⚠️ 以下所有内容总结都来自于 大语言模型的能力,如有错误,仅供参考,谨慎使用
🔴 请注意:千万不要用于严肃的学术场景,只能用于论文阅读前的初筛!
💗 如果您觉得我们的项目对您有帮助 ChatPaperFree ,还请您给我们一些鼓励!⭐️ HuggingFace免费体验
2025-05-08 更新
MFCLIP: Multi-modal Fine-grained CLIP for Generalizable Diffusion Face Forgery Detection
Authors:Yaning Zhang, Tianyi Wang, Zitong Yu, Zan Gao, Linlin Shen, Shengyong Chen
The rapid development of photo-realistic face generation methods has raised significant concerns in society and academia, highlighting the urgent need for robust and generalizable face forgery detection (FFD) techniques. Although existing approaches mainly capture face forgery patterns using image modality, other modalities like fine-grained noises and texts are not fully explored, which limits the generalization capability of the model. In addition, most FFD methods tend to identify facial images generated by GAN, but struggle to detect unseen diffusion-synthesized ones. To address the limitations, we aim to leverage the cutting-edge foundation model, contrastive language-image pre-training (CLIP), to achieve generalizable diffusion face forgery detection (DFFD). In this paper, we propose a novel multi-modal fine-grained CLIP (MFCLIP) model, which mines comprehensive and fine-grained forgery traces across image-noise modalities via language-guided face forgery representation learning, to facilitate the advancement of DFFD. Specifically, we devise a fine-grained language encoder (FLE) that extracts fine global language features from hierarchical text prompts. We design a multi-modal vision encoder (MVE) to capture global image forgery embeddings as well as fine-grained noise forgery patterns extracted from the richest patch, and integrate them to mine general visual forgery traces. Moreover, we build an innovative plug-and-play sample pair attention (SPA) method to emphasize relevant negative pairs and suppress irrelevant ones, allowing cross-modality sample pairs to conduct more flexible alignment. Extensive experiments and visualizations show that our model outperforms the state of the arts on different settings like cross-generator, cross-forgery, and cross-dataset evaluations.
人脸识别技术的快速发展在社会和学术界引发了广泛关注,凸显了对稳健且可泛化的面部伪造检测(FFD)技术的迫切需求。尽管现有方法主要使用图像模式来捕捉面部伪造模式,但其他模式(如细微噪声和文本)尚未得到完全探索,这限制了模型的泛化能力。此外,大多数FFD方法往往能够识别由生成对抗网络(GAN)生成的面部图像,但难以检测未见过的扩散合成图像。
针对这些局限,我们旨在利用最前沿的基础模型——对比语言图像预训练(CLIP),实现可泛化的扩散面部伪造检测(DFFD)。在本文中,我们提出了一种新颖的多模态精细粒度CLIP(MFCLIP)模型,它通过语言引导的面部伪造表示学习,挖掘图像噪声模态之间全面且精细的伪造痕迹,以促进DFFD的发展。
论文及项目相关链接
Summary
人脸识别技术迅速发展带来的伪造脸技术引起社会与学术界的关注,对通用化的人脸伪造检测(FFD)技术需求迫切。现有方法主要依赖图像模态捕捉伪造痕迹,忽视了精细纹理噪声和文字模态的潜力,限制了模型的泛化能力。为解决这一问题,研究者借助预训练模型CLIP技术,提出了基于多模态精细纹理的CLIP模型(MFCLIP),该模型通过学习语言引导的面庞伪造表达挖掘跨图像噪声模态的全面精细伪造痕迹,促进了扩散伪造脸检测的发展。通过设计精细语言编码器(FLE)和多模态视觉编码器(MVE),以及创新的样本对注意力(SPA)方法,实现模态间的灵活对齐,取得了在跨生成器、跨伪造及跨数据集等多种评估场景下的良好性能提升。
Key Takeaways
- 人脸伪造技术引起社会与学术界的关注,对通用化的人脸伪造检测技术需求迫切。
- 当前人脸伪造检测主要依赖图像模态,忽略了其他模态如精细纹理噪声和文字模态的重要性。
- 利用预训练模型CLIP技术提出了一种新型的多模态CLIP模型(MFCLIP),融合了语言引导的面庞伪造表达学习。
- MFCLIP模型通过设计精细语言编码器(FLE)和多模态视觉编码器(MVE)挖掘全面的精细伪造痕迹。
- 创新性的样本对注意力(SPA)方法强化了模型性能,实现了模态间的灵活对齐。
- MFCLIP模型在多种评估场景下表现出优异的性能,包括跨生成器、跨伪造和跨数据集评估。
点此查看论文截图
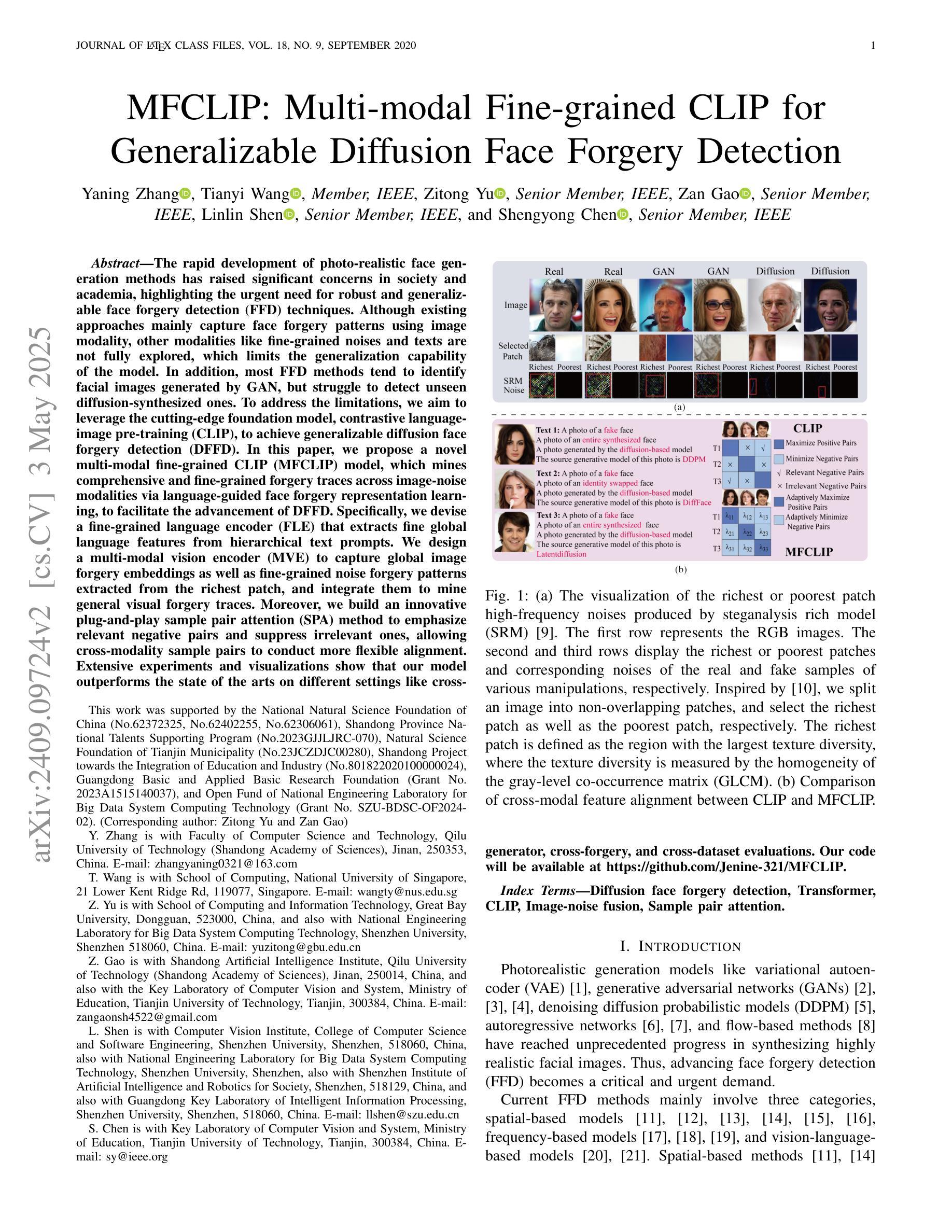
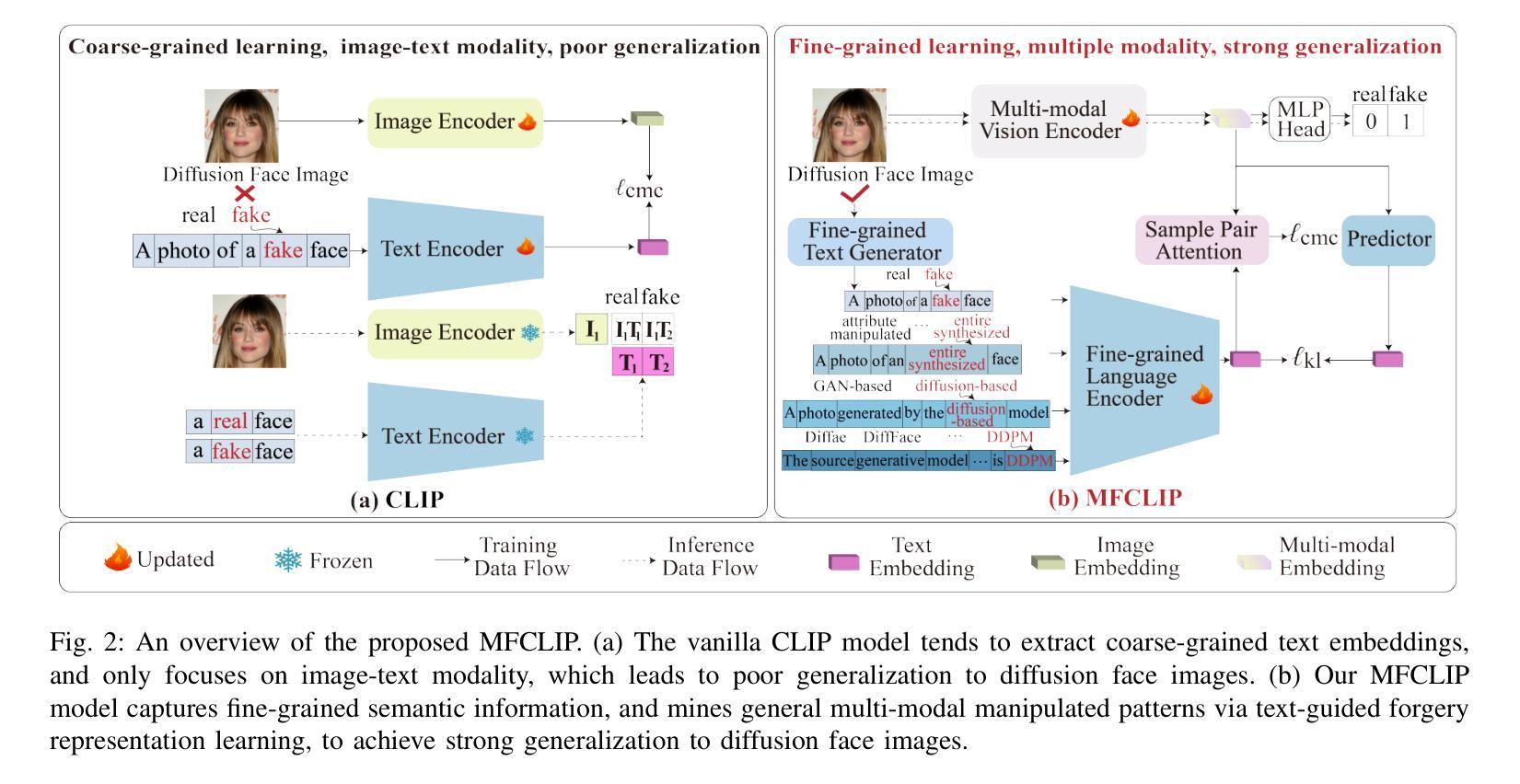
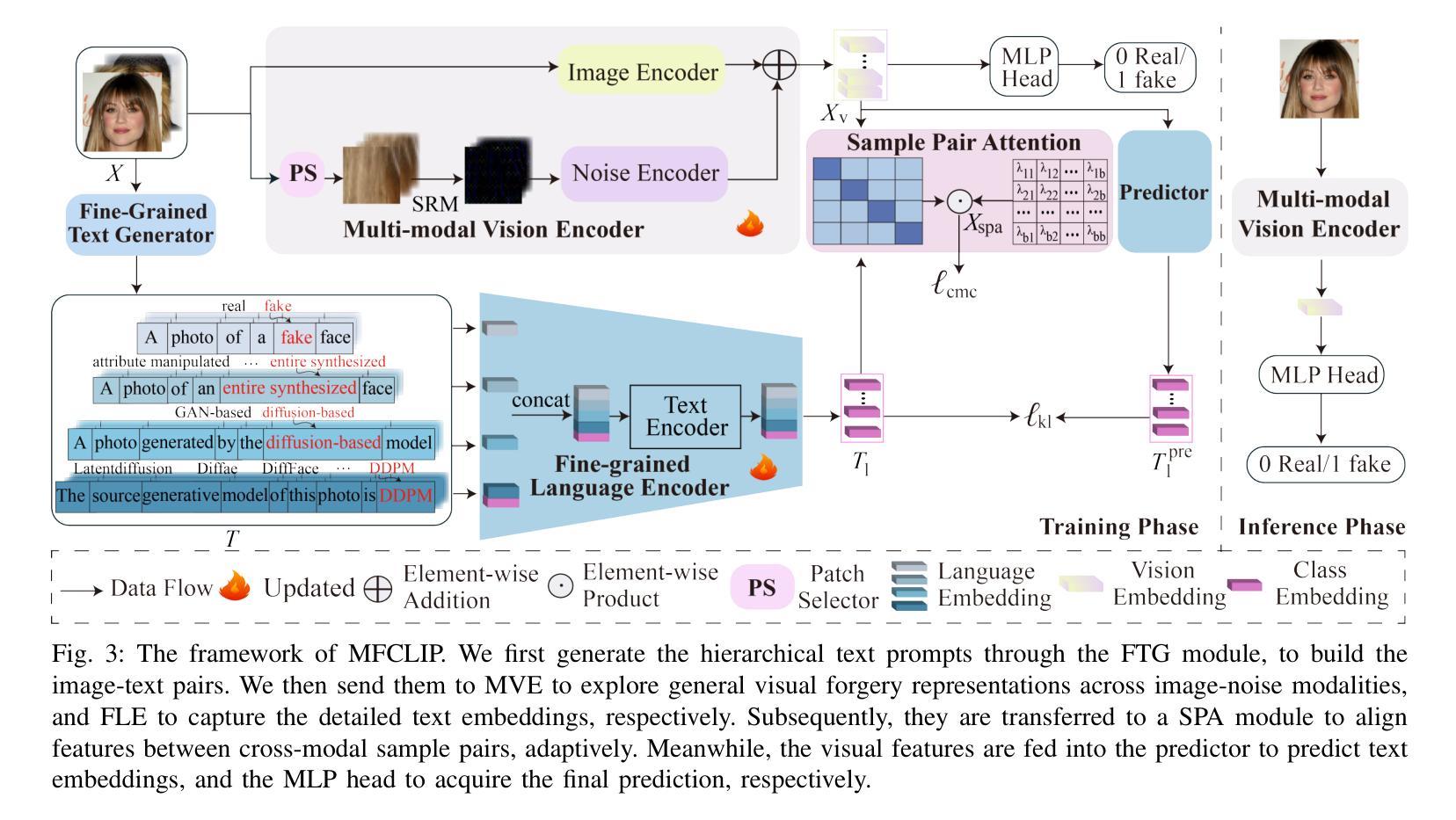
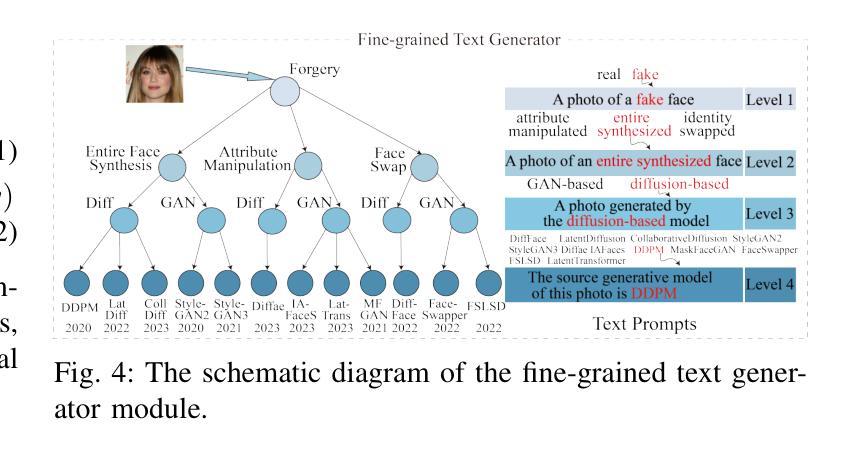
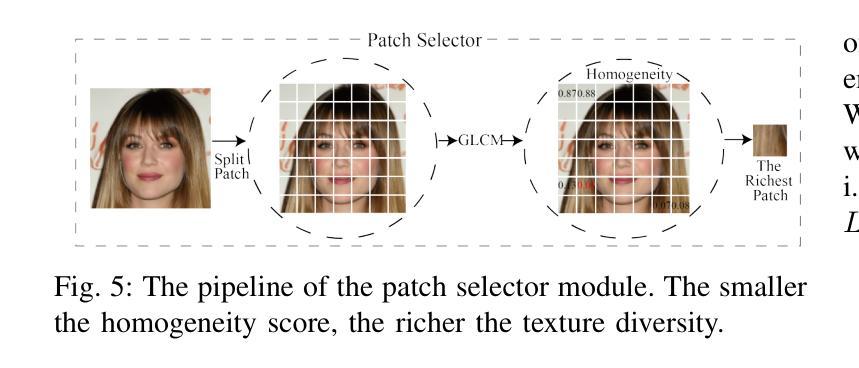
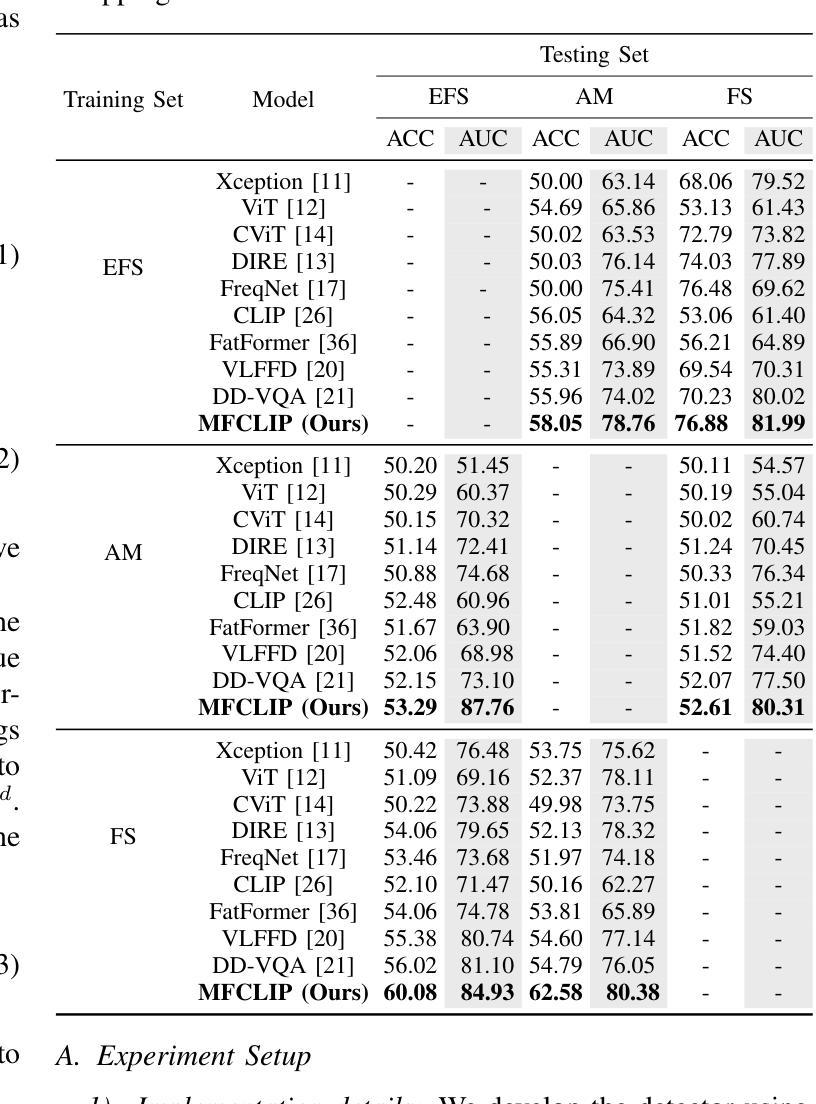