⚠️ 以下所有内容总结都来自于 大语言模型的能力,如有错误,仅供参考,谨慎使用
🔴 请注意:千万不要用于严肃的学术场景,只能用于论文阅读前的初筛!
💗 如果您觉得我们的项目对您有帮助 ChatPaperFree ,还请您给我们一些鼓励!⭐️ HuggingFace免费体验
2025-05-09 更新
MAISY: Motion-Aware Image SYnthesis for MedicalImage Motion Correction
Authors:Andrew Zhang, Hao Wang, Shuchang Ye, Michael Fulham, Jinman Kim
Patient motion during medical image acquisition causes blurring, ghosting, and distorts organs, which makes image interpretation challenging.Current state-of-the-art algorithms using Generative Adversarial Network (GAN)-based methods with their ability to learn the mappings between corrupted images and their ground truth via Structural Similarity Index Measure (SSIM) loss effectively generate motion-free images. However, we identified the following limitations: (i) they mainly focus on global structural characteristics and therefore overlook localized features that often carry critical pathological information, and (ii) the SSIM loss function struggles to handle images with varying pixel intensities, luminance factors, and variance. In this study, we propose Motion-Aware Image SYnthesis (MAISY) which initially characterize motion and then uses it for correction by: (a) leveraging the foundation model Segment Anything Model (SAM), to dynamically learn spatial patterns along anatomical boundaries where motion artifacts are most pronounced and, (b) introducing the Variance-Selective SSIM (VS-SSIM) loss which adaptively emphasizes spatial regions with high pixel variance to preserve essential anatomical details during artifact correction. Experiments on chest and head CT datasets demonstrate that our model outperformed the state-of-the-art counterparts, with Peak Signal-to-Noise Ratio (PSNR) increasing by 40%, SSIM by 10%, and Dice by 16%.
患者在医学影像采集过程中的运动会导致图像模糊、鬼影和器官扭曲,从而使图像解读具有挑战性。当前最先进的算法采用基于生成对抗网络(GAN)的方法,它们能够通过结构相似性度量(SSIM)损失来学习受污染图像与真实图像之间的映射,从而有效地生成无运动图像。但我们发现了以下局限性:(i)它们主要关注全局结构特征,因此忽略了通常携带关键病理信息的局部特征;(ii)SSIM损失函数在处理像素强度、亮度和对比度等因素变化的图像时遇到困难。本研究提出了运动感知图像合成(MAISY),它首先表征运动,然后利用运动进行校正:通过利用分段任何模型(SAM)的基础模型,动态学习解剖边界处的空间模式,其中运动伪影最为明显;并引入方差选择性SSIM(VS-SSIM)损失,该损失自适应地强调具有高像素方差的区域,以在伪影校正过程中保留重要的解剖细节。在胸部和头部CT数据集上的实验表明,我们的模型优于最先进的同类模型,峰值信噪比(PSNR)提高了40%,结构相似性度量(SSIM)提高了10%,迪氏系数(Dice)提高了16%。
论文及项目相关链接
Summary
医学图像获取过程中患者运动会导致图像模糊、鬼影和器官扭曲,使得图像解读困难。当前先进算法使用基于生成对抗网络(GAN)的方法,通过结构相似性度量(SSIM)损失学习失真图像与真实图像之间的映射,以生成无运动图像。然而,存在忽略局部特征和SSIM损失函数处理像素强度、亮度因素和方差不同的图像时的挑战。本研究提出Motion-Aware Image SYnthesis(MAISY),首先表征运动,然后利用运动进行校正:通过利用Segment Anything Model(SAM)动态学习解剖边界的空间模式,并引入Variance-Selective SSIM(VS-SSIM)损失,以在矫正伪影时保留重要的解剖细节。实验表明,该模型较先进模型有更好表现,Peak Signal-to-Noise Ratio(PSNR)提升40%,SSIM提升10%,Dice提升16%。
Key Takeaways
- 患者运动在医学图像获取中导致图像质量问题,使得图像解读困难。
- 当前GAN算法使用SSIM损失学习图像映射以消除运动影响,但存在忽略局部特征和处理像素强度差异时的挑战。
- 本研究提出MAISY模型,利用SAM动态学习解剖边界的空间模式以进行运动表征和校正。
- MAISY模型引入VS-SSIM损失,以更好地处理像素强度差异并保留重要解剖细节。
- 实验表明MAISY模型较先进模型表现更优,PSNR、SSIM和Dice等指标有所提升。
- MAISY模型能够更有效地处理胸部和头部CT数据集。
点此查看论文截图
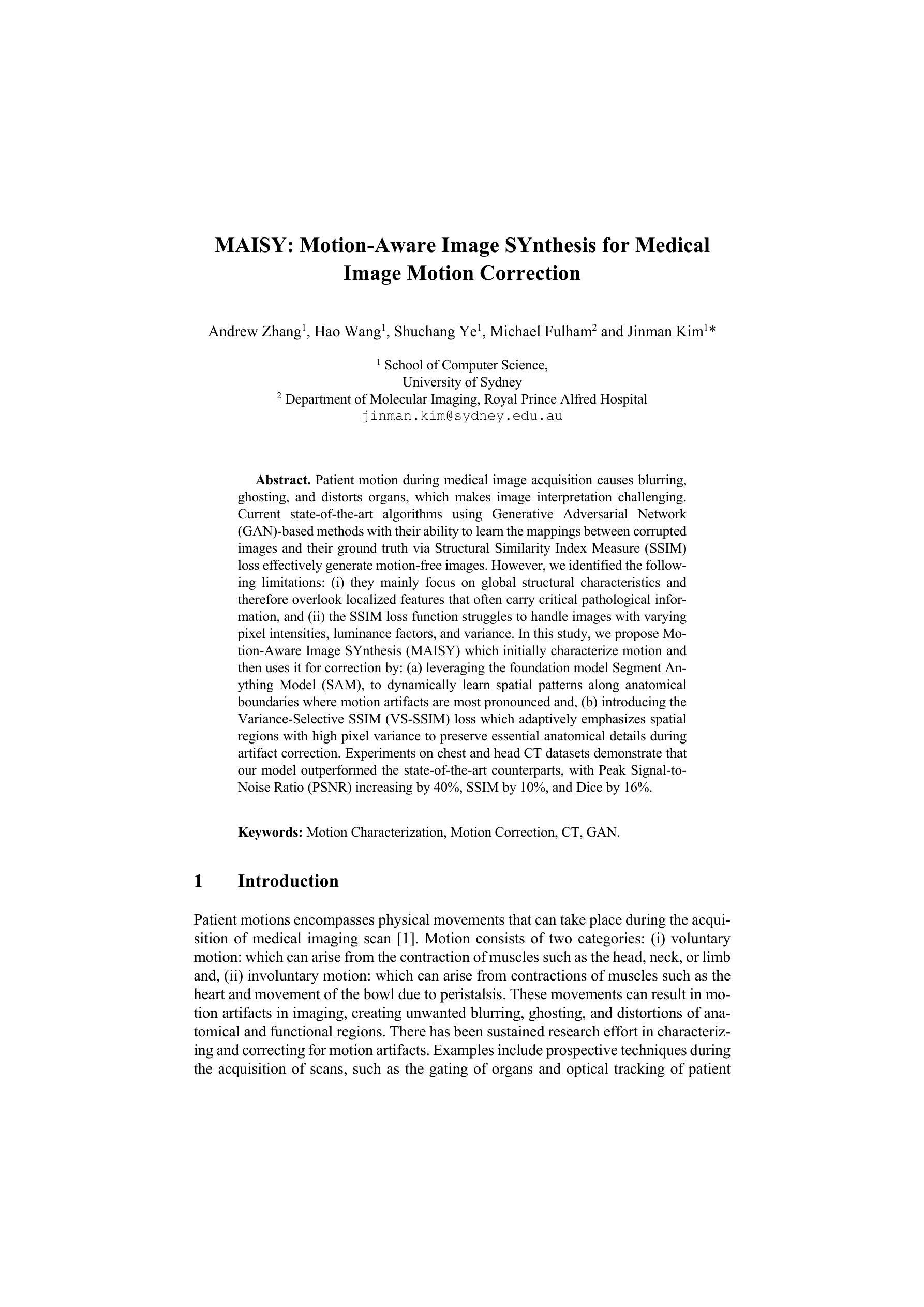
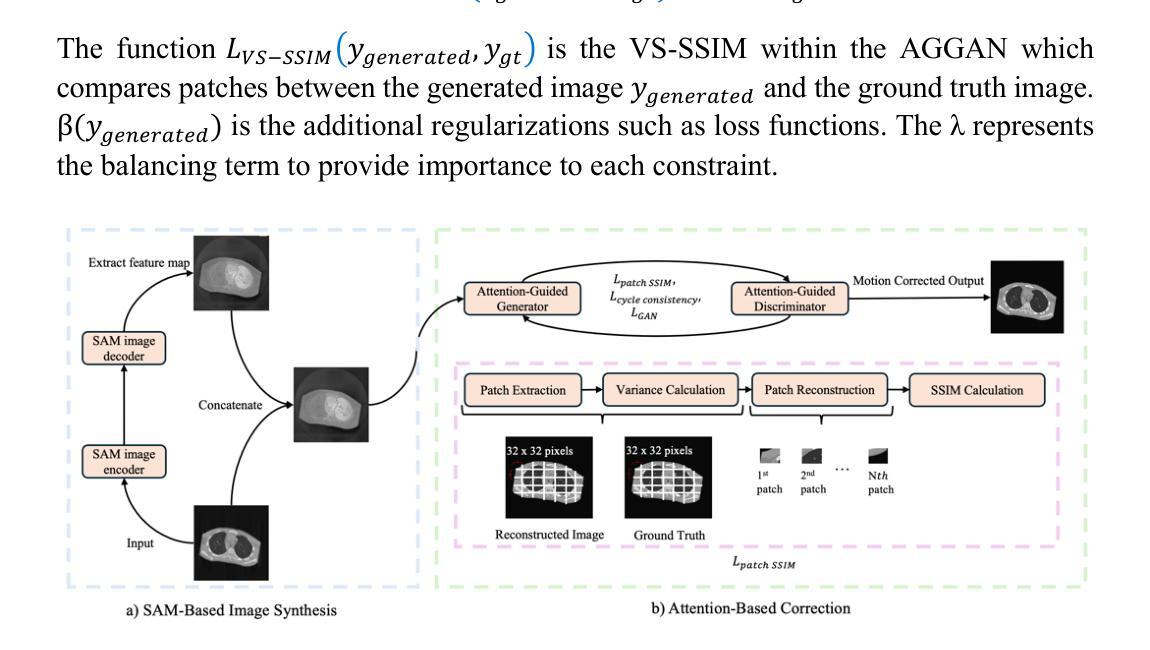
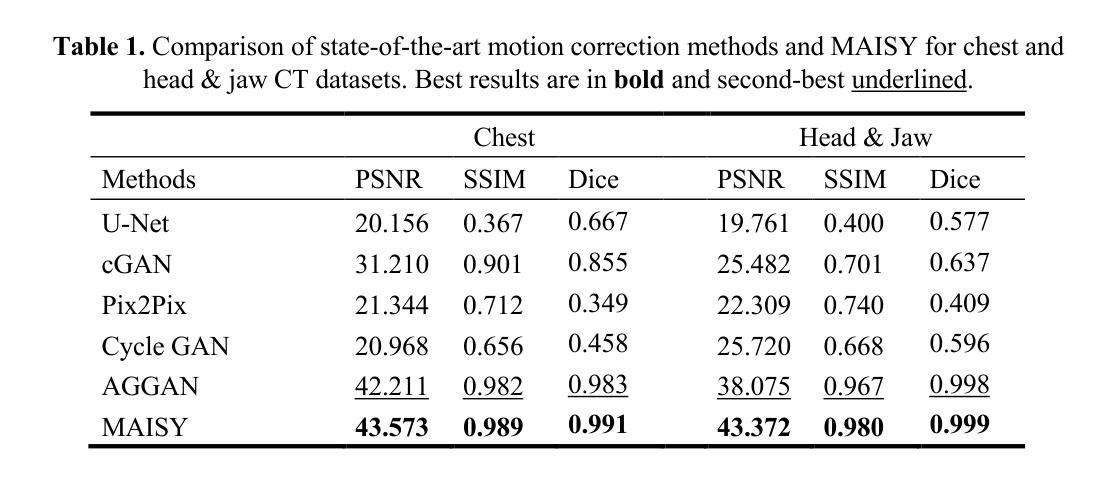