⚠️ 以下所有内容总结都来自于 大语言模型的能力,如有错误,仅供参考,谨慎使用
🔴 请注意:千万不要用于严肃的学术场景,只能用于论文阅读前的初筛!
💗 如果您觉得我们的项目对您有帮助 ChatPaperFree ,还请您给我们一些鼓励!⭐️ HuggingFace免费体验
2025-05-08 更新
A Robust Monotonic Single-Index Model for Skewed and Heavy-Tailed Data: A Deep Neural Network Approach Applied to Periodontal Studies
Authors:Qingyang Liu, Shijie Wang, Ray Bai, Dipankar Bandyopadhyay
Periodontal pocket depth is a widely used biomarker for diagnosing risk of periodontal disease. However, pocket depth typically exhibits skewness and heavy-tailedness, and its relationship with clinical risk factors is often nonlinear. Motivated by periodontal studies, this paper develops a robust single-index modal regression framework for analyzing skewed and heavy-tailed data. Our method has the following novel features: (1) a flexible two-piece scale Student-$t$ error distribution that generalizes both normal and two-piece scale normal distributions; (2) a deep neural network with guaranteed monotonicity constraints to estimate the unknown single-index function; and (3) theoretical guarantees, including model identifiability and a universal approximation theorem. Our single-index model combines the flexibility of neural networks and the two-piece scale Student-$t$ distribution, delivering robust mode-based estimation that is resistant to outliers, while retaining clinical interpretability through parametric index coefficients. We demonstrate the performance of our method through simulation studies and an application to periodontal disease data from the HealthPartners Institute of Minnesota. The proposed methodology is implemented in the \textsf{R} package \href{https://doi.org/10.32614/CRAN.package.DNNSIM}{\textsc{DNNSIM}}.
牙周袋深度是诊断牙周病风险的一个广泛使用的生物标志物。然而,袋深度通常表现出偏斜和重尾特性,其与临床风险因素的关系通常是非线性的。本文受牙周研究启发,开发了一个稳健的单指数模态回归框架,用于分析偏斜和重尾数据。我们的方法具有以下新颖特点:(1)一个灵活的两段尺度学生-t误差分布,它既概括了正态分布和两段尺度正态分布;(2)一个深度神经网络,通过保证单调性约束来估计未知的单指数函数;(3)理论保证,包括模型可识别性和通用近似定理。我们的单指数模型结合了神经网络的两段尺度学生-t分布的灵活性,提供了稳健的基于模式的估计,对异常值具有抵抗力,同时通过参数指数系数保持临床可解释性。我们通过模拟研究和一项针对明尼苏达HealthPartners研究所的牙周病数据的应用,展示了该方法的性能。所提出的方法在R包https://doi.org/10.32614/CRAN.package.DNNSIM中的\textDNNSIM>中实现。
论文及项目相关链接
PDF 27 pages,13 figures
Summary
这篇论文针对牙周病风险诊断中的生物标志物牙周袋深度进行研究。由于牙周袋深度数据存在偏斜和重尾特性,且其与临床风险因素的关系往往非线性,论文提出了一种稳健的单指数模态回归框架来分析这类数据。该方法具有灵活的两段尺度学生t误差分布、满足单调性约束的深度神经网络和理论保证等新颖特点。通过模拟研究和应用至明尼苏达健康伙伴研究所的牙周病数据,验证了该方法的性能。此方法结合了神经网络的灵活性和两段尺度学生t分布,提供稳健的模式估计,对异常值具有抵抗力,同时通过参数指数系数保持临床可解释性。
Key Takeaways
- 牙周袋深度是诊断牙周病风险的重要生物标志物,但其数据存在偏斜和重尾特性。
- 现有方法与临床风险因素的关系常呈非线性,需更精准的模型进行分析。
- 论文提出了一个稳健的单指数模态回归框架,适用于分析此类数据。
- 该方法具备新颖特点:灵活的两段尺度学生t误差分布、满足单调性约束的深度神经网络以及包括模型可识别性和通用逼近定理的理论保证。
- 方法结合了神经网络的灵活性和两段尺度学生t分布的稳健性,提供模式估计,对异常值具有抗性,并保持临床可解释性。
- 通过模拟研究和实际应用于牙周病数据,验证了该方法的性能。
点此查看论文截图
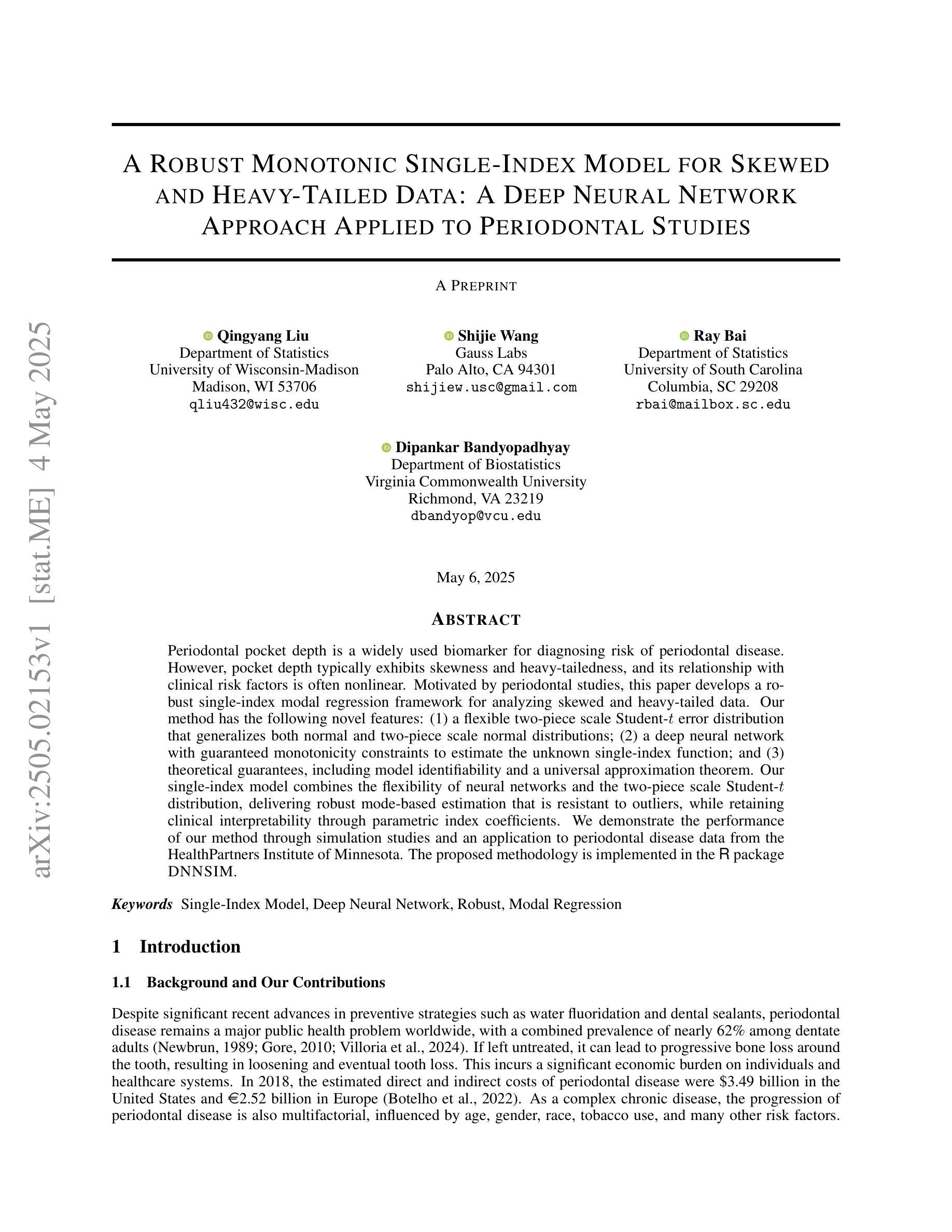
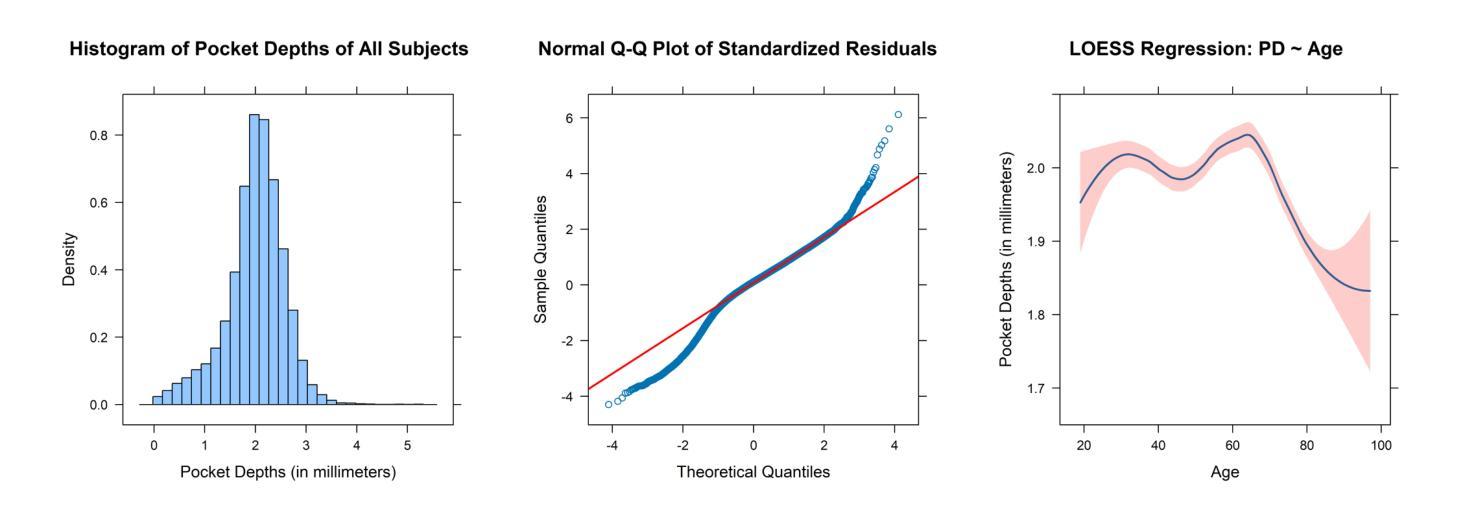
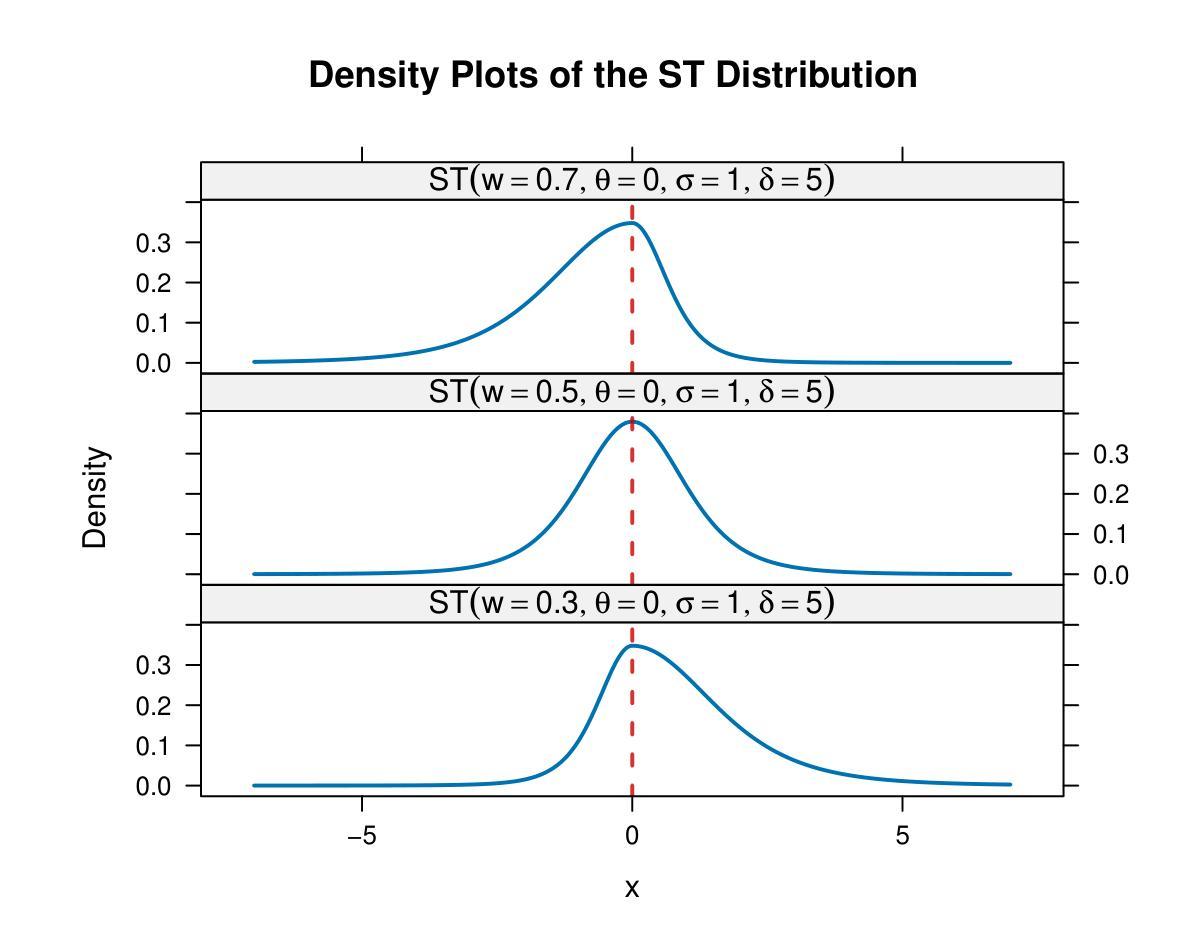