⚠️ 以下所有内容总结都来自于 大语言模型的能力,如有错误,仅供参考,谨慎使用
🔴 请注意:千万不要用于严肃的学术场景,只能用于论文阅读前的初筛!
💗 如果您觉得我们的项目对您有帮助 ChatPaperFree ,还请您给我们一些鼓励!⭐️ HuggingFace免费体验
2025-05-09 更新
MonoCoP: Chain-of-Prediction for Monocular 3D Object Detection
Authors:Zhihao Zhang, Abhinav Kumar, Girish Chandar Ganesan, Xiaoming Liu
Accurately predicting 3D attributes is crucial for monocular 3D object detection (Mono3D), with depth estimation posing the greatest challenge due to the inherent ambiguity in mapping 2D images to 3D space. While existing methods leverage multiple depth cues (e.g., estimating depth uncertainty, modeling depth error) to improve depth accuracy, they overlook that accurate depth prediction requires conditioning on other 3D attributes, as these attributes are intrinsically inter-correlated through the 3D to 2D projection, which ultimately limits overall accuracy and stability. Inspired by Chain-of-Thought (CoT) in large language models (LLMs), this paper proposes MonoCoP, which leverages a Chain-of-Prediction (CoP) to predict attributes sequentially and conditionally via three key designs. First, it employs a lightweight AttributeNet (AN) for each 3D attribute to learn attribute-specific features. Next, MonoCoP constructs an explicit chain to propagate these learned features from one attribute to the next. Finally, MonoCoP uses a residual connection to aggregate features for each attribute along the chain, ensuring that later attribute predictions are conditioned on all previously processed attributes without forgetting the features of earlier ones. Experimental results show that our MonoCoP achieves state-of-the-art (SoTA) performance on the KITTI leaderboard without requiring additional data and further surpasses existing methods on the Waymo and nuScenes frontal datasets.
准确预测3D属性对于单目3D目标检测(Mono3D)至关重要,深度估计由于将2D图像映射到3D空间时固有的歧义性而构成最大挑战。现有方法虽然利用多种深度线索(例如估计深度不确定性、建模深度误差)来提高深度准确性,但它们忽略了准确的深度预测需要依赖于其他3D属性,因为这些属性通过3D到2D的投影固有地相互关联,这最终限制了总体准确性和稳定性。本文受大型语言模型(LLM)中的思维链(Chain-of-Thought,CoT)的启发,提出了一种名为MonoCoP的方法,它利用预测链(Chain-of-Prediction,CoP)按顺序和有条件地预测属性,主要通过三个关键设计来实现。首先,它为每个3D属性采用轻量级的AttributeNet(AN)来学习特定于属性的特征。接下来,MonoCoP构建了一个明确的链来传播从一个属性学习到的特征到下一个属性。最后,MonoCoP使用残差连接来沿链聚合每个属性的特征,确保后续的属性预测依赖于所有先前处理的属性,同时不会忘记早期的属性特征。实验结果表明,我们的MonoCoP在KITTI排行榜上达到了最先进的性能,且无需额外数据,并且在Waymo和nuScenes正面数据集上超越了现有方法。
论文及项目相关链接
Summary
本文提出一种基于Chain-of-Prediction(CoP)的单眼3D物体检测新方法MonoCoP。该方法通过预测链(Chain-of-Prediction)顺序预测并条件化多个三维属性,提升了预测的准确性与稳定性。具体来说,它通过利用特定属性学习网络AttributeNet以及残联合来汇聚不同属性的特征,并通过明确的链条结构将特征从一个属性传播到下一个属性。实验结果在KITTI排行榜上达到了领先水平,且在Waymo和nuScenes数据集上超过了现有方法。
Key Takeaways
以下是该文本中的关键要点,以简化的形式列出:
- 单眼3D物体检测(Mono3D)中,准确预测三维属性至关重要,其中深度估计最具挑战性。
- 现有方法虽然利用多种深度线索提高深度准确性,但忽略了其他三维属性的条件预测,这些属性通过三维到二维的投影固有地相互关联。
- 本文提出的MonoCoP方法利用Chain-of-Prediction(CoP)按顺序预测并条件化多个三维属性。
- MonoCoP通过三个关键设计实现预测链:使用AttributeNet学习特定属性特征、构建明确的链条传播特征以及使用残联合汇聚不同属性的特征。
点此查看论文截图
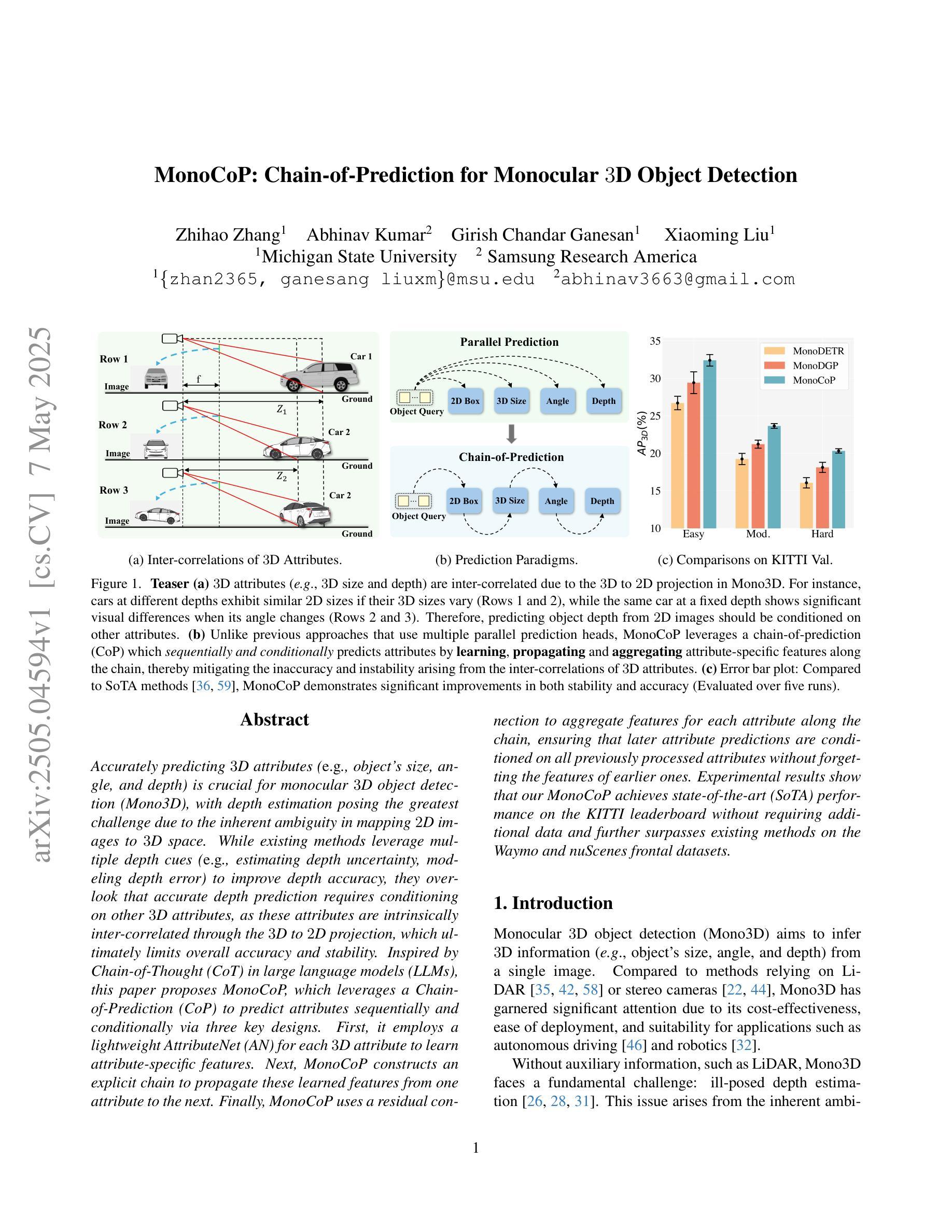
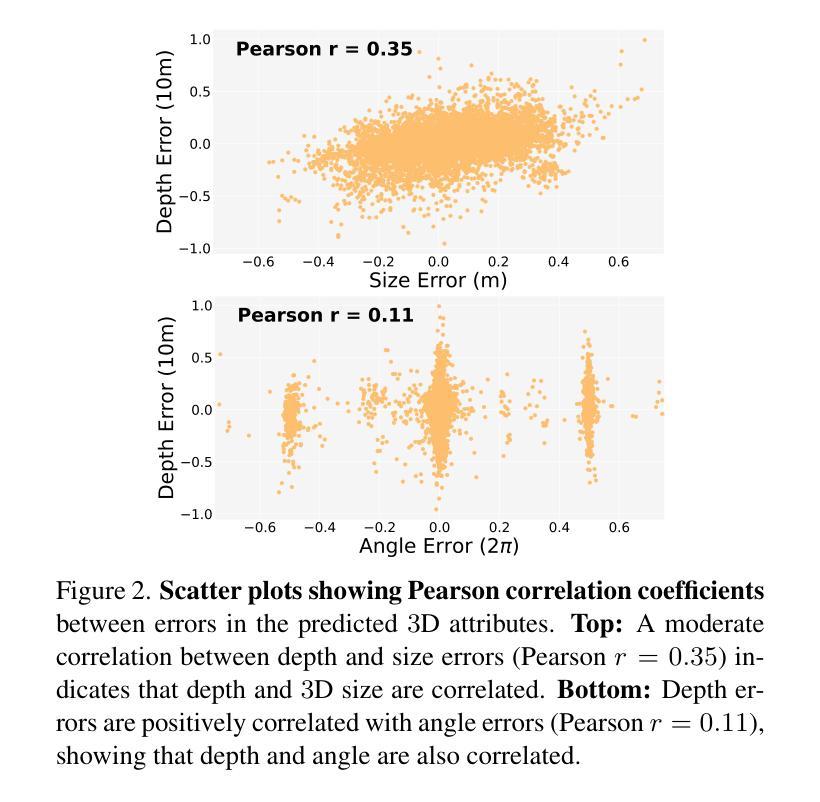
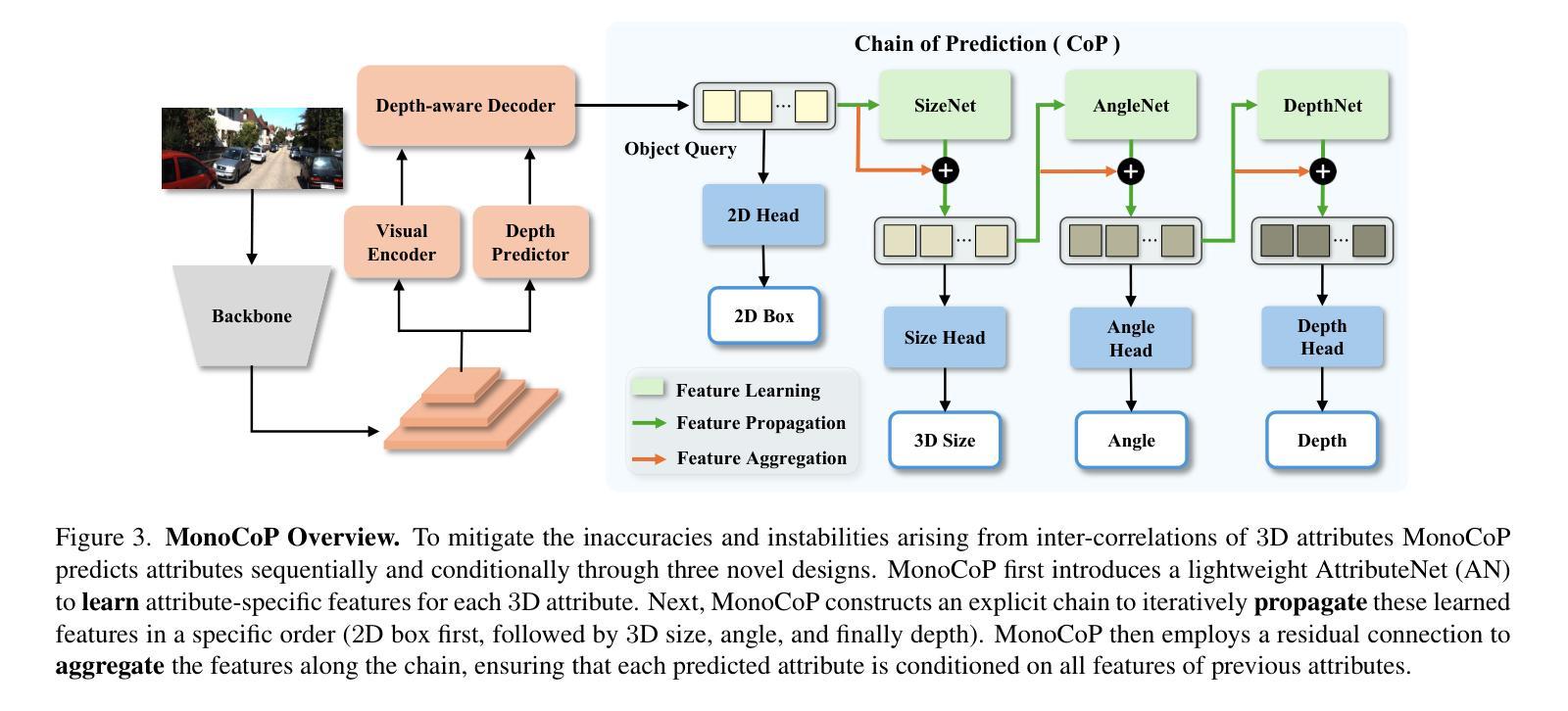
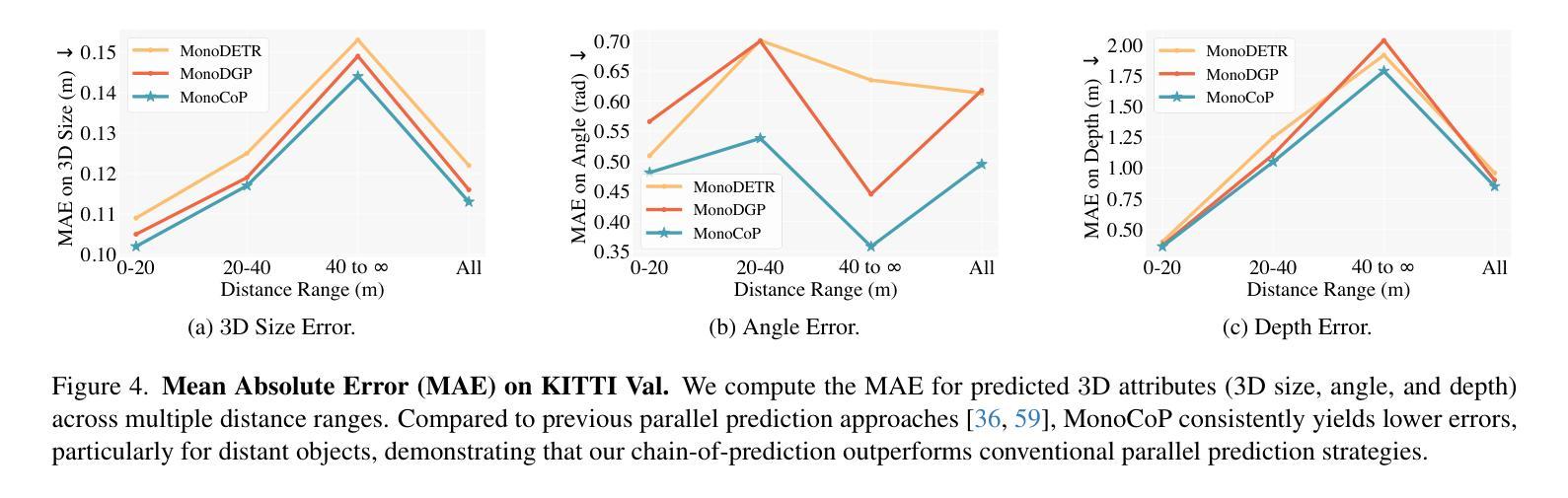
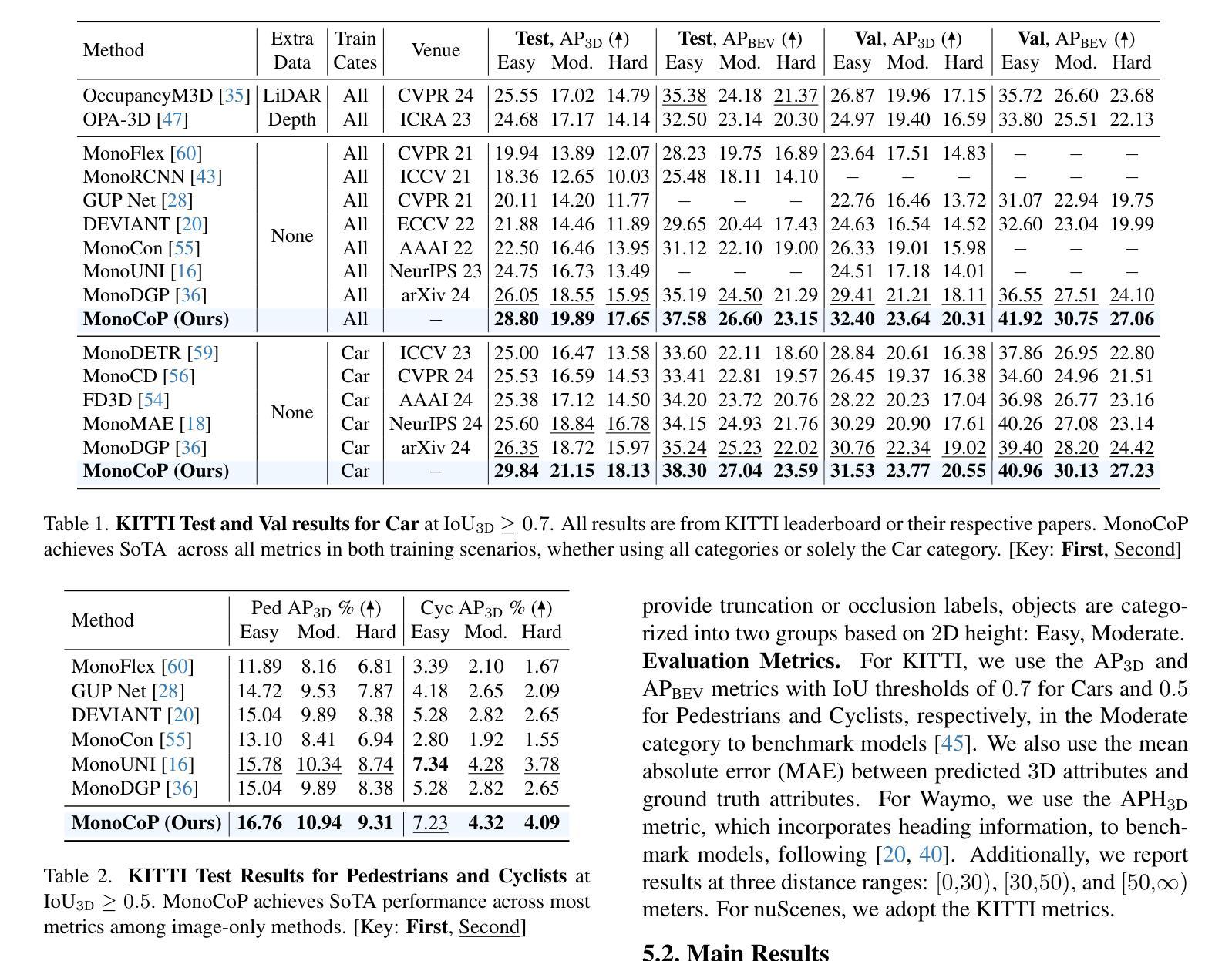