⚠️ 以下所有内容总结都来自于 大语言模型的能力,如有错误,仅供参考,谨慎使用
🔴 请注意:千万不要用于严肃的学术场景,只能用于论文阅读前的初筛!
💗 如果您觉得我们的项目对您有帮助 ChatPaperFree ,还请您给我们一些鼓励!⭐️ HuggingFace免费体验
2025-05-09 更新
PAHA: Parts-Aware Audio-Driven Human Animation with Diffusion Model
Authors:Y. B. Wang, S. Z. Zhou, J. F. Wu, T. Hu, J. N. Zhang, Y. Liu
Audio-driven human animation technology is widely used in human-computer interaction, and the emergence of diffusion models has further advanced its development. Currently, most methods rely on multi-stage generation and intermediate representations, resulting in long inference time and issues with generation quality in specific foreground regions and audio-motion consistency. These shortcomings are primarily due to the lack of localized fine-grained supervised guidance. To address above challenges, we propose PAHA, an end-to-end audio-driven upper-body human animation framework with diffusion model. We introduce two key methods: Parts-Aware Re-weighting (PAR) and Parts Consistency Enhancement (PCE). PAR dynamically adjusts regional training loss weights based on pose confidence scores, effectively improving visual quality. PCE constructs and trains diffusion-based regional audio-visual classifiers to improve the consistency of motion and co-speech audio. Afterwards, we design two novel inference guidance methods for the foregoing classifiers, Sequential Guidance (SG) and Differential Guidance (DG), to balance efficiency and quality respectively. Additionally, we build CNAS, the first public Chinese News Anchor Speech dataset, to advance research and validation in this field. Extensive experimental results and user studies demonstrate that PAHA significantly outperforms existing methods in audio-motion alignment and video-related evaluations. The codes and CNAS dataset will be released upon acceptance.
音频驱动的人形动画技术广泛应用于人机交互领域,扩散模型的出现进一步推动了其发展。目前,大多数方法依赖于多阶段生成和中间表示,导致推理时间长,特定前景区域生成质量和音频运动一致性存在问题。这些缺点主要是由于缺乏局部精细监督指导。为了解决上述挑战,我们提出了基于扩散模型的端到端音频驱动上身人体动画框架PAHA。我们介绍了两种关键方法:零件感知重新加权(PAR)和零件一致性增强(PCE)。PAR根据姿势置信度分数动态调整区域训练损失权重,有效提高视觉质量。PCE构建并训练基于扩散的区域音频视觉分类器,提高运动一致性和语音音频的协调性。之后,我们为前述分类器设计了两种新颖推理指导方法,即顺序指导(SG)和差异指导(DG),以平衡效率和质量。此外,我们建立了首个公共中文新闻主播语音数据集CNAS,以推动该领域的研究和验证。大量的实验结果和用户研究表明,PAHA在音频运动对齐和视频相关评估方面显著优于现有方法。代码和CNAS数据集将在接受后发布。
论文及项目相关链接
Summary
本文介绍了音频驱动的人体动画技术的最新进展,提出了一种基于扩散模型的端到端人体动画框架PAHA,并介绍了其中的两大关键方法:零件感知重加权(PAR)和零件一致性增强(PCE)。该框架能有效解决现有技术的不足,提高音频与运动的一致性及生成质量。同时,构建了首个中文新闻主播语音数据集CNAS,以推动相关领域的研究和验证。
Key Takeaways
- 音频驱动的人体动画技术在人机交互中广泛应用,扩散模型的出现进一步推动了其发展。
- 当前方法存在长期推理时间和生成质量的问题,特别是在前景区域和音频运动一致性方面。
- PAHA框架通过引入PAR和PCE两大关键方法,有效提高音频与运动的一致性及生成质量。
- PAR方法能动态调整区域训练损失权重,提高视觉效果。
- PCE方法构建并训练基于扩散的区域音频视觉分类器,改善运动一致性。
- 框架中还设计了两种新型推理指导方法SG和DG,以平衡效率和质量。
- 构建了首个中文新闻主播语音数据集CNAS,用于推动研究和验证。
点此查看论文截图
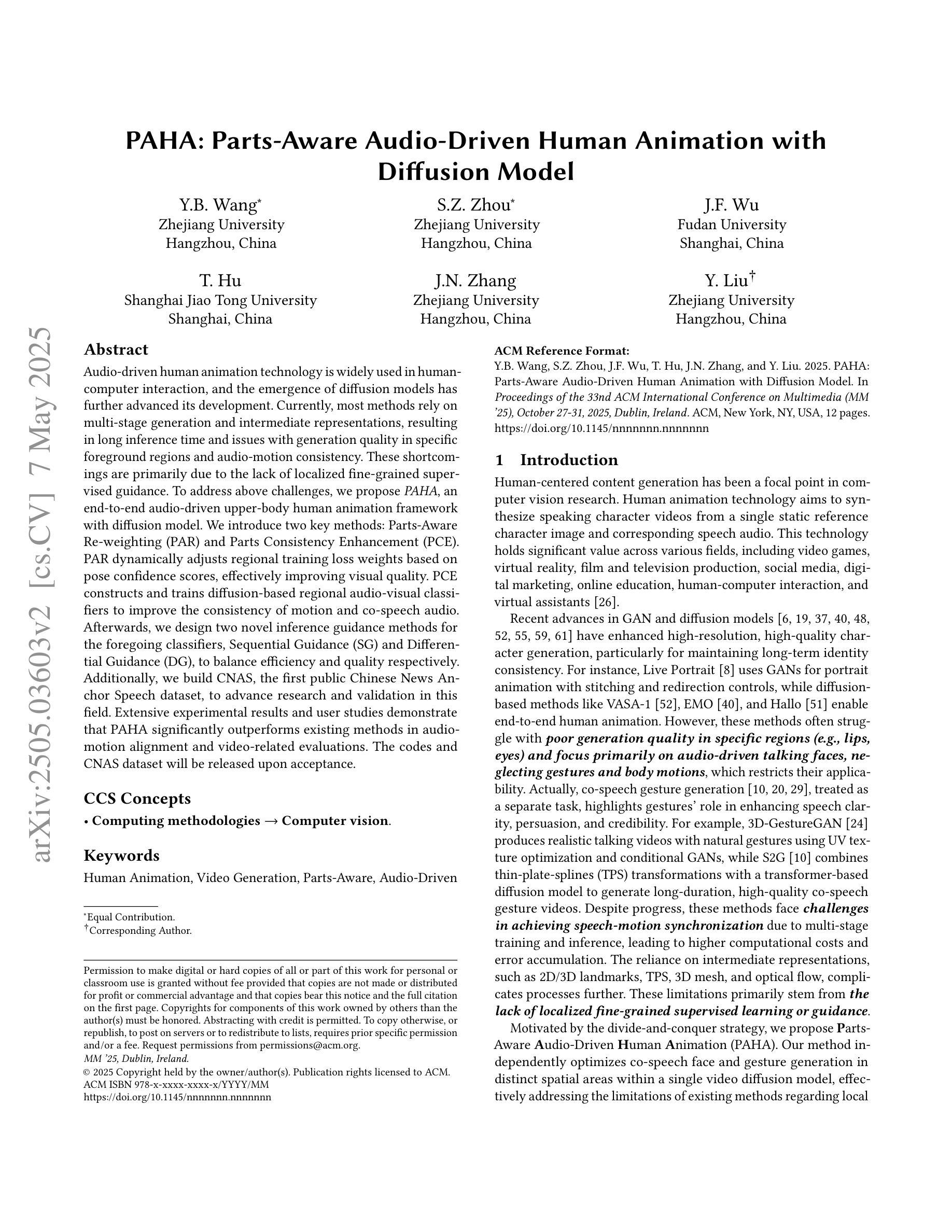
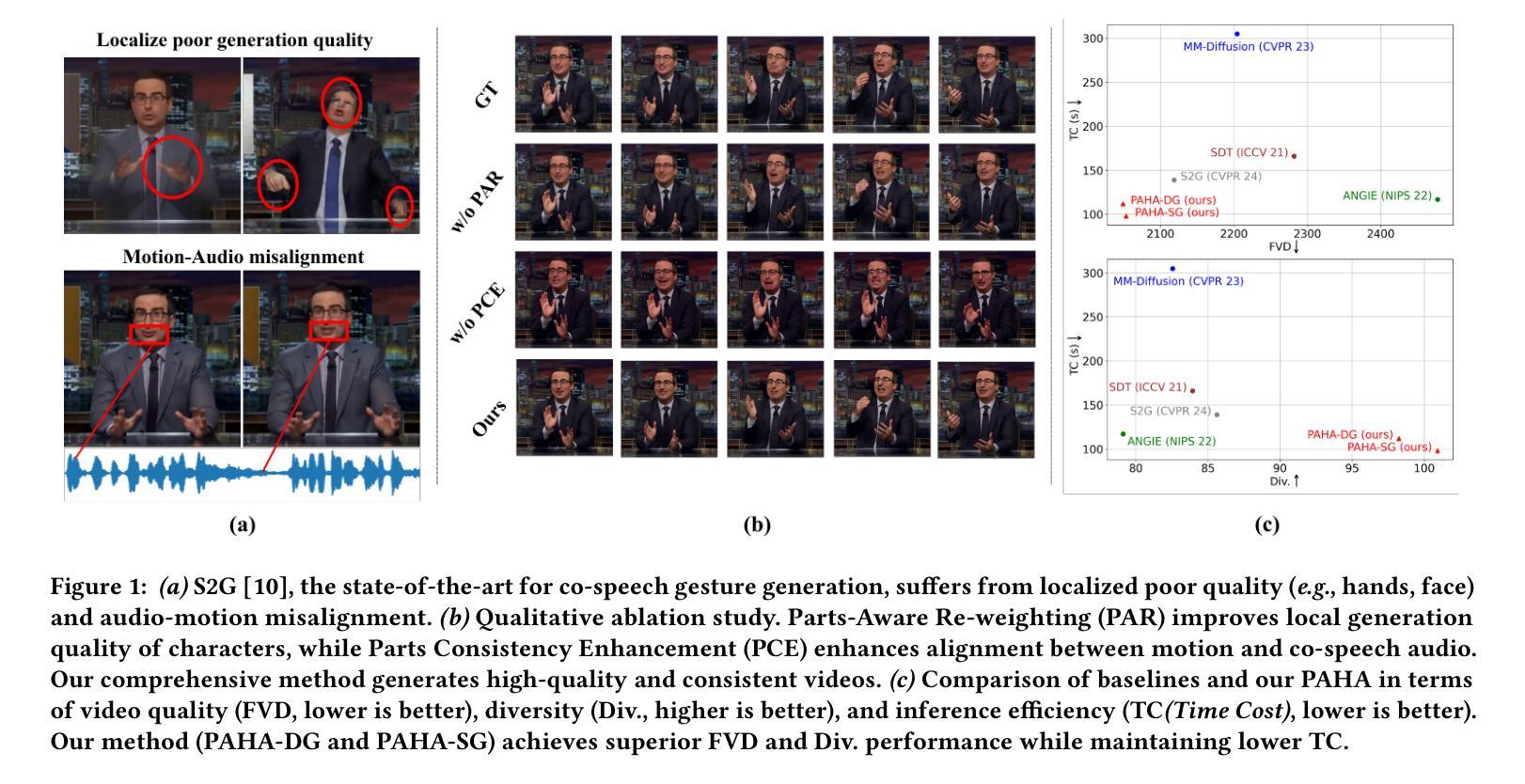
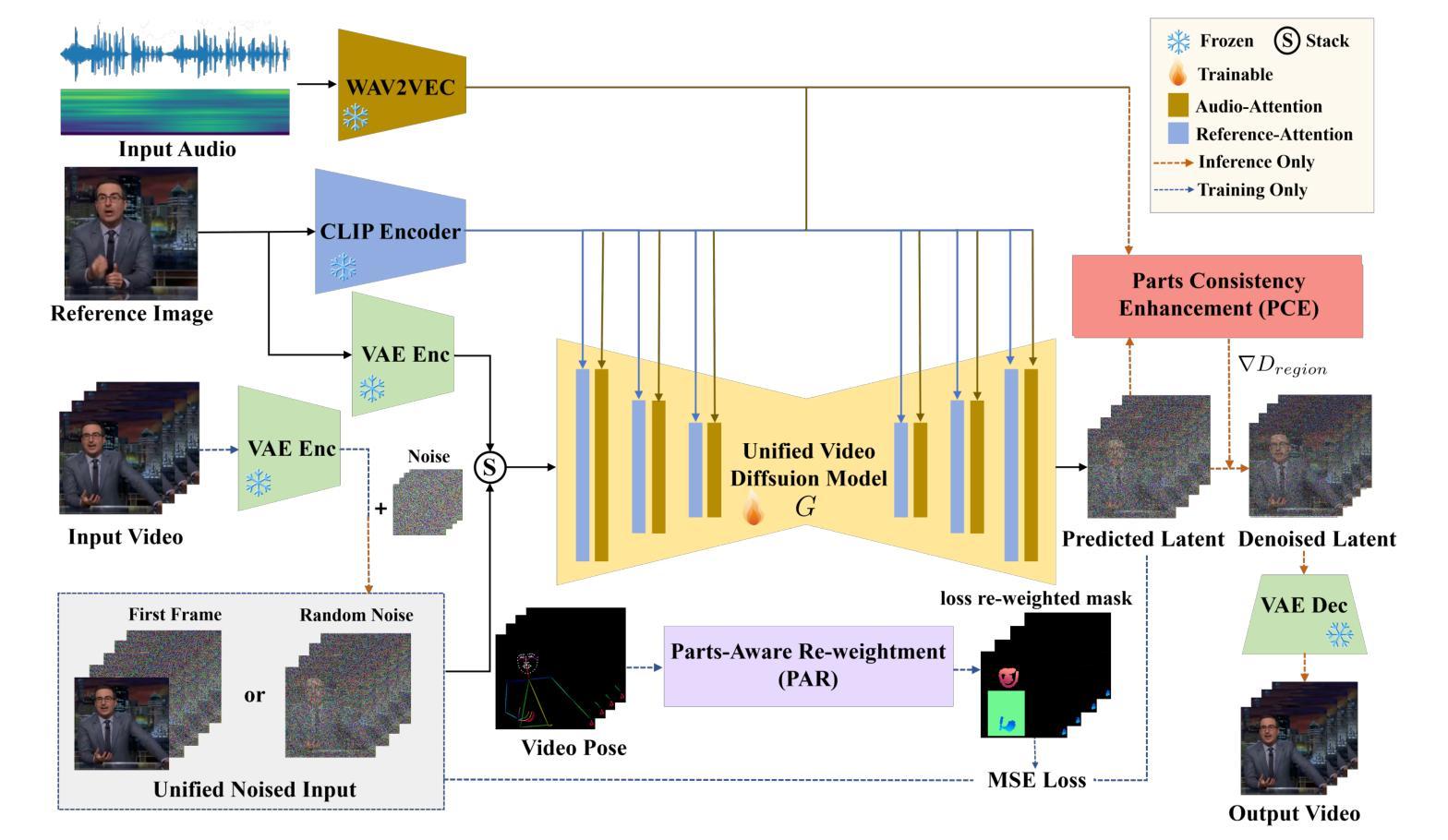
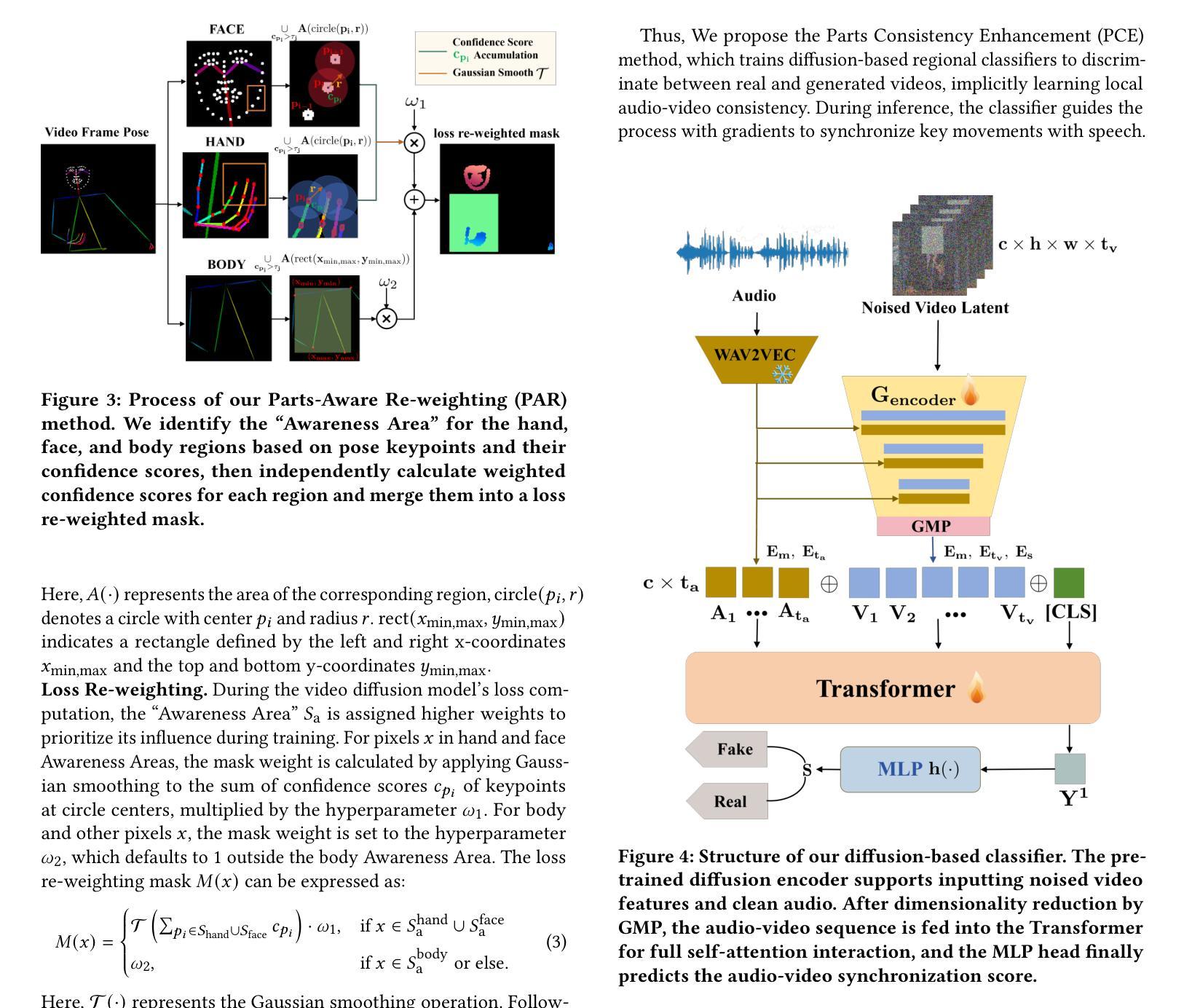
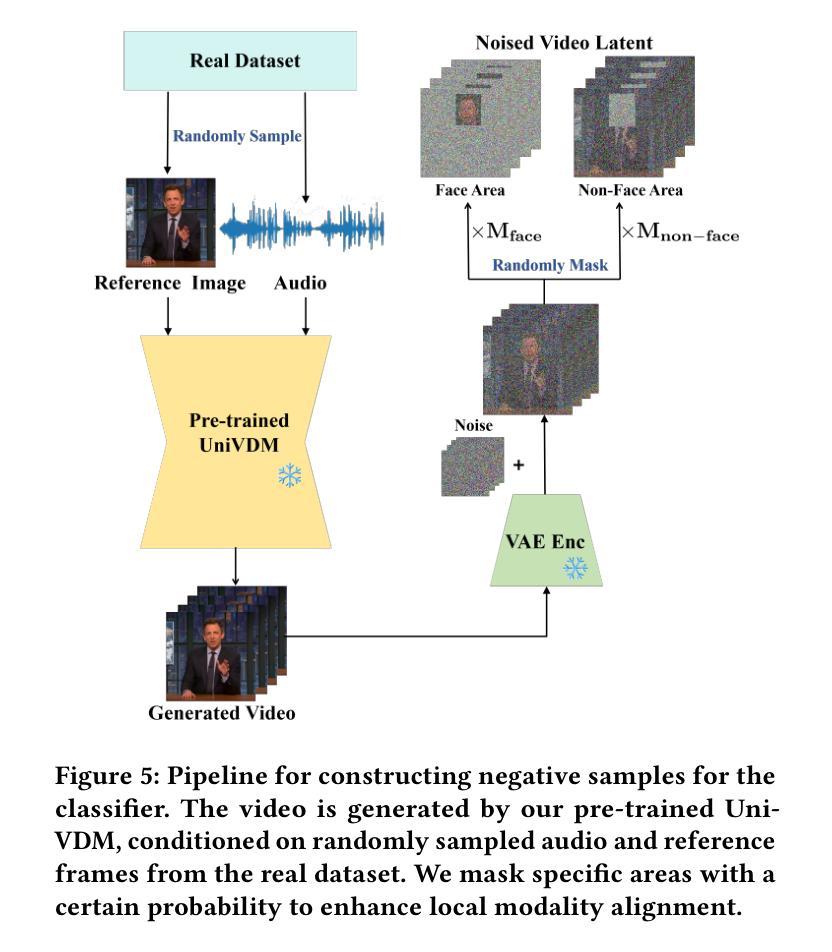