⚠️ 以下所有内容总结都来自于 大语言模型的能力,如有错误,仅供参考,谨慎使用
🔴 请注意:千万不要用于严肃的学术场景,只能用于论文阅读前的初筛!
💗 如果您觉得我们的项目对您有帮助 ChatPaperFree ,还请您给我们一些鼓励!⭐️ HuggingFace免费体验
2025-05-10 更新
FlexSpeech: Towards Stable, Controllable and Expressive Text-to-Speech
Authors:Linhan Ma, Dake Guo, He Wang, Jin Xu, Lei Xie
Current speech generation research can be categorized into two primary classes: non-autoregressive and autoregressive. The fundamental distinction between these approaches lies in the duration prediction strategy employed for predictable-length sequences. The NAR methods ensure stability in speech generation by explicitly and independently modeling the duration of each phonetic unit. Conversely, AR methods employ an autoregressive paradigm to predict the compressed speech token by implicitly modeling duration with Markov properties. Although this approach improves prosody, it does not provide the structural guarantees necessary for stability. To simultaneously address the issues of stability and naturalness in speech generation, we propose FlexSpeech, a stable, controllable, and expressive TTS model. The motivation behind FlexSpeech is to incorporate Markov dependencies and preference optimization directly on the duration predictor to boost its naturalness while maintaining explicit modeling of the phonetic units to ensure stability. Specifically, we decompose the speech generation task into two components: an AR duration predictor and a NAR acoustic model. The acoustic model is trained on a substantial amount of data to learn to render audio more stably, given reference audio prosody and phone durations. The duration predictor is optimized in a lightweight manner for different stylistic variations, thereby enabling rapid style transfer while maintaining a decoupled relationship with the specified speaker timbre. Experimental results demonstrate that our approach achieves SOTA stability and naturalness in zero-shot TTS. More importantly, when transferring to a specific stylistic domain, we can accomplish lightweight optimization of the duration module solely with about 100 data samples, without the need to adjust the acoustic model, thereby enabling rapid and stable style transfer.
目前的语音生成研究可以主要分为两大类:非自回归和自回归。这两种方法之间的根本区别在于用于预测长度序列的持续时间预测策略。NAR方法通过显式且独立地建模每个语音单元的持续时间,确保语音生成的稳定性。相反,AR方法采用自回归范式,通过隐式地利用马尔可夫属性对压缩语音符号进行预测。尽管这种方法改善了韵律,但它并没有提供保证稳定性的必要结构。为了解决语音生成中的稳定性和自然性问题,我们提出了FlexSpeech,一个稳定、可控、表达性强的TTS模型。FlexSpeech的动机是在持续时间预测器上直接融入马尔可夫依赖关系和偏好优化,以提高其自然性,同时保持对语音单元的显式建模以确保稳定性。具体来说,我们将语音生成任务分解为两个组件:AR持续时间预测器和NAR声学模型。声学模型经过大量数据训练,能够在给定参考音频韵律和语音单元持续时间的情况下,更稳定地呈现音频。持续时间预测器以轻巧的方式进行优化,以适应不同的风格变化,从而在保持与指定演讲者音色的分离关系的同时实现快速风格转换。实验结果表明,我们的方法在实现零样本TTS时达到了最先进的稳定性和自然性。更重要的是,在转移到特定风格领域时,我们仅使用约100个数据样本就可以完成持续时间模块的轻量化优化,而无需调整声学模型,从而实现快速稳定的风格转换。
论文及项目相关链接
PDF 10 pages, 5 figures
摘要
当前语音生成研究可分为非自回归和自回归两大类。它们的根本区别在于对可预测长度序列的持续时间预测策略。NAR方法通过明确独立地建模每个语音单元的持续时间,确保了语音生成的稳定性。相比之下,AR方法采用自回归范式预测压缩语音标记,通过马尔可夫属性隐式建模持续时间。虽然这种方法改善了韵律,但它没有提供保证稳定性的结构。为了解决语音生成中的稳定性和自然性问题,我们提出了FlexSpeech,一个稳定、可控、表现力强的TTS模型。FlexSpeech的动机是在持续时间预测器上直接融入马尔可夫依赖和偏好优化,以提高其自然性的同时,通过明确建模语音单元来确保稳定性。具体来说,我们将语音生成任务分解为两个组件:AR持续时间预测器和NAR声学模型。声学模型在大量数据上进行训练,学习在给定参考音频韵律和电话持续时间的情况下更稳定地呈现音频。持续时间预测器以轻巧的方式进行优化,以适应不同的风格变化,从而在保持与指定说话人音色解耦的同时实现快速风格转换。实验结果表明,我们的方法在实现零射击TTS的稳定性和自然性方面达到了最新水平。更重要的是,转移到特定风格领域时,我们仅通过约100个数据样本对持续时间模块进行轻量化优化,无需调整声学模型,从而实现快速稳定的风格转换。
关键见解
- 当前语音生成研究分为非自回归和自回归两大类,二者在持续时间预测策略上有所不同。
- NAR方法通过明确建模语音单元的持续时间确保语音生成的稳定性。
- AR方法采用自回归范式预测压缩语音标记,但可能缺乏结构稳定性。
- FlexSpeech模型结合AR和NAR方法的优点,旨在实现稳定、自然且可控的语音生成。
- FlexSpeech通过将语音生成任务分解为两个组件(AR持续时间预测器和NAR声学模型)来提高性能。
- 实验中,FlexSpeech在零射击TTS中表现出卓越的稳定性和自然性。
点此查看论文截图
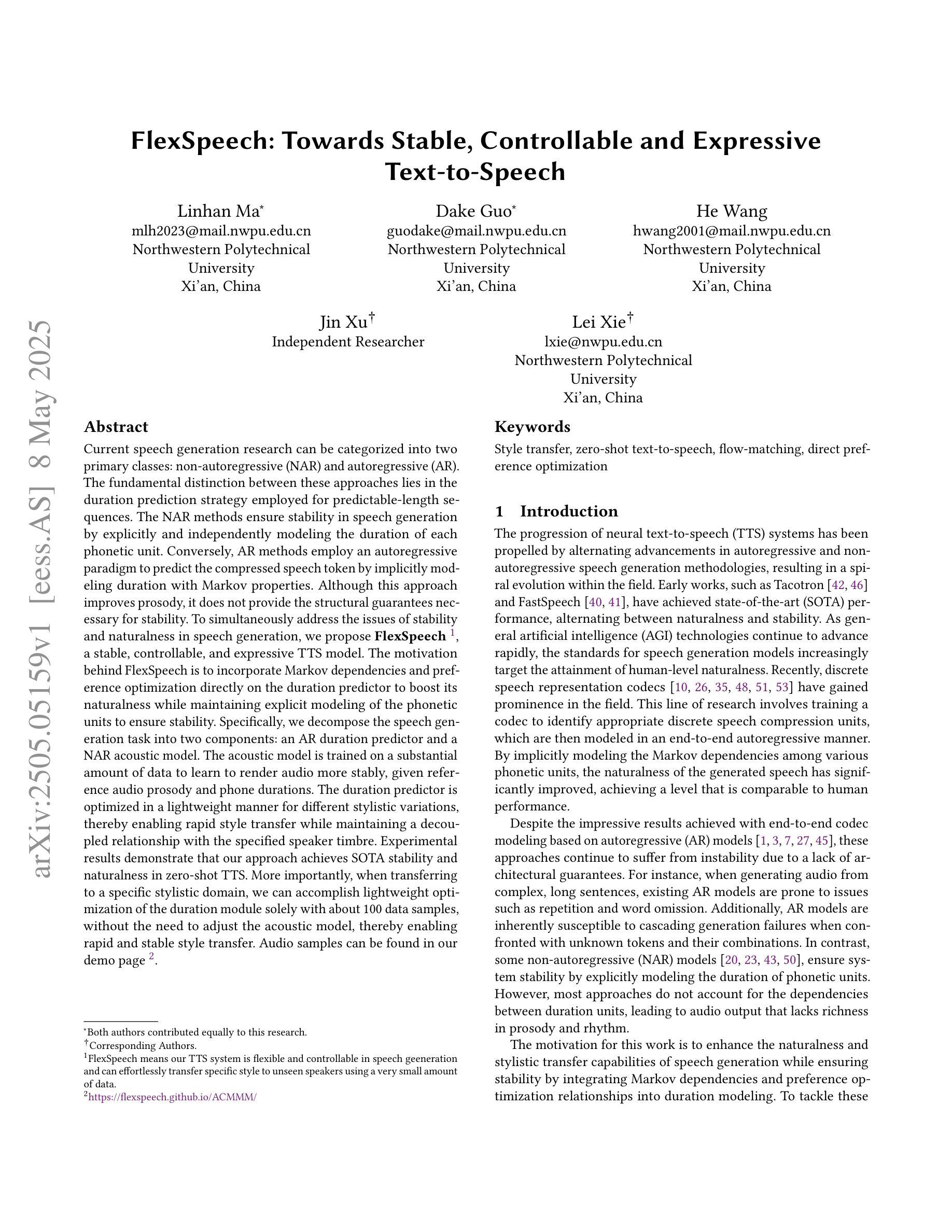
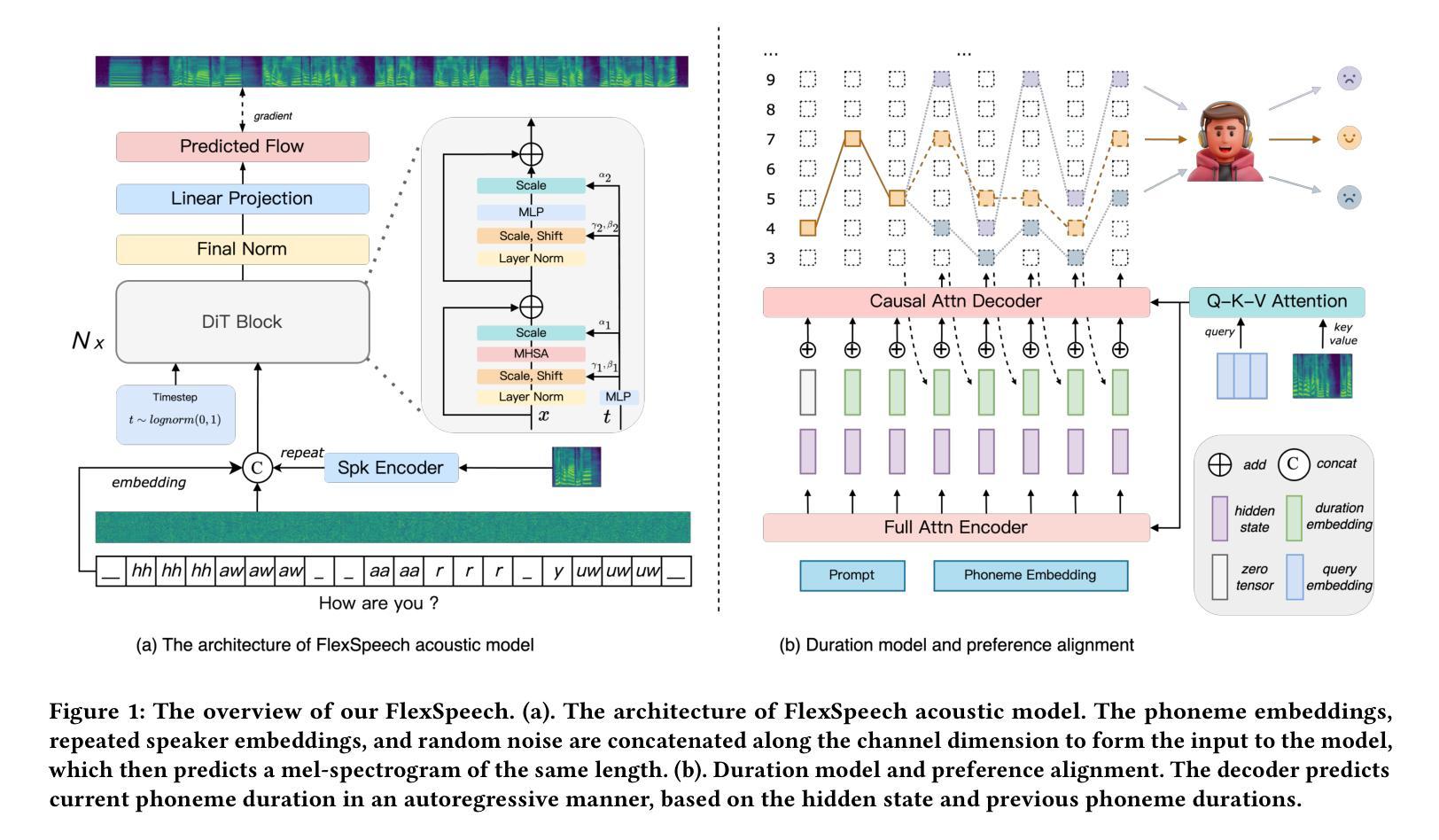
Teochew-Wild: The First In-the-wild Teochew Dataset with Orthographic Annotations
Authors:Linrong Pan, Chenglong Jiang, Gaoze Hou, Ying Gao
This paper reports the construction of the Teochew-Wild, a speech corpus of the Teochew dialect. The corpus includes 18.9 hours of in-the-wild Teochew speech data from multiple speakers, covering both formal and colloquial expressions, with precise orthographic and pinyin annotations. Additionally, we provide supplementary text processing tools and resources to propel research and applications in speech tasks for this low-resource language, such as automatic speech recognition (ASR) and text-to-speech (TTS). To the best of our knowledge, this is the first publicly available Teochew dataset with accurate orthographic annotations. We conduct experiments on the corpus, and the results validate its effectiveness in ASR and TTS tasks.
本文报道了潮汕方言语音语料库“Teochew-Wild”的构建情况。该语料库包含来自多名发音人的18.9小时自然状态下的潮汕方言语音数据,涵盖正式和口语表达,带有精确的汉字和拼音标注。此外,我们还提供了补充的文本处理工具和资源,以推动针对这种低资源语言的语音任务(如自动语音识别(ASR)和文本到语音(TTS))的研究和应用。据我们所知,这是第一个带有精确汉字标注的公开可用的潮汕数据集。我们在语料库上进行了实验,结果表明其在ASR和TTS任务中的有效性。
论文及项目相关链接
Summary
本文构建了一个潮汕方言的语音语料库——Teochew-Wild。该语料库包含了来自多名演讲者的18.9小时的野外潮汕方言语音数据,涵盖正式和口语表达,带有精确的汉字和拼音注释。还提供辅助文本处理工具和资源,推动低资源语言的语音任务研究与应用,如自动语音识别(ASR)和文本到语音(TTS)。据我们所知,这是第一个带有精确汉字注释的公开潮汕数据集。实验结果验证了其在ASR和TTS任务中的有效性。
Key Takeaways
- Teochew-Wild是首个公开可用的带有精确汉字注释的潮汕方言语音语料库。
- 该语料库包含18.9小时的野外潮汕方言语音数据,涵盖正式和口语表达。
- 提供了包括自动语音识别(ASR)和文本到语音(TTS)在内的多种语音任务的研究资源。
- 通过对语料库的实证研究,验证了其在ASR和TTS任务中的有效性。
- 该语料库的建设有助于推动潮汕方言的研究与应用。
- 提供了辅助文本处理工具,便于研究者使用潮汕方言语音数据集进行更深入的分析和研究。
点此查看论文截图
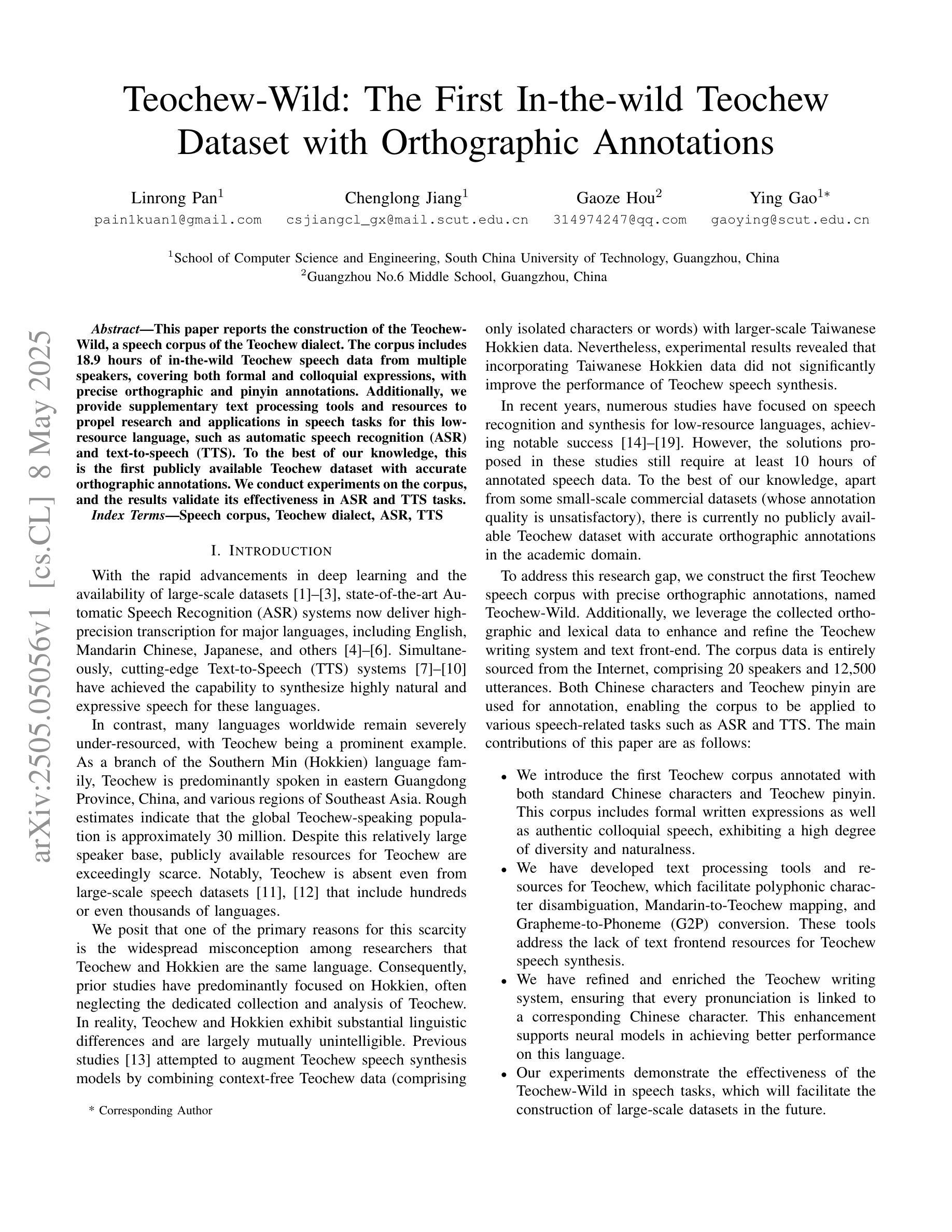
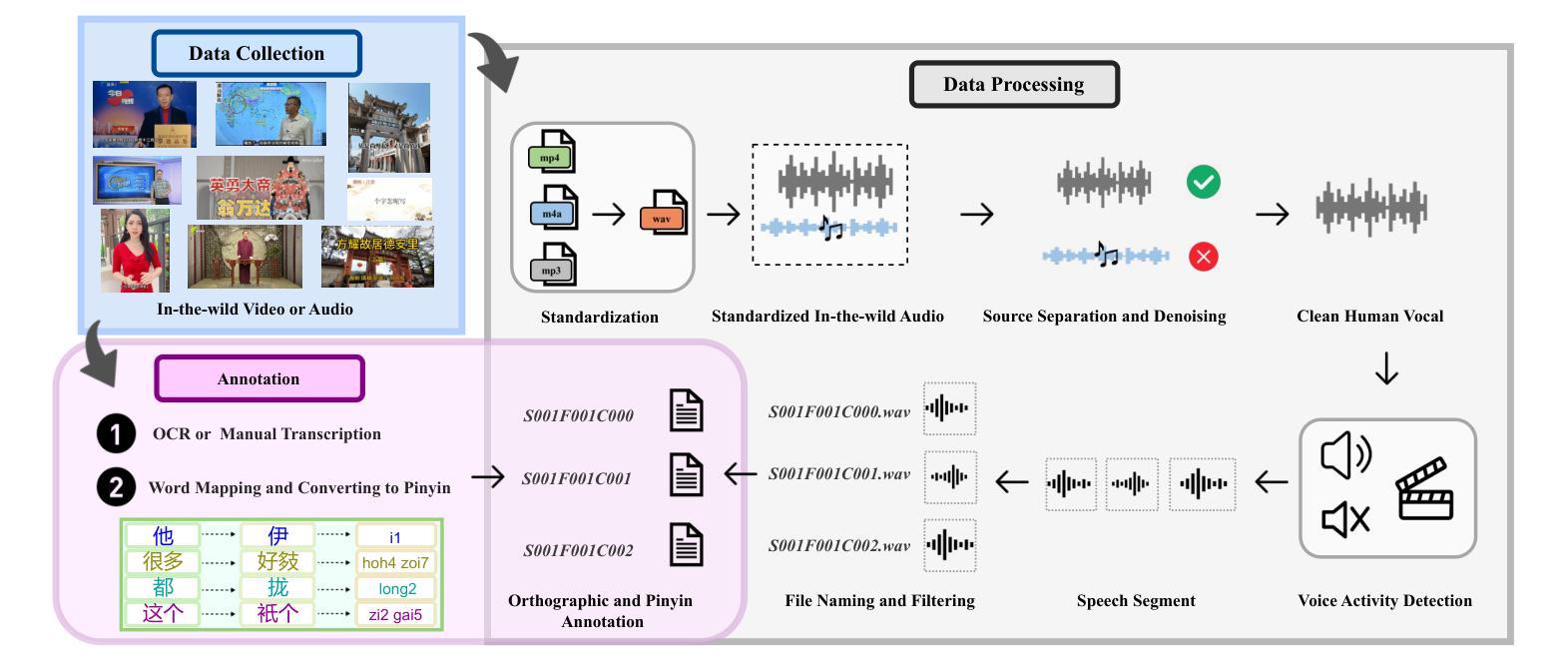
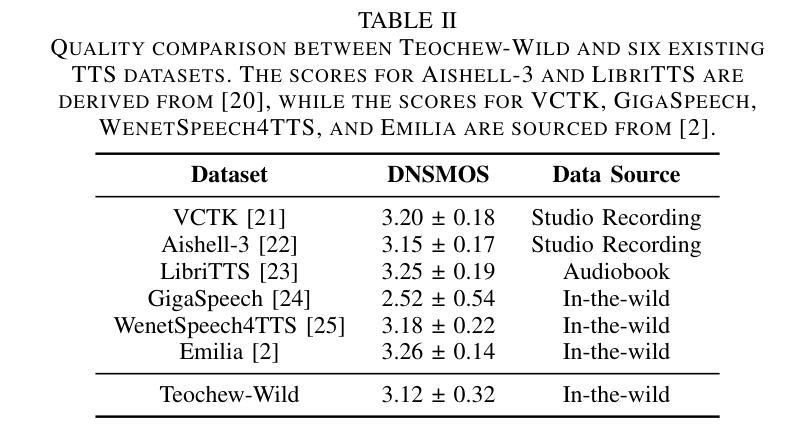
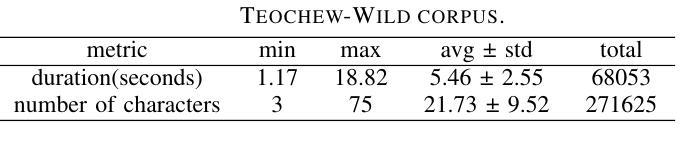
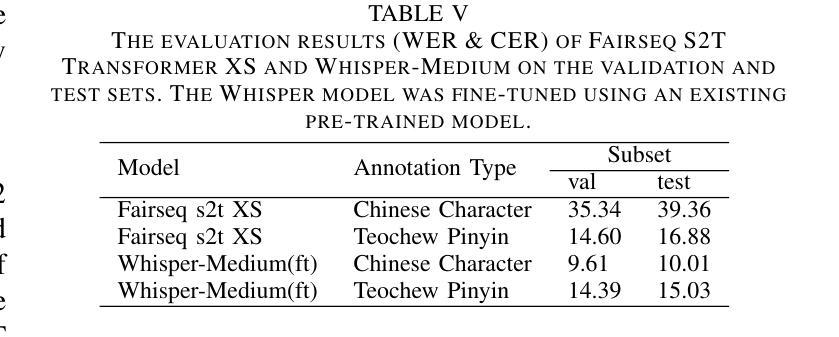
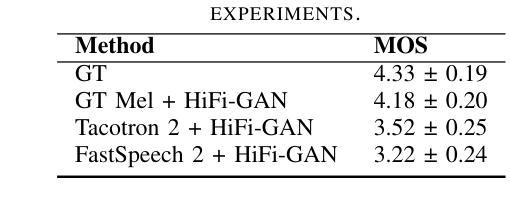