⚠️ 以下所有内容总结都来自于 大语言模型的能力,如有错误,仅供参考,谨慎使用
🔴 请注意:千万不要用于严肃的学术场景,只能用于论文阅读前的初筛!
💗 如果您觉得我们的项目对您有帮助 ChatPaperFree ,还请您给我们一些鼓励!⭐️ HuggingFace免费体验
2025-05-08 更新
A Typology of Synthetic Datasets for Dialogue Processing in Clinical Contexts
Authors:Steven Bedrick, A. Seza Doğruöz, Sergiu Nisioi
Synthetic data sets are used across linguistic domains and NLP tasks, particularly in scenarios where authentic data is limited (or even non-existent). One such domain is that of clinical (healthcare) contexts, where there exist significant and long-standing challenges (e.g., privacy, anonymization, and data governance) which have led to the development of an increasing number of synthetic datasets. One increasingly important category of clinical dataset is that of clinical dialogues which are especially sensitive and difficult to collect, and as such are commonly synthesized. While such synthetic datasets have been shown to be sufficient in some situations, little theory exists to inform how they may be best used and generalized to new applications. In this paper, we provide an overview of how synthetic datasets are created, evaluated and being used for dialogue related tasks in the medical domain. Additionally, we propose a novel typology for use in classifying types and degrees of data synthesis, to facilitate comparison and evaluation.
合成数据集被广泛应用于各种语言领域和NLP任务,特别是在真实数据有限(或根本不存在)的场景中。其中一个这样的领域是临床(医疗)环境,这里存在长期且重大的挑战(例如隐私、匿名化和数据治理),这导致了合成数据集的数量不断增加。临床数据集中越来越重要的一类是临床对话数据,这些对话数据尤其敏感且难以收集,因此通常会被合成。虽然这些合成数据集在某些情况下已被证明是足够的,但关于如何最好地将其应用于新应用的理论却很少。在本文中,我们概述了如何在医疗领域中为对话相关任务创建、评估和使用合成数据集。此外,我们提出了一种新型分类法,用于对合成数据的类型和程度进行分类,以便于比较和评估。
论文及项目相关链接
Summary
医学领域,特别是在临床语境下,合成数据集被广泛应用于对话相关任务。尽管合成数据集在某些情况下足够使用,但关于如何最佳使用和将其推广到新的应用的理论仍缺乏。本文介绍了合成数据集在医疗领域的创建、评估和使用方法,并提出了一种新的分类和评估类型的数据合成类型,以供参考和比较。
Key Takeaways
- 合成数据集广泛应用于医学领域的对话相关任务。
- 临床语境下的数据收集存在显著挑战,如隐私、匿名化和数据治理问题。
- 合成数据集的发展解决了真实数据难以获取的问题。
- 合成数据集在某些情况下足够使用,但缺乏最佳使用和推广的理论指导。
- 本文介绍了合成数据集的创建、评估和使用方法。
点此查看论文截图
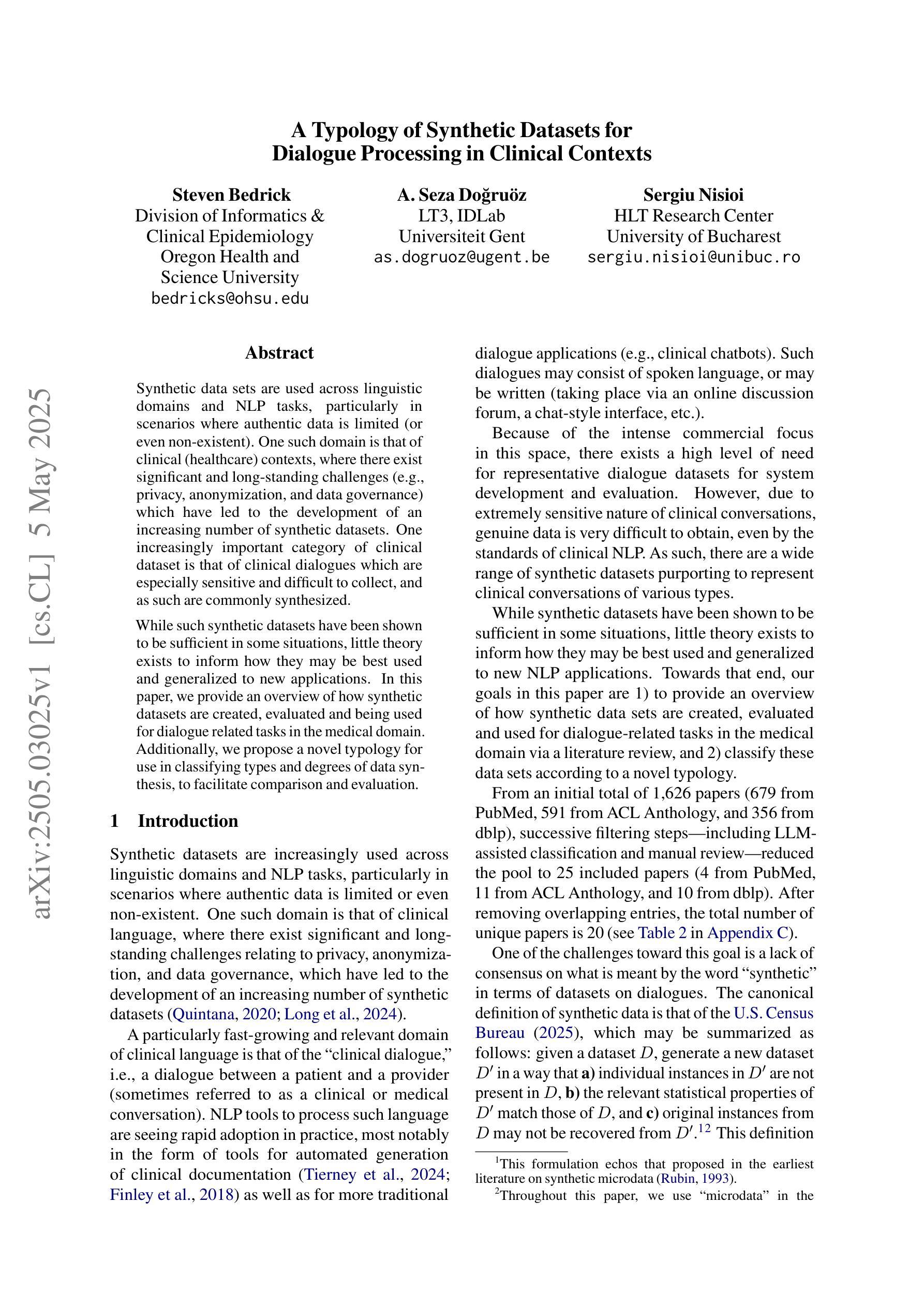
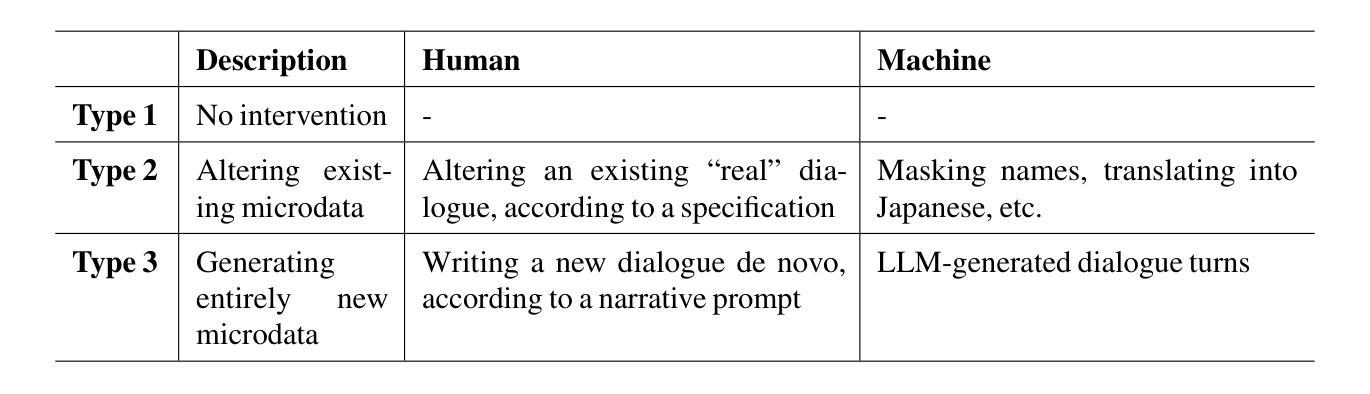
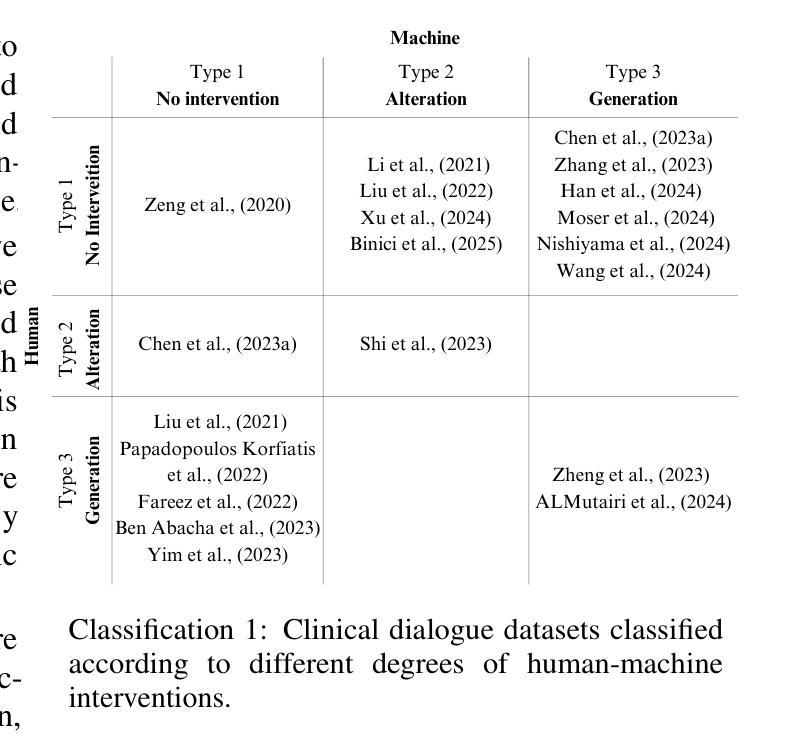
Modeling the Impact of Group Interactions on Climate-related Opinion Change in Reddit
Authors:Alessia Antelmi, Carmine Spagnuolo, Luca Maria Aiello
Opinion dynamics models describe the evolution of behavioral changes within social networks and are essential for informing strategies aimed at fostering positive collective changes, such as climate action initiatives. When applied to social media interactions, these models typically represent social exchanges in a dyadic format to allow for a convenient encoding of interactions into a graph where edges represent the flow of information from one individual to another. However, this structural assumption fails to adequately reflect the nature of group discussions prevalent on many social media platforms. To address this limitation, we present a temporal hypergraph model that effectively captures the group dynamics inherent in conversational threads, and we apply it to discussions about climate change on Reddit. This model predicts temporal shifts in stance towards climate issues at the level of individual users. In contrast to traditional studies in opinion dynamics that typically rely on simulations or limited empirical validation, our approach is tested against a comprehensive ground truth estimated by a large language model at the level of individual user comments. Our findings demonstrate that using hypergraphs to model group interactions yields superior predictions of the microscopic dynamics of opinion formation, compared to state-of-the-art models based on dyadic interactions. Although our research contributes to the understanding of these complex social systems, significant challenges remain in capturing the nuances of how opinions are formed and evolve within online spaces.
观点动态模型描述社会网络中行为变化的过程,对于制定旨在促进积极集体变化的策略(如气候行动倡议)至关重要。当应用于社交媒体互动时,这些模型通常以二元形式代表社会交流,便于将互动编码成图形,其中边缘代表信息从一个个体流向另一个个体。然而,这一结构假设未能充分反映许多社交媒体平台上普遍存在的群体讨论的性质。为了解决这个问题,我们提出了一个时间超图模型,该模型有效地捕捉了话题线程中的群体动态,并将其应用于Reddit上关于气候变化的讨论。该模型预测了个人用户对气候问题立场的时间变化。与传统的观点动态研究相比,后者通常依赖于模拟或有限的实证验证,我们的方法通过个人用户评论级别的大型语言模型进行全面真实的评估。我们的研究发现,与基于二元互动的先进模型相比,使用超图对群体互动进行建模在预测微观意见形成动力学方面具有更好的预测性。尽管我们的研究有助于理解这些复杂的社交系统,但在捕捉在线空间内意见形成和演变细微差别方面仍存在重大挑战。
论文及项目相关链接
Summary
本文介绍了意见动态模型在描述社会网络内行为变化演进中的重要性,特别是在推动气候行动等积极集体变化方面的策略价值。针对社交媒体互动的传统模型在反映群体讨论方面的局限性,提出了一种基于时序超图的模型,有效捕捉讨论线程中的群体动态,并应用于Reddit上的气候变化讨论。该模型能够预测个体用户对气候问题立场的时间变化。与传统意见动态研究相比,该研究通过大型语言模型对个体用户评论的细致分析进行了验证,表明基于群体互动的超图模型在预测意见形成的微观动态方面具有优越性。尽管存在挑战,但该研究为理解复杂社会系统提供了贡献。
Key Takeaways
- 意见动态模型描述社会网络内行为变化的演进,对推动集体变化策略至关重要。
- 传统模型在反映社交媒体上的群体讨论方面存在局限性。
- 提出的时序超图模型有效捕捉讨论线程中的群体动态。
- 模型应用于Reddit上的气候变化讨论,能预测个体用户对气候问题的立场变化。
- 与传统意见动态研究相比,该研究在预测微观动态方面具有优越性。
- 大型语言模型用于分析个体用户评论,提供了更为准确的实证验证。
点此查看论文截图
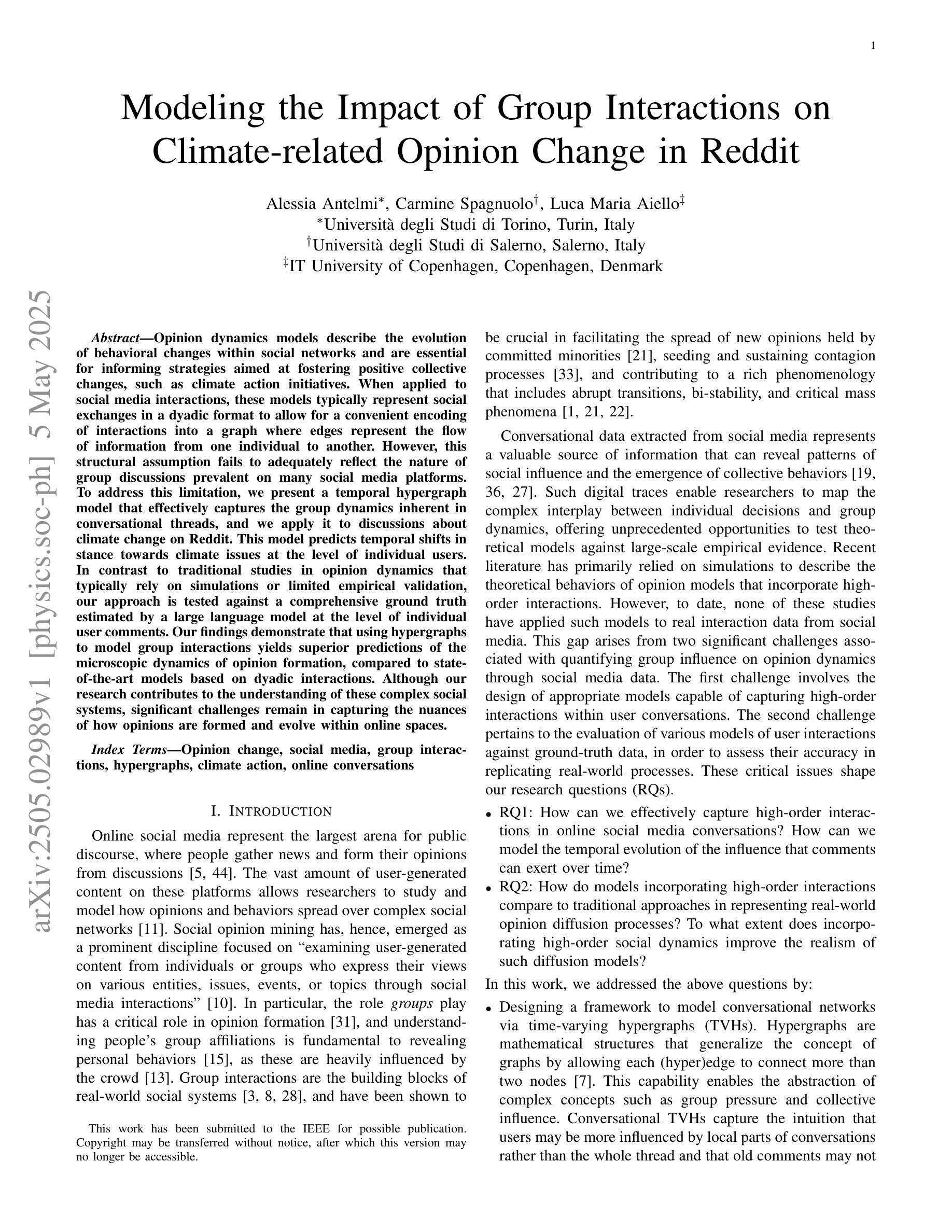
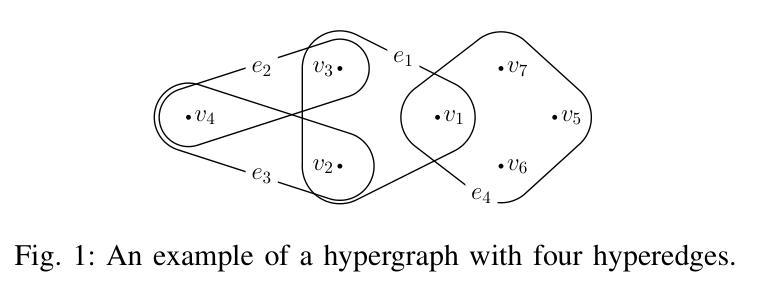
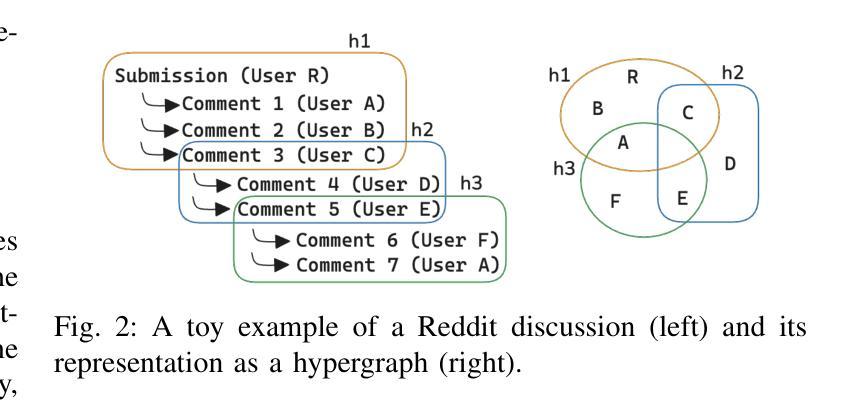
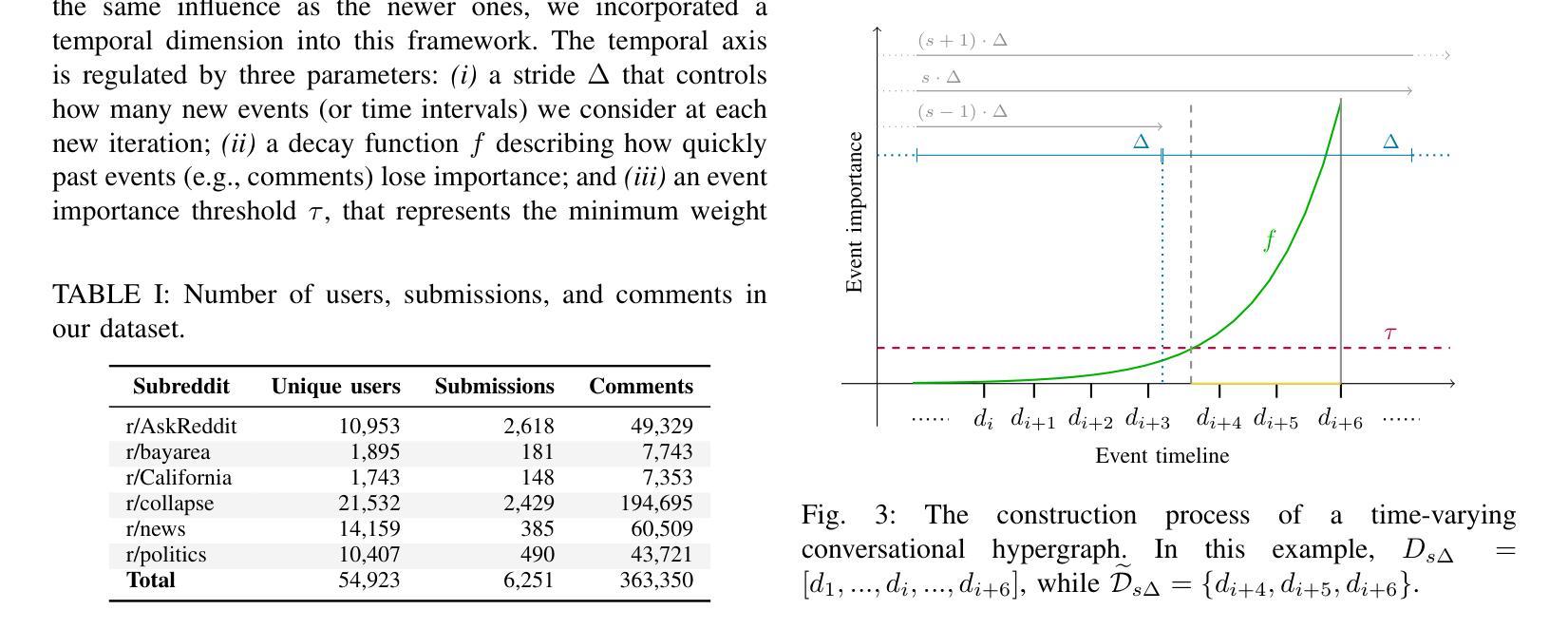
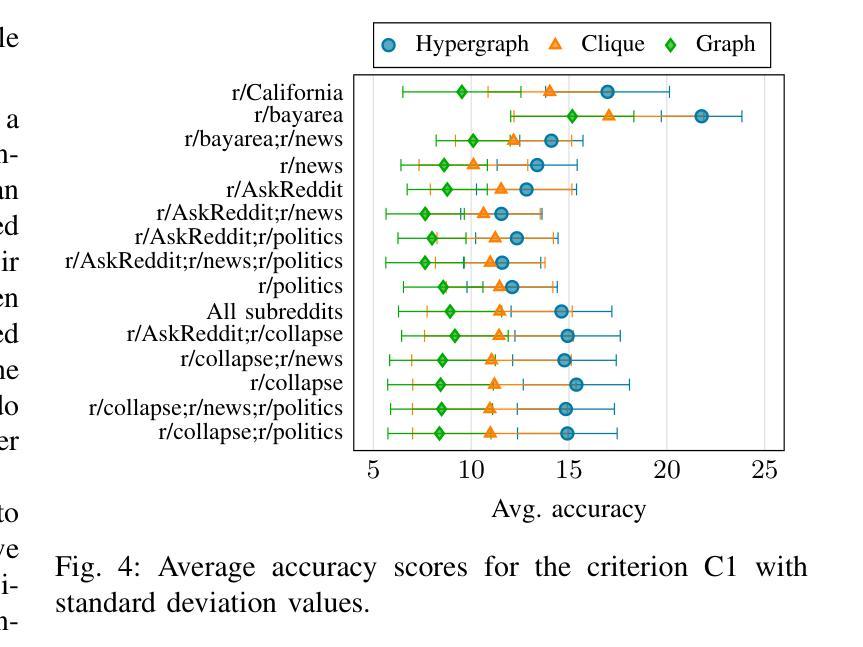
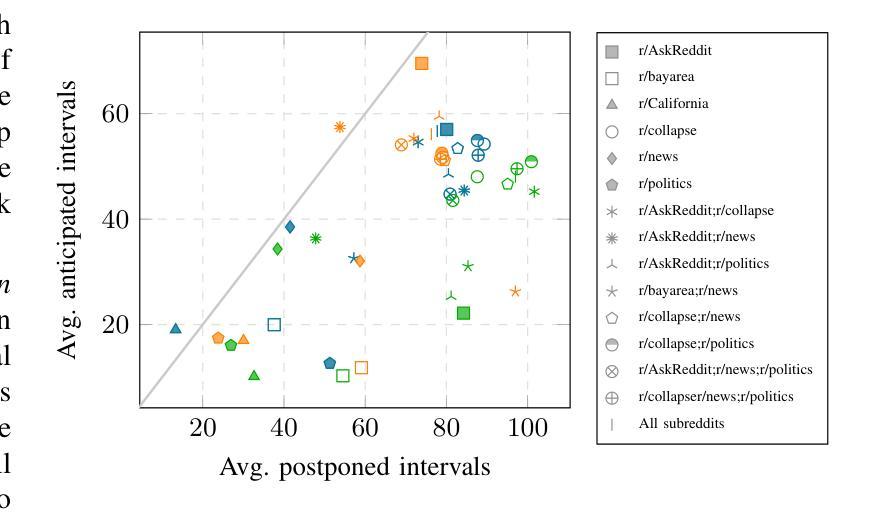
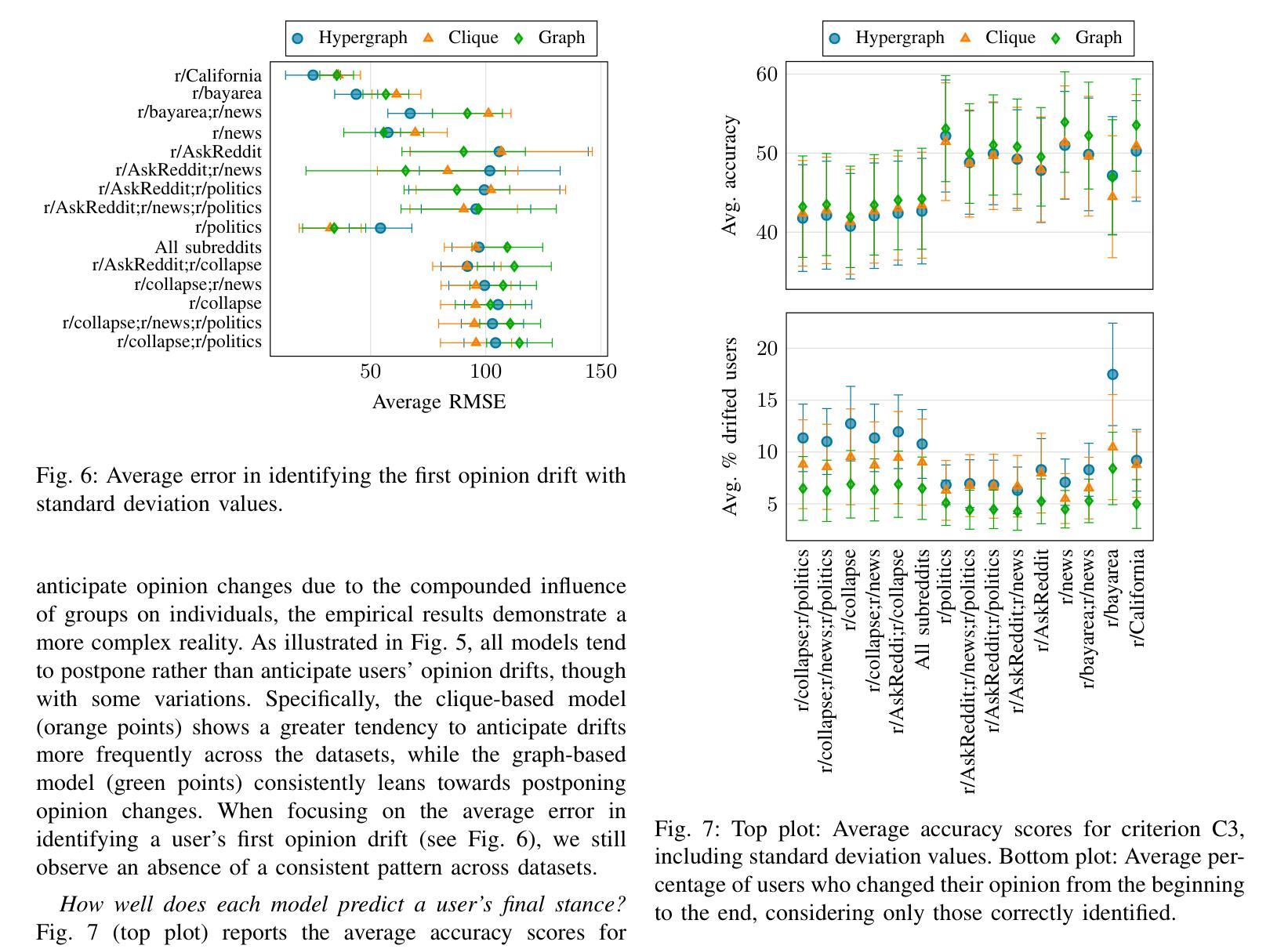
Enhancing Chemical Reaction and Retrosynthesis Prediction with Large Language Model and Dual-task Learning
Authors:Xuan Lin, Qingrui Liu, Hongxin Xiang, Daojian Zeng, Xiangxiang Zeng
Chemical reaction and retrosynthesis prediction are fundamental tasks in drug discovery. Recently, large language models (LLMs) have shown potential in many domains. However, directly applying LLMs to these tasks faces two major challenges: (i) lacking a large-scale chemical synthesis-related instruction dataset; (ii) ignoring the close correlation between reaction and retrosynthesis prediction for the existing fine-tuning strategies. To address these challenges, we propose ChemDual, a novel LLM framework for accurate chemical synthesis. Specifically, considering the high cost of data acquisition for reaction and retrosynthesis, ChemDual regards the reaction-and-retrosynthesis of molecules as a related recombination-and-fragmentation process and constructs a large-scale of 4.4 million instruction dataset. Furthermore, ChemDual introduces an enhanced LLaMA, equipped with a multi-scale tokenizer and dual-task learning strategy, to jointly optimize the process of recombination and fragmentation as well as the tasks between reaction and retrosynthesis prediction. Extensive experiments on Mol-Instruction and USPTO-50K datasets demonstrate that ChemDual achieves state-of-the-art performance in both predictions of reaction and retrosynthesis, outperforming the existing conventional single-task approaches and the general open-source LLMs. Through molecular docking analysis, ChemDual generates compounds with diverse and strong protein binding affinity, further highlighting its strong potential in drug design.
化学反应和逆合成预测是药物发现中的基本任务。最近,大型语言模型(LLM)在许多领域显示出潜力。然而,直接将LLM应用于这些任务面临两大挑战:(i)缺乏大规模化学合成相关指令数据集;(ii)现有微调策略忽视了反应和逆合成预测之间的密切关联。为了解决这些挑战,我们提出了ChemDual,一个用于精确化学合成的新型LLM框架。具体来说,考虑到化学反应和逆合成的数据获取成本高昂,ChemDual将分子的反应和逆合成视为相关的重组和断裂过程,并构建了一个大规模包含440万条指令的数据集。此外,ChemDual引入了一种增强的LLaMA,配备了多尺度分词器和双任务学习策略,以联合优化重组和断裂过程以及反应和逆合成预测任务之间的优化。在Mol-Instruction和USPTO-50K数据集上的大量实验表明,ChemDual在反应和逆合成的预测方面达到了最新技术水平,超越了现有的常规单任务方法和通用的开源LLM。通过分子对接分析,ChemDual生成了具有多样性和强蛋白质结合亲和力的化合物,进一步凸显了其在药物设计中的强大潜力。
论文及项目相关链接
PDF Accepted for publication at IJCAI 2025
Summary
该文本介绍了在药物发现中化学反应和合成预测的重要性,并指出了大型语言模型在这两个任务上应用面临的挑战。为解决这些问题,提出了一种名为ChemDual的新型LLM框架,通过构建大规模指令数据集,引入增强型LLaMA模型,实现了化学反应与合成预测的高精度。ChemDual在分子反应与合成中将分子视为一个相关的重组与断裂过程,并通过多尺度分词器和双重任务学习策略进行优化。实验证明,ChemDual在反应与合成预测方面达到了最先进的性能,并通过分子对接分析生成了具有多样性和强蛋白质结合亲和力的化合物,显示出其在药物设计中的巨大潜力。
Key Takeaways
- 化学反应和合成预测是药物发现中的核心任务。
- 大型语言模型在这两个任务上的应用面临挑战,主要问题在于缺乏大规模化学合成相关指令数据集以及现有精细调整策略忽视了反应和合成预测之间的密切联系。
- ChemDual框架被提出来解决这些问题,它将分子反应和合成视为一个相关的重组和断裂过程。
- ChemDual构建了大规模指令数据集,并引入了增强型LLaMA模型,该模型配备了多尺度分词器和双重任务学习策略。
- 实验证明ChemDual在反应和合成预测方面达到了最先进的性能。
- ChemDual通过分子对接分析生成了具有多样性和强蛋白质结合亲和力的化合物。
点此查看论文截图
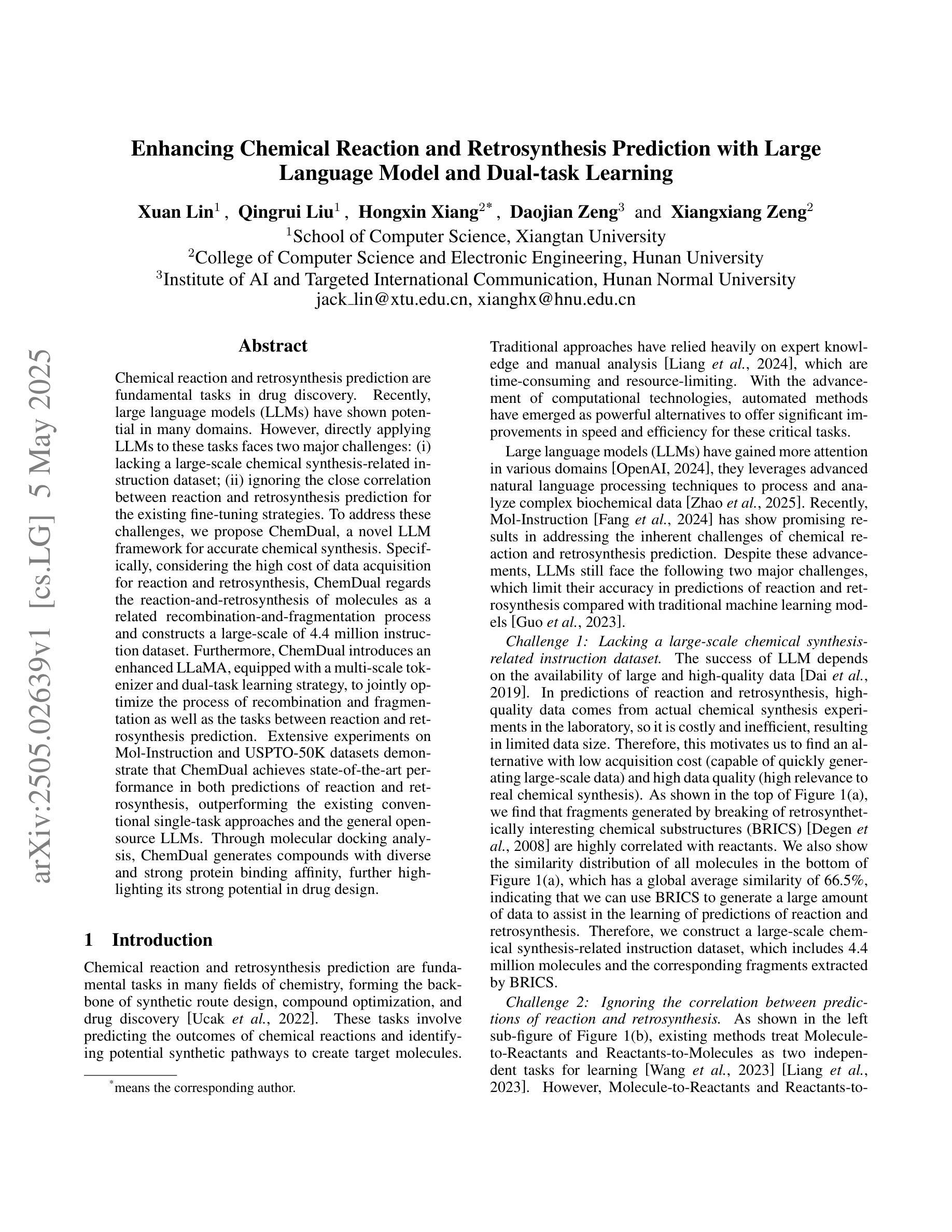
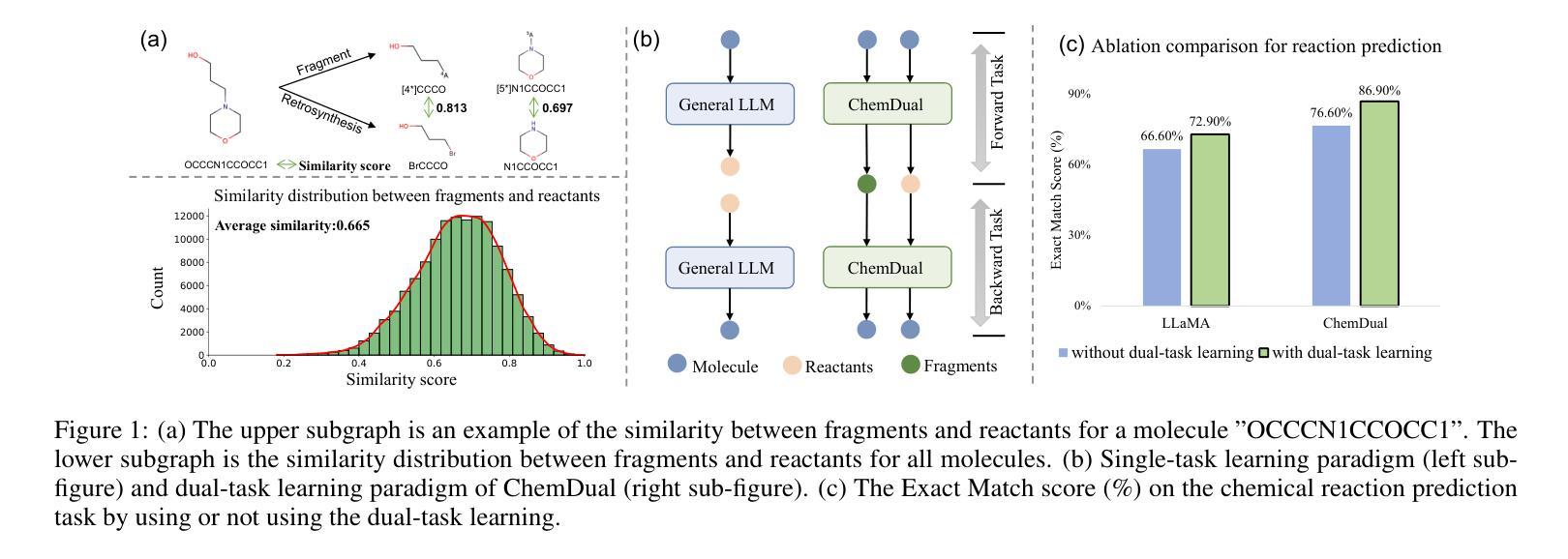
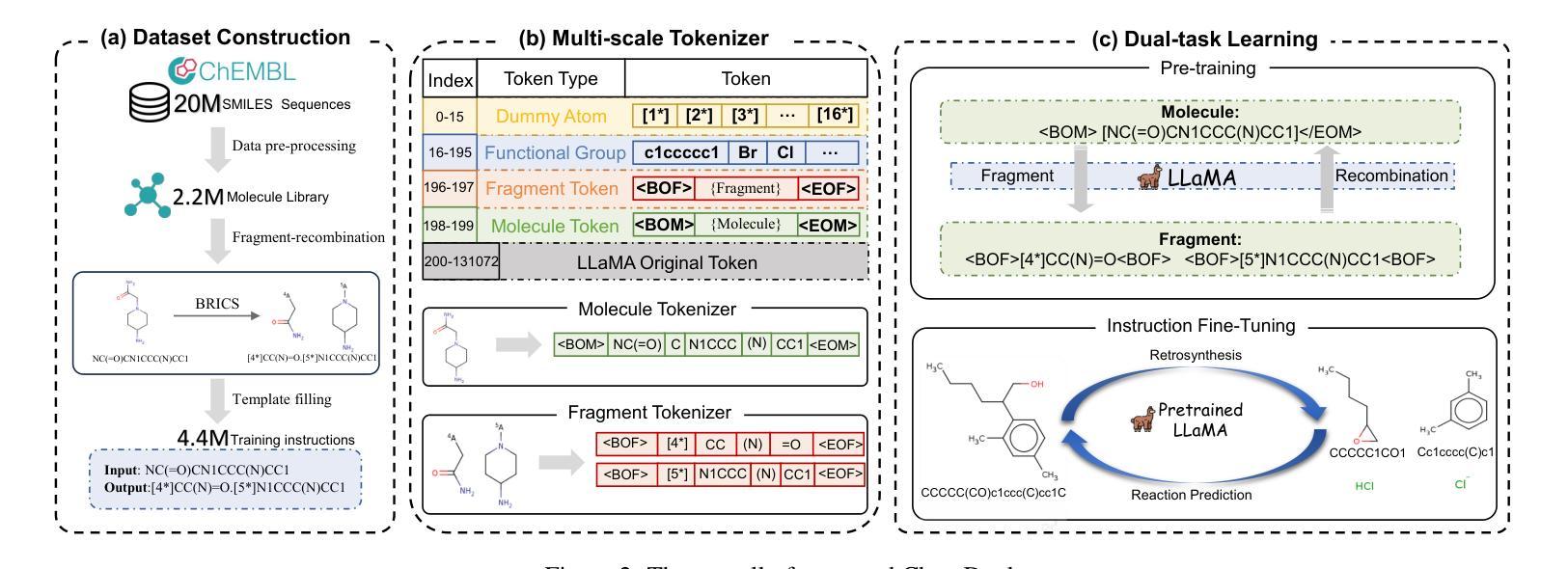
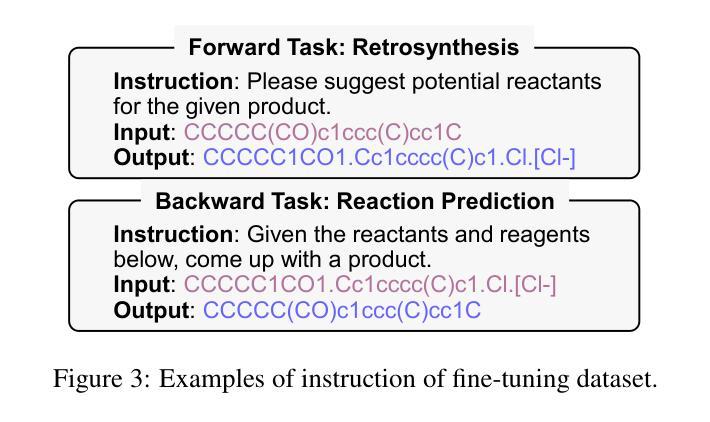
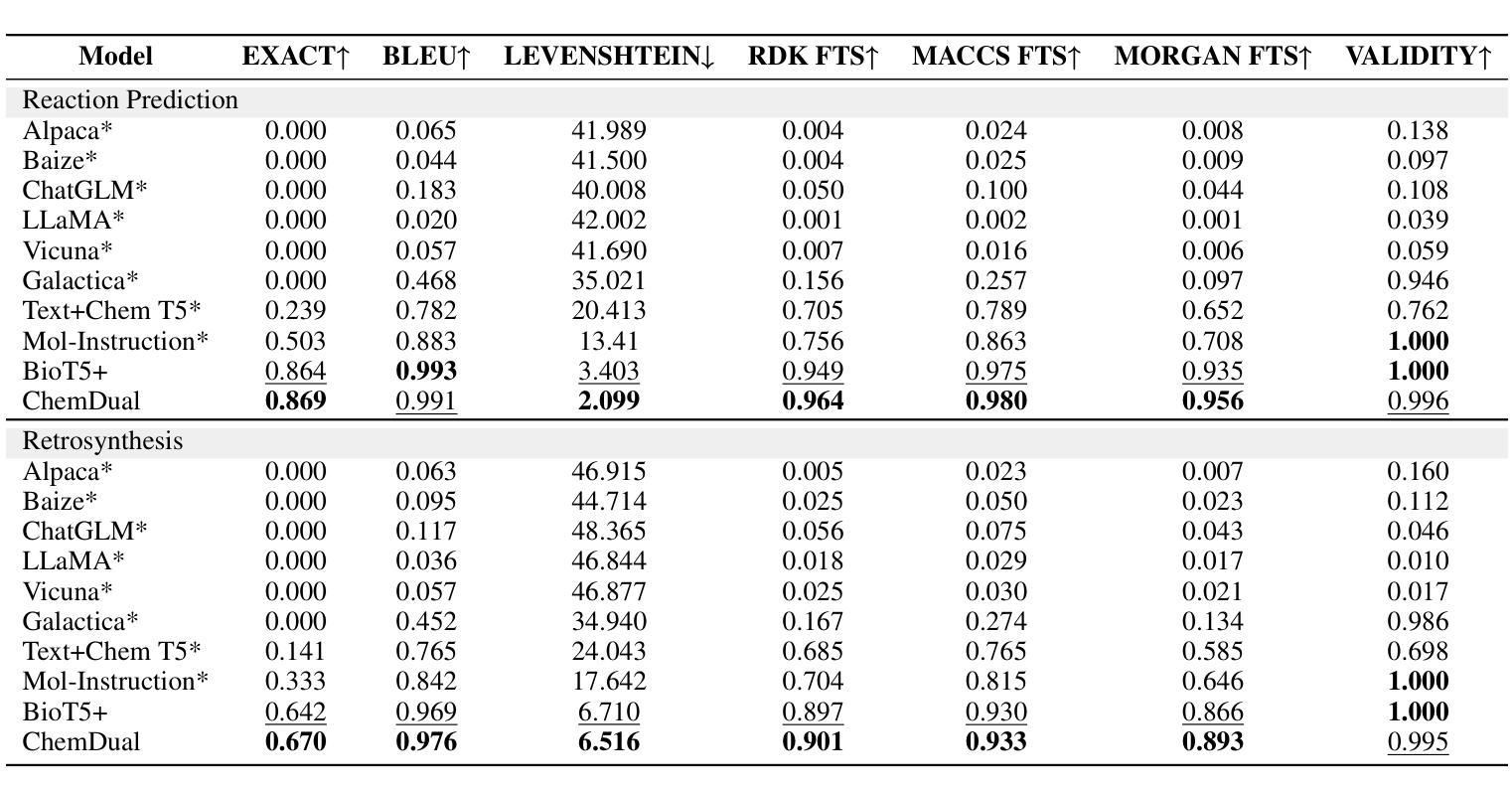
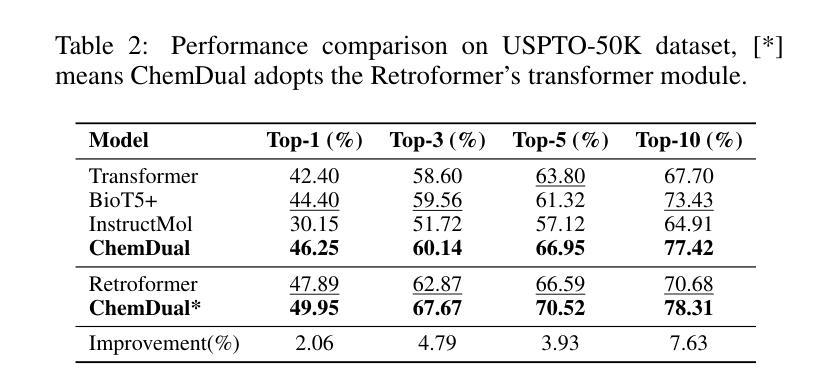
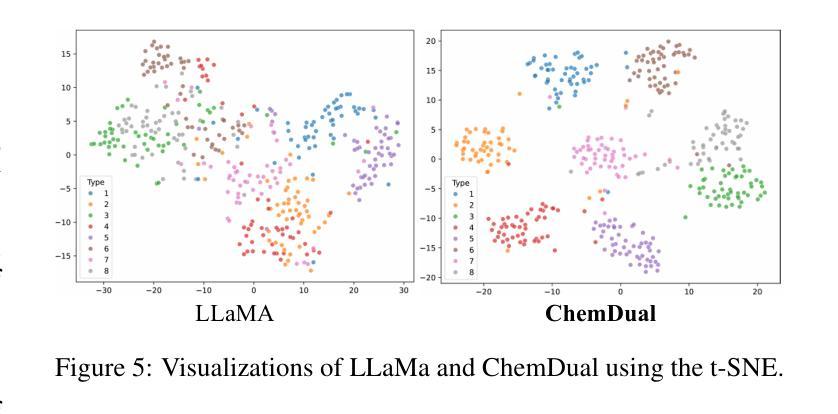
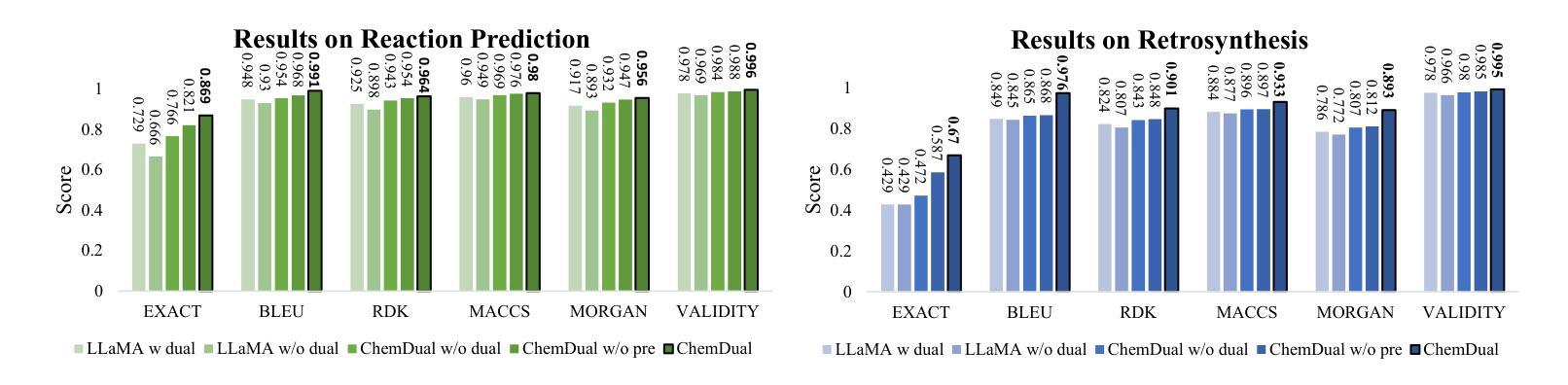
Towards Film-Making Production Dialogue, Narration, Monologue Adaptive Moving Dubbing Benchmarks
Authors:Chaoyi Wang, Junjie Zheng, Zihao Chen, Shiyu Xia, Chaofan Ding, Xiaohao Zhang, Xi Tao, Xiaoming He, Xinhan Di
Movie dubbing has advanced significantly, yet assessing the real-world effectiveness of these models remains challenging. A comprehensive evaluation benchmark is crucial for two key reasons: 1) Existing metrics fail to fully capture the complexities of dialogue, narration, monologue, and actor adaptability in movie dubbing. 2) A practical evaluation system should offer valuable insights to improve movie dubbing quality and advancement in film production. To this end, we introduce Talking Adaptive Dubbing Benchmarks (TA-Dubbing), designed to improve film production by adapting to dialogue, narration, monologue, and actors in movie dubbing. TA-Dubbing offers several key advantages: 1) Comprehensive Dimensions: TA-Dubbing covers a variety of dimensions of movie dubbing, incorporating metric evaluations for both movie understanding and speech generation. 2) Versatile Benchmarking: TA-Dubbing is designed to evaluate state-of-the-art movie dubbing models and advanced multi-modal large language models. 3) Full Open-Sourcing: We fully open-source TA-Dubbing at https://github.com/woka- 0a/DeepDubber- V1 including all video suits, evaluation methods, annotations. We also continuously integrate new movie dubbing models into the TA-Dubbing leaderboard at https://github.com/woka- 0a/DeepDubber-V1 to drive forward the field of movie dubbing.
电影配音已经取得了显著的进步,但评估这些模型在现实世界中的效果仍然具有挑战性。综合评价基准至关重要,出于两个关键原因:1)现有指标未能充分捕捉电影配音中的对话、旁白、独白和演员适应性的复杂性。2)一个实用的评估系统应该提供有价值的见解,以提高电影配音质量和电影制作进展。为此,我们引入了谈话自适应配音基准(TA-Dubbing),旨在通过适应电影配音中的对话、旁白、独白和演员来提高电影制作水平。TA-Dubbing提供了几个关键优势:1)综合维度:TA-Dubbing涵盖了电影配音的多个维度,结合了电影理解和语音生成的指标评估。2)通用基准测试:TA-Dubbing旨在评估最先进的电影配音模型和高级多模态大型语言模型。3)完全开源:我们在https://github.com/woka-0a/DeepDubber-V1上完全开源TA-Dubbing,包括所有视频套件、评估方法和注释。我们还不断将新的电影配音模型集成到TA-Dubbing排行榜中,以推动电影配音领域的发展。
论文及项目相关链接
PDF 6 pages, 3 figures, accepted to the AI for Content Creation workshop at CVPR 2025 in Nashville, TN
Summary
电影配音技术已显著提升,但评估其在实际应用中的效果仍然具有挑战性。为了全面评估电影配音模型,我们引入了Talking Adaptive Dubbing Benchmarks(TA-Dubbing),以提高电影制作中对电影对话、旁白、独白和演员适应性的评价能力。TA-Dubbing具有全面的维度、灵活的基准测试和完全开源的特点。
Key Takeaways
- 电影配音技术的评估仍面临挑战,需要一个全面的评估基准。
- 现有评价指标未能充分捕捉电影对话、旁白、独白和演员适应性的复杂性。
- TA-Dubbing旨在提高电影制作中对电影配音的评价能力。
- TA-Dubbing具有全面的维度,涵盖了电影配音的各个方面。
- TA-Dubbing可灵活用于评估先进的电影配音模型和多模态大型语言模型。
- TA-Dubbing完全开源,包括视频套件、评估方法和注释等。
点此查看论文截图
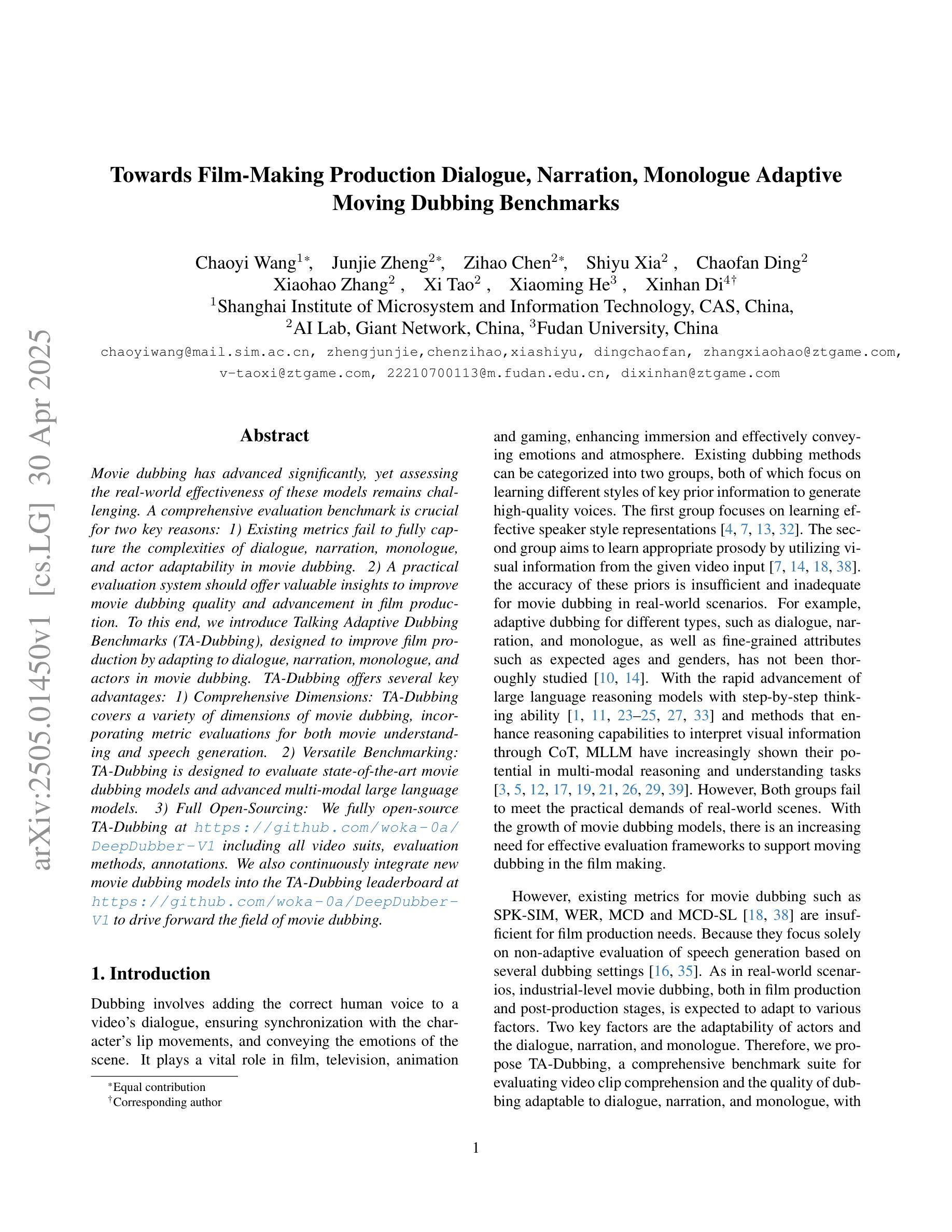
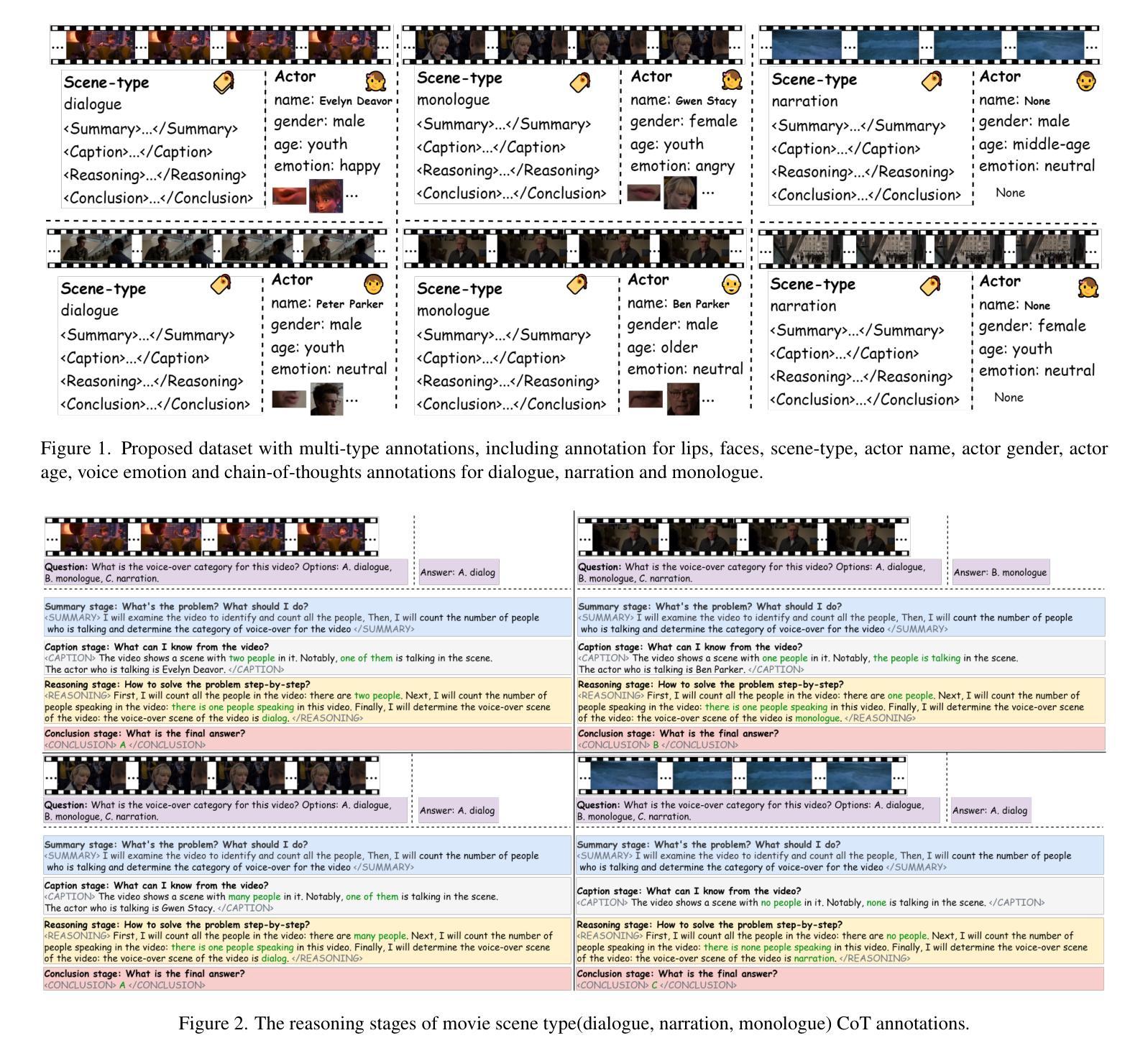
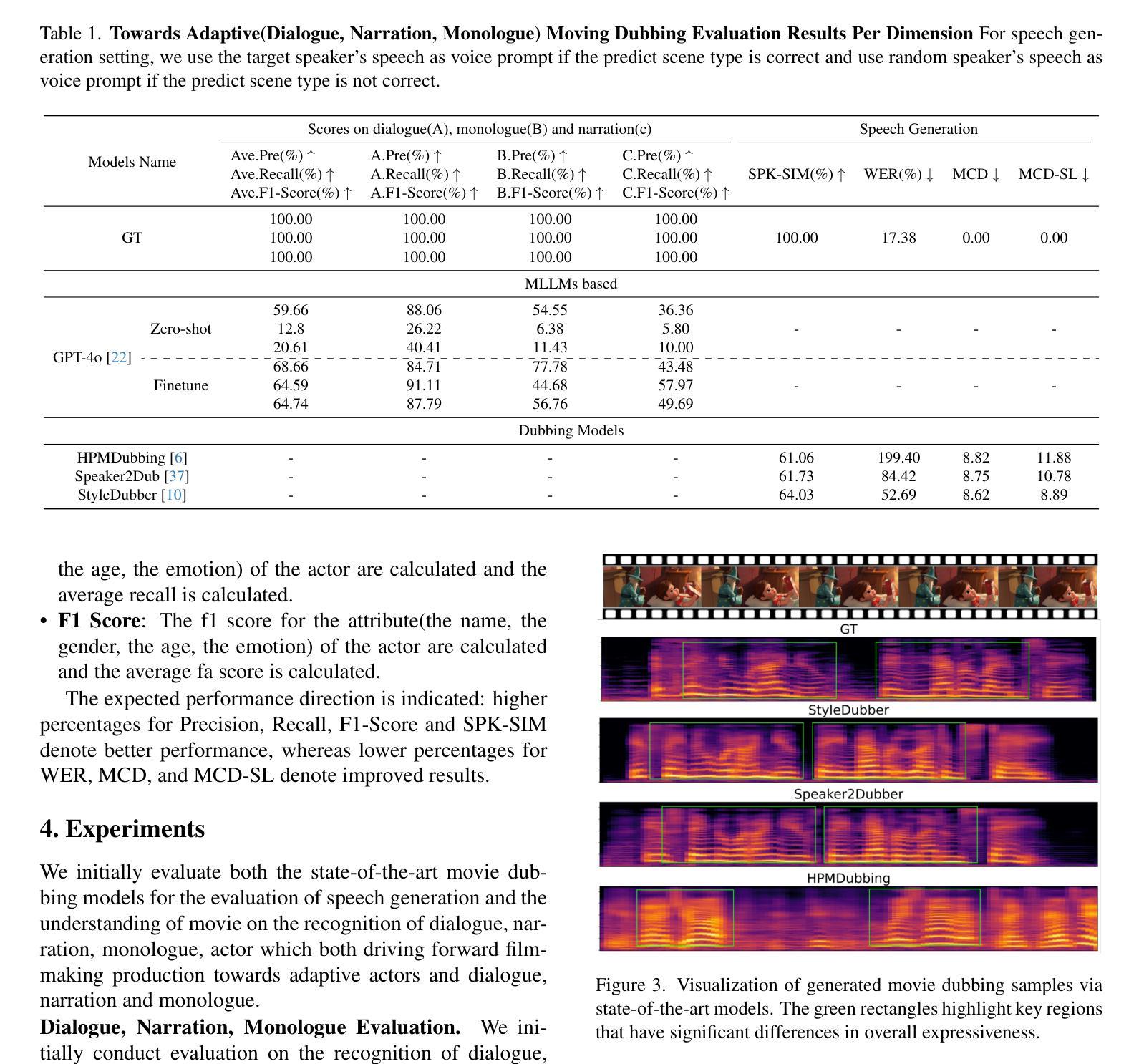