⚠️ 以下所有内容总结都来自于 大语言模型的能力,如有错误,仅供参考,谨慎使用
🔴 请注意:千万不要用于严肃的学术场景,只能用于论文阅读前的初筛!
💗 如果您觉得我们的项目对您有帮助 ChatPaperFree ,还请您给我们一些鼓励!⭐️ HuggingFace免费体验
2025-05-08 更新
Reinforced Correlation Between Vision and Language for Precise Medical AI Assistant
Authors:Haonan Wang, Jiaji Mao, Lehan Wang, Qixiang Zhang, Marawan Elbatel, Yi Qin, Huijun Hu, Baoxun Li, Wenhui Deng, Weifeng Qin, Hongrui Li, Jialin Liang, Jun Shen, Xiaomeng Li
Medical AI assistants support doctors in disease diagnosis, medical image analysis, and report generation. However, they still face significant challenges in clinical use, including limited accuracy with multimodal content and insufficient validation in real-world settings. We propose RCMed, a full-stack AI assistant that improves multimodal alignment in both input and output, enabling precise anatomical delineation, accurate localization, and reliable diagnosis through hierarchical vision-language grounding. A self-reinforcing correlation mechanism allows visual features to inform language context, while language semantics guide pixel-wise attention, forming a closed loop that refines both modalities. This correlation is enhanced by a color region description strategy, translating anatomical structures into semantically rich text to learn shape-location-text relationships across scales. Trained on 20 million image-mask-description triplets, RCMed achieves state-of-the-art precision in contextualizing irregular lesions and subtle anatomical boundaries, excelling in 165 clinical tasks across 9 modalities. It achieved a 23.5% relative improvement in cell segmentation from microscopy images over prior methods. RCMed’s strong vision-language alignment enables exceptional generalization, with state-of-the-art performance in external validation across 20 clinically significant cancer types, including novel tasks. This work demonstrates how integrated multimodal models capture fine-grained patterns, enabling human-level interpretation in complex scenarios and advancing human-centric AI healthcare.
医疗人工智能助手支持医生进行疾病诊断、医学图像分析和报告生成。然而,他们在临床使用中还面临诸多挑战,包括多模态内容精度有限和真实世界环境中的验证不足。我们提出了RCMed,这是一个端到端的人工智能助手,它改进了输入和输出的多模态对齐,能够通过分层视觉语言定位实现精确解剖划分、准确定位和可靠诊断。自我加强的关联机制允许视觉特征为语言上下文提供信息,而语言语义引导像素级注意力,形成一个闭环,精炼了两种模式。这种关联通过颜色区域描述策略得到加强,将解剖结构转化为语义丰富的文本,学习跨尺度的形状-位置-文本关系。在2000万个图像-掩膜-描述三元组上进行训练,RCMed在定位不规则病变和微妙解剖边界方面达到了最先进的精度,在9种模式的165个临床任务中表现出色。与先前的方法相比,它在显微镜图像的细胞分割方面实现了相对23.5%的改进。RCMed强大的视觉语言对齐功能实现了出色的泛化能力,在20种具有临床意义的癌症类型的外部验证中达到了最先进的性能,包括新任务。这项工作展示了集成多模态模型如何捕捉精细模式,在复杂场景中实现人类水平的解释能力,并推动以人为本的人工智能医疗保健的发展。
论文及项目相关链接
Summary:
医疗人工智能助手在疾病诊断、医学图像分析和报告生成方面支持医生工作。然而,在实际应用中仍面临多模态内容准确性有限和真实世界环境下验证不足等挑战。本文提出一种全栈式人工智能助手RCMed,通过改进多模态输入输出的对齐方式,实现精确解剖界定、准确定位和可靠诊断。其采用层次化的视觉语言定位技术,通过自我加强的关联机制,实现视觉特征与语言语境的信息交流,提高两种模态的精度。在200万张图像、遮罩和描述三元组训练数据支持下,RCMed在定位不规则病变和微妙解剖边界方面达到最新精准度水平,并在165项临床任务中表现出卓越性能。此外,RCMed在显微镜图像细胞分割方面较前期方法实现了23.5%的相对改进。其强大的视觉语言对齐能力使RCMed在外部验证中表现出卓越泛化能力,涵盖20种临床重要的癌症类型,包括新型任务。本研究展示了集成多模态模型如何捕捉细微模式,实现复杂场景下的人类水平解读,推动以人为中心的人工智能医疗健康发展。
Key Takeaways:
- 医疗AI助手在临床应用中面临多模态内容准确性和真实世界验证挑战。
- RCMed是一种全栈式AI助手,改进了多模态输入输出的对齐方式。
- RCMed实现了视觉特征与语言语境的信息交流,提高诊断精确性。
- RCMed在多种临床任务中表现优异,达到最新精准度水平。
- RCMed在显微镜图像细胞分割方面较前期方法有显著改进。
- RCMed具有强大的视觉语言对齐能力,广泛适用于不同类型的癌症诊断。
- 集成多模态模型有助于捕捉细微模式,推动医疗AI的发展。
点此查看论文截图
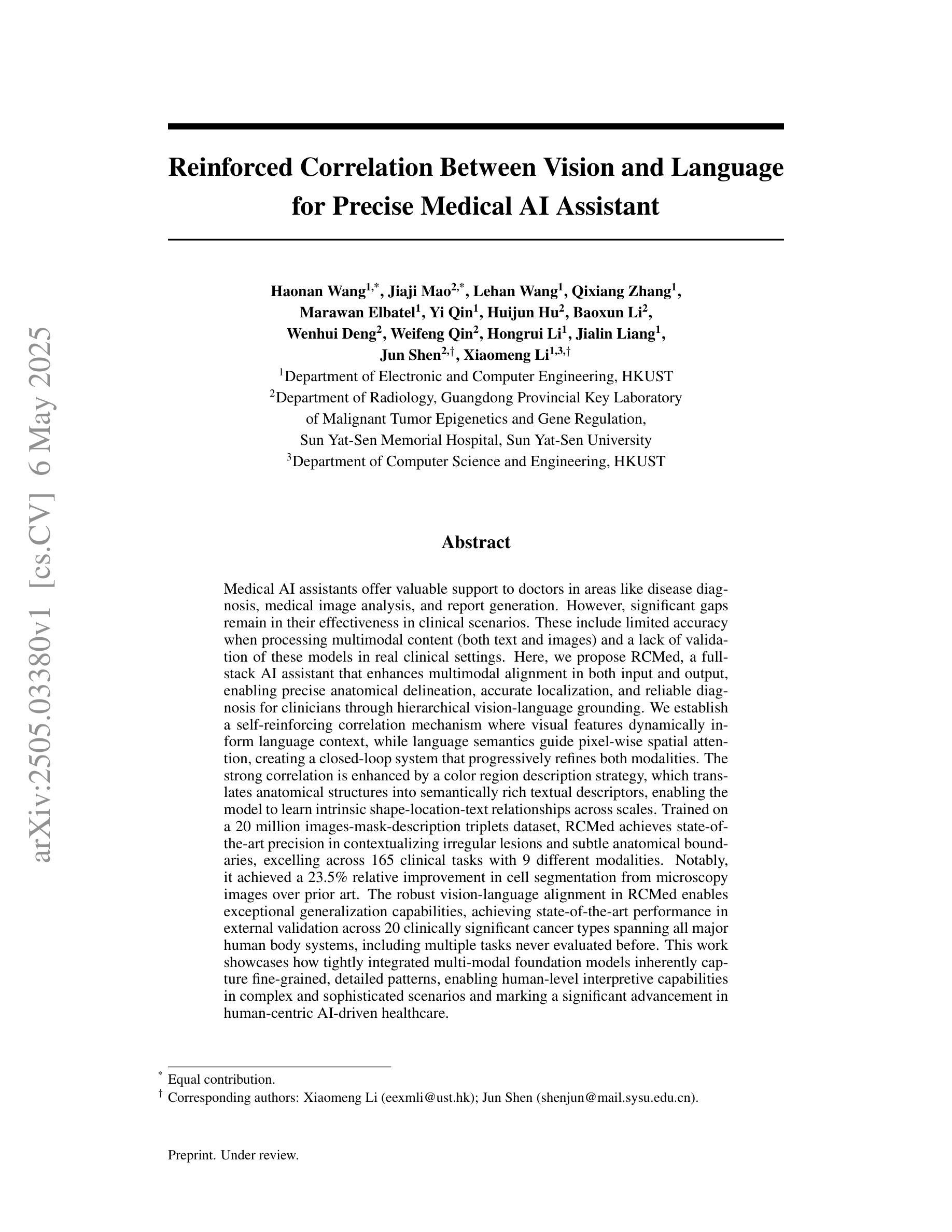
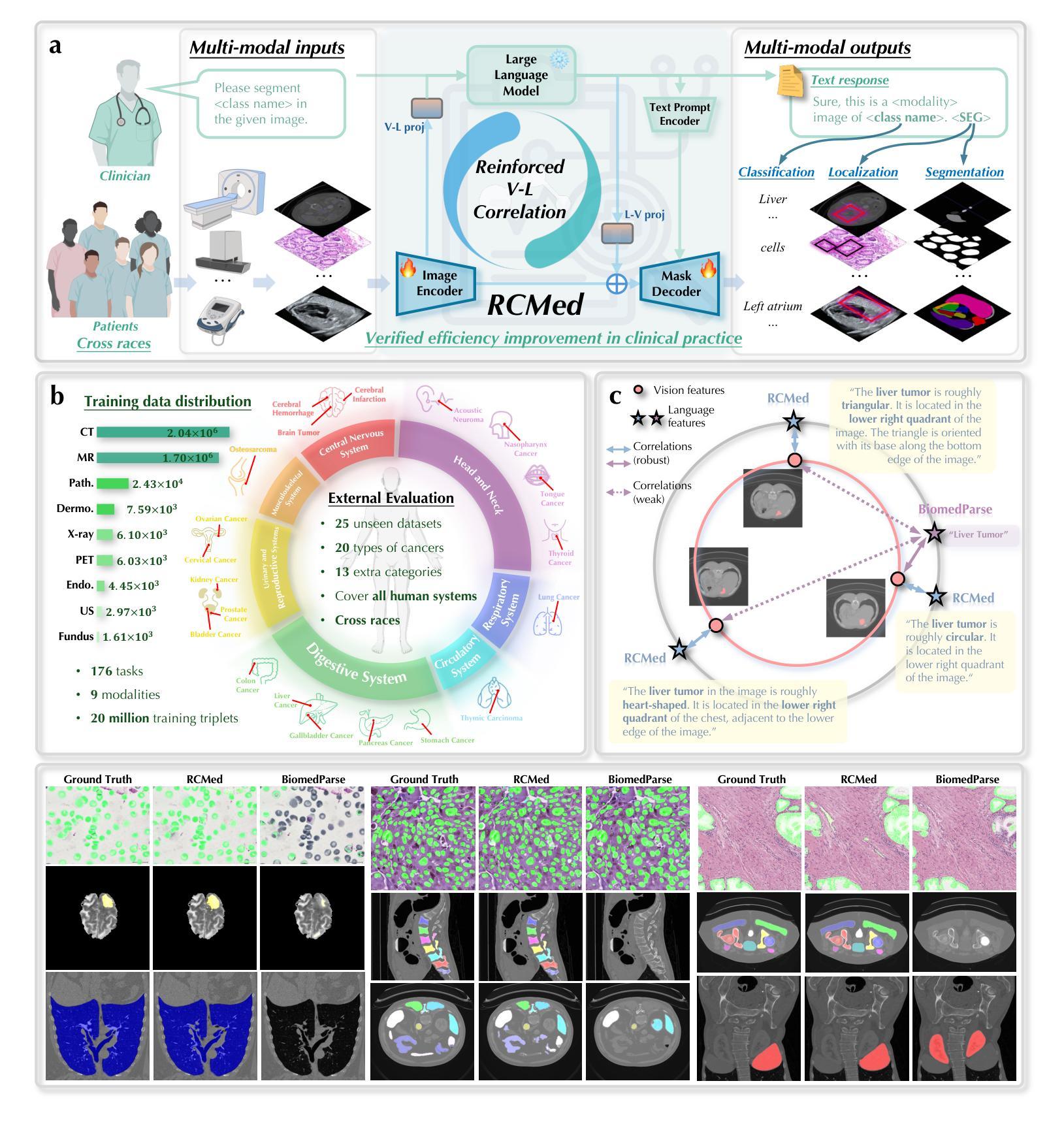
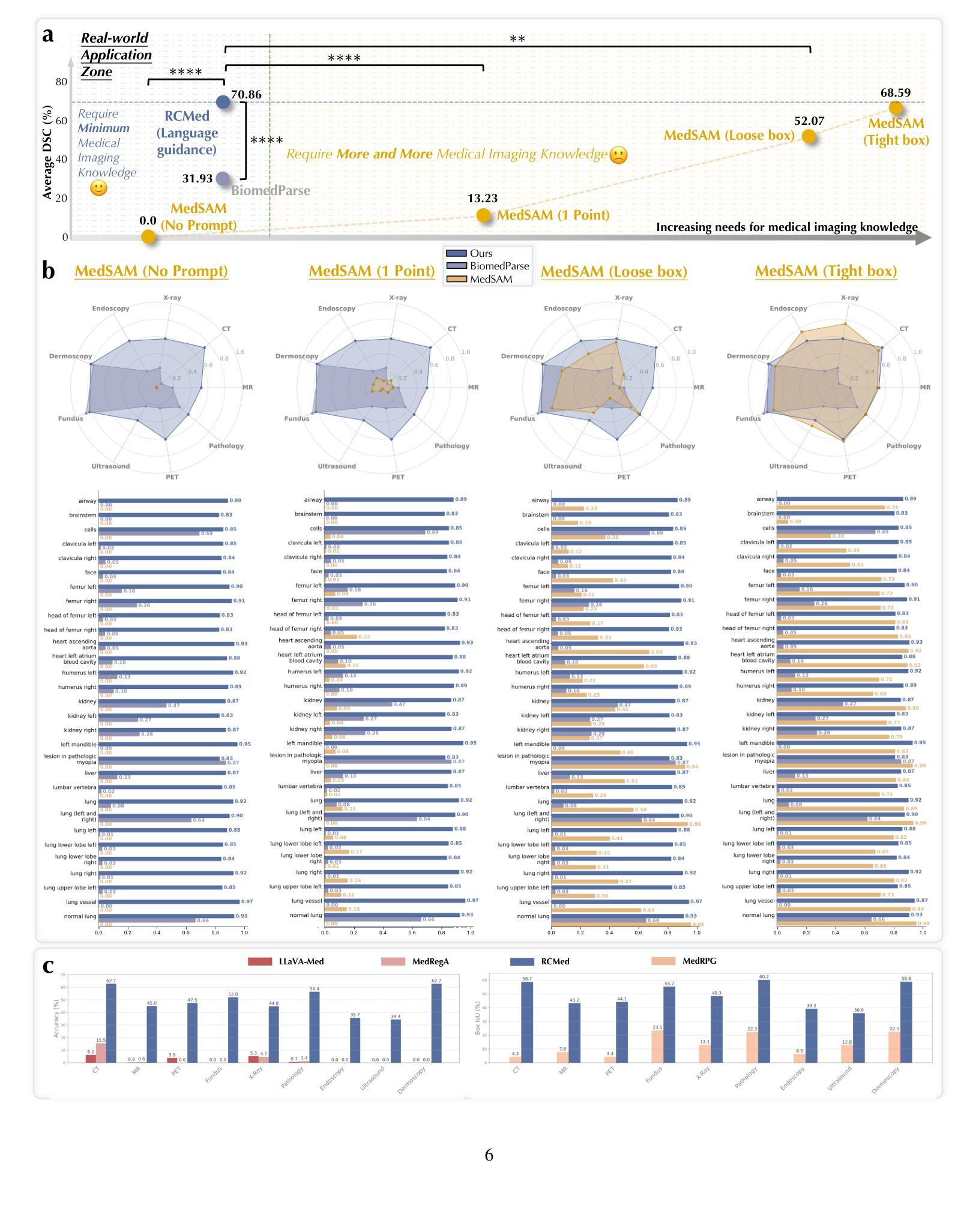
Seeing the Abstract: Translating the Abstract Language for Vision Language Models
Authors:Davide Talon, Federico Girella, Ziyue Liu, Marco Cristani, Yiming Wang
Natural language goes beyond dryly describing visual content. It contains rich abstract concepts to express feeling, creativity and properties that cannot be directly perceived. Yet, current research in Vision Language Models (VLMs) has not shed light on abstract-oriented language. Our research breaks new ground by uncovering its wide presence and under-estimated value, with extensive analysis. Particularly, we focus our investigation on the fashion domain, a highly-representative field with abstract expressions. By analyzing recent large-scale multimodal fashion datasets, we find that abstract terms have a dominant presence, rivaling the concrete ones, providing novel information, and being useful in the retrieval task. However, a critical challenge emerges: current general-purpose or fashion-specific VLMs are pre-trained with databases that lack sufficient abstract words in their text corpora, thus hindering their ability to effectively represent abstract-oriented language. We propose a training-free and model-agnostic method, Abstract-to-Concrete Translator (ACT), to shift abstract representations towards well-represented concrete ones in the VLM latent space, using pre-trained models and existing multimodal databases. On the text-to-image retrieval task, despite being training-free, ACT outperforms the fine-tuned VLMs in both same- and cross-dataset settings, exhibiting its effectiveness with a strong generalization capability. Moreover, the improvement introduced by ACT is consistent with various VLMs, making it a plug-and-play solution.
自然语言不仅仅是枯燥地描述视觉内容。它包含丰富的抽象概念,用于表达情感、创造力和无法直接感知的属性。然而,目前关于视觉语言模型(VLMs)的研究并没有关注抽象导向的语言。我们的研究通过深入的分析,首次揭示了其在多个领域中的广泛存在和低估的价值。特别是,我们将调查重点放在时尚领域,这是一个具有抽象表达的代表性领域。通过分析最新的大规模多模式时尚数据集,我们发现抽象术语的存在占据主导地位,与具体术语相当,提供新颖的信息,并在检索任务中很有用。但是,出现了一个关键挑战:当前的通用或特定于时尚的VLMs都是使用其文本语料库中缺乏足够抽象词的数据库进行预训练的,这阻碍了它们有效地表示抽象导向语言的能力。我们提出了一种无需训练和模型特定的方法,即抽象到具体翻译器(ACT),利用预训练模型和现有多模式数据库,将抽象表示转向在VLM潜在空间中的良好表示的具体表示。在文本到图像检索任务上,尽管ACT是无需训练的,但在同一数据集和跨数据集设置中,它的性能都优于经过微调的VLMs,显示出其强大的通用能力和有效性。此外,ACT所带来的改进与各种VLMs都是一致的,使其成为即插即用的解决方案。
论文及项目相关链接
PDF Accepted to CVPR25. Project page: https://davidetalon.github.io/fashionact-page/
Summary
本文研究了自然语言在描述视觉内容方面的丰富性,尤其是针对时尚领域中的抽象表达。研究发现在大规模时尚数据集中抽象术语普遍存在并具重要价值。然而,现有的视觉语言模型在表示抽象语言方面存在不足。为此,本文提出了一种无需训练的、模型通用的方法——抽象到具体翻译器(ACT),利用预训练模型和现有多模式数据库,将抽象表示转向VLM潜在空间中的良好表示的具体表示。在文本到图像的检索任务上,ACT表现优异,具有良好的泛化能力。
Key Takeaways
- 自然语言包含丰富的抽象概念,能够表达感觉、创造力和无法直接感知的属性。
- 在时尚领域,抽象表达具有代表性,并普遍存在。
- 大规模时尚数据集中的抽象术语与具体术语相当,为信息检索提供了新颖视角。
- 现有的视觉语言模型(VLMs)在表示抽象语言方面存在不足,因为它们通常是在缺乏足够抽象词汇的数据库中进行预训练的。
- 提出了一种无需训练的模型通用方法——抽象到具体翻译器(ACT),以改善VLMs在表示抽象语言方面的不足。
- ACT方法在文本到图像检索任务上表现优异,超越了精细调整的VLMs,在同数据集和跨数据集设置中都展现了其有效性。
点此查看论文截图
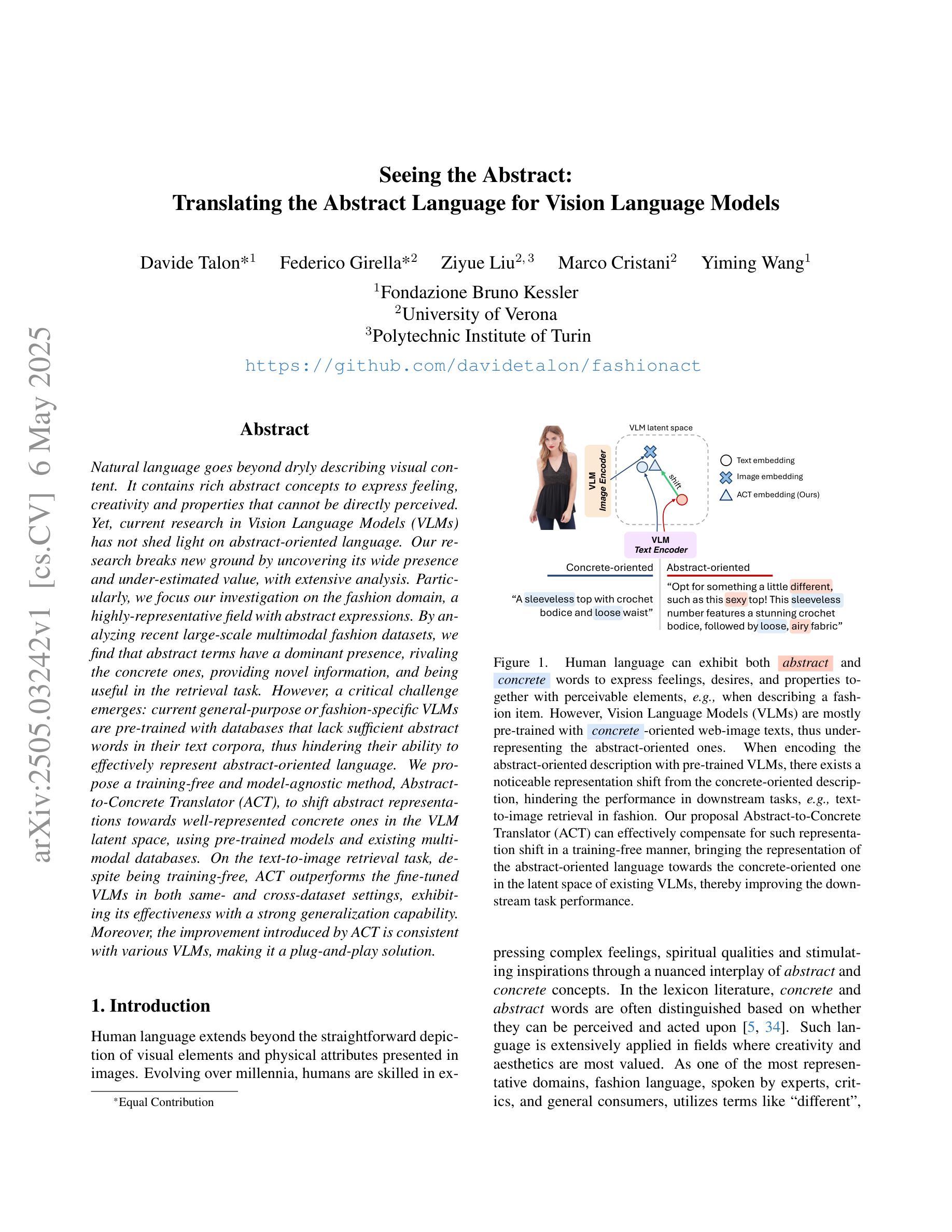
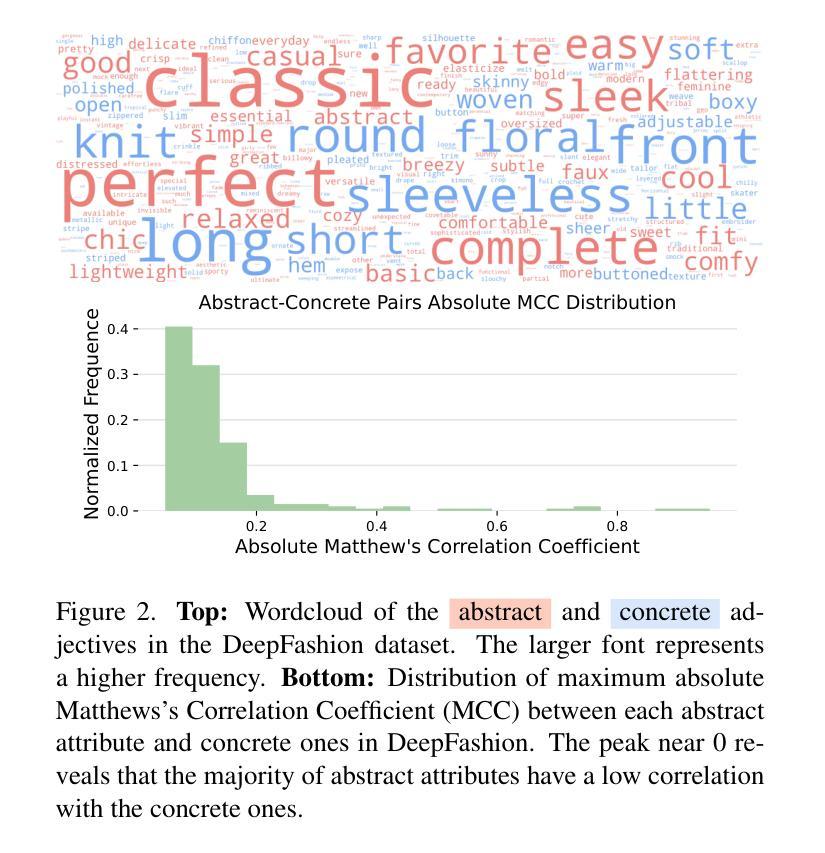
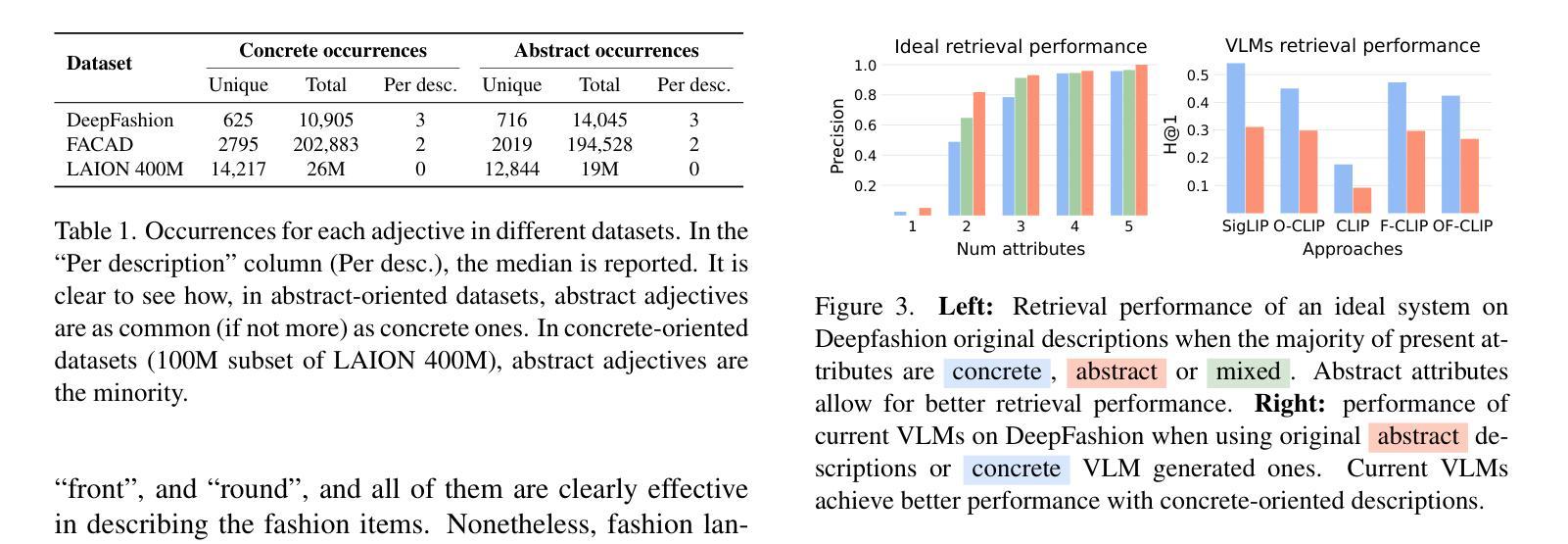
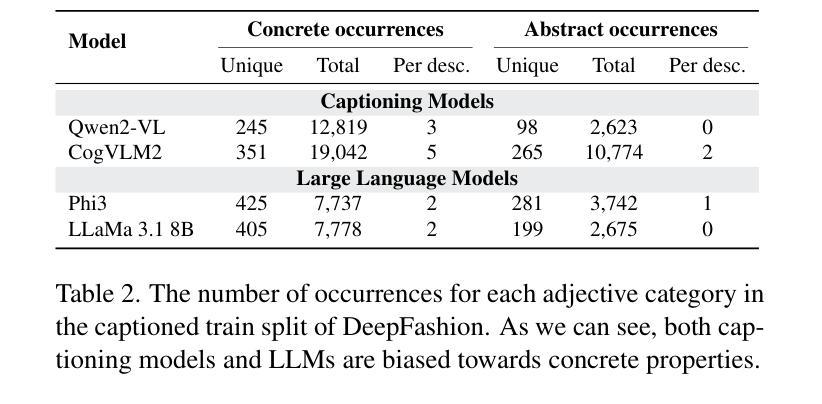
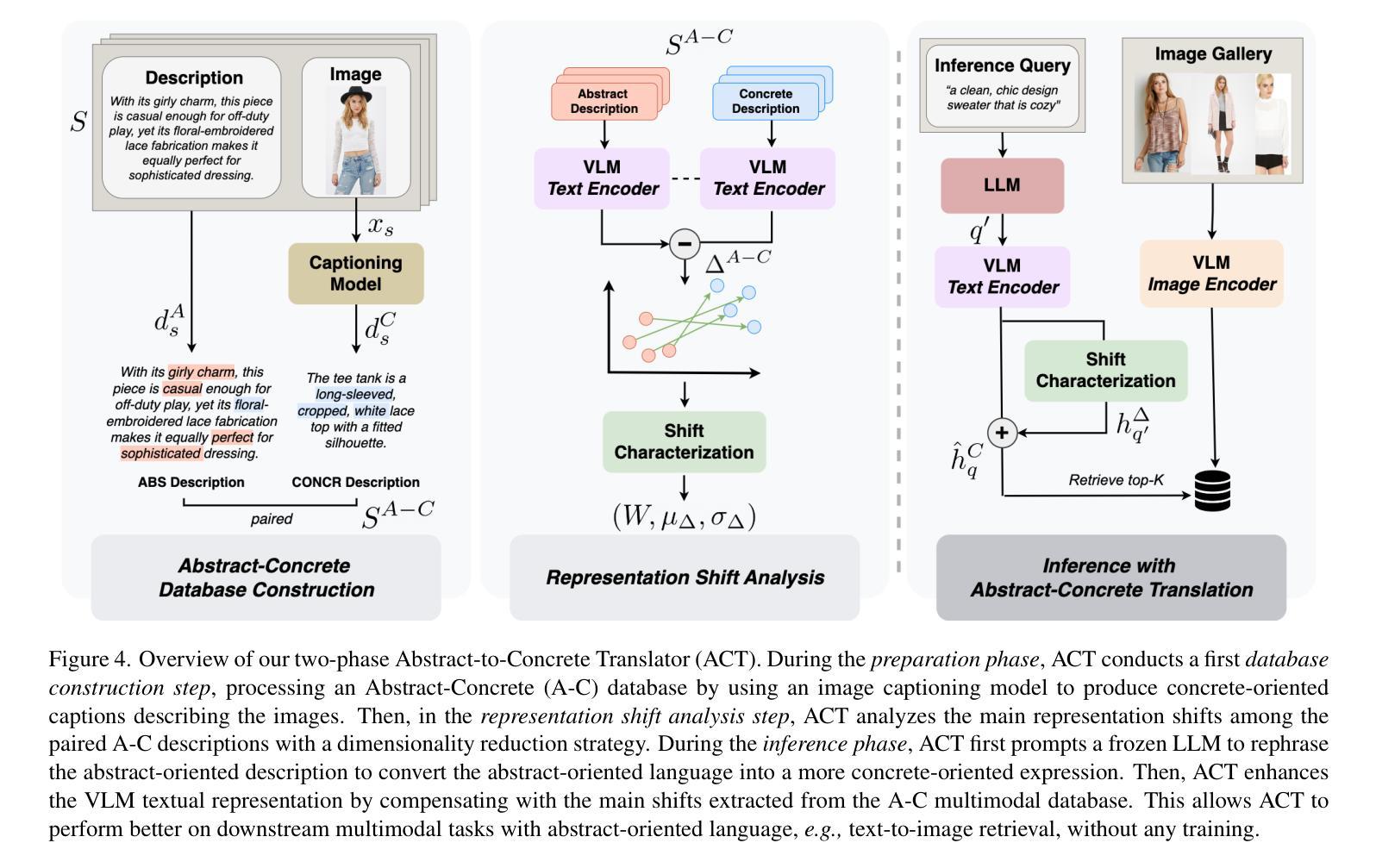
Path and Bone-Contour Regularized Unpaired MRI-to-CT Translation
Authors:Teng Zhou, Jax Luo, Yuping Sun, Yiheng Tan, Shun Yao, Nazim Haouchine, Scott Raymond
Accurate MRI-to-CT translation promises the integration of complementary imaging information without the need for additional imaging sessions. Given the practical challenges associated with acquiring paired MRI and CT scans, the development of robust methods capable of leveraging unpaired datasets is essential for advancing the MRI-to-CT translation. Current unpaired MRI-to-CT translation methods, which predominantly rely on cycle consistency and contrastive learning frameworks, frequently encounter challenges in accurately translating anatomical features that are highly discernible on CT but less distinguishable on MRI, such as bone structures. This limitation renders these approaches less suitable for applications in radiation therapy, where precise bone representation is essential for accurate treatment planning. To address this challenge, we propose a path- and bone-contour regularized approach for unpaired MRI-to-CT translation. In our method, MRI and CT images are projected to a shared latent space, where the MRI-to-CT mapping is modeled as a continuous flow governed by neural ordinary differential equations. The optimal mapping is obtained by minimizing the transition path length of the flow. To enhance the accuracy of translated bone structures, we introduce a trainable neural network to generate bone contours from MRI and implement mechanisms to directly and indirectly encourage the model to focus on bone contours and their adjacent regions. Evaluations conducted on three datasets demonstrate that our method outperforms existing unpaired MRI-to-CT translation approaches, achieving lower overall error rates. Moreover, in a downstream bone segmentation task, our approach exhibits superior performance in preserving the fidelity of bone structures. Our code is available at: https://github.com/kennysyp/PaBoT.
准确的MRI到CT转换技术能够在不需要额外的成像会话的情况下,实现互补成像信息的集成。考虑到获取配对MRI和CT扫描的实际挑战,开发能够利用未配对数据集的方法对于推进MRI到CT转换技术的发展至关重要。当前主要依赖于循环一致性和对比学习框架的非配对MRI到CT转换方法,在将MRI上不太可分辨但在CT上可清晰识别的解剖特征进行准确转换时经常面临挑战,例如骨骼结构。这一局限性使得这些方法在放射治疗中的应用不太适用,因为精确骨骼表示对于准确的治疗计划至关重要。为了应对这一挑战,我们提出了一种用于非配对MRI到CT转换的路径和骨骼轮廓正则化方法。在我们的方法中,MRI和CT图像被投影到一个共享潜在空间,其中MRI到CT的映射被建模为由神经常微分方程控制的连续流。通过最小化流的过渡路径长度来获得最佳映射。为了提高翻译后骨骼结构的准确性,我们引入了一个可训练的神经网络从MRI生成骨骼轮廓,并实施了直接和间接鼓励模型关注骨骼轮廓及其相邻区域的机制。在三个数据集上的评估表明,我们的方法优于现有的非配对MRI到CT转换方法,实现了更低的总体错误率。此外,在下游的骨骼分割任务中,我们的方法在保持骨骼结构保真度方面表现出卓越的性能。我们的代码可通过以下链接获取:https://github.com/kennysyp/PaBoT 。
论文及项目相关链接
Summary
本文介绍了一种针对非配对MRI-CT转换的新方法,解决了准确翻译在CT上可分辨但在MRI上难以区分的解剖特征(如骨骼结构)的挑战。新方法通过路径和骨骼轮廓正则化进行MRI-CT转换,利用神经常微分方程建模映射关系,并引入可训练的神经网络生成骨骼轮廓以提高翻译准确性。评估结果表明,该方法在三个数据集上的性能优于现有非配对MRI-CT转换方法,并在下游骨骼分割任务中表现出优越的性能。代码已公开在GitHub上。
Key Takeaways
- 新方法解决了MRI-CT转换中准确翻译特定解剖特征的挑战,特别是骨骼结构。
- 方法通过路径和骨骼轮廓正则化进行MRI-CT转换,映射关系通过神经常微分方程建模。
- 利用可训练的神经网络生成骨骼轮廓,提高翻译的准确性。
- 在三个数据集上的评估表明,新方法性能优于现有非配对MRI-CT转换方法。
- 下游骨骼分割任务中表现出优越性能。
点此查看论文截图
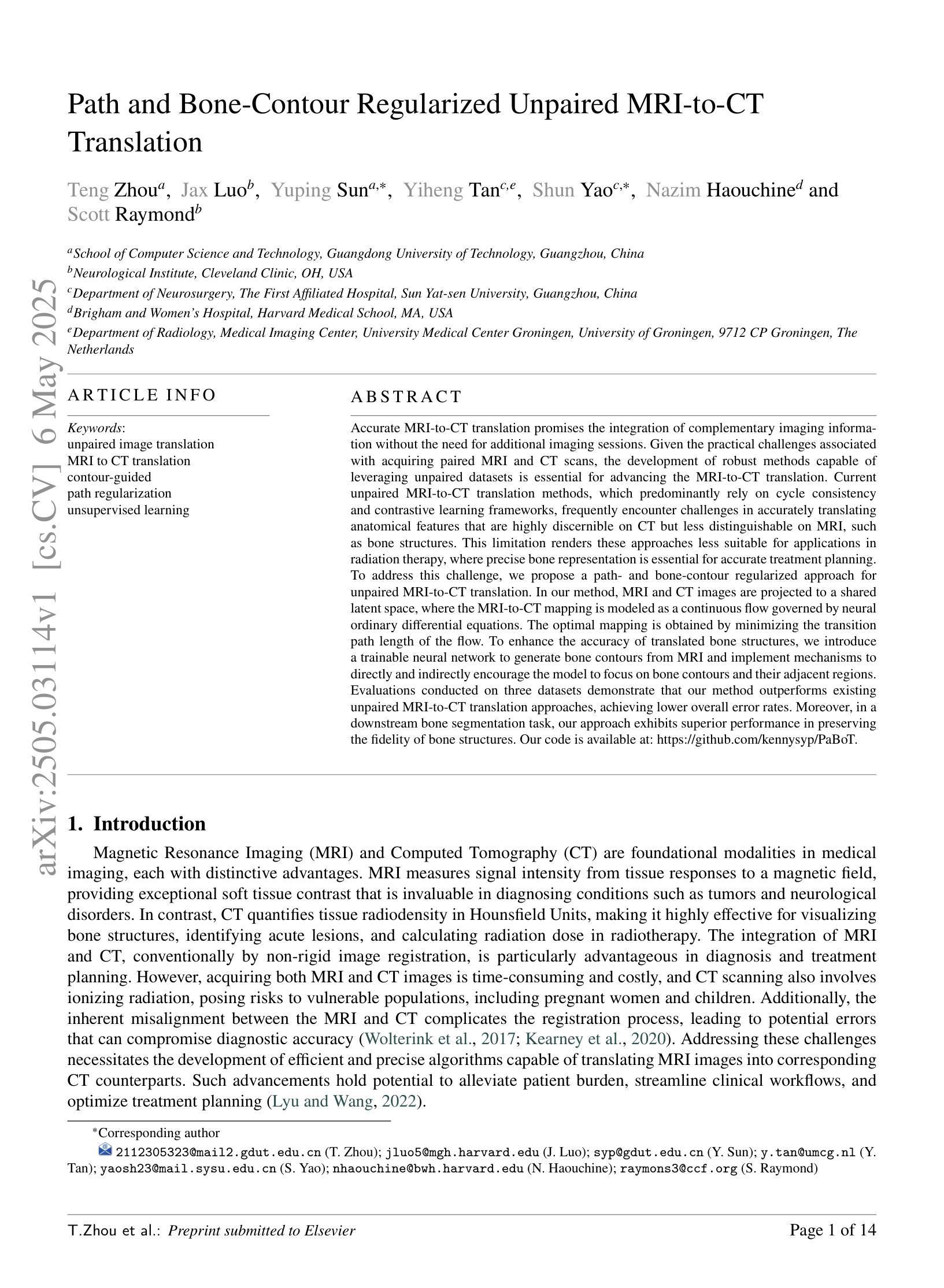
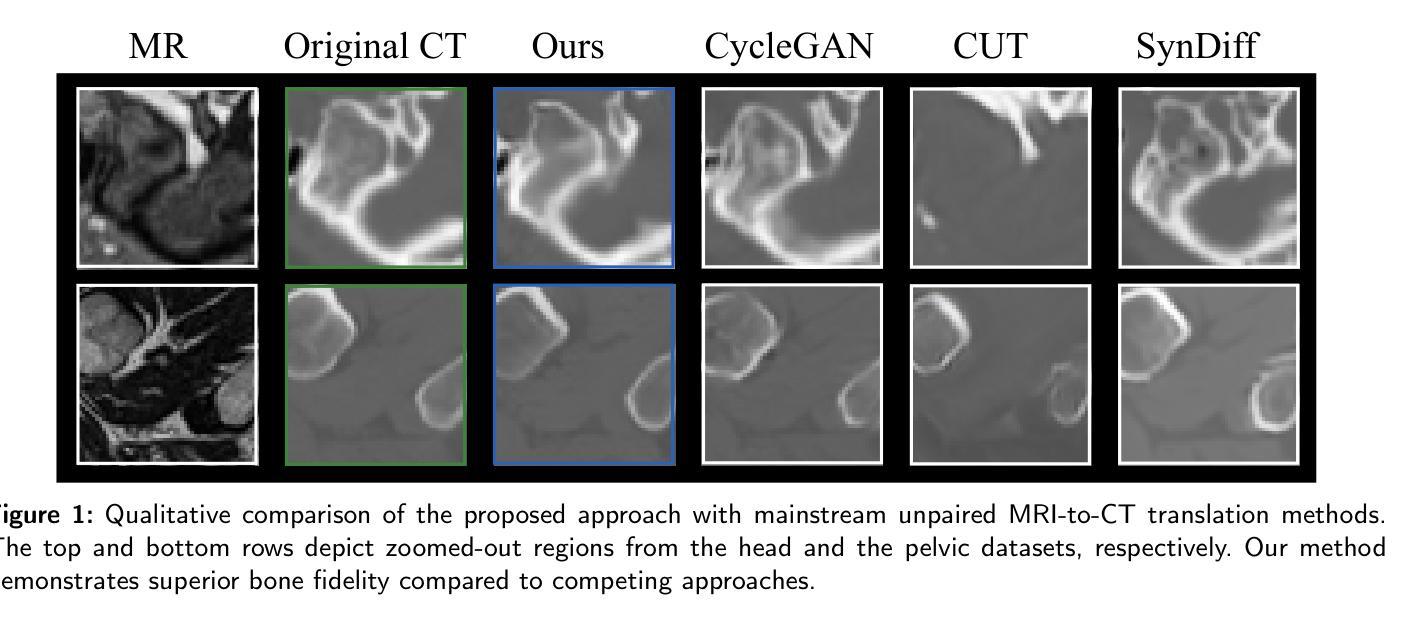
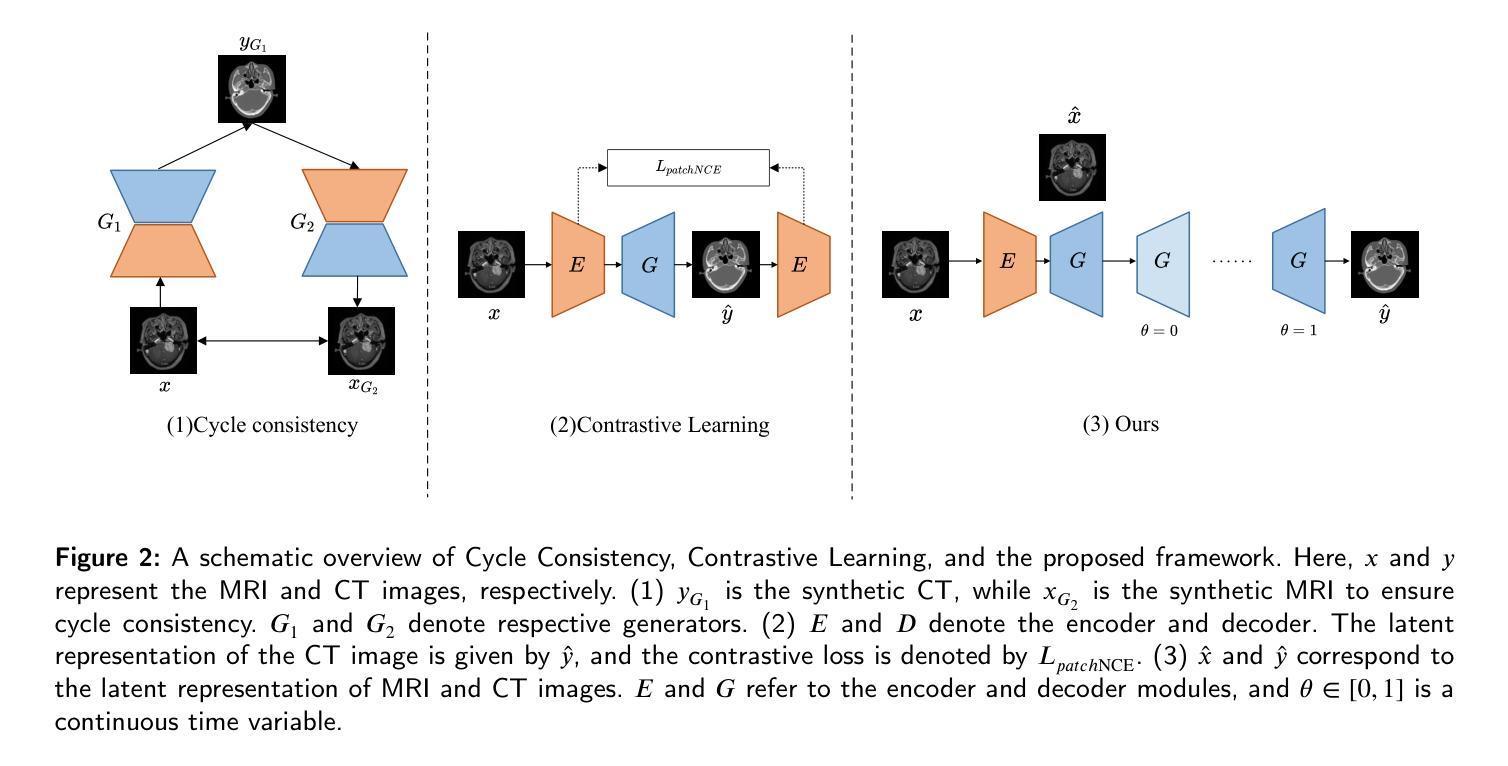
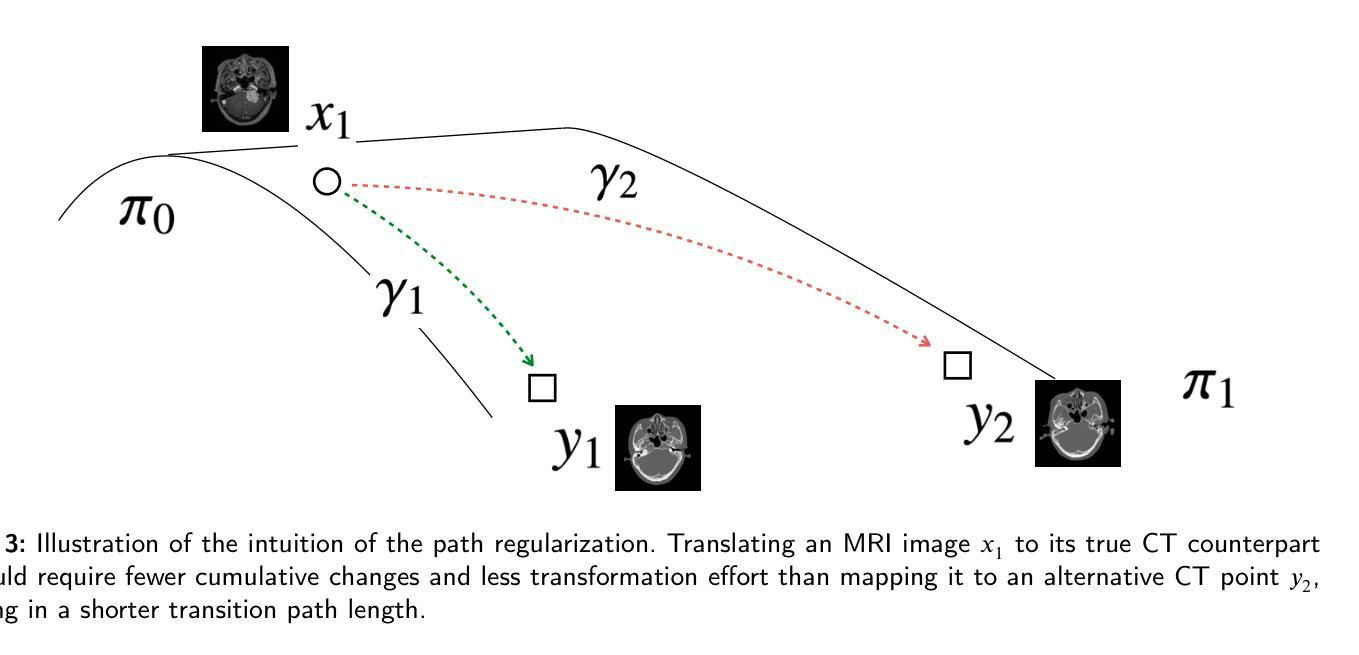
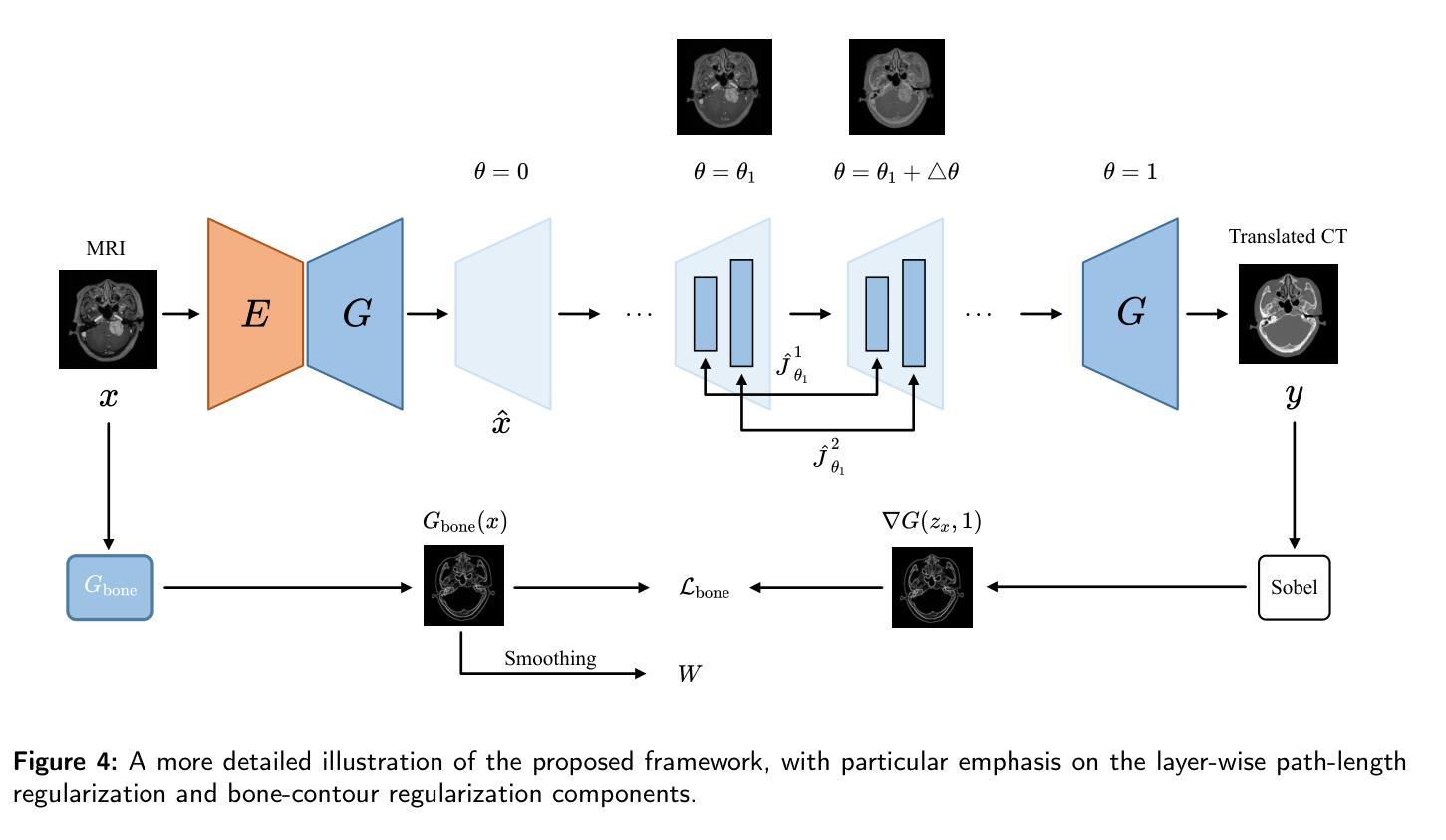
Sim2Real in endoscopy segmentation with a novel structure aware image translation
Authors:Clara Tomasini, Luis Riazuelo, Ana C. Murillo
Automatic segmentation of anatomical landmarks in endoscopic images can provide assistance to doctors and surgeons for diagnosis, treatments or medical training. However, obtaining the annotations required to train commonly used supervised learning methods is a tedious and difficult task, in particular for real images. While ground truth annotations are easier to obtain for synthetic data, models trained on such data often do not generalize well to real data. Generative approaches can add realistic texture to it, but face difficulties to maintain the structure of the original scene. The main contribution in this work is a novel image translation model that adds realistic texture to simulated endoscopic images while keeping the key scene layout information. Our approach produces realistic images in different endoscopy scenarios. We demonstrate these images can effectively be used to successfully train a model for a challenging end task without any real labeled data. In particular, we demonstrate our approach for the task of fold segmentation in colonoscopy images. Folds are key anatomical landmarks that can occlude parts of the colon mucosa and possible polyps. Our approach generates realistic images maintaining the shape and location of the original folds, after the image-style-translation, better than existing methods. We run experiments both on a novel simulated dataset for fold segmentation, and real data from the EndoMapper (EM) dataset. All our new generated data and new EM metadata is being released to facilitate further research, as no public benchmark is currently available for the task of fold segmentation.
自动分割内窥镜图像中的解剖标志可以为医生、外科医生提供诊断、治疗或医学训练方面的帮助。然而,获取训练常用监督学习方法所需的注释是一项乏味且困难的任务,尤其是对于真实图像。虽然对于合成数据而言,更容易获得真实情况的注释,但以这些数据训练的模型往往对真实数据的泛化能力不佳。生成方法可以在其上增加逼真的纹理,但在保持原始场景结构方面面临困难。这项工作的主要贡献是一种新型图像翻译模型,该模型可为模拟的内窥镜图像添加逼真的纹理,同时保留关键场景布局信息。我们的方法能够在不同的内窥镜场景中生成逼真的图像。我们证明这些图像可以有效地用于成功训练一个用于具有挑战性的终端任务的模型,无需任何真实标记数据。特别是,我们针对结肠镜图像中的折叠分割任务展示了我们的方法。折叠是关键的解剖标志,可能遮挡结肠粘膜和部分可能的息肉。我们的方法在图像风格翻译后生成了保持原始折叠形状和位置的逼真图像,优于现有方法。我们在针对折叠分割任务的新型模拟数据集和来自EndoMapper(EM)的真实数据集上进行了实验。我们发布所有新生成的数据和新的EM元数据,以促进进一步的研究,因为目前尚无针对折叠分割任务的公开基准测试集。
论文及项目相关链接
Summary:本研究开发了一种新的图像翻译模型,该模型能为模拟的内窥镜图像添加逼真的纹理,同时保留关键场景布局信息。模型生成的图像可用于训练无需真实标记数据的模型,对于结肠镜检查图像的折叠分割等任务具有良好的效果。
Key Takeaways:
- 自动分割内窥镜图像中的解剖标志可以为医生、外科医生提供诊断、治疗或医学培训的帮助。
- 获得通常用于监督学习方法的注释是非常繁琐和困难的任务,特别是对于真实图像。
- 虽然真实数据的标记注释更容易获得,但基于合成数据训练的模型通常不能很好地推广到真实数据。
- 本研究提出了一种新的图像翻译模型,该模型能够在模拟内窥镜图像上添加逼真的纹理并保持关键场景布局信息。
- 该方法生成的图像在不同内窥镜场景中都很逼真。
- 模型对于折叠分割等任务效果良好,可成功训练模型并用于执行具有挑战性的任务,无需任何真实标记数据。所生成的折叠分割数据集和新数据集公开提供,以推动相关研究的发展。对于当前的折叠分割任务尚无公开基准数据集的问题有所改善。该数据集还发布了一个附加数据集以便于进一步的探索研究。
点此查看论文截图
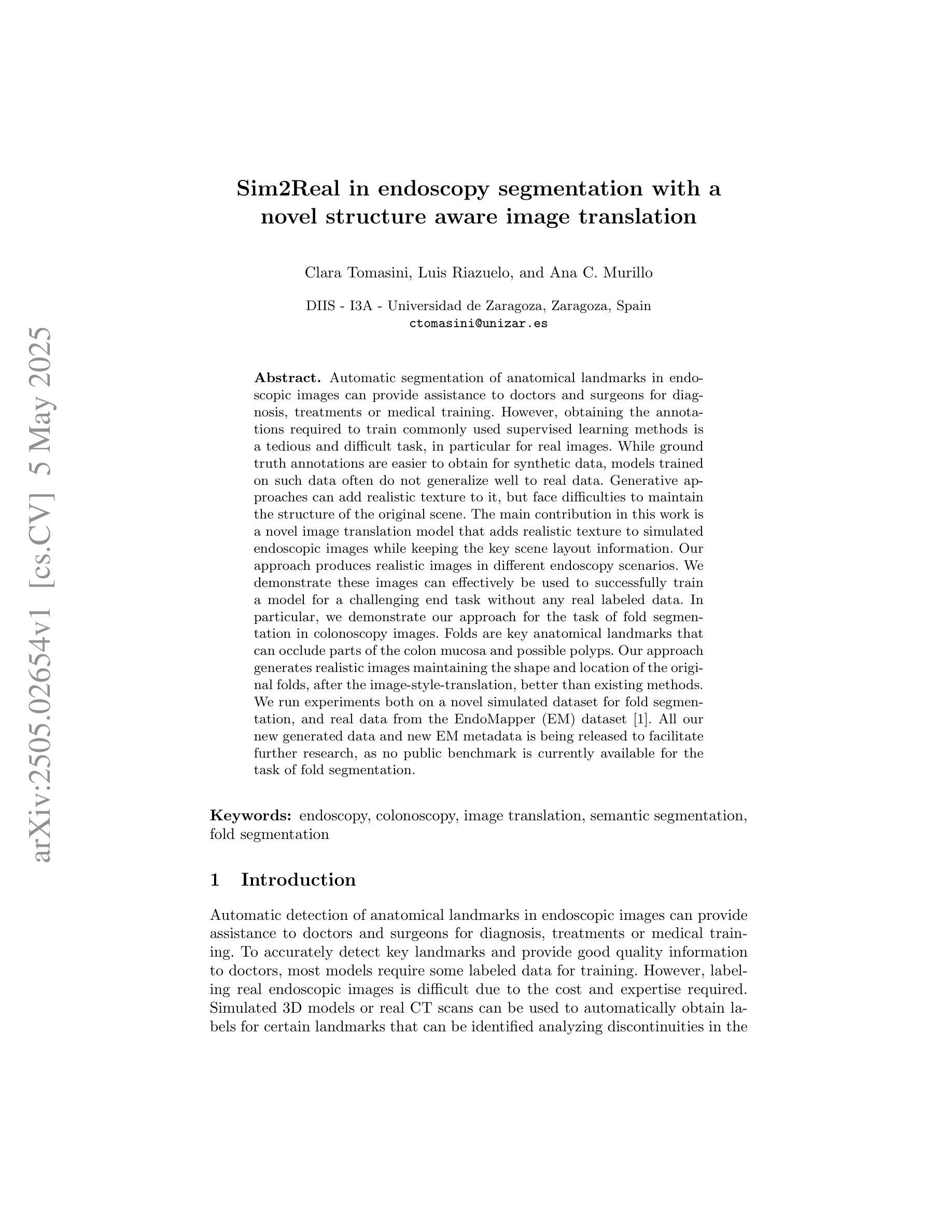
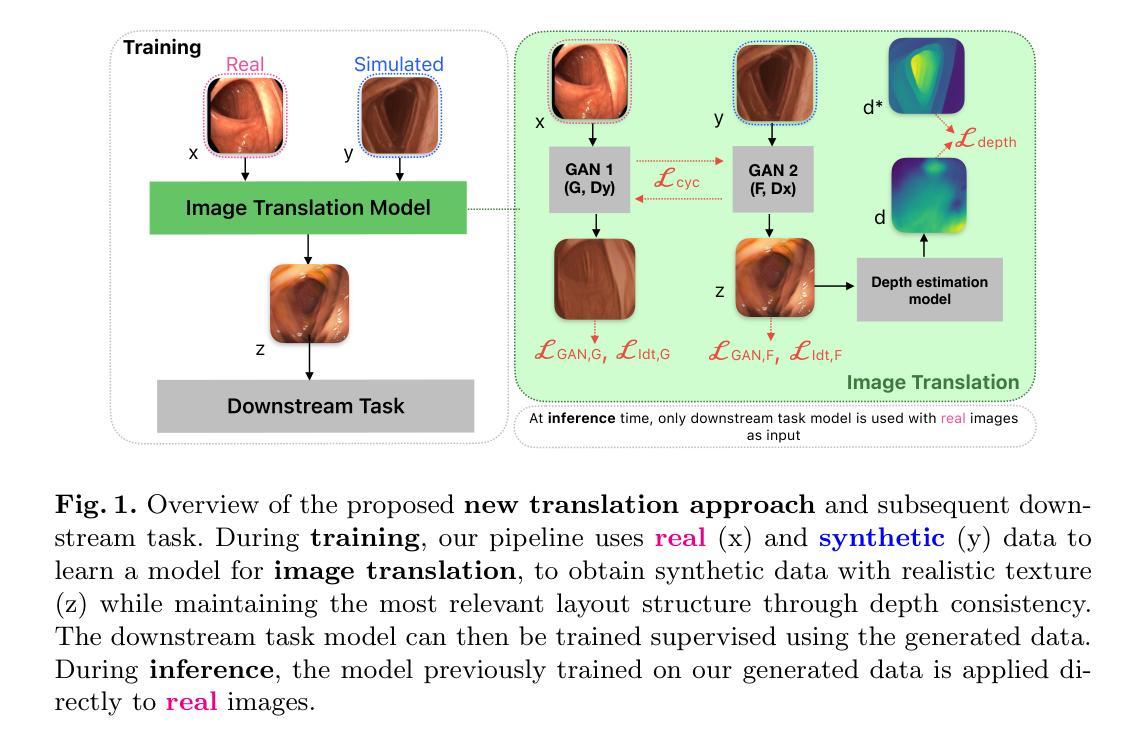
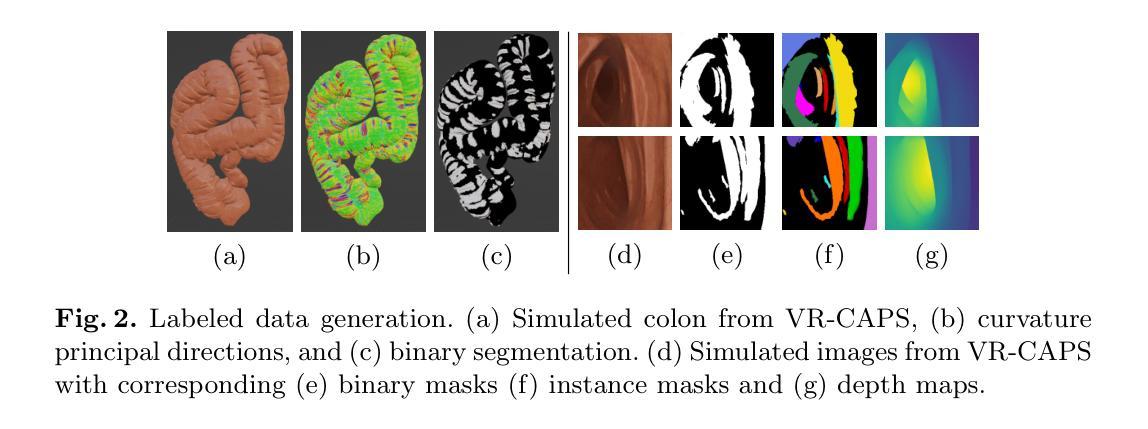
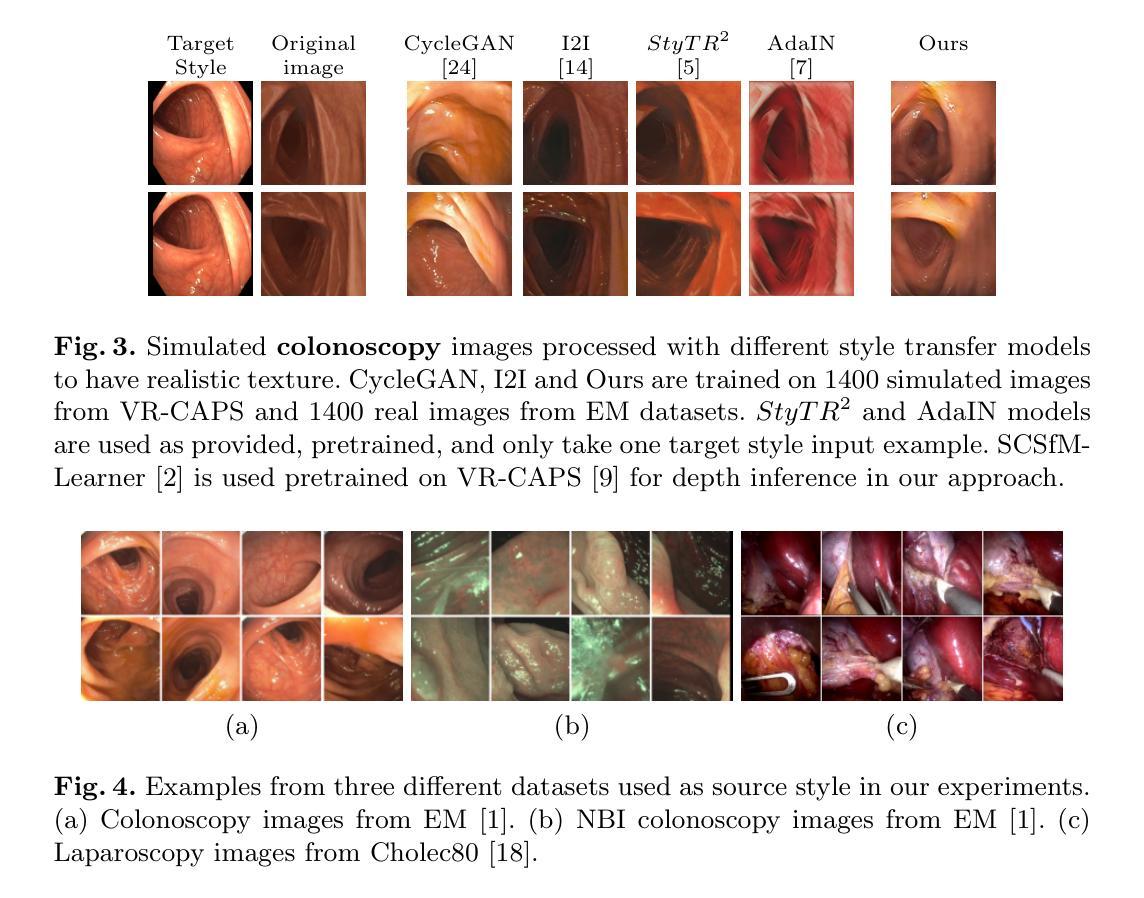
Regression is all you need for medical image translation
Authors:Sebastian Rassmann, David Kügler, Christian Ewert, Martin Reuter
The acquisition of information-rich images within a limited time budget is crucial in medical imaging. Medical image translation (MIT) can help enhance and supplement existing datasets by generating synthetic images from acquired data. While Generative Adversarial Nets (GANs) and Diffusion Models (DMs) have achieved remarkable success in natural image generation, their benefits - creativity and image realism - do not necessarily transfer to medical applications where highly accurate anatomical information is required. In fact, the imitation of acquisition noise or content hallucination hinder clinical utility. Here, we introduce YODA (You Only Denoise once - or Average), a novel 2.5D diffusion-based framework for volumetric MIT. YODA unites diffusion and regression paradigms to produce realistic or noise-free outputs. Furthermore, we propose Expectation-Approximation (ExpA) DM sampling, which draws inspiration from MRI signal averaging. ExpA-sampling suppresses generated noise and, thus, eliminates noise from biasing the evaluation of image quality. Through extensive experiments on four diverse multi-modal datasets - comprising multi-contrast brain MRI and pelvic MRI-CT - we show that diffusion and regression sampling yield similar results in practice. As such, the computational overhead of diffusion sampling does not provide systematic benefits in medical information translation. Building on these insights, we demonstrate that YODA outperforms several state-of-the-art GAN and DM methods. Notably, YODA-generated images are shown to be interchangeable with, or even superior to, physical acquisitions for several downstream tasks. Our findings challenge the presumed advantages of DMs in MIT and pave the way for the practical application of MIT in medical imaging.
在医疗成像中,在有限的时间预算内获取信息丰富的图像至关重要。医学图像翻译(MIT)可以通过从获取的数据生成合成图像来帮助增强和补充现有数据集。虽然生成对抗网络(GANs)和扩散模型(DMs)在自然图像生成方面取得了显著的成功,它们在医疗应用中的优势——创造力和图像逼真度——并不一定会带来所需的高度准确的解剖信息。事实上,对获取噪声的模仿或内容幻觉会阻碍其在临床上的实用性。在这里,我们介绍了YODA(You Only Denoise once - or Average,你只去噪一次或平均),这是一个基于体积的新型2.5D扩散式MIT框架。YODA结合了扩散和回归范式来产生逼真的或无噪声的输出。此外,我们提出了受MRI信号平均启发的期望近似(ExpA)DM采样。ExpA采样抑制生成的噪声,从而消除噪声对图像质量评估的偏见。我们在四个不同的多模式数据集上进行了大量实验,包括多对比度脑部MRI和盆腔MRI-CT,我们证明扩散和回归采样在实践中产生了类似的结果。因此,扩散采样的计算开销在医学信息翻译中并没有提供系统的优势。基于这些见解,我们证明了YODA优于几种最先进的GAN和DM方法。值得注意的是,YODA生成的图像被证明可以与多个下游任务中的实际采集图像互换,甚至在某些情况下表现更优秀。我们的研究挑战了DM在MIT中的假设优势,为MIT在医疗成像中的实际应用铺平了道路。
论文及项目相关链接
Summary
本文介绍了在医疗成像领域中,信息丰富图像的获取对时间预算的限制至关重要。医疗图像翻译(MIT)可以通过生成合成图像来增强和补充现有数据集。尽管生成对抗网络(GANs)和扩散模型(DMs)在自然图像生成方面取得了显著的成功,但它们在医疗应用中的优势——创造性和图像真实性——并不一定能够发挥,因为医疗应用需要高度准确的解剖信息。本文提出了一种新型的2.5D扩散基于体积的MIT框架YODA,它将扩散和回归范式结合起来产生现实或去噪输出。此外,还提出了期望近似(ExpA)DM采样,它受到MRI信号平均的启发,能够抑制生成的噪声,从而消除噪声对图像质量评估的偏见。实验表明,YODA在某些任务上表现出超越先进GAN和DM方法的效果,生成的图像可与物理采集互换甚至更胜一筹。本文的发现挑战了DM在MIT中的假设优势,为MIT在医疗成像中的实际应用铺平了道路。
Key Takeaways
- 医疗图像翻译(MIT)对于增强和补充现有医疗数据集具有重要意义。
- 尽管GANs和DMs在自然图像生成方面表现出色,但它们不一定适用于需要高度准确解剖信息的医疗应用。
- 新提出的YODA框架结合了扩散和回归范式,用于生成真实的或去噪的医疗图像。
- ExpA-sampling能够抑制生成的噪声,提高图像质量评估的准确性。
- YODA在某些任务上的表现超越了现有的GAN和DM方法。
- YODA生成的图像可以与物理采集的图像互换,甚至在某些任务上表现更好。
点此查看论文截图
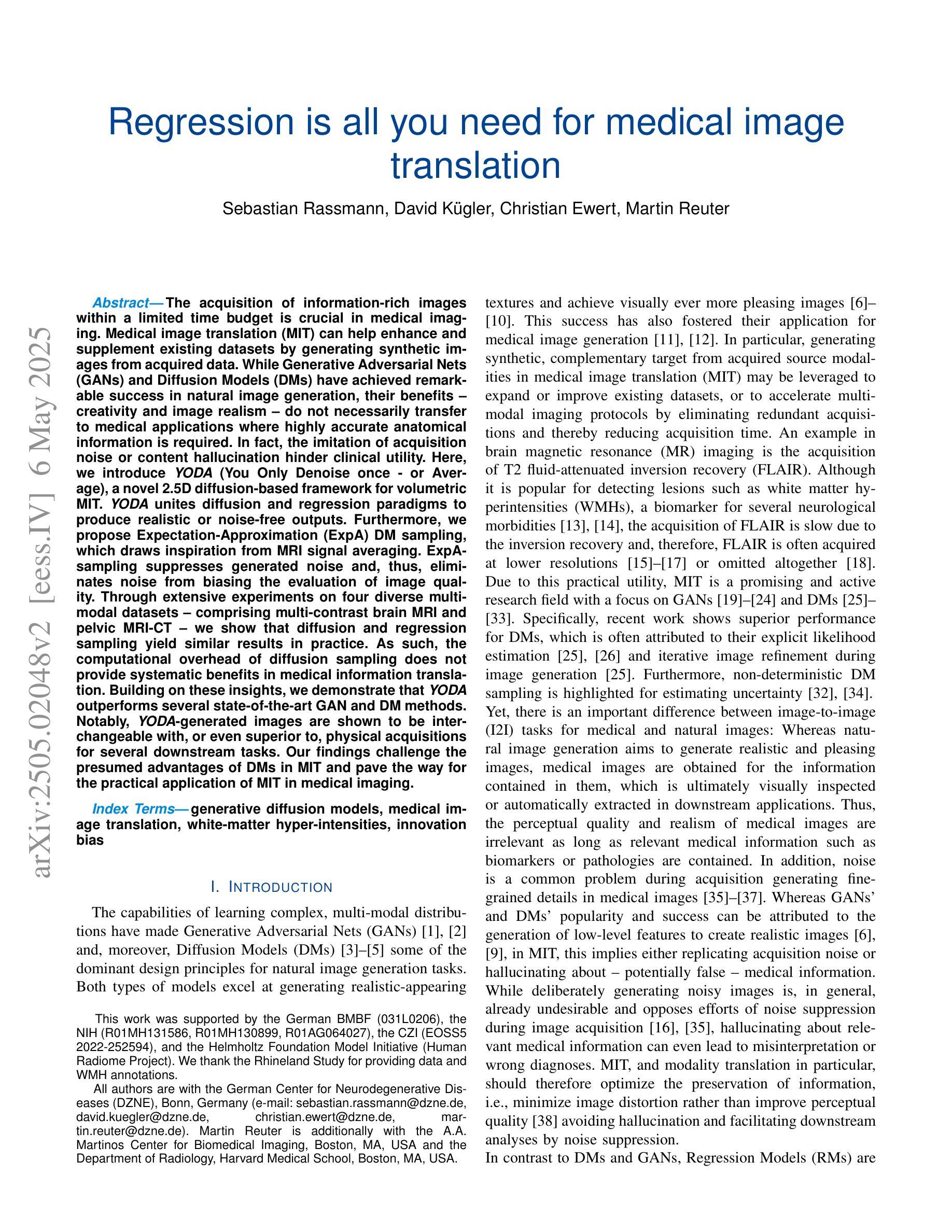
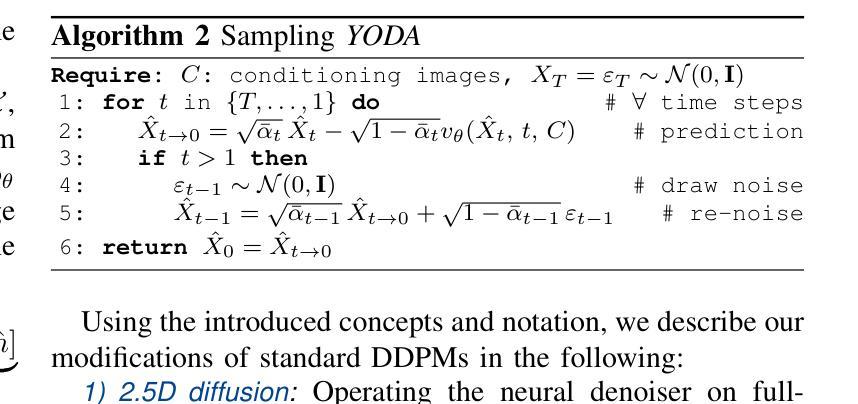
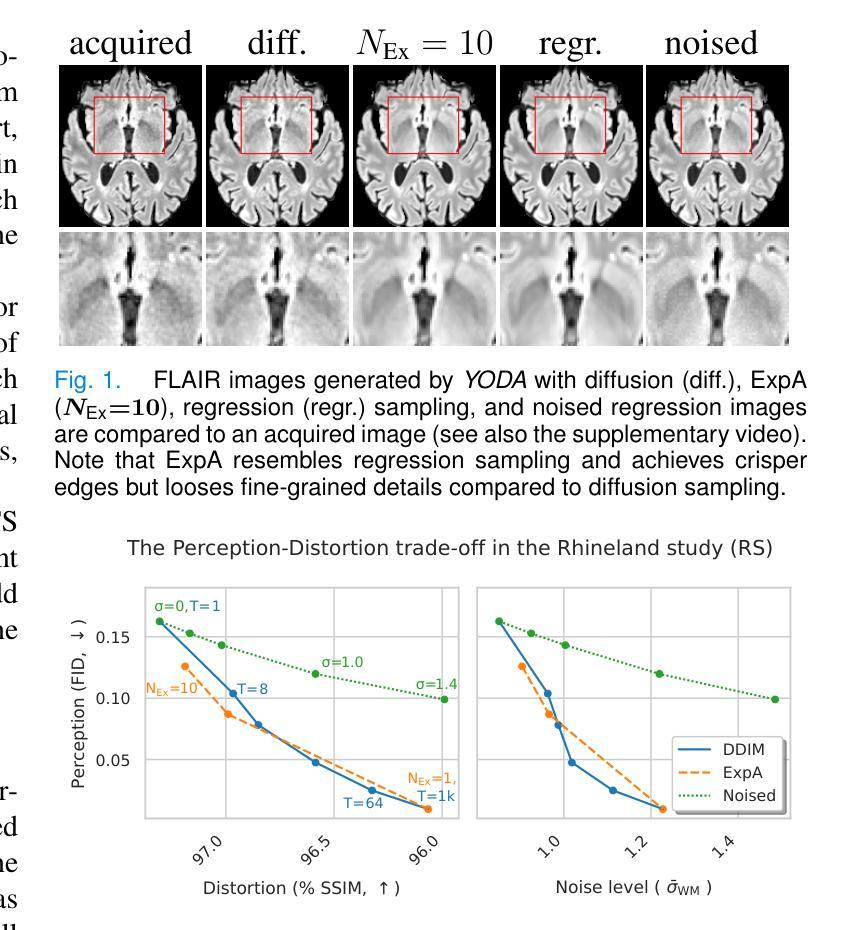
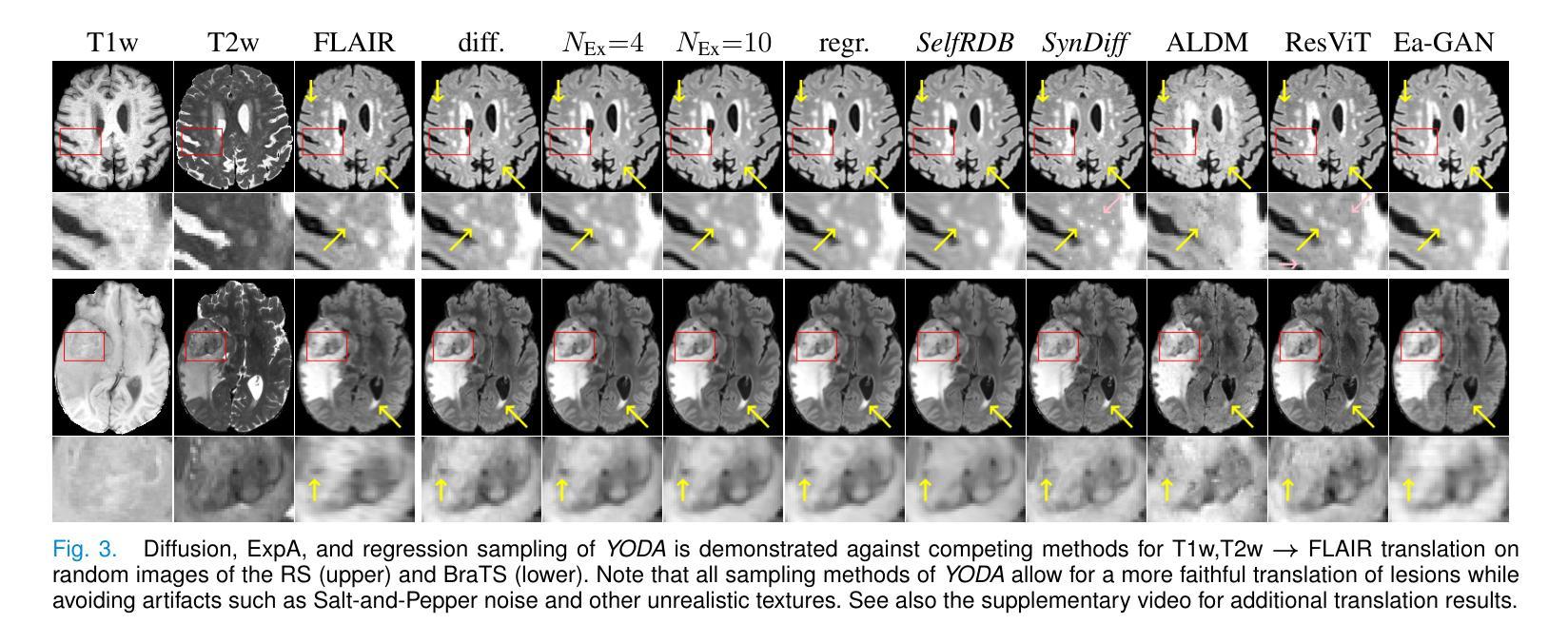
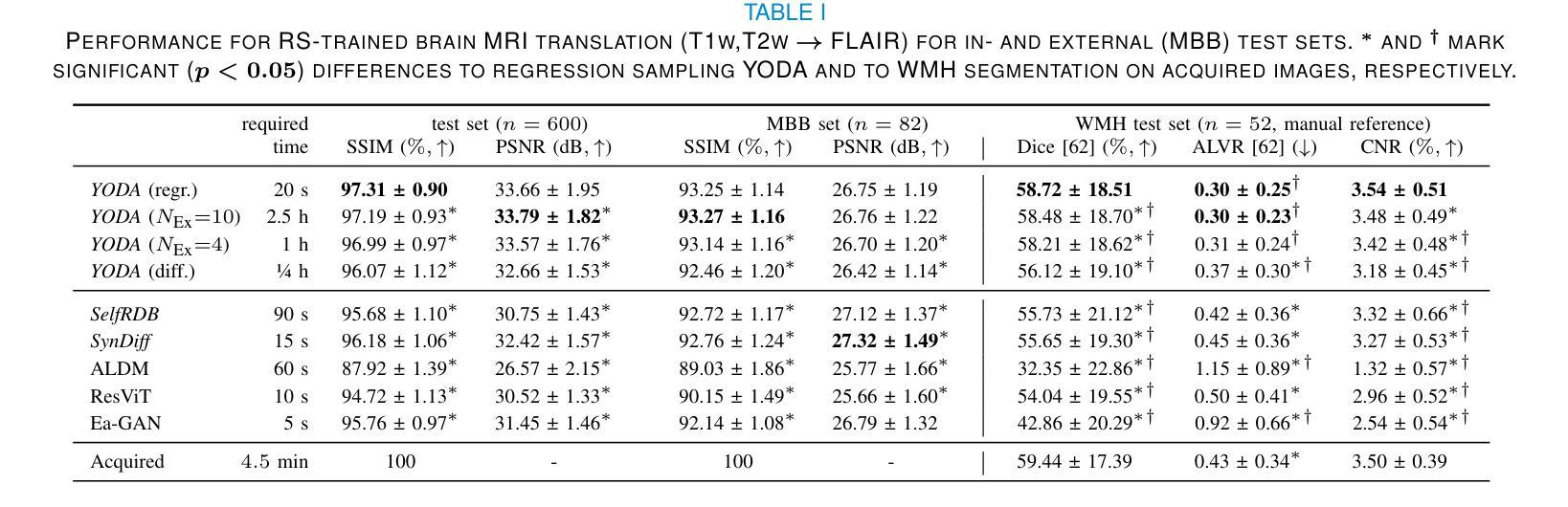
Mind2Matter: Creating 3D Models from EEG Signals
Authors:Xia Deng, Shen Chen, Jiale Zhou, Lei Li
The reconstruction of 3D objects from brain signals has gained significant attention in brain-computer interface (BCI) research. Current research predominantly utilizes functional magnetic resonance imaging (fMRI) for 3D reconstruction tasks due to its excellent spatial resolution. Nevertheless, the clinical utility of fMRI is limited by its prohibitive costs and inability to support real-time operations. In comparison, electroencephalography (EEG) presents distinct advantages as an affordable, non-invasive, and mobile solution for real-time brain-computer interaction systems. While recent advances in deep learning have enabled remarkable progress in image generation from neural data, decoding EEG signals into structured 3D representations remains largely unexplored. In this paper, we propose a novel framework that translates EEG recordings into 3D object reconstructions by leveraging neural decoding techniques and generative models. Our approach involves training an EEG encoder to extract spatiotemporal visual features, fine-tuning a large language model to interpret these features into descriptive multimodal outputs, and leveraging generative 3D Gaussians with layout-guided control to synthesize the final 3D structures. Experiments demonstrate that our model captures salient geometric and semantic features, paving the way for applications in brain-computer interfaces (BCIs), virtual reality, and neuroprosthetics. Our code is available in https://github.com/sddwwww/Mind2Matter.
大脑信号重建三维物体在脑机接口(BCI)研究中受到广泛关注。目前的研究主要利用功能性磁共振成像(fMRI)进行三维重建任务,因为其具有出色的空间分辨率。然而,fMRI的临床应用受限于其高昂的成本和不支持实时操作。相比之下,脑电图(EEG)作为实时脑机交互系统的经济、无创、可移动解决方案,具有明显优势。虽然深度学习领域的最新进展在神经数据生成图像方面取得了显著进展,但将EEG信号解码为结构化三维表示仍待探索。在本文中,我们提出了一种新型框架,利用神经解码技术和生成模型将EEG记录转化为三维物体重建。我们的方法包括训练EEG编码器以提取时空视觉特征,微调大型语言模型以将这些特征解释为描述性多模式输出,并利用布局指导控制的生成三维高斯分布来合成最终的三维结构。实验表明,我们的模型捕捉了显著的几何和语义特征,为脑机接口(BCI)、虚拟现实和神经仿生器件的应用开辟了道路。我们的代码可通过以下网址获取:https://github.com/sddwwww/Mind2Matter 。
论文及项目相关链接
Summary
基于脑电信号进行三维物体重建的研究在脑机接口领域受到广泛关注。当前研究主要利用功能磁共振成像进行三维重建,但其高昂成本和无法实现实时操作限制了临床应用。相较之下,脑电图具有经济、无创、适用于实时脑机交互系统的优势。本文提出一种利用神经解码技术和生成模型将脑电图转化为三维物体重建的新框架,包括训练脑电图编码器提取时空视觉特征、微调大型语言模型以解释这些特征并生成多模式输出,以及利用布局引导控制的生成三维高斯合成最终三维结构。实验证明,该模型能够捕捉显著的几何和语义特征,为脑机接口、虚拟现实和神经仿生学等领域的应用开辟了道路。
Key Takeaways
- 脑电信号用于重建三维物体在脑机接口领域受到关注。
- 当前主要使用功能磁共振成像进行三维重建,但其成本高且无法实现实时操作。
- 脑电图作为一种经济、无创、适用于实时脑机交互系统的技术具有优势。
- 本文提出一种基于神经解码技术和生成模型的框架,将脑电图转化为三维物体重建。
- 该框架包括训练脑电图编码器、微调语言模型以及利用生成模型合成三维结构。
- 实验证明该模型能够捕捉显著的几何和语义特征。
- 该技术为脑机接口、虚拟现实和神经仿生学等领域的应用提供了新思路。
点此查看论文截图
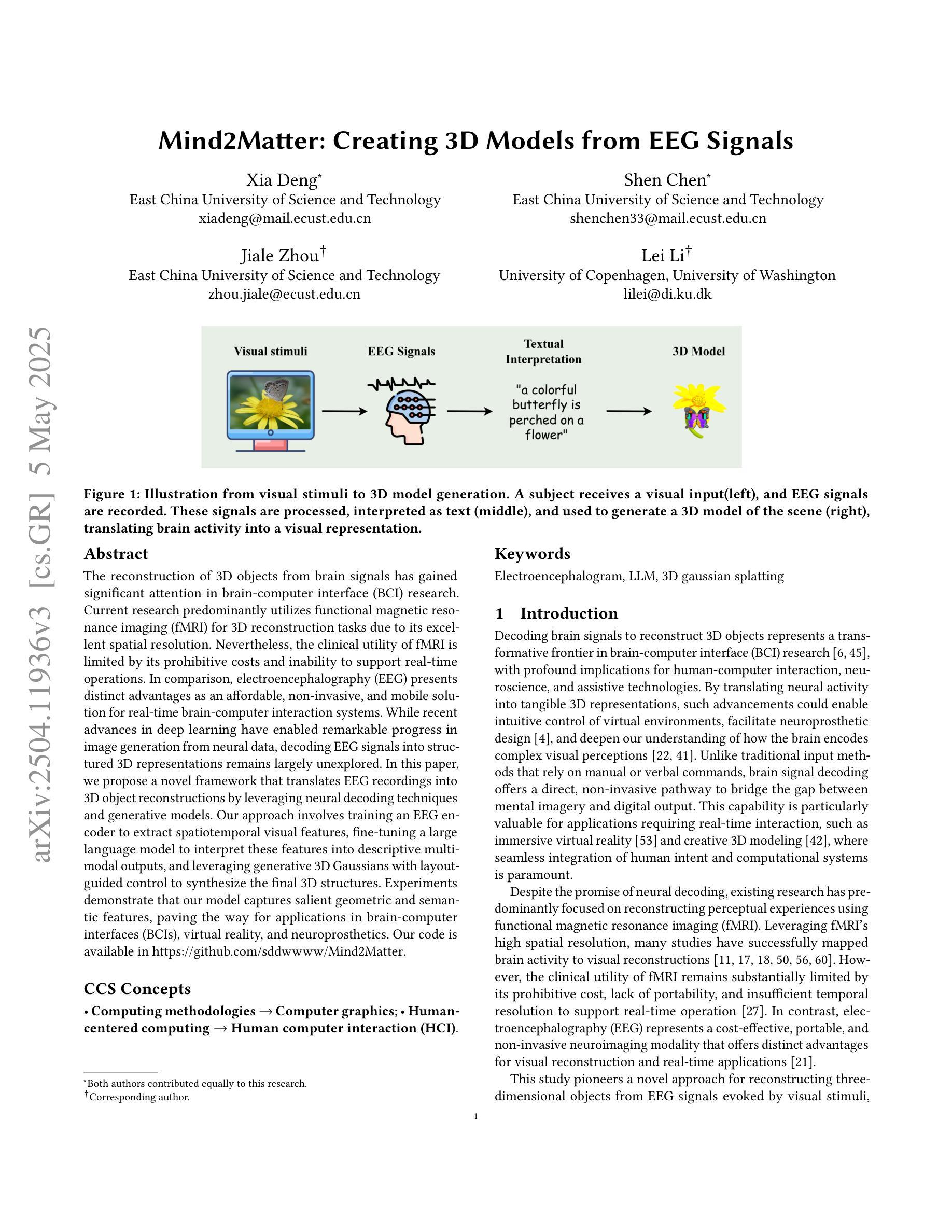
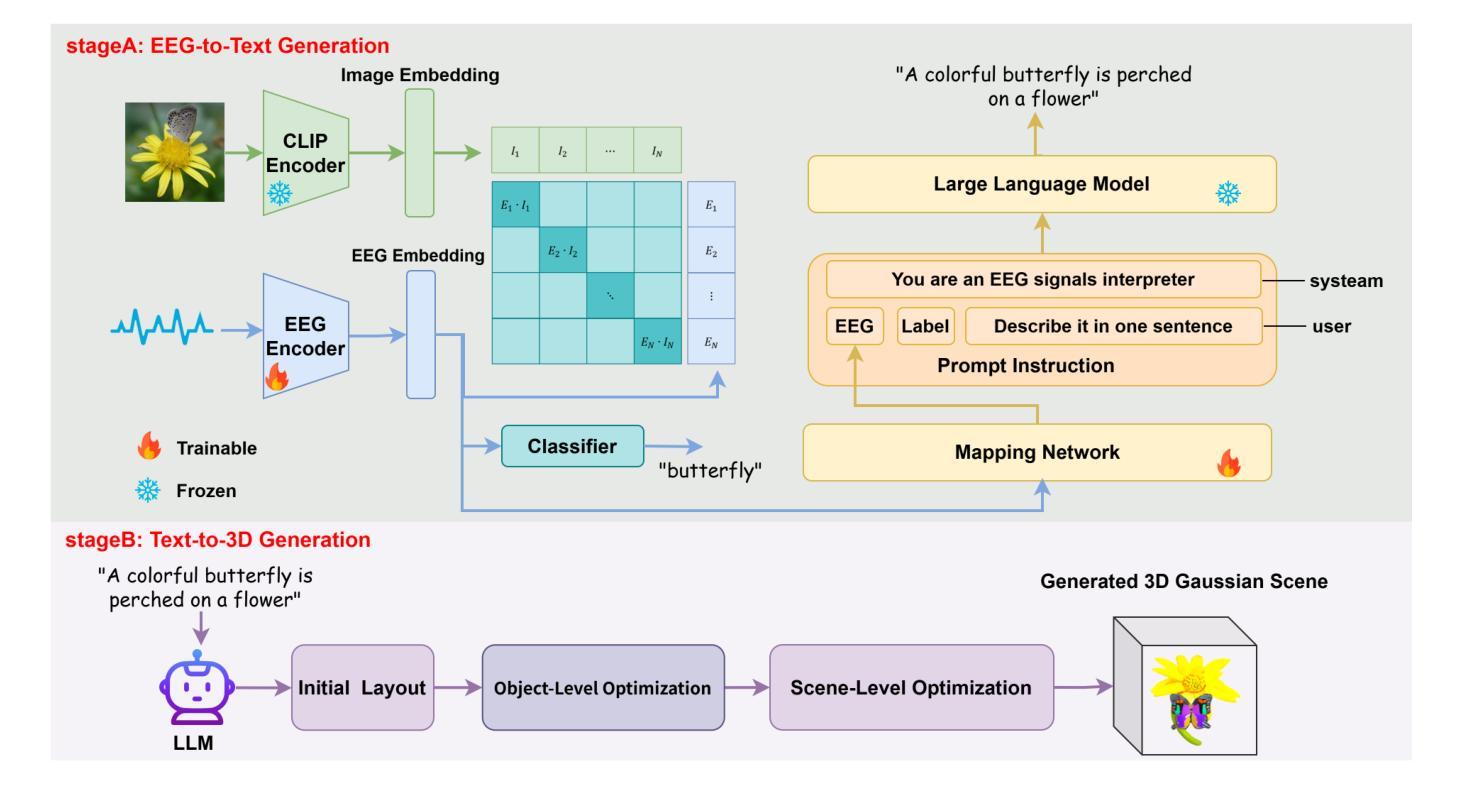
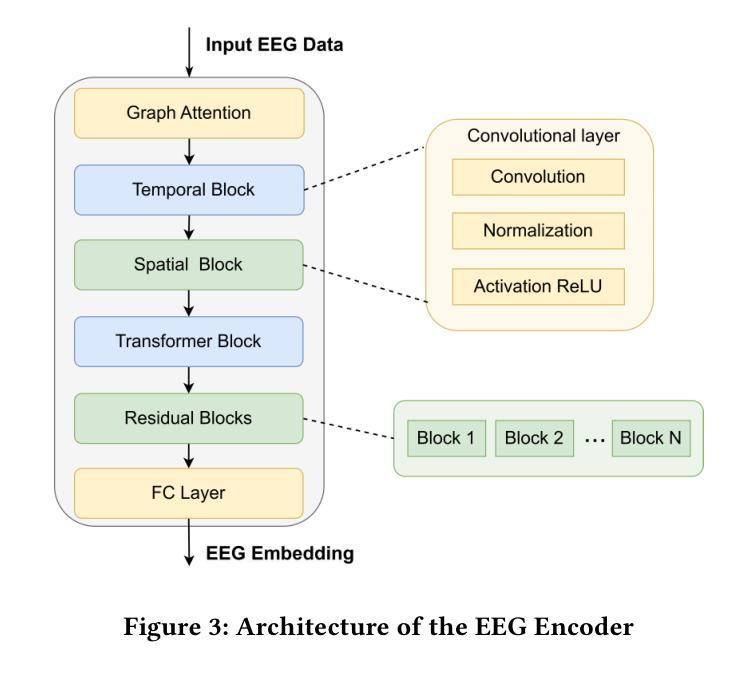
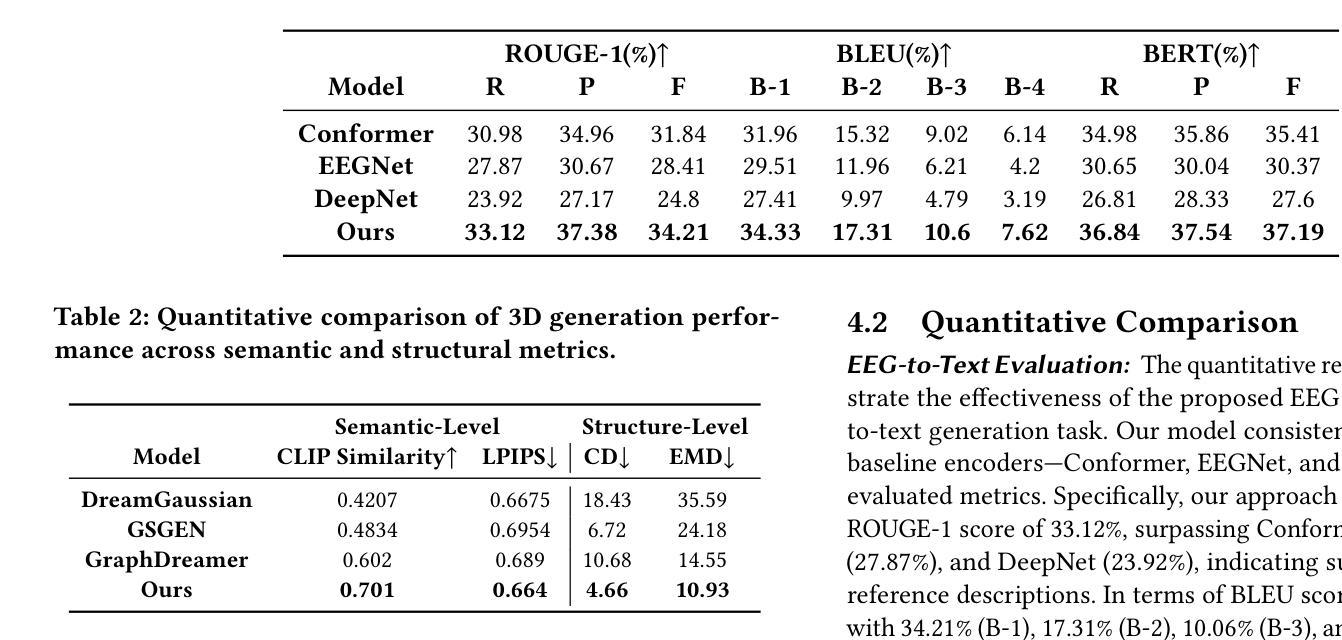