⚠️ 以下所有内容总结都来自于 大语言模型的能力,如有错误,仅供参考,谨慎使用
🔴 请注意:千万不要用于严肃的学术场景,只能用于论文阅读前的初筛!
💗 如果您觉得我们的项目对您有帮助 ChatPaperFree ,还请您给我们一些鼓励!⭐️ HuggingFace免费体验
2025-05-09 更新
Advancing Zero-shot Text-to-Speech Intelligibility across Diverse Domains via Preference Alignment
Authors:Xueyao Zhang, Yuancheng Wang, Chaoren Wang, Ziniu Li, Zhuo Chen, Zhizheng Wu
Modern zero-shot text-to-speech (TTS) systems, despite using extensive pre-training, often struggle in challenging scenarios such as tongue twisters, repeated words, code-switching, and cross-lingual synthesis, leading to intelligibility issues. To address these limitations, this paper leverages preference alignment techniques, which enable targeted construction of out-of-pretraining-distribution data to enhance performance. We introduce a new dataset, named the Intelligibility Preference Speech Dataset (INTP), and extend the Direct Preference Optimization (DPO) framework to accommodate diverse TTS architectures. After INTP alignment, in addition to intelligibility, we observe overall improvements including naturalness, similarity, and audio quality for multiple TTS models across diverse domains. Based on that, we also verify the weak-to-strong generalization ability of INTP for more intelligible models such as CosyVoice 2 and Ints. Moreover, we showcase the potential for further improvements through iterative alignment based on Ints. Audio samples are available at https://intalign.github.io/.
尽管现代零样本文本到语音(TTS)系统采用了大量的预训练,但在应对挑战场景时仍面临困难,如绕口令、重复单词、代码切换和跨语言合成等,导致语音清晰度问题。为了克服这些局限性,本文采用偏好对齐技术,该技术能够实现超出预训练分布数据的针对性构建,从而提高性能。我们引入了一个新的数据集,名为“清晰度偏好语音数据集(INTP)”,并将直接偏好优化(DPO)框架扩展到适应多种TTS架构。经过INTP对齐后,除了清晰度外,我们还观察到整体改进,包括自然度、相似性和音频质量,这些改进适用于多个跨不同领域的TTS模型。基于此,我们还验证了INTP对于如CosyVoice 2和Ints等更具清晰度的模型的弱到强泛化能力。此外,我们通过基于Ints的迭代对齐展示了进一步改进的潜力。音频样本可在https://intalign.github.io/获取。
论文及项目相关链接
Summary
本文介绍了现代零样本文本到语音(TTS)系统在复杂场景中的局限性,如连续重复的单词和多语言合成等。为解决这些问题,文章采用了偏好对齐技术,通过构建超出预训练分布的数据集来提高性能。文章引入了一个新的数据集——Intelligibility Preference Speech Dataset(INTP),并扩展了Direct Preference Optimization(DPO)框架以适应不同的TTS架构。通过INTP对齐后,不仅提高了语音的可懂度,还观察到多个TTS模型在不同领域的整体性能有所提升,包括自然度、相似性和音质。此外,验证了INTP对于更智能模型的弱到强泛化能力,如CosyVoice 2和Ints等。同时展示了通过迭代对齐进一步提高性能的潜力。
Key Takeaways
- 现代零样本TTS系统在复杂场景中面临挑战,如连续重复的单词和多语言合成等,导致语音可懂度问题。
- 偏好对齐技术被引入来解决这些问题,通过构建超出预训练分布的数据集来增强性能。
- 引入了一个新的数据集——INTP,用于提高语音的可懂度和整体性能。
- 扩展了DPO框架以适应不同的TTS架构。
- 通过INTP对齐后,多个TTS模型的整体性能有所提升,包括自然度、相似性和音质等方面。
- INTP具有弱到强的泛化能力,适用于更智能的模型如CosyVoice 2和Ints等。
- 通过迭代对齐,有潜力进一步提高性能。
点此查看论文截图
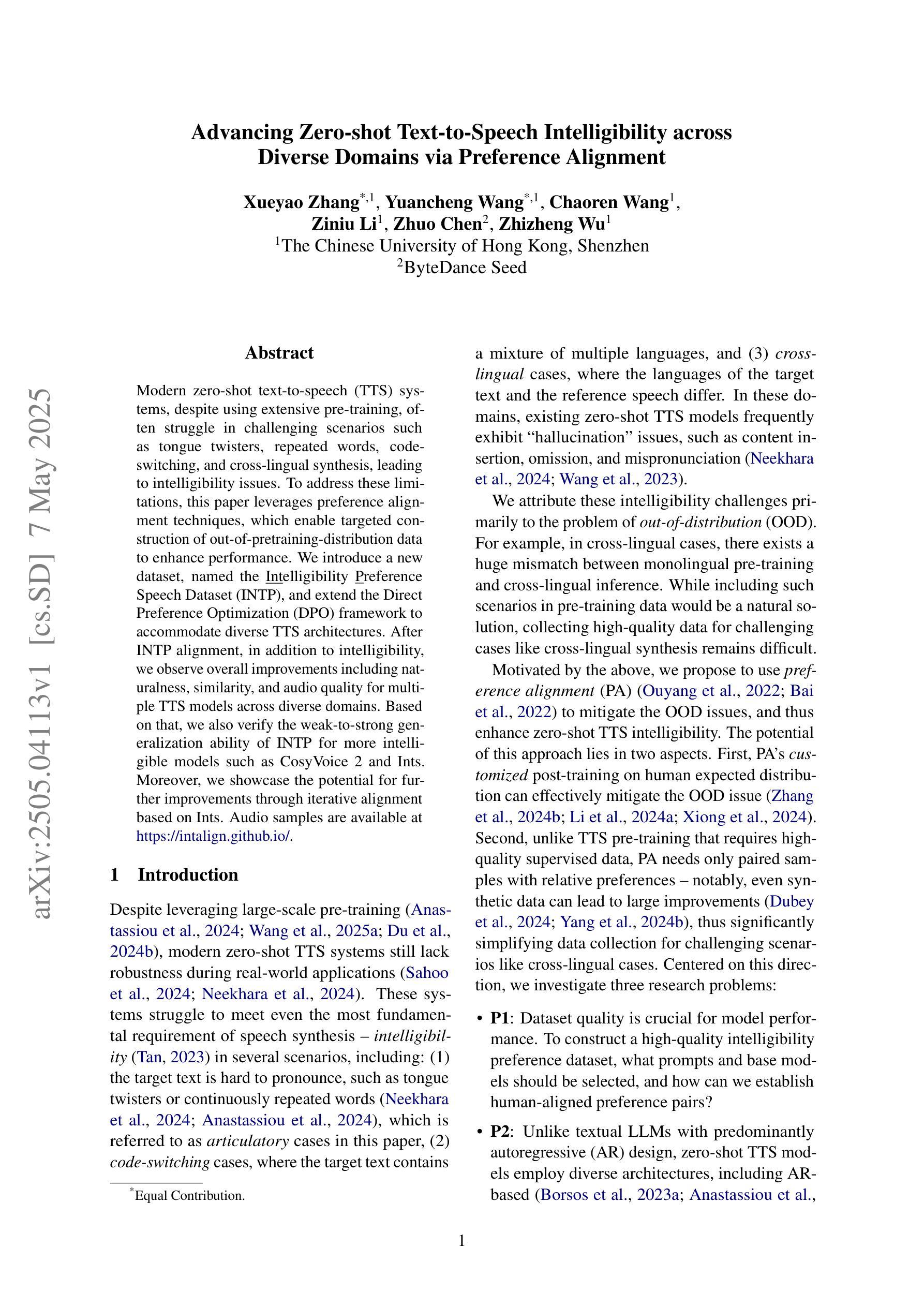
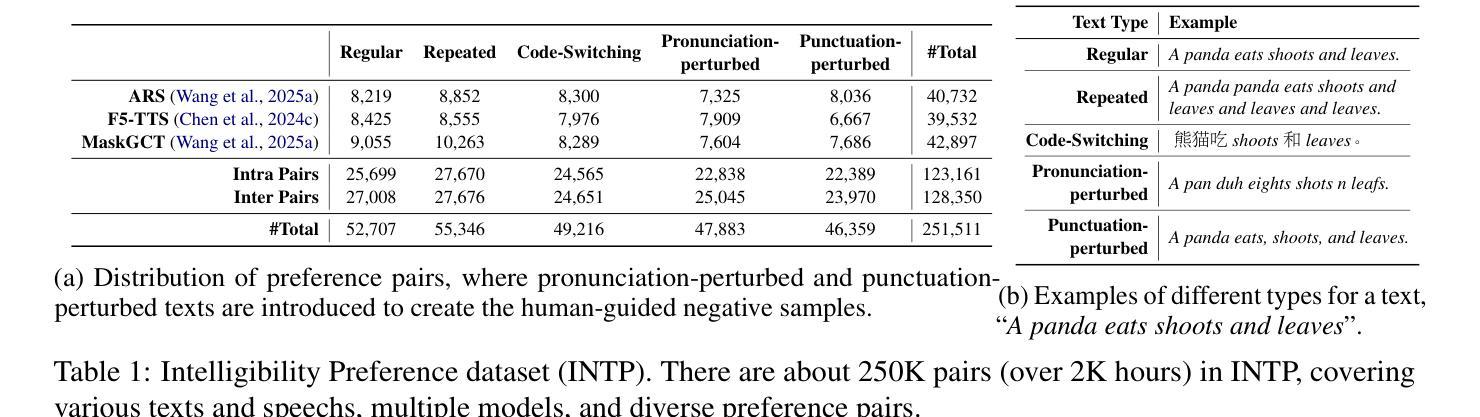
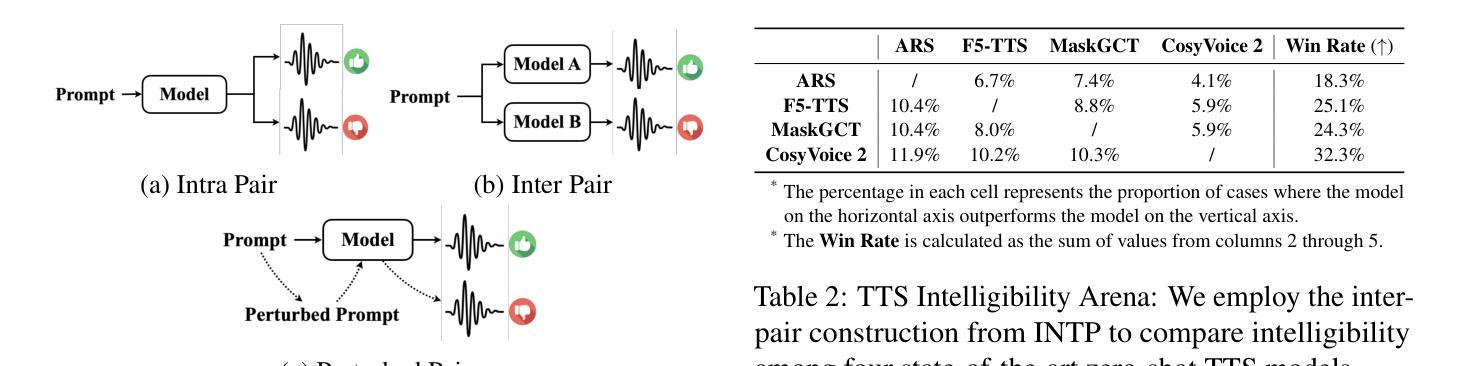
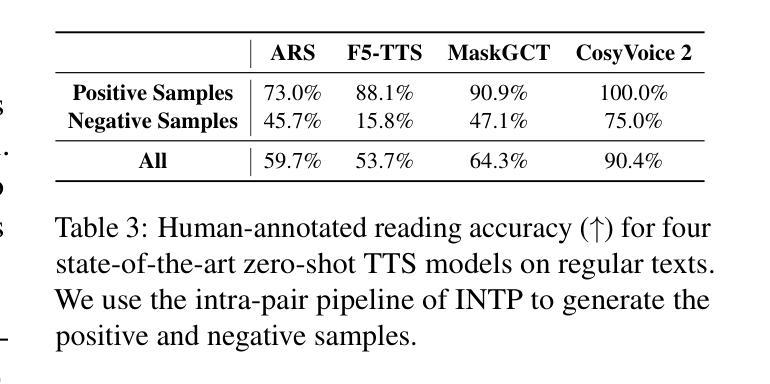
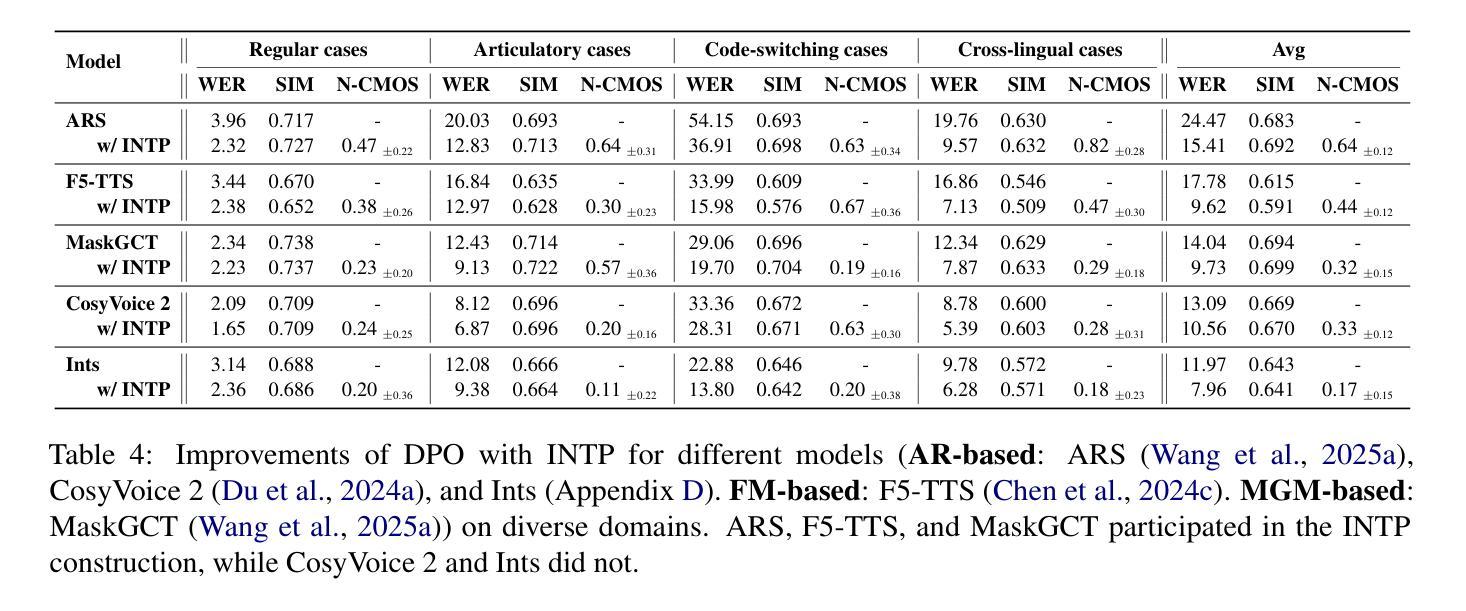
SelectTTS: Synthesizing Anyone’s Voice via Discrete Unit-Based Frame Selection
Authors:Ismail Rasim Ulgen, Shreeram Suresh Chandra, Junchen Lu, Berrak Sisman
Synthesizing the voices of unseen speakers remains a persisting challenge in multi-speaker text-to-speech (TTS). Existing methods model speaker characteristics through speaker conditioning during training, leading to increased model complexity and limiting reproducibility and accessibility. A lower-complexity method would enable speech synthesis research with limited computational and data resources to reach to a wider use. To this end, we propose SelectTTS, a simple and effective alternative. SelectTTS selects appropriate frames from the target speaker and decodes them using frame-level self-supervised learning (SSL) features. We demonstrate that this approach can effectively capture speaker characteristics for unseen speakers and achieves performance comparable to state-of-the-art multi-speaker TTS frameworks on both objective and subjective metrics. By directly selecting frames from the target speaker’s speech, SelectTTS enables generalization to unseen speakers with significantly lower model complexity. Compared to baselines such as XTTS-v2 and VALL-E, SelectTTS achieves better speaker similarity while reducing model parameters by over 8x and training data requirements by 270x.
在多说话者文本转语音(TTS)中,合成未见说话者的声音仍然是一个持续存在的挑战。现有方法通过训练过程中的说话者条件来模拟说话者特征,这增加了模型复杂度并限制了可重复性和可访问性。一种复杂度较低的方法将使使用有限计算和数据资源的语音合成研究能够更广泛地应用。为此,我们提出了SelectTTS,这是一个简单有效的替代方案。SelectTTS从目标说话者中选择适当的帧,并使用帧级自监督学习(SSL)特征进行解码。我们证明这种方法可以有效地捕捉未见说话者的特征,并在客观和主观指标上实现与最新多说话者TTS框架相当的性能。通过直接从目标说话者的语音中选择帧,SelectTTS能够以显著降低的模型复杂度泛化到未见说话者。与XTTS-v2和VALL-E等基线相比,SelectTTS在降低模型参数超过8倍和训练数据需求减少270倍的情况下,实现了更好的说话者相似性。
论文及项目相关链接
PDF Submitted to IEEE Signal Processing Letters
摘要
在多说话者文本转语音(TTS)中,合成未说话者的声音仍然是一个持续的挑战。现有方法通过训练过程中的说话者条件建模说话者特征,导致模型复杂度增加,限制可重复性和可访问性。为此,我们提出SelectTTS,一种简单有效的替代方法。SelectTTS从目标说话者选择适当的帧,并使用帧级自我监督学习(SSL)特征进行解码。我们证明这种方法可以有效地捕捉未说话者的特征,并在客观和主观指标上与最先进的多说话者TTS框架实现相当的性能。通过直接从目标说话者的语音中选择帧,SelectTTS能够以显著更低的模型复杂度泛化到未说话者。与XTTS-v2和VALL-E等基线相比,SelectTTS在减少模型参数超过8倍、训练数据需求减少270倍的情况下,实现了更好的说话者相似性。
要点
- 多说话者文本转语音(TTS)中合成未说话者声音是挑战。
- 现有方法通过训练过程中的说话者条件建模,增加模型复杂度和限制可访问性。
- 提出SelectTTS,简单有效的替代方法。
- SelectTTS从目标说话者选择适当的帧并使用帧级自我监督学习(SSL)特征解码。
- SelectTTS能有效捕捉未说话者的特征,性能与最先进的TTS框架相当。
- SelectTTS泛化能力强,模型复杂度低。
点此查看论文截图
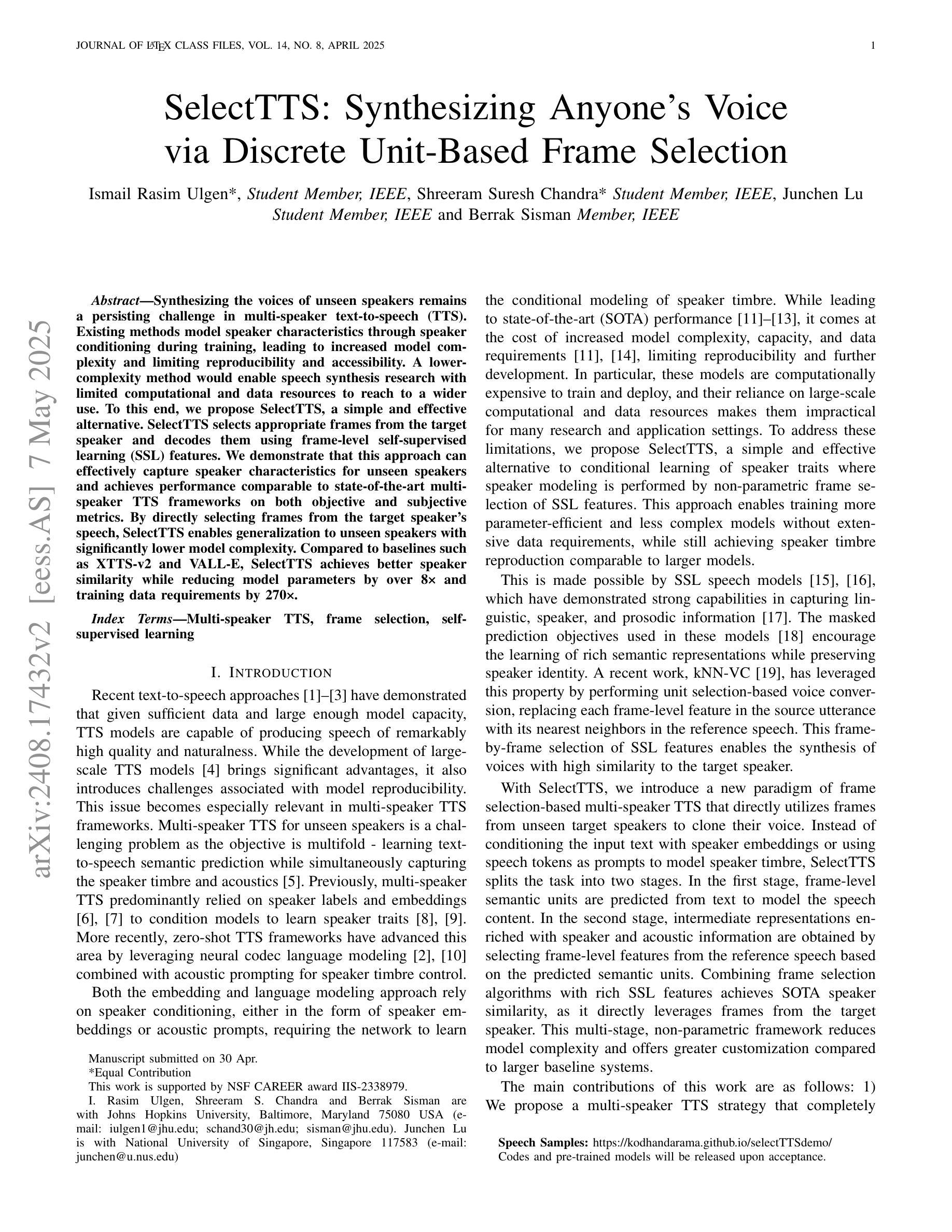
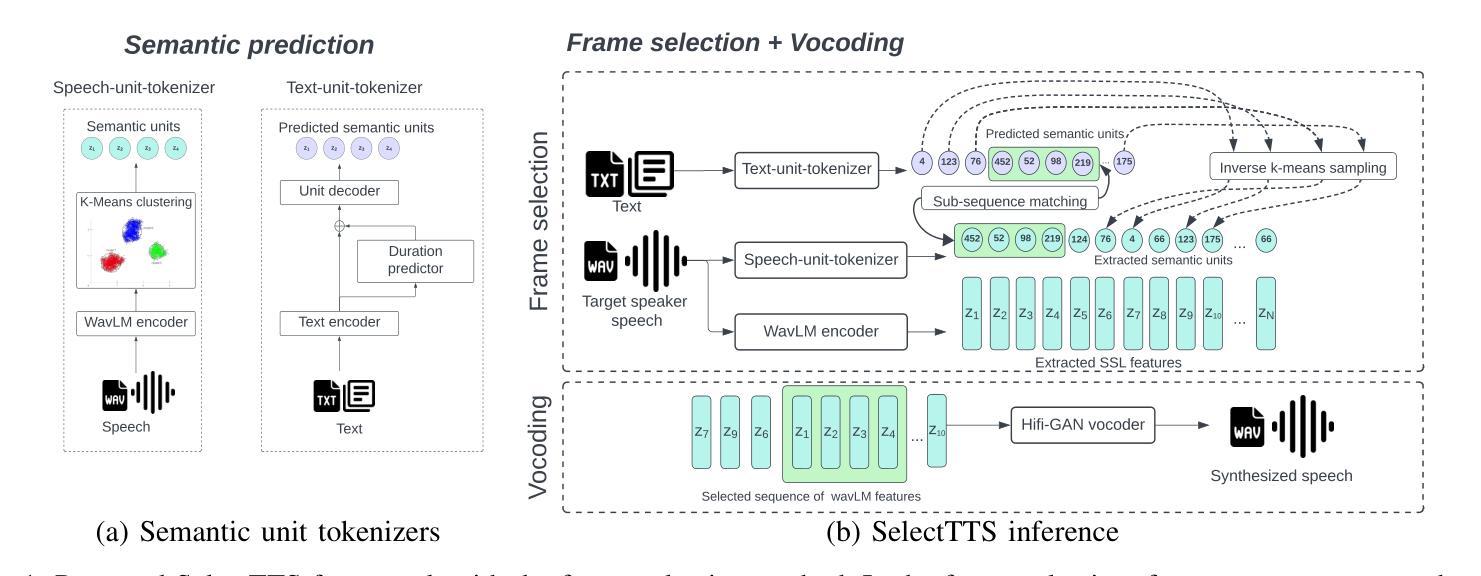
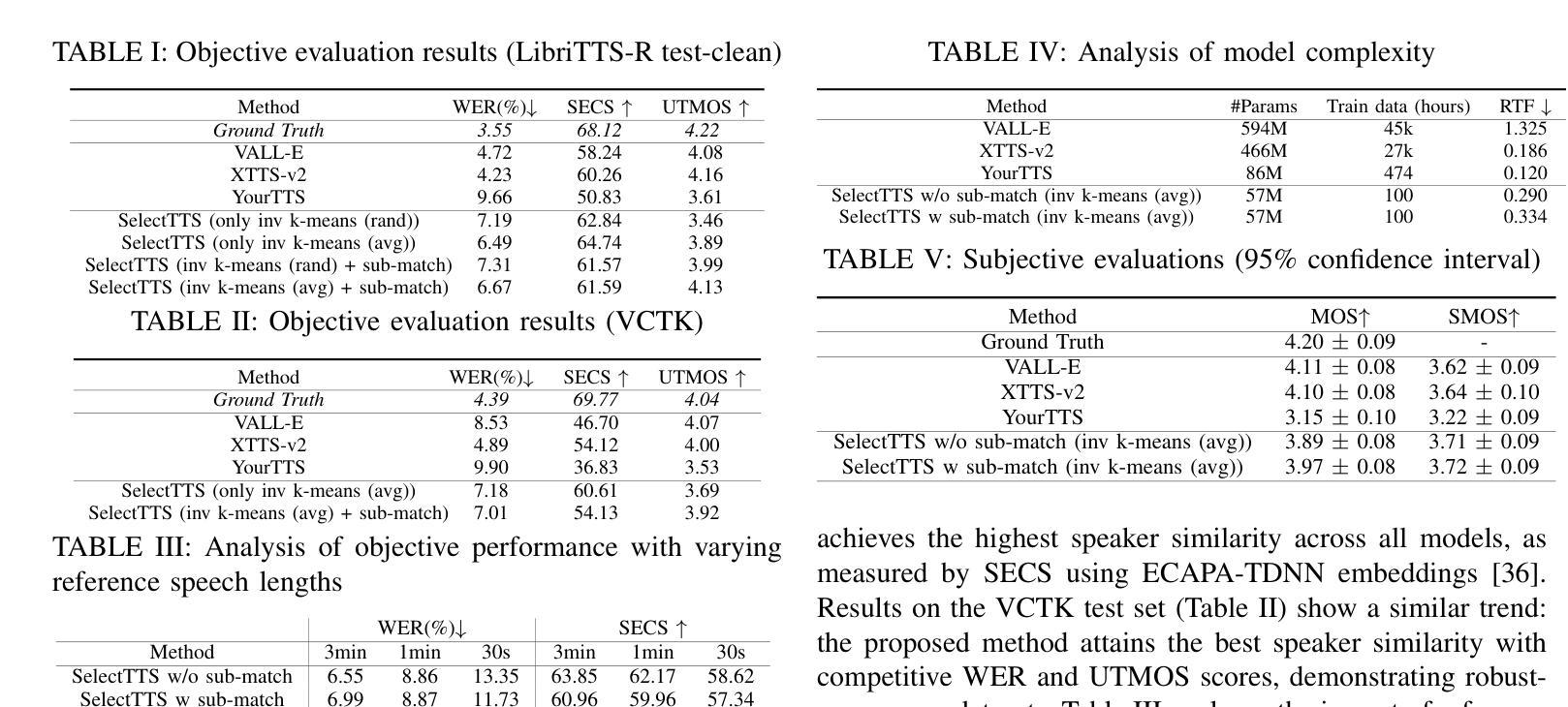