⚠️ 以下所有内容总结都来自于 大语言模型的能力,如有错误,仅供参考,谨慎使用
🔴 请注意:千万不要用于严肃的学术场景,只能用于论文阅读前的初筛!
💗 如果您觉得我们的项目对您有帮助 ChatPaperFree ,还请您给我们一些鼓励!⭐️ HuggingFace免费体验
2025-05-09 更新
Person Recognition at Altitude and Range: Fusion of Face, Body Shape and Gait
Authors:Feng Liu, Nicholas Chimitt, Lanqing Guo, Jitesh Jain, Aditya Kane, Minchul Kim, Wes Robbins, Yiyang Su, Dingqiang Ye, Xingguang Zhang, Jie Zhu, Siddharth Satyakam, Christopher Perry, Stanley H. Chan, Arun Ross, Humphrey Shi, Zhangyang Wang, Anil Jain, Xiaoming Liu
We address the problem of whole-body person recognition in unconstrained environments. This problem arises in surveillance scenarios such as those in the IARPA Biometric Recognition and Identification at Altitude and Range (BRIAR) program, where biometric data is captured at long standoff distances, elevated viewing angles, and under adverse atmospheric conditions (e.g., turbulence and high wind velocity). To this end, we propose FarSight, a unified end-to-end system for person recognition that integrates complementary biometric cues across face, gait, and body shape modalities. FarSight incorporates novel algorithms across four core modules: multi-subject detection and tracking, recognition-aware video restoration, modality-specific biometric feature encoding, and quality-guided multi-modal fusion. These components are designed to work cohesively under degraded image conditions, large pose and scale variations, and cross-domain gaps. Extensive experiments on the BRIAR dataset, one of the most comprehensive benchmarks for long-range, multi-modal biometric recognition, demonstrate the effectiveness of FarSight. Compared to our preliminary system, this system achieves a 34.1% absolute gain in 1:1 verification accuracy (TAR@0.1% FAR), a 17.8% increase in closed-set identification (Rank-20), and a 34.3% reduction in open-set identification errors (FNIR@1% FPIR). Furthermore, FarSight was evaluated in the 2025 NIST RTE Face in Video Evaluation (FIVE), which conducts standardized face recognition testing on the BRIAR dataset. These results establish FarSight as a state-of-the-art solution for operational biometric recognition in challenging real-world conditions.
我们致力于解决非约束环境下全身人员识别的问题。这个问题出现在监控场景中,例如在IARPA(智能评估风险代理计划)的高空范围生物识别(BRIAR)项目中,生物识别数据是在远距离、高视角观测和恶劣大气条件(例如湍流和强风)下收集的。为此,我们提出了FarSight,这是一个端到端的全身人员识别系统,它通过融合面部、步态和体形等生物识别特征来解决这个问题。FarSight集成了四个核心模块的全新算法:多目标检测和跟踪、识别感知视频恢复、模态特定生物特征编码以及质量引导多模态融合。这些组件被设计为在图像退化、大姿态和尺度变化和跨域差异的情况下协同工作。在BRIAR数据集上的大量实验表明,FarSight在远程多模态生物识别方面是最全面、最有效的基准测试之一。与我们的初步系统相比,FarSight在1:1验证精度上实现了34.1%的绝对提升(TAR@0.1% FAR),在封闭集识别中提高了17.8%(Rank-20),在开放集识别错误中减少了34.3%(FNIR@1% FPIR)。此外,FarSight在NIST RTE视频面部识别评估(FIVE)中的评估结果证明了其在具有挑战性的真实条件下进行实战生物识别的最新解决方案地位。
论文及项目相关链接
PDF 18 pages, 12 figures
Summary
本文解决了在不受限制的环境中全身人员识别的问题。该问题出现在监控场景中,例如在IARPA的BIAR项目中,生物识别数据是在长距离、高视角和恶劣大气条件下捕获的。为此,本文提出了FarSight系统,这是一个端到端的人员识别系统,通过整合面部、步态和体态等多模态的生物识别信息来解决该问题。FarSight包含四个核心模块:多主体检测与追踪、识别感知视频恢复、模态特定生物特征编码以及质量引导多模态融合。在BIAR数据集上的大量实验证明了FarSight的有效性。与初步系统相比,FarSight在身份验证准确性方面实现了34.1%的绝对提升,在封闭集识别中提高了17.8%,在开放集识别错误中减少了34.3%。此外,FarSight在2025年NIST的RTE面部视频评估中表现优异。总的来说,FarSight是解决具有挑战性的现实条件下操作生物识别问题的最新解决方案。
Key Takeaways
- FarSight系统解决了在不受限制环境中全身人员识别的问题,适用于监控场景。
- FarSight集成了面部、步态和体态等多模态的生物识别信息。
- FarSight包含四个核心模块:多主体检测与追踪、识别感知视频恢复、模态特定生物特征编码以及质量引导多模态融合。
- 在BIAR数据集上的实验证明了FarSight的有效性。
- 与初步系统相比,FarSight在身份验证、封闭集识别和开放集识别错误方面有了显著改进。
- FarSight在2025年NIST的RTE面部视频评估中表现优异。
点此查看论文截图
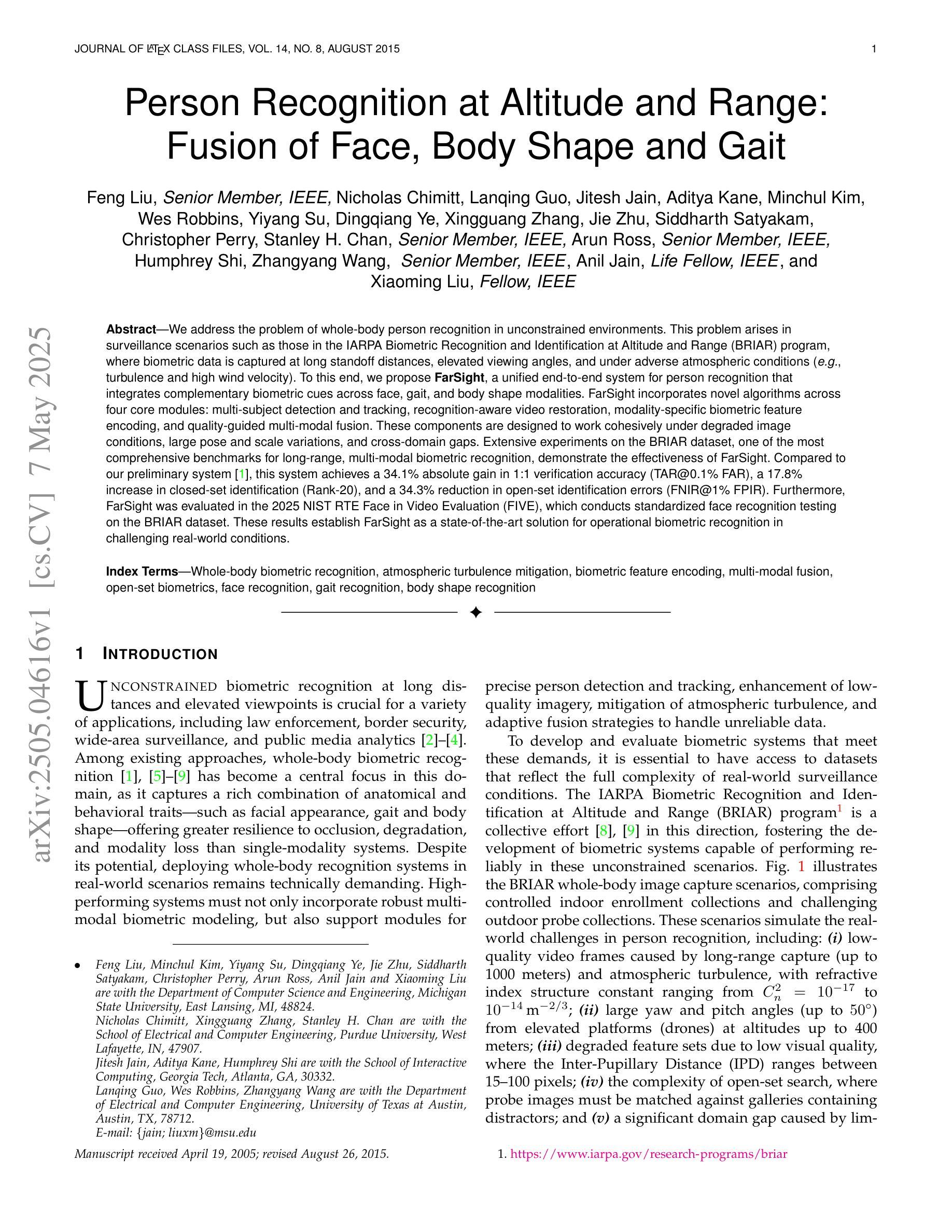
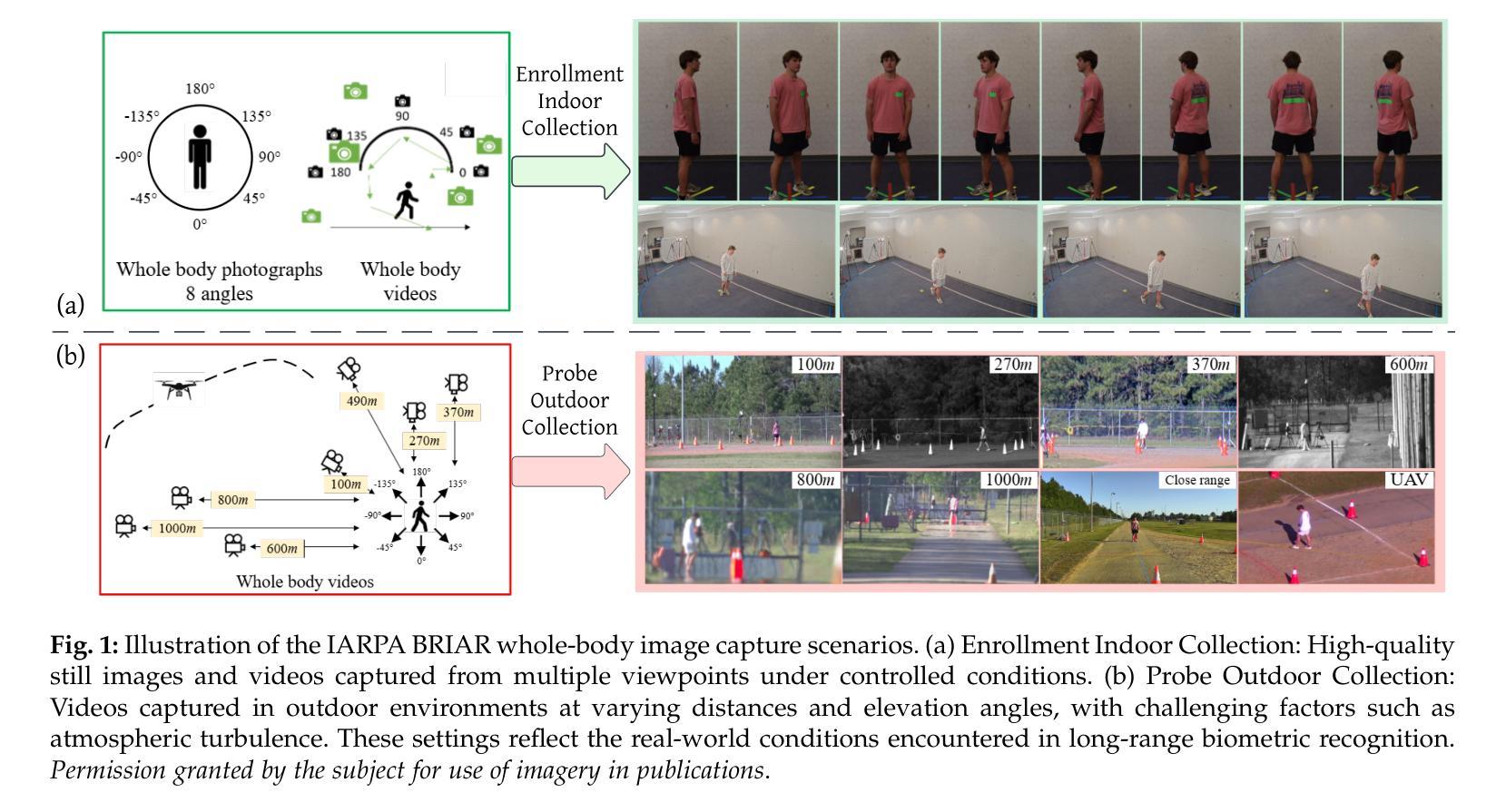
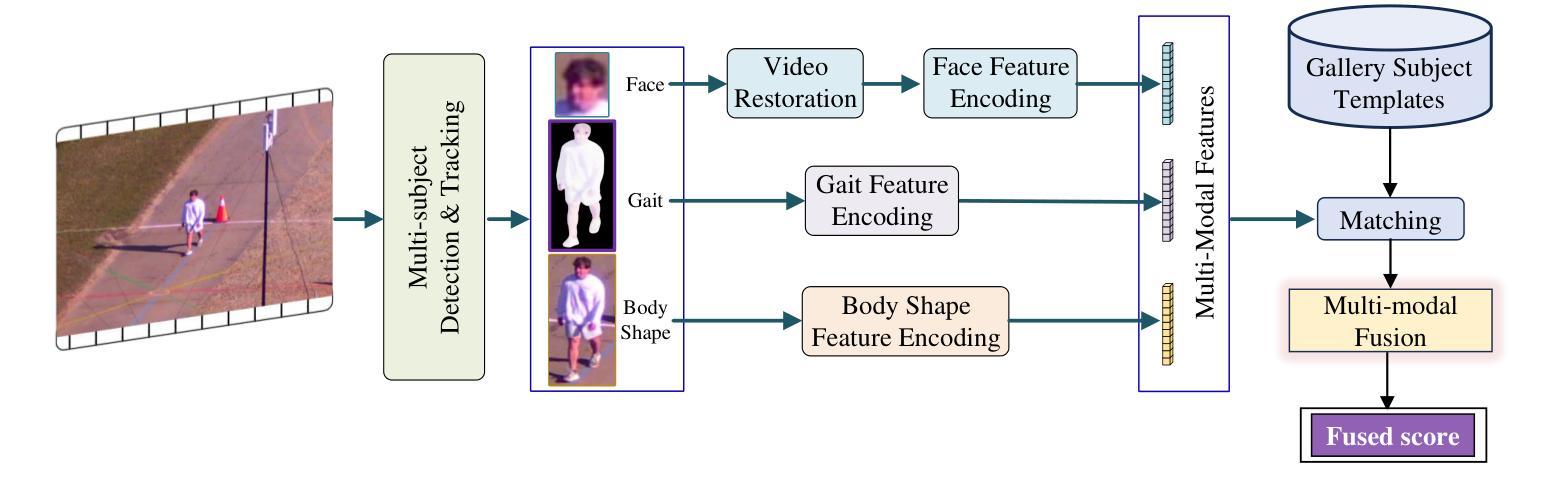
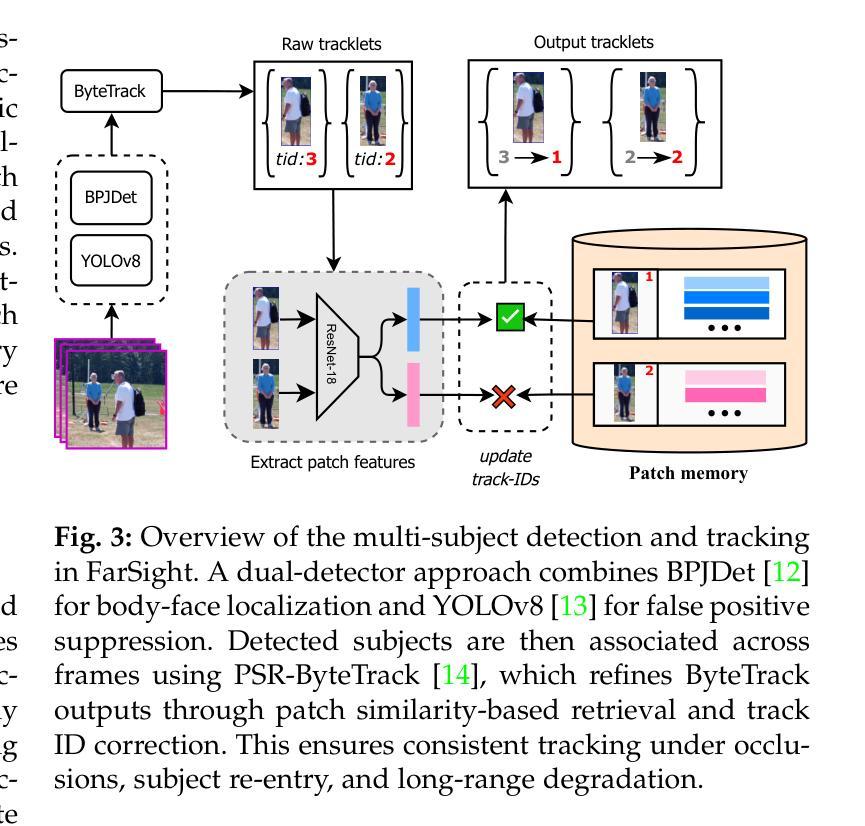
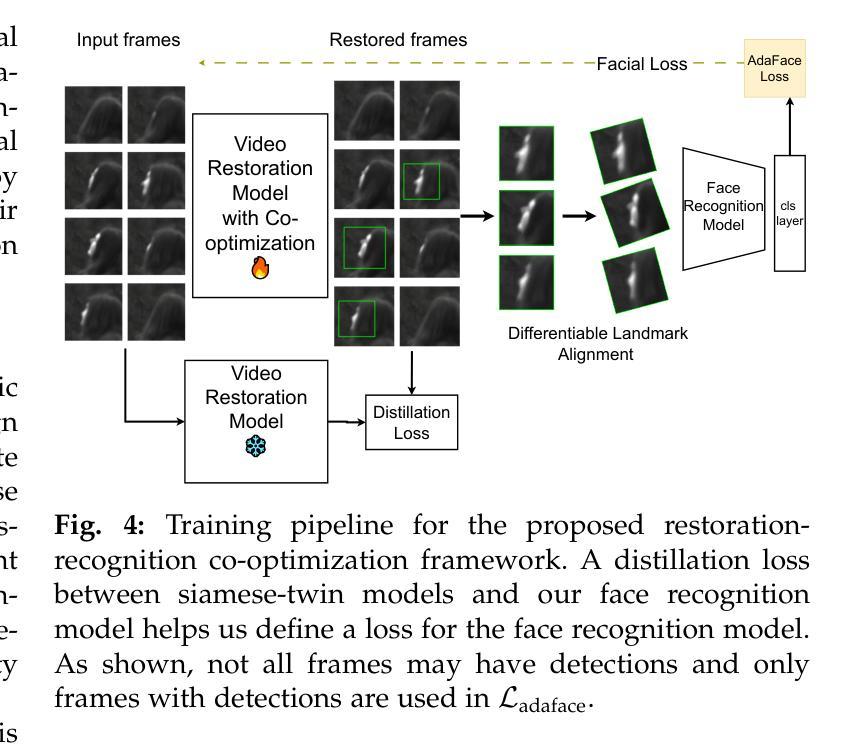