⚠️ 以下所有内容总结都来自于 大语言模型的能力,如有错误,仅供参考,谨慎使用
🔴 请注意:千万不要用于严肃的学术场景,只能用于论文阅读前的初筛!
💗 如果您觉得我们的项目对您有帮助 ChatPaperFree ,还请您给我们一些鼓励!⭐️ HuggingFace免费体验
2025-05-09 更新
MSA-UNet3+: Multi-Scale Attention UNet3+ with New Supervised Prototypical Contrastive Loss for Coronary DSA Image Segmentation
Authors:Rayan Merghani Ahmed, Adnan Iltaf, Mohamed Elmanna, Gang Zhao, Hongliang Li, Yue Du, Bin Li, Shoujun Zhou
Accurate segmentation of coronary Digital Subtraction Angiography images is essential to diagnose and treat coronary artery diseases. Despite advances in deep learning, challenges such as high intra-class variance and class imbalance limit precise vessel delineation. Most existing approaches for coronary DSA segmentation cannot address these issues. Also, existing segmentation network’s encoders do not directly generate semantic embeddings, which could enable the decoder to reconstruct segmentation masks effectively from these well-defined features. We propose a Supervised Prototypical Contrastive Loss that fuses supervised and prototypical contrastive learning to enhance coronary DSA image segmentation. The supervised contrastive loss enforces semantic embeddings in the encoder, improving feature differentiation. The prototypical contrastive loss allows the model to focus on the foreground class while alleviating the high intra-class variance and class imbalance problems by concentrating only on the hard-to-classify background samples. We implement the proposed SPCL loss within an MSA-UNet3+: a Multi-Scale Attention-Enhanced UNet3+ architecture. The architecture integrates key components: a Multi-Scale Attention Encoder and a Multi-Scale Dilated Bottleneck designed to enhance multi-scale feature extraction and a Contextual Attention Fusion Module built to keep fine-grained details while improving contextual understanding. Experiments on a private coronary DSA dataset show that MSA-UNet3+ outperforms state-of-the-art methods, achieving the highest Dice coefficient and F1-score and significantly reducing ASD and ACD. The developed framework provides clinicians with precise vessel segmentation, enabling accurate identification of coronary stenosis and supporting informed diagnostic and therapeutic decisions. The code will be released at https://github.com/rayanmerghani/MSA-UNet3plus.
冠状动脉数字减影血管造影(DSA)图像的准确分割对于冠状动脉疾病的诊断和治疗至关重要。尽管深度学习有所进展,但类内差异高和类别不平衡等挑战仍然限制了对血管精确描绘的能力。大多数现有的冠状动脉DSA分割方法无法解决这些问题。此外,现有的分割网络编码器并没有直接生成语义嵌入,这可能会使解码器无法从这些定义明确的特征中有效地重建分割掩膜。我们提出了一种有监督原型对比损失(Supervised Prototypical Contrastive Loss),它将有监督学习和原型对比学习相结合,以提高冠状动脉DSA图像的分割能力。有监督对比损失在编码器中强制执行语义嵌入,提高特征差异化。原型对比损失允许模型专注于前景类,并通过仅关注难以分类的背景样本,缓解类内差异高和类别不平衡问题。我们在MSA-UNet3+架构中实现了所提出的SPCL损失:一种集多尺度注意力增强UNet3+于一体的架构。该架构集成了关键组件:多尺度注意力编码器和多尺度膨胀瓶颈,旨在增强多尺度特征提取,以及上下文注意力融合模块,旨在保留精细细节的同时提高上下文理解。在私有冠状动脉DSA数据集上的实验表明,MSA-UNet3+优于最先进的方法,具有最高的狄克系数和F1分数,并显著降低ASD和ACD。所开发的框架为临床医生提供了精确的血管分割,能够准确识别冠状动脉狭窄,并支持做出有根据的诊断和治疗决策。代码将在https://github.com/rayanmerghani/MSA-UNet3plus上发布。
论文及项目相关链接
PDF Work in progress
Summary
本文提出一种融合监督对比损失和原型对比损失的监督原型对比损失(Supervised Prototypical Contrastive Loss),并应用于冠状动脉数字减影血管造影(DSA)图像的分割任务。为提高特征提取能力,采用多尺度注意力增强UNet3+(MSA-UNet3+)架构,实现对冠状动脉的精确分割,有助于医生准确诊断冠心病并支持治疗决策。
Key Takeaways
- 冠状动脉数字减影血管造影(DSA)图像的精确分割对诊断和治疗冠心病至关重要。
- 现有方法在处理冠状动脉DSA图像分割时面临高类内方差和类别不平衡的挑战。
- 提出了融合监督对比损失和原型对比损失的监督原型对比损失(SPCL),以提高特征区分度和模型性能。
- 采用多尺度注意力增强UNet3+(MSA-UNet3+)架构,包括多尺度注意力编码器、多尺度扩张瓶颈和上下文注意力融合模块。
- 实验结果显示,MSA-UNet3+在私有冠状动脉DSA数据集上实现了较高的Dice系数和F1分数,并显著降低了ASD和ACD。
- 所开发的框架为医生提供了精确的血管分割,有助于准确识别冠状动脉狭窄,支持临床诊断和治疗的决策过程。
点此查看论文截图
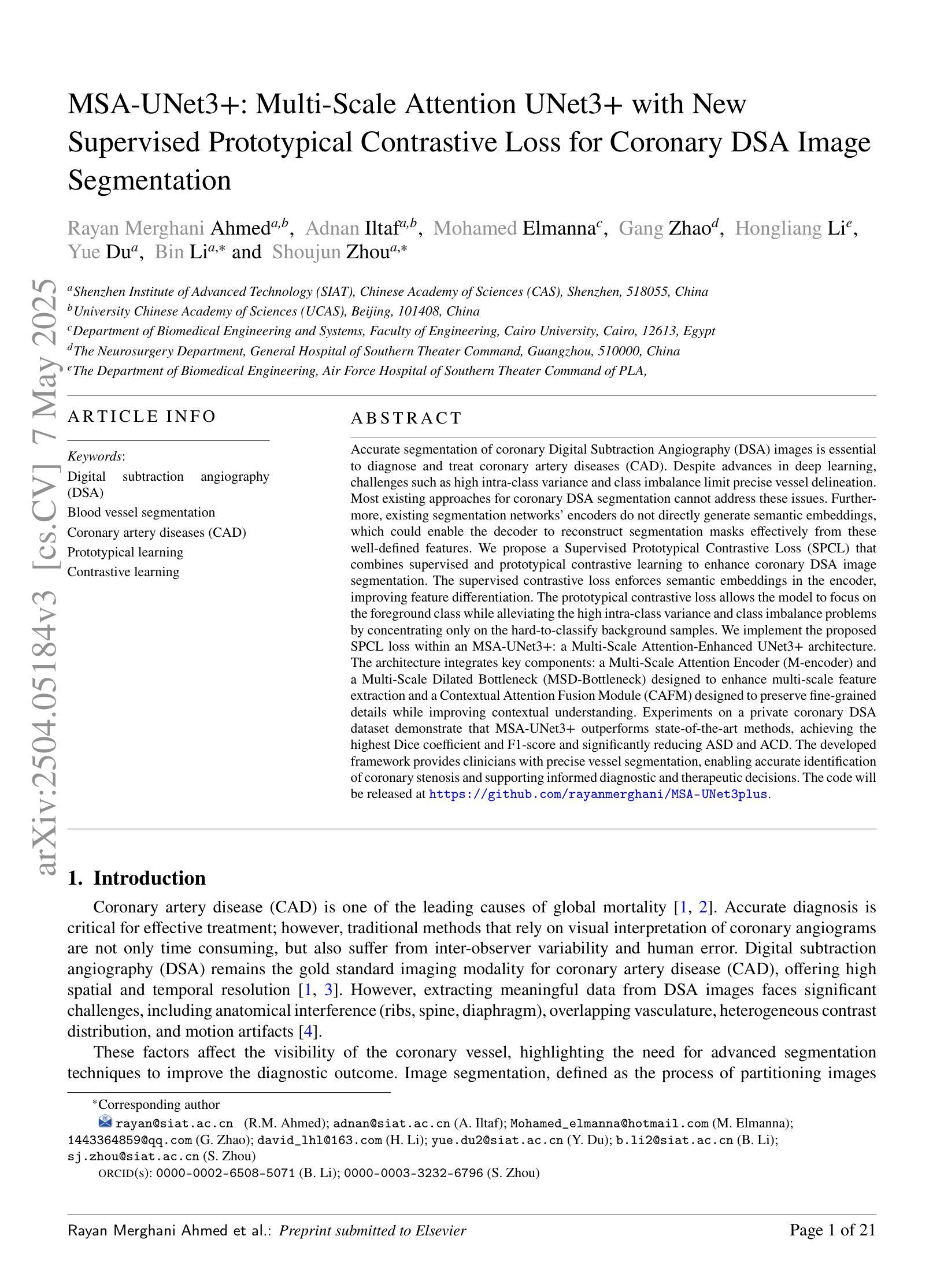
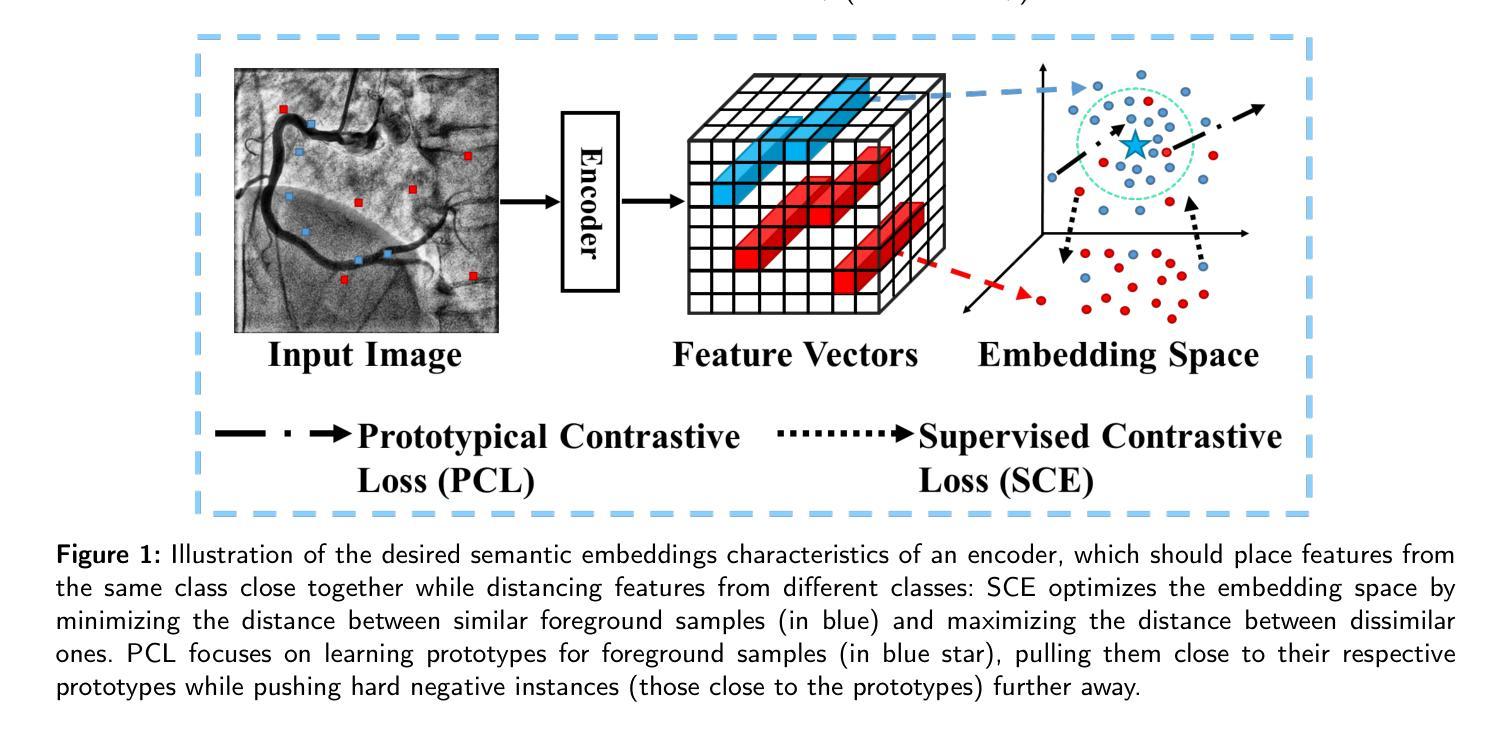
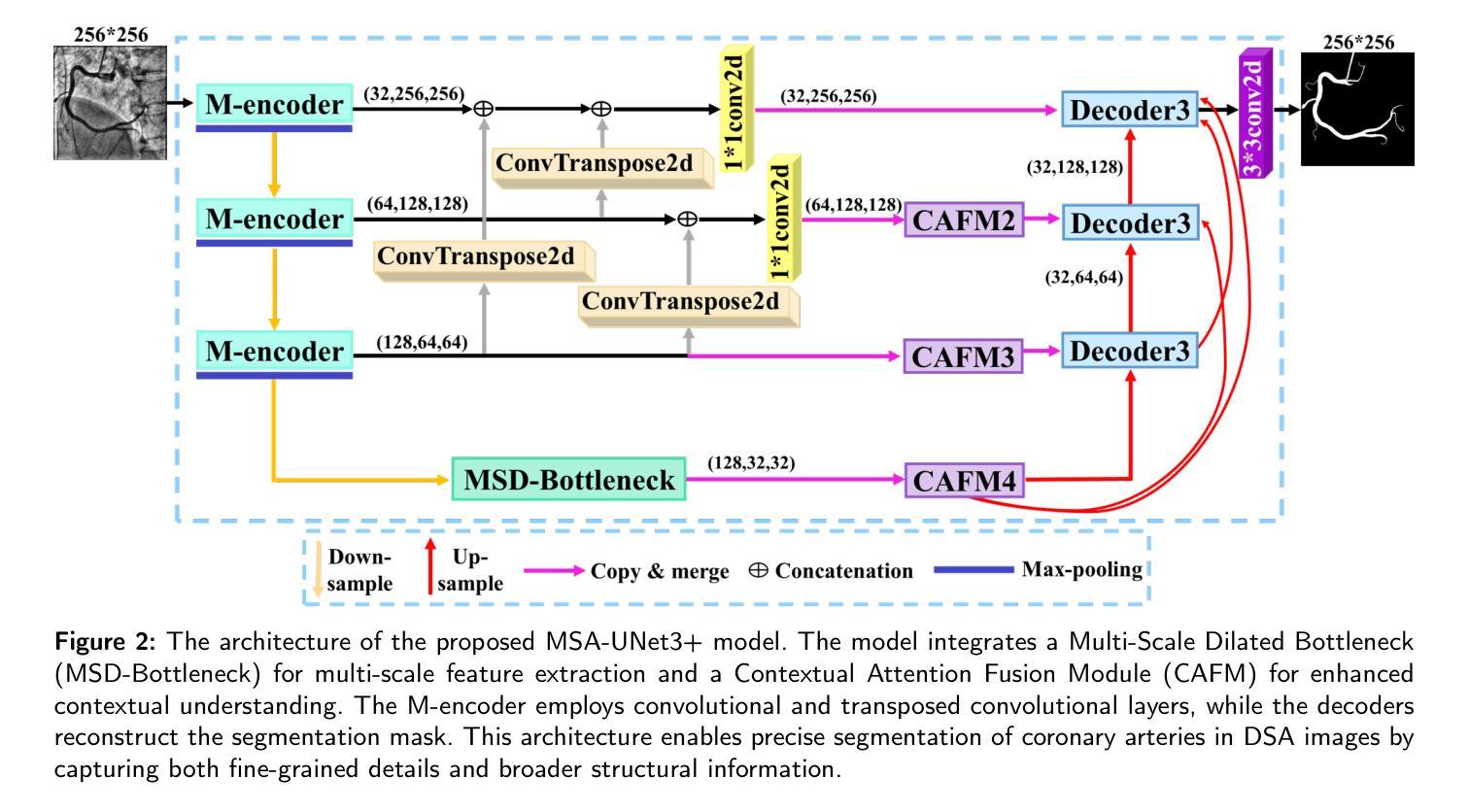
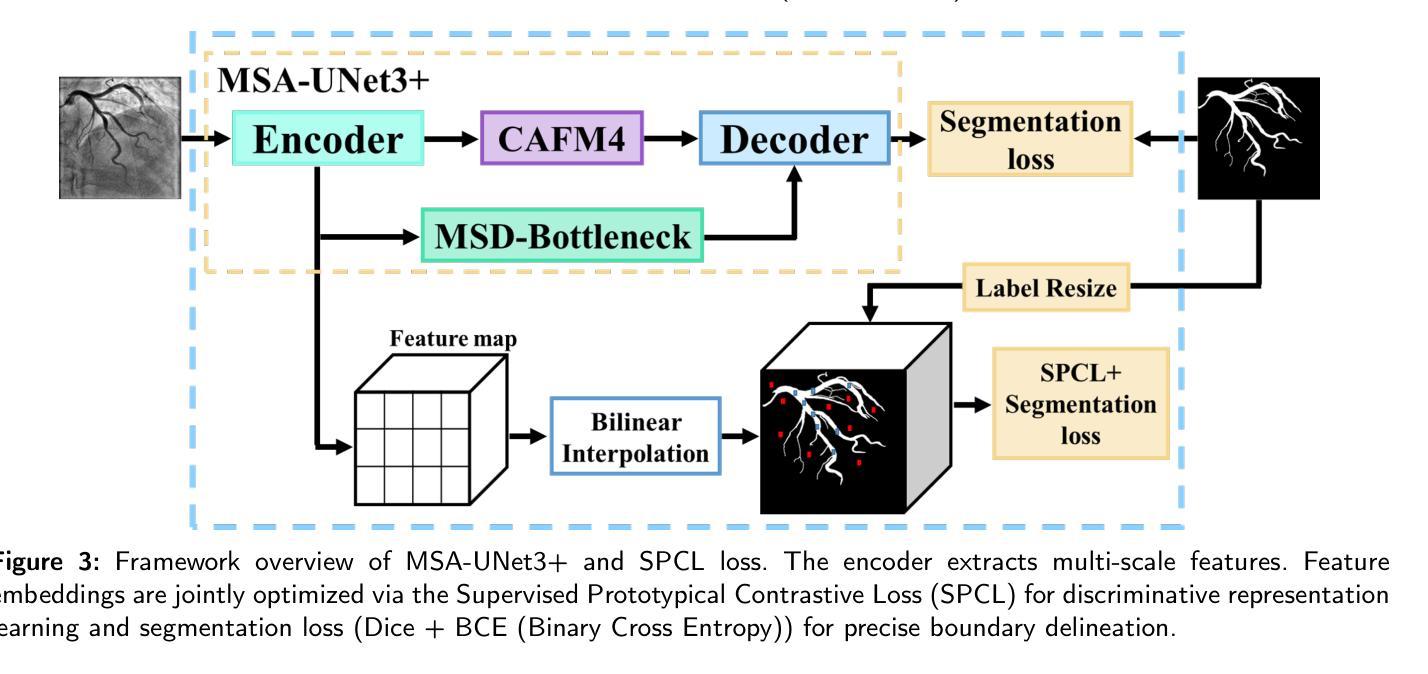
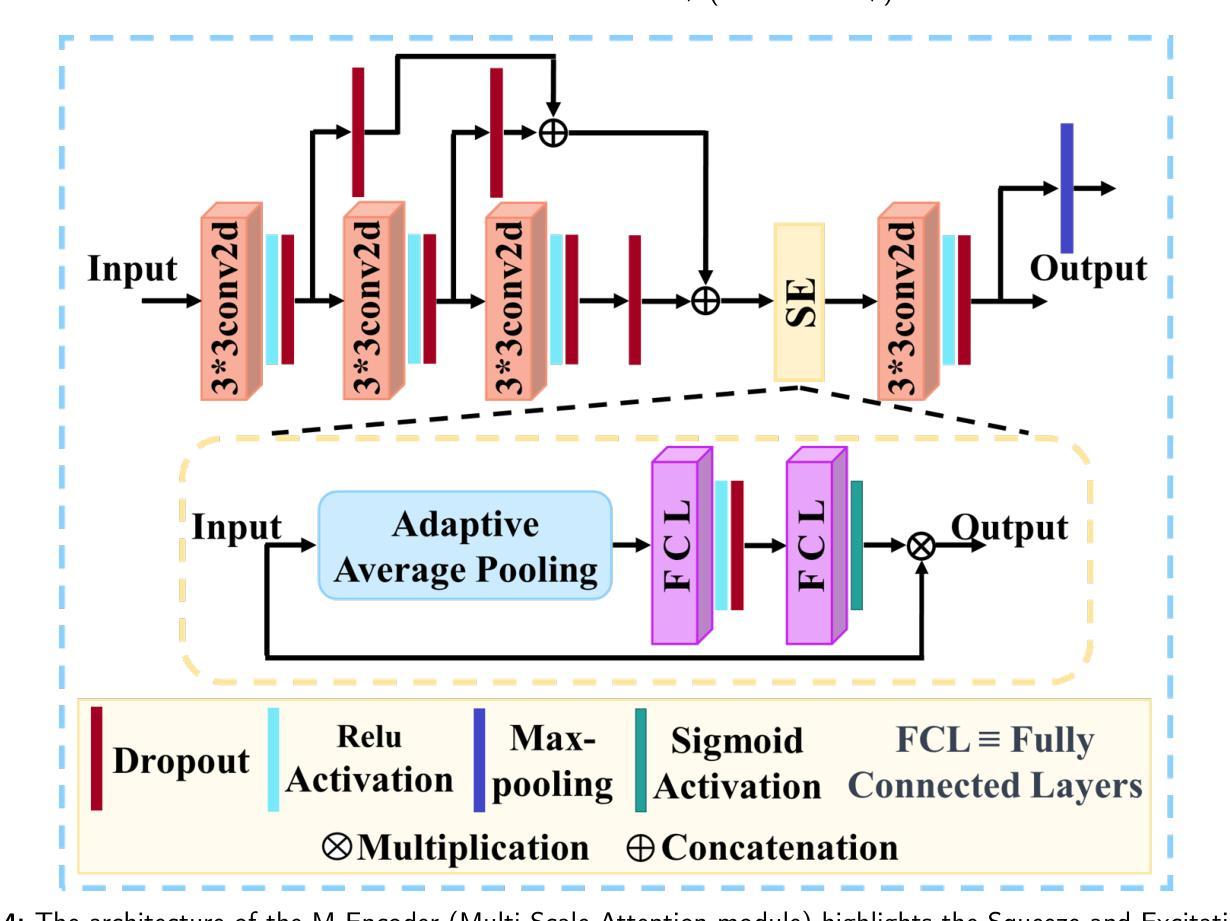