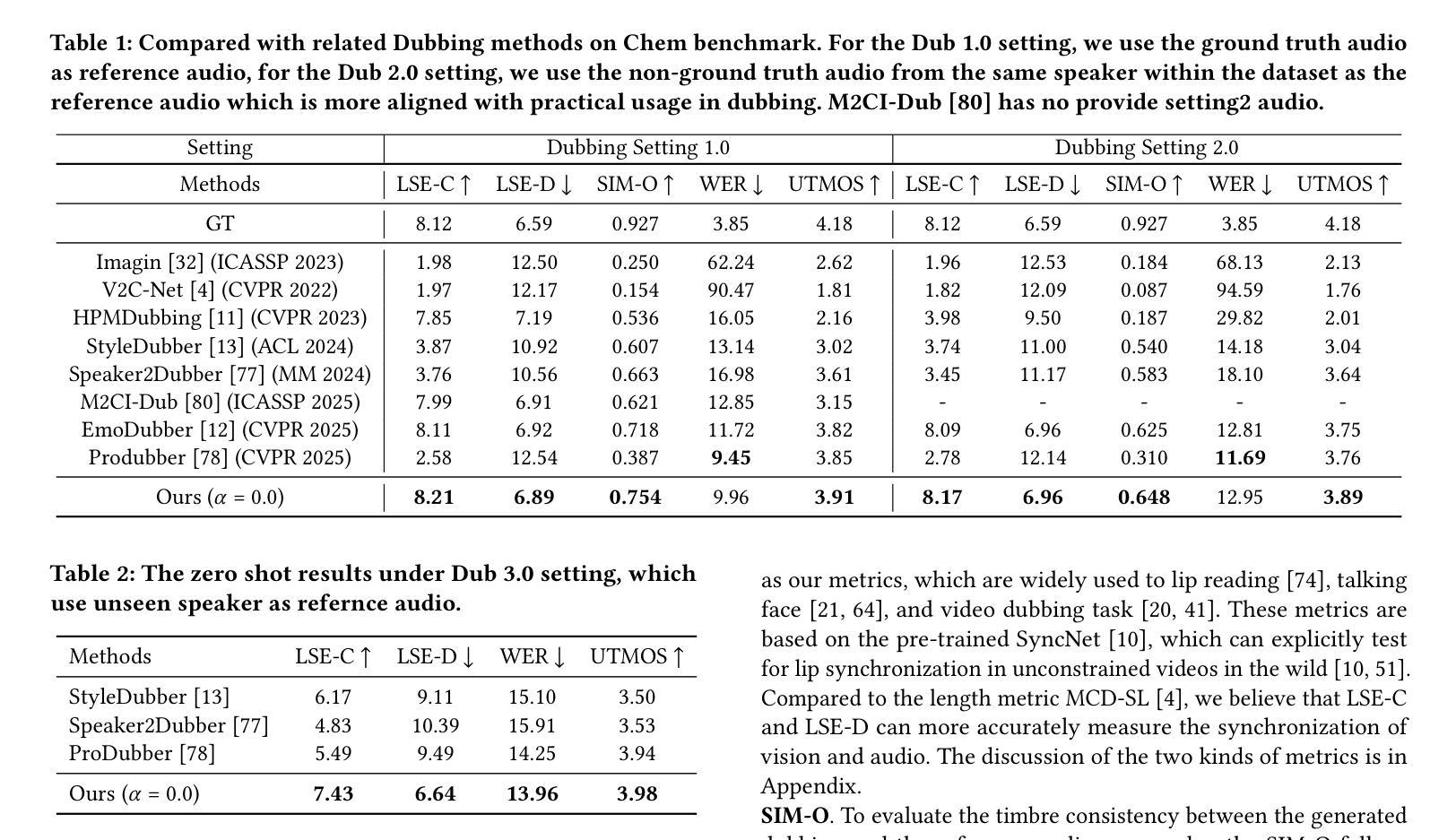