⚠️ 以下所有内容总结都来自于 大语言模型的能力,如有错误,仅供参考,谨慎使用
🔴 请注意:千万不要用于严肃的学术场景,只能用于论文阅读前的初筛!
💗 如果您觉得我们的项目对您有帮助 ChatPaperFree ,还请您给我们一些鼓励!⭐️ HuggingFace免费体验
2025-05-08 更新
TSTMotion: Training-free Scene-aware Text-to-motion Generation
Authors:Ziyan Guo, Haoxuan Qu, Hossein Rahmani, Dewen Soh, Ping Hu, Qiuhong Ke, Jun Liu
Text-to-motion generation has recently garnered significant research interest, primarily focusing on generating human motion sequences in blank backgrounds. However, human motions commonly occur within diverse 3D scenes, which has prompted exploration into scene-aware text-to-motion generation methods. Yet, existing scene-aware methods often rely on large-scale ground-truth motion sequences in diverse 3D scenes, which poses practical challenges due to the expensive cost. To mitigate this challenge, we are the first to propose a \textbf{T}raining-free \textbf{S}cene-aware \textbf{T}ext-to-\textbf{Motion} framework, dubbed as \textbf{TSTMotion}, that efficiently empowers pre-trained blank-background motion generators with the scene-aware capability. Specifically, conditioned on the given 3D scene and text description, we adopt foundation models together to reason, predict and validate a scene-aware motion guidance. Then, the motion guidance is incorporated into the blank-background motion generators with two modifications, resulting in scene-aware text-driven motion sequences. Extensive experiments demonstrate the efficacy and generalizability of our proposed framework. We release our code in \href{https://tstmotion.github.io/}{Project Page}.
文本到动作生成近期引起了研究者的极大兴趣,主要集中于在空白背景下生成人类动作序列。然而,人类动作通常发生在多样的3D场景中,这促使人们探索场景感知的文本到动作生成方法。然而,现有的场景感知方法常常依赖于大规模真实场景的多样运动序列,这在实际应用中带来了高昂的成本挑战。为了缓解这一挑战,我们首次提出了一个无需训练的场景感知文本到动作框架,名为“TSTMotion”,该框架能够高效地使预训练的空白背景动作生成器具备场景感知能力。具体来说,根据给定的3D场景和文本描述,我们采用基础模型进行推理、预测和验证场景感知动作指导。然后,将该动作指导融入空白背景动作生成器,并进行两次修改,从而生成场景感知的文本驱动动作序列。大量实验证明了我们提出的框架的有效性和通用性。我们已在项目页面[https://tstmotion.github.io/]上发布了我们的代码。
论文及项目相关链接
PDF Accepted by ICME2025
Summary
文本至动作生成技术近期受到研究关注,主要集中于在空白背景下生成人类动作序列。然而,人类动作常发生在多样的3D场景中,促使研究者探索场景感知的文本至动作生成方法。但现有场景感知方法常依赖于大规模真实场景下的动作序列数据,这在实际应用中造成了高昂的成本。为解决此挑战,我们首次提出一种无需训练的场景感知文本至动作生成框架,简称TSTMotion。它能有效增强预训练的空白背景动作生成器的场景感知能力。通过给定的3D场景和文本描述,我们采用基础模型进行推理、预测和验证场景感知动作指导。然后,将此动作指导融入空白背景动作生成器并进行两次修改,从而生成场景感知的文本驱动动作序列。大量实验证明了我们框架的有效性和泛化能力。
Key Takeaways
- Text-to-motion generation研究集中在空白背景下生成人类动作序列。
- 人类动作常发生在多样的3D场景中,需要探索场景感知的文本至动作生成方法。
- 现有场景感知方法依赖大规模真实场景下的动作序列数据,造成实践中的挑战。
- 首次提出无需训练的场景感知文本至动作生成框架TSTMotion。
- TSTMotion能增强预训练空白背景动作生成器的场景感知能力。
- 通过给定的3D场景和文本描述,采用基础模型进行推理、预测和验证场景感知动作指导。
点此查看论文截图
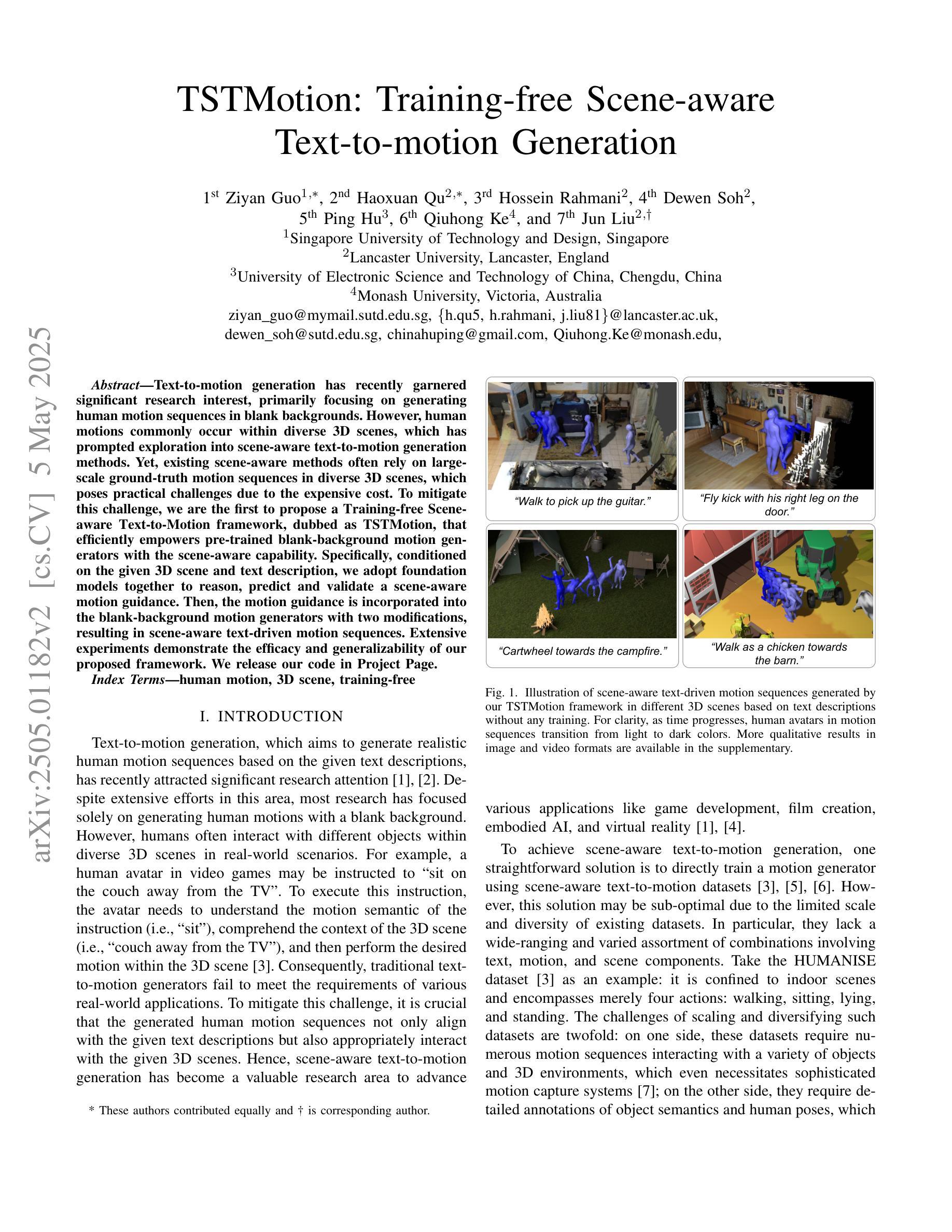
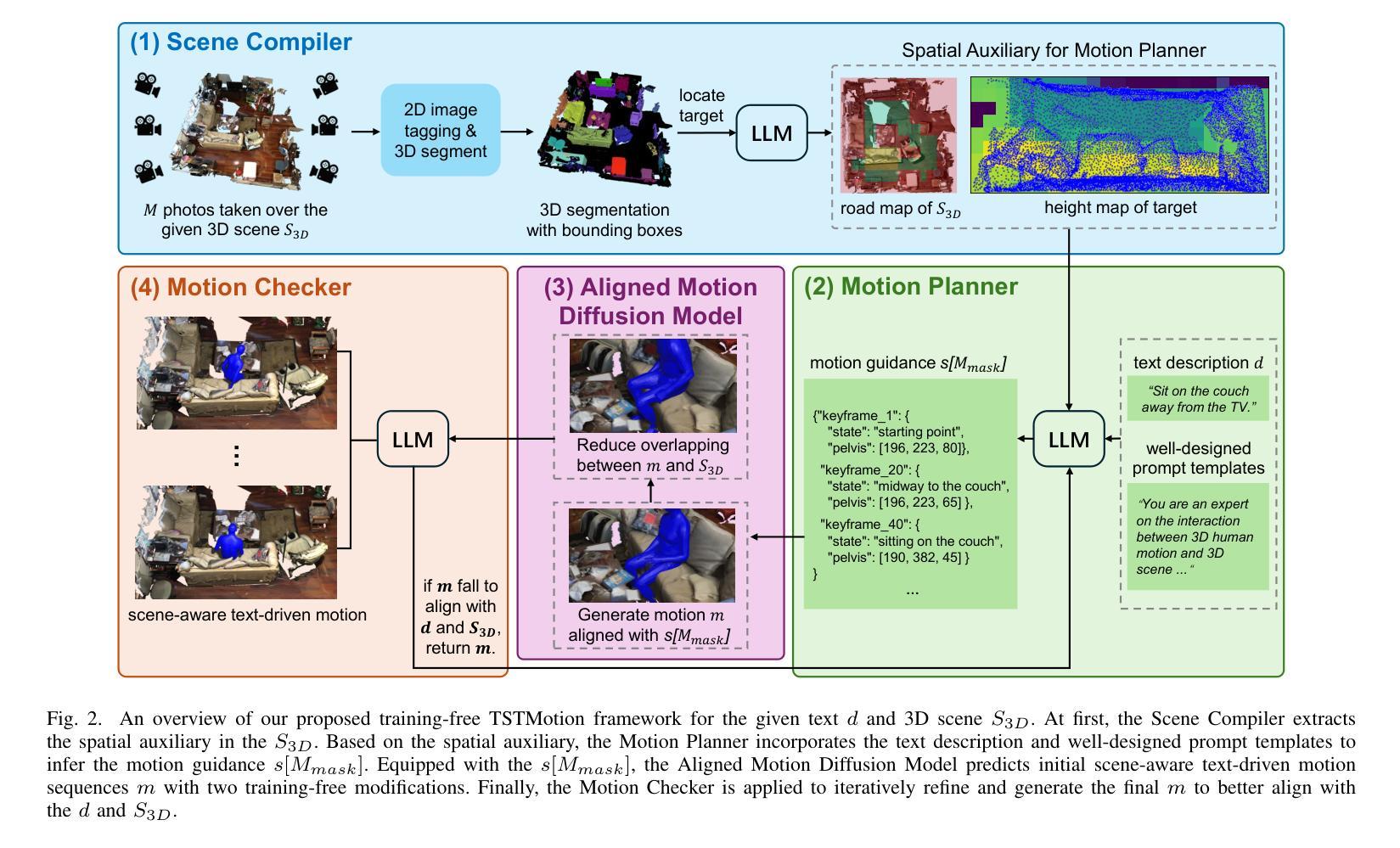
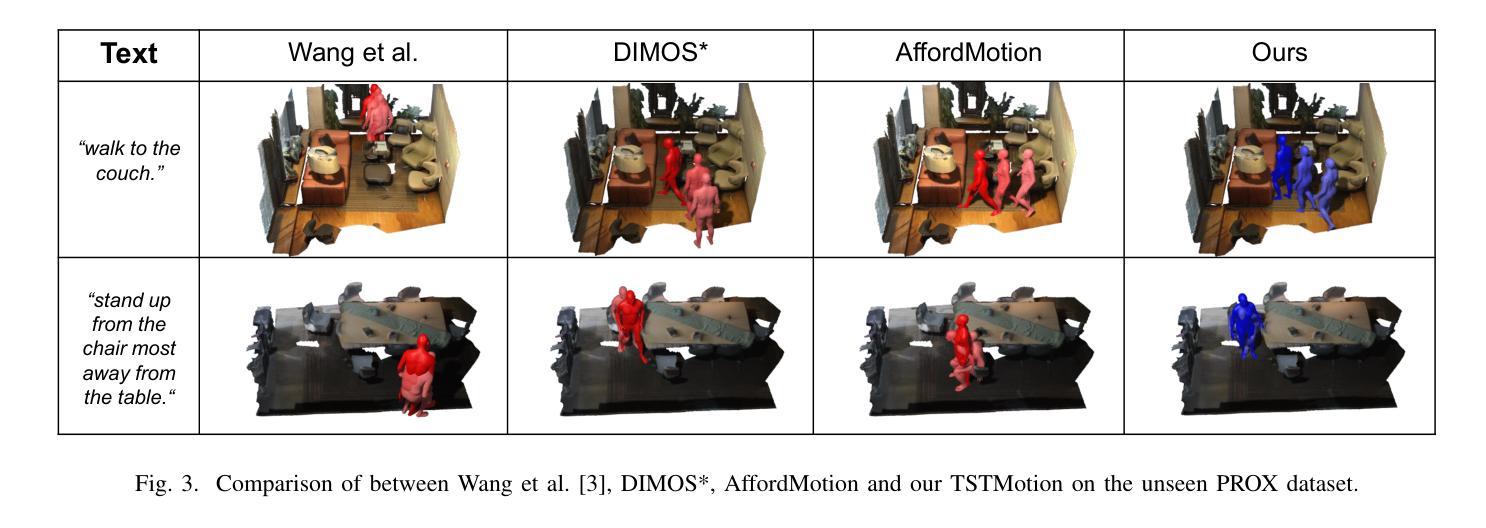

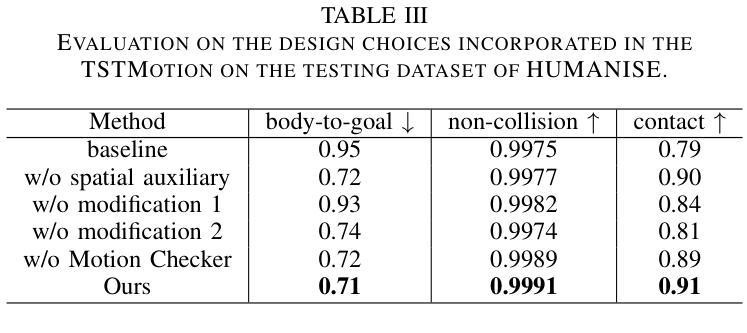