⚠️ 以下所有内容总结都来自于 大语言模型的能力,如有错误,仅供参考,谨慎使用
🔴 请注意:千万不要用于严肃的学术场景,只能用于论文阅读前的初筛!
💗 如果您觉得我们的项目对您有帮助 ChatPaperFree ,还请您给我们一些鼓励!⭐️ HuggingFace免费体验
2025-05-14 更新
My Emotion on your face: The use of Facial Keypoint Detection to preserve Emotions in Latent Space Editing
Authors:Jingrui He, Andrew Stephen McGough
Generative Adversarial Network approaches such as StyleGAN/2 provide two key benefits: the ability to generate photo-realistic face images and possessing a semantically structured latent space from which these images are created. Many approaches have emerged for editing images derived from vectors in the latent space of a pre-trained StyleGAN/2 models by identifying semantically meaningful directions (e.g., gender or age) in the latent space. By moving the vector in a specific direction, the ideal result would only change the target feature while preserving all the other features. Providing an ideal data augmentation approach for gesture research as it could be used to generate numerous image variations whilst keeping the facial expressions intact. However, entanglement issues, where changing one feature inevitably affects other features, impacts the ability to preserve facial expressions. To address this, we propose the use of an addition to the loss function of a Facial Keypoint Detection model to restrict changes to the facial expressions. Building on top of an existing model, adding the proposed Human Face Landmark Detection (HFLD) loss, provided by a pre-trained Facial Keypoint Detection model, to the original loss function. We quantitatively and qualitatively evaluate the existing and our extended model, showing the effectiveness of our approach in addressing the entanglement issue and maintaining the facial expression. Our approach achieves up to 49% reduction in the change of emotion in our experiments. Moreover, we show the benefit of our approach by comparing with state-of-the-art models. By increasing the ability to preserve the facial gesture and expression during facial transformation, we present a way to create human face images with fixed expression but different appearances, making it a reliable data augmentation approach for Facial Gesture and Expression research.
生成对抗网络(GAN)方法,如StyleGAN/2,提供了两大关键优势:能够生成逼真的面部图像,以及拥有一个语义结构化的潜在空间,这些图像正是从这个空间中生成的。许多方法已经涌现,通过识别潜在空间中语义上有意义的方向(例如性别或年龄),来编辑来自预训练StyleGAN/2模型的向量。通过将向量向特定方向移动,理想的结果只会改变目标特征,同时保留其他所有特征。它为手势研究提供了一种理想的数据增强方法,因为可以用于生成多种图像变体,同时保持面部表情不变。然而,纠缠问题(改变一个特征不可避免地会影响其他特征)影响了保留面部表情的能力。为了解决这一问题,我们建议在面部关键点检测模型的损失函数中添加一项来限制面部表情的变化。我们基于一个现有模型,将预先训练的面部关键点检测模型提供的人脸地标检测(HFLD)损失添加到原始损失函数中。我们对现有模型和我们的扩展模型进行了定量和定性的评估,结果显示我们的方法在解决纠缠问题并保持面部表情方面非常有效。在我们的实验中,我们的方法实现了情感变化减少高达49%。此外,通过与最新模型的比较,我们展示了我们的方法的好处。通过提高在面部变换过程中保留面部姿态和表情的能力,我们展示了一种创建具有固定表情但外观不同的面部图像的方法,使其成为面部姿态和表情研究的一种可靠的数据增强方法。
论文及项目相关链接
PDF Submitted to 2nd International Workshop on Synthetic Data for Face and Gesture Analysis at IEEE FG 2025
Summary
基于StyleGAN/2的生成对抗网络方法具有两大优势:能够生成逼真的人脸图像,并拥有一个创建这些图像时使用的语义结构化潜在空间。本文主要探讨了如何在预训练的StyleGAN/2模型的潜在空间中编辑图像向量,通过识别潜在空间中的语义方向(如性别或年龄)来改变图像。同时,为解决变换时面部特征纠缠问题,本文提出在面部关键点检测模型的损失函数中添加人类面部地标检测(HFLD)损失来限制面部表情的变化。通过定量和定性评估,本文方法能有效解决纠缠问题并维持面部表情,减少了高达49%的情感变化。此外,本文展示了该方法相较于其他先进模型的优势,提供了一种在面部变换中保持固定表情和外观的方法,使其成为面部姿态和表情研究的可靠数据增强方法。
Key Takeaways
- StyleGAN/2可生成逼真的人脸图像并具有语义结构化潜在空间。
- 通过编辑潜在空间中的图像向量,可改变图像的目标特征而其他特征保持不变。
- 面部特征纠缠问题会影响表情的保持,提出使用面部关键点检测模型的损失函数添加HFLD损失来解决。
- 方法有效解决面部特征纠缠问题,并减少情感变化达49%。
- 与其他先进模型相比,该方法在维持面部表情方面具有优势。
- 本文提供了一种在面部变换中保持固定表情和外观的方法。
点此查看论文截图
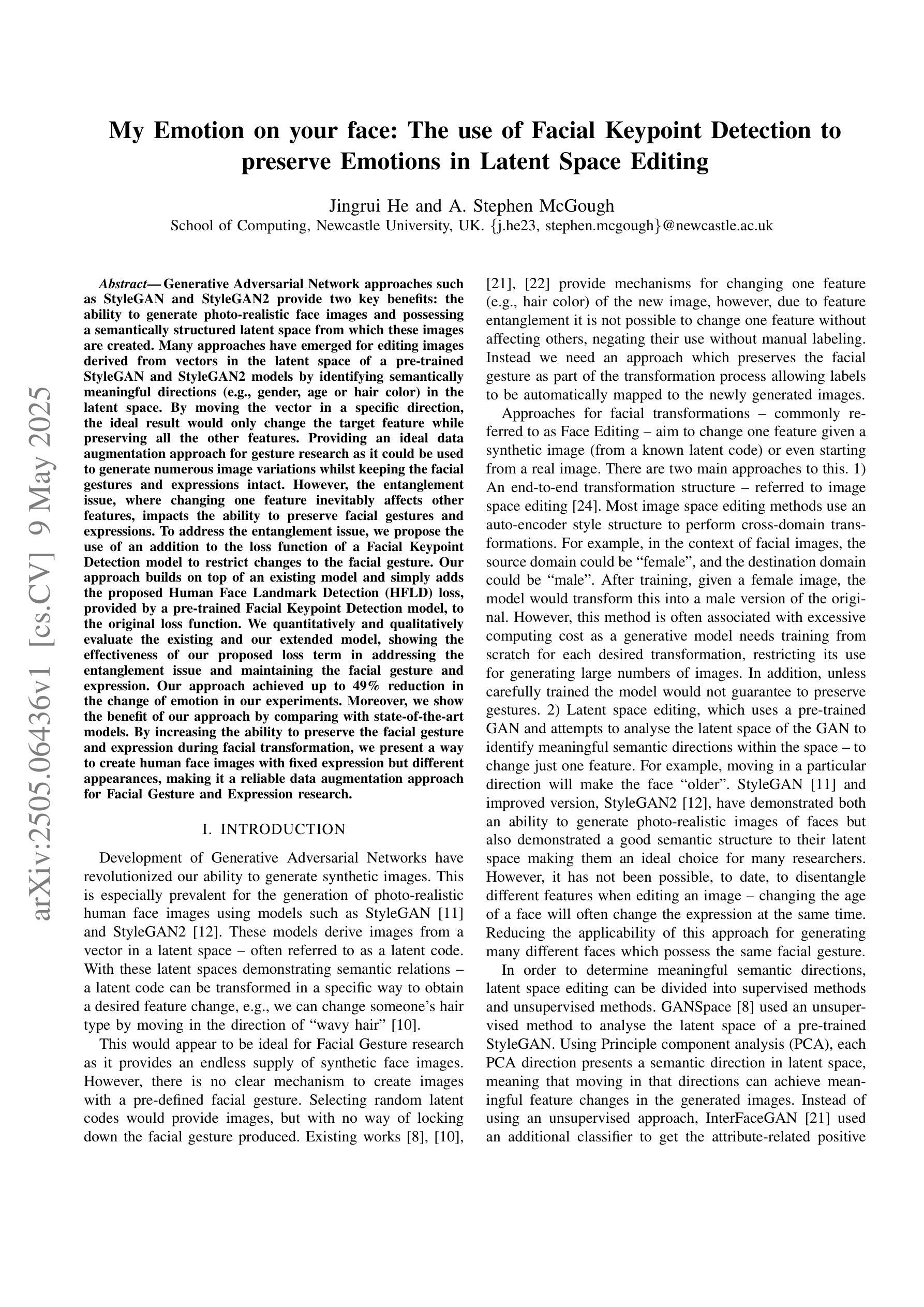
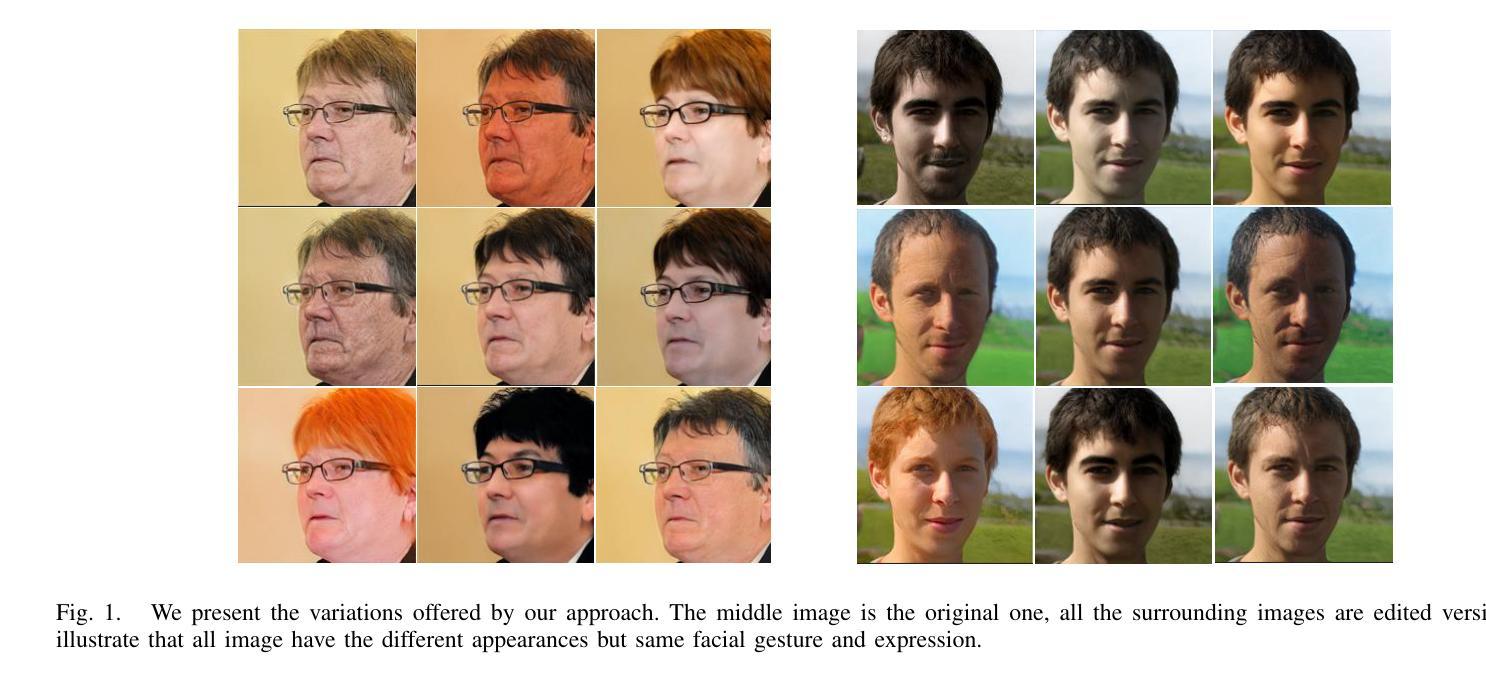
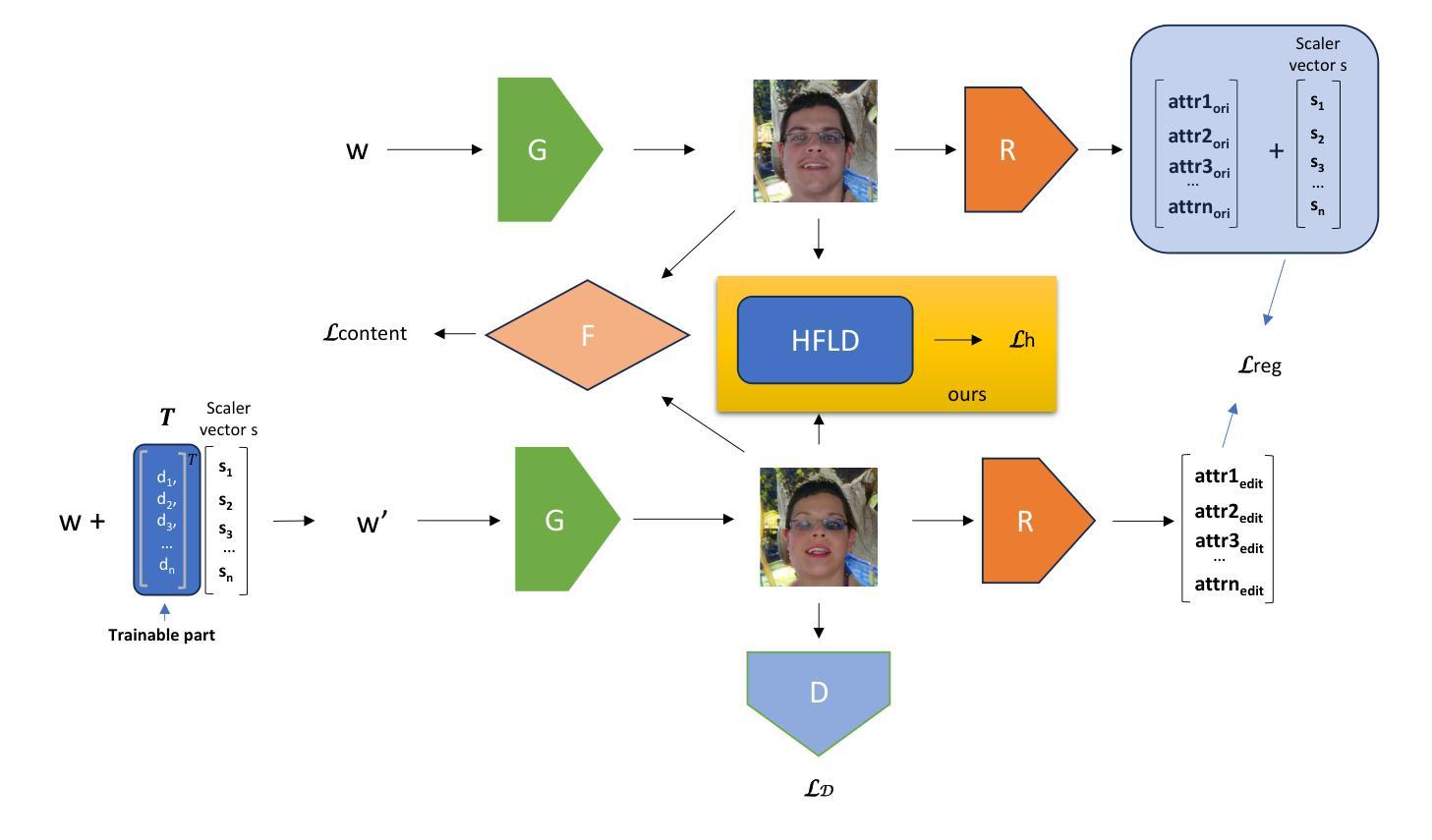
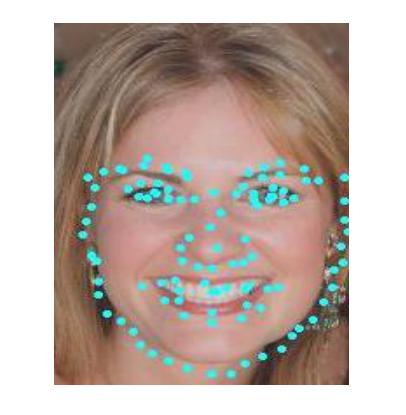
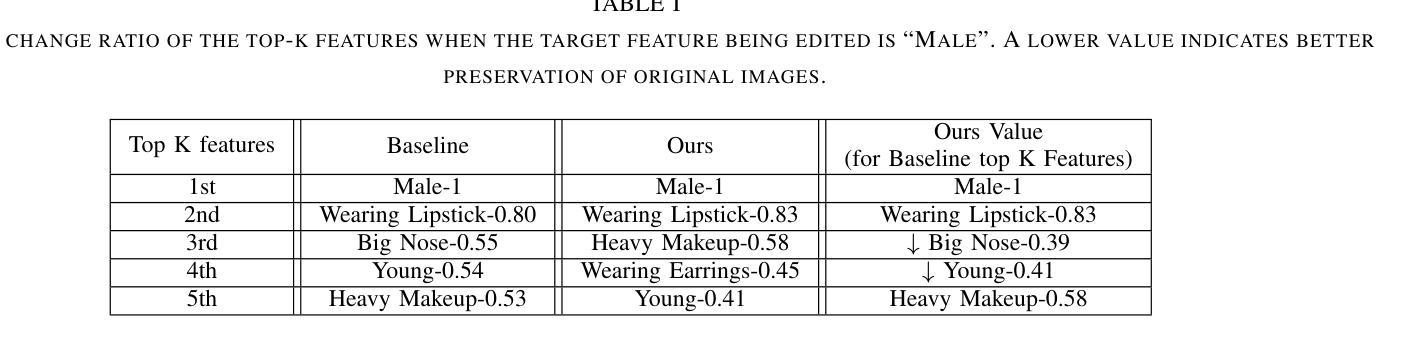
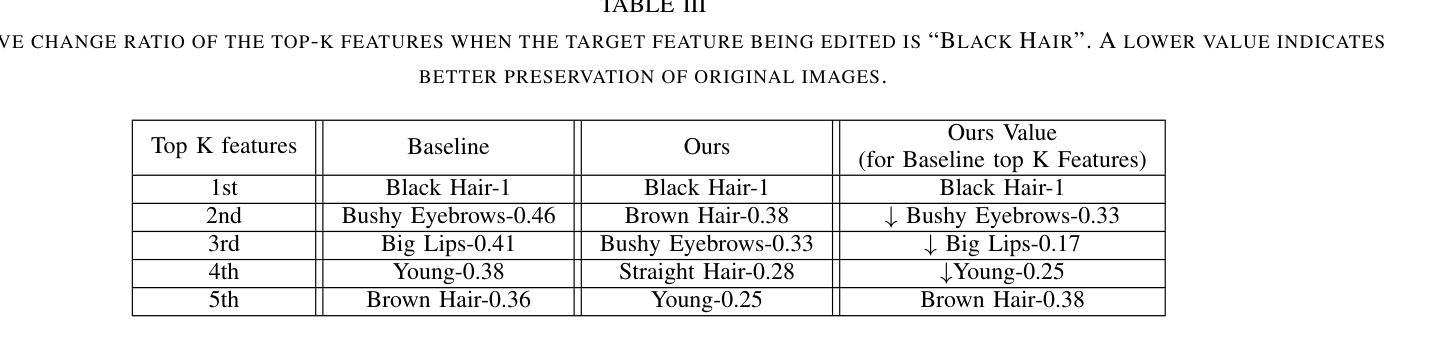
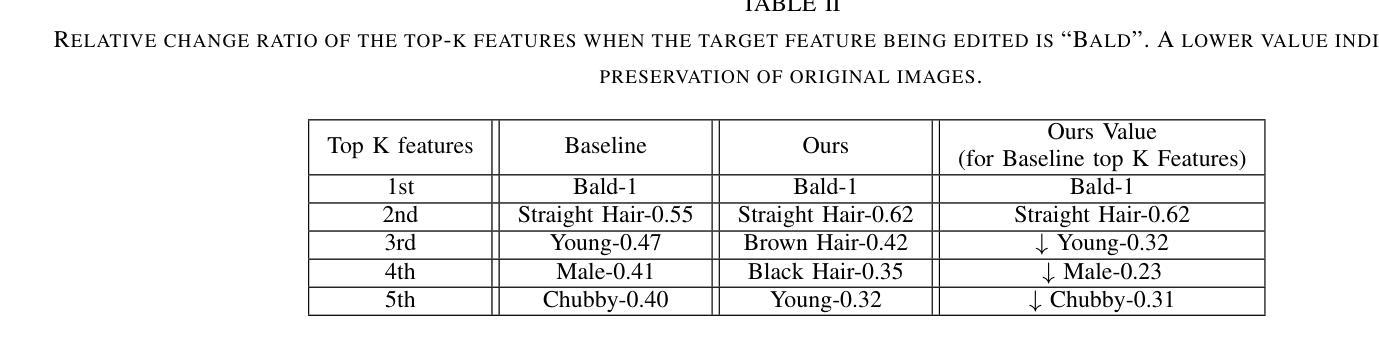
