⚠️ 以下所有内容总结都来自于 大语言模型的能力,如有错误,仅供参考,谨慎使用
🔴 请注意:千万不要用于严肃的学术场景,只能用于论文阅读前的初筛!
💗 如果您觉得我们的项目对您有帮助 ChatPaperFree ,还请您给我们一些鼓励!⭐️ HuggingFace免费体验
2025-05-14 更新
MAGE:A Multi-stage Avatar Generator with Sparse Observations
Authors:Fangyu Du, Yang Yang, Xuehao Gao, Hongye Hou
Inferring full-body poses from Head Mounted Devices, which capture only 3-joint observations from the head and wrists, is a challenging task with wide AR/VR applications. Previous attempts focus on learning one-stage motion mapping and thus suffer from an over-large inference space for unobserved body joint motions. This often leads to unsatisfactory lower-body predictions and poor temporal consistency, resulting in unrealistic or incoherent motion sequences. To address this, we propose a powerful Multi-stage Avatar GEnerator named MAGE that factorizes this one-stage direct motion mapping learning with a progressive prediction strategy. Specifically, given initial 3-joint motions, MAGE gradually inferring multi-scale body part poses at different abstract granularity levels, starting from a 6-part body representation and gradually refining to 22 joints. With decreasing abstract levels step by step, MAGE introduces more motion context priors from former prediction stages and thus improves realistic motion completion with richer constraint conditions and less ambiguity. Extensive experiments on large-scale datasets verify that MAGE significantly outperforms state-of-the-art methods with better accuracy and continuity.
从头戴式设备推断全身姿势是一项具有广泛AR/VR应用挑战性的任务,这些设备仅捕获头部和手腕的3关节观察结果。以前的尝试主要集中在学习一次性运动映射上,因此遭受未观察到的身体关节运动的过大推断空间的困扰。这经常导致下半身预测不理想和时间上的连贯性不佳,从而产生不真实或不一致的运动序列。为了解决这一问题,我们提出了一种强大的多阶段化身生成器,名为MAGE,它通过渐进预测策略分解了一次性直接运动映射学习。具体来说,给定最初的3关节运动,MAGE从6部分身体表示开始,逐步推断不同抽象粒度级别的多尺度身体部位姿势,并逐渐细化到22个关节。通过逐步降低抽象级别,MAGE引入了来自前一个预测阶段的更多运动上下文先验信息,从而利用更丰富的约束条件和较少的模糊性提高了现实运动的完成度。大规模数据集上的大量实验证实,MAGE在准确性和连续性方面显著优于最新方法。
论文及项目相关链接
Summary
基于头戴式设备仅捕捉头部和手腕的3关节观测数据进行全身姿态推断是一项充满挑战的任务,在AR/VR领域具有广泛应用前景。此前的方法大多侧重于学习一次性动作映射,因此面临未观测到的身体关节动作推断空间过大的问题,常常导致下半身预测不准确,时间连续性差,产生不真实或不连贯的动作序列。为解决这一问题,我们提出了一种强大的多阶段化身生成器(Multi-stage Avatar GEnerator,简称MAGE)。它采用逐步预测策略,将一次性直接动作映射学习分解为多个阶段。具体来说,给定初始的3关节动作,MAGE逐步推断不同抽象粒度级别的多部分身体姿态,从6部分身体表示开始,逐步精细到22个关节。通过逐步降低抽象层次,MAGE引入了更多来自先前预测阶段的动作上下文先验知识,从而提高了具有更丰富约束条件和较少模糊性的真实动作完成能力。大规模数据集上的实验表明,MAGE在准确性和连续性方面显著优于最先进的方法。
Key Takeaways
- 仅通过头戴设备观测的头部和手腕3关节数据推断全身姿态具有挑战性。
- 此前的方法因一次性动作映射学习面临大推断空间问题。
- MAGE采用多阶段逐步预测策略来解决这个问题。
- MAGE从抽象的6部分身体表示开始,逐步精细到具体的22个关节。
- MAGE通过引入更多先前预测阶段的动作上下文先验知识来提高预测的真实性和连续性。
- 实验证明,在大型数据集上,MAGE在准确性和连续性方面优于其他方法。
点此查看论文截图
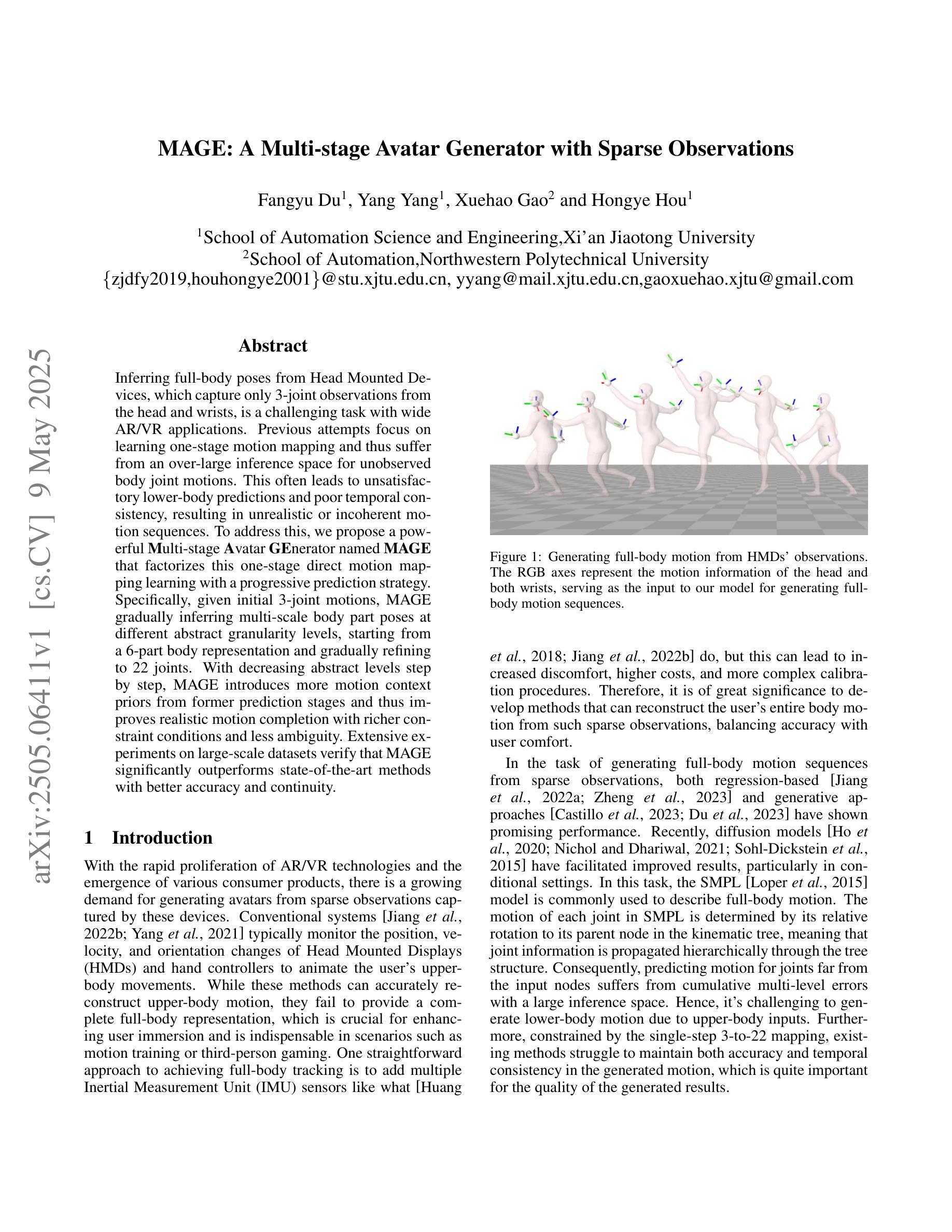
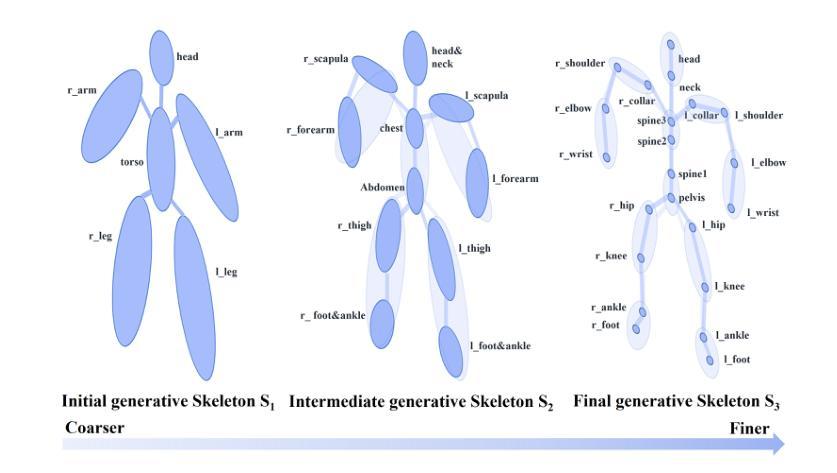
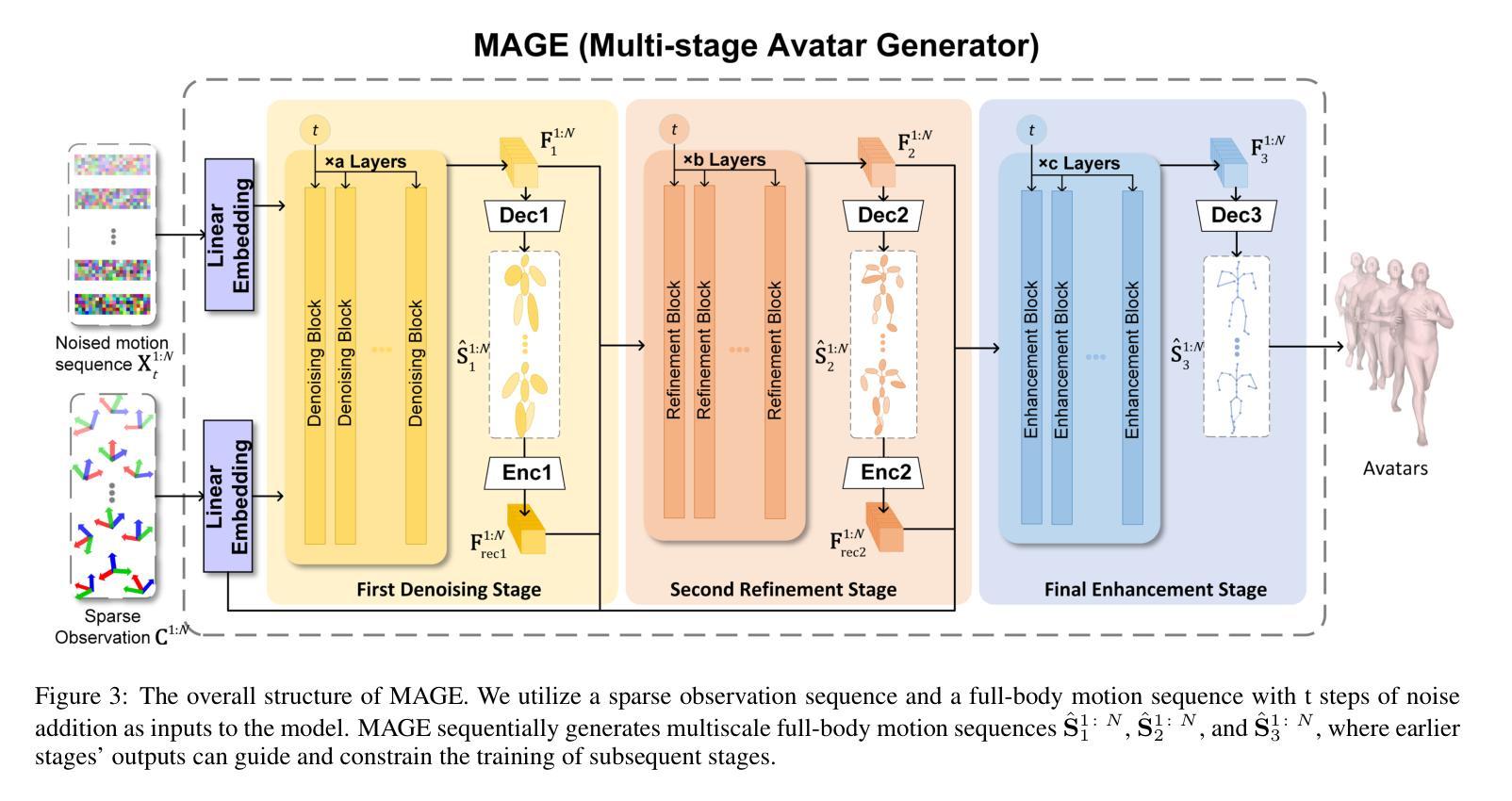
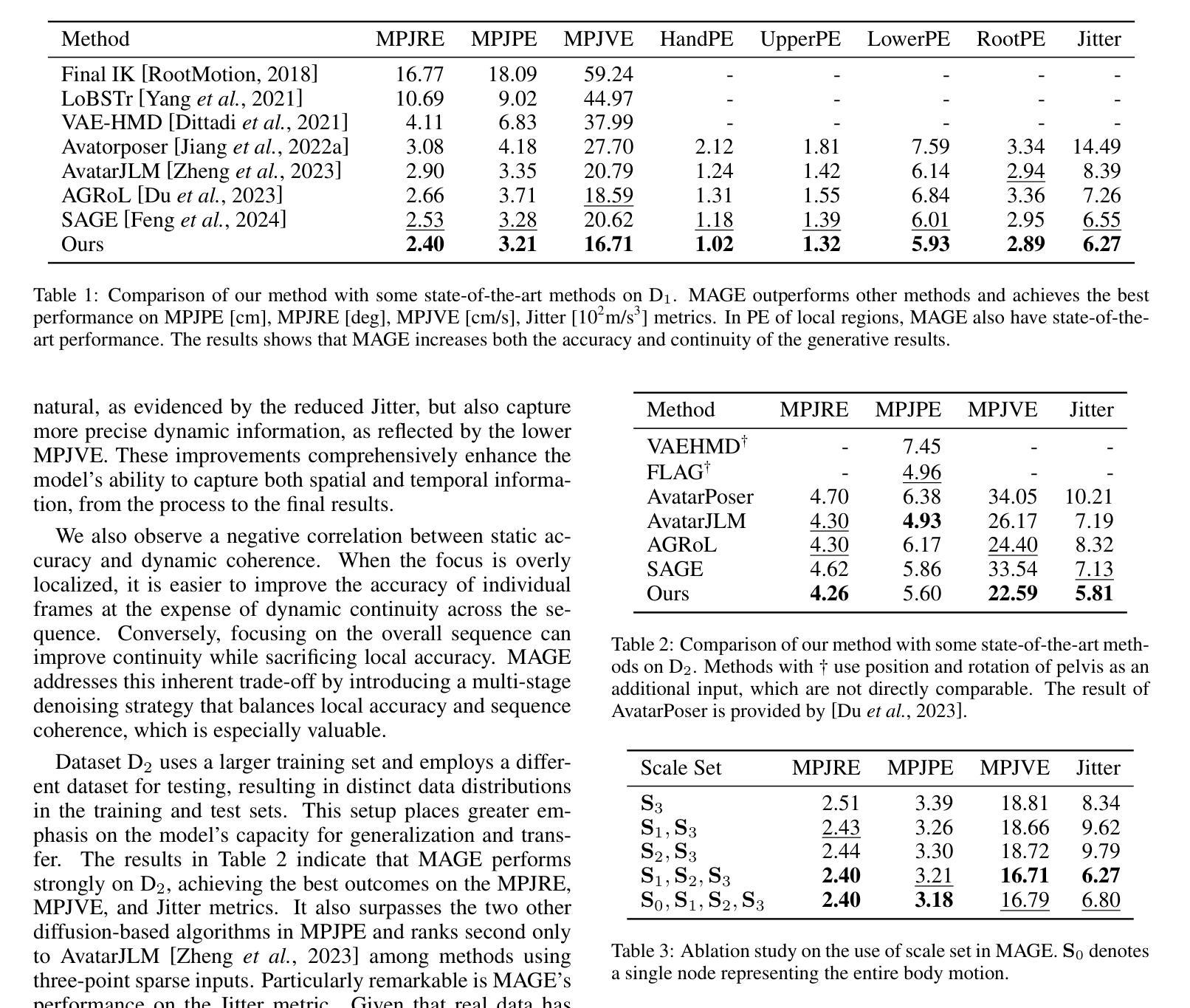
TeGA: Texture Space Gaussian Avatars for High-Resolution Dynamic Head Modeling
Authors:Gengyan Li, Paulo Gotardo, Timo Bolkart, Stephan Garbin, Kripasindhu Sarkar, Abhimitra Meka, Alexandros Lattas, Thabo Beeler
Sparse volumetric reconstruction and rendering via 3D Gaussian splatting have recently enabled animatable 3D head avatars that are rendered under arbitrary viewpoints with impressive photorealism. Today, such photoreal avatars are seen as a key component in emerging applications in telepresence, extended reality, and entertainment. Building a photoreal avatar requires estimating the complex non-rigid motion of different facial components as seen in input video images; due to inaccurate motion estimation, animatable models typically present a loss of fidelity and detail when compared to their non-animatable counterparts, built from an individual facial expression. Also, recent state-of-the-art models are often affected by memory limitations that reduce the number of 3D Gaussians used for modeling, leading to lower detail and quality. To address these problems, we present a new high-detail 3D head avatar model that improves upon the state of the art, largely increasing the number of 3D Gaussians and modeling quality for rendering at 4K resolution. Our high-quality model is reconstructed from multiview input video and builds on top of a mesh-based 3D morphable model, which provides a coarse deformation layer for the head. Photoreal appearance is modelled by 3D Gaussians embedded within the continuous UVD tangent space of this mesh, allowing for more effective densification where most needed. Additionally, these Gaussians are warped by a novel UVD deformation field to capture subtle, localized motion. Our key contribution is the novel deformable Gaussian encoding and overall fitting procedure that allows our head model to preserve appearance detail, while capturing facial motion and other transient high-frequency features such as skin wrinkling.
通过三维高斯平铺实现的稀疏体积重建和渲染,最近已经能够创建出可以在任意视角呈现生动三维头像的动画角色,具有令人印象深刻的逼真度。如今,这种逼真的头像被视为远程存在、扩展现实和娱乐等新兴应用中的关键组成部分。构建逼真的头像需要估计输入视频图像中不同面部组件的复杂非刚性运动;由于运动估计不准确,与基于单个面部表情的非动画模型相比,动画模型通常在保真度和细节上有所损失。此外,最近最先进的模型通常受到内存限制的影响,减少了用于建模的三维高斯数量,导致细节和质量降低。为了解决这些问题,我们提出了一种新的高细节三维头像模型,该模型超越了当前的技术水平,大大提高了用于4K分辨率渲染的三维高斯数量和建模质量。我们的高质量模型是从多角度输入视频重建的,建立在基于网格的三维可变形模型之上,该模型为头部提供了一个粗糙的变形层。通过嵌入此网格的连续UVD切线空间中的三维高斯来模拟逼真的外观,允许在最需要的地方进行更有效的密集化。此外,这些高斯被新的UVD变形场扭曲,以捕捉细微、局部的运动。我们的主要贡献是新型的可变形高斯编码和整体拟合程序,它允许我们的头部模型在捕捉面部运动和其他短暂的高频特征(如皮肤皱纹)的同时保留外观细节。
论文及项目相关链接
PDF 10 pages, 9 figures, supplementary results found at: https://syntec-research.github.io/UVGA/, to be published in SIGGRAPH 2025
Summary
本文介绍了通过三维高斯喷射技术创建动态三维头像的方法。该方法使用多视角输入视频进行高质量模型重建,采用基于网格的三维可变形模型提供头部粗糙变形层。通过嵌入连续UVD切线空间的3D高斯,模拟真实感外观,并通过新型UVD变形场对高斯进行扭曲,捕捉细微的局部运动。本文的关键贡献是创新的可变形高斯编码和整体拟合程序,允许头部模型在捕捉面部运动和其他瞬时高频特征(如皮肤皱纹)的同时保持外观细节。
Key Takeaways
- 3D Gaussian splatting技术用于创建可动画的三维头像。
- 多视角输入视频用于高质量模型重建。
- 基于网格的三维可变形模型提供头部粗糙变形层。
- 嵌入UVD切线空间的3D高斯模拟真实感外观。
- UVD变形场用于捕捉细微的局部运动。
- 创新的可变形高斯编码和整体拟合程序是本文的关键贡献。
点此查看论文截图
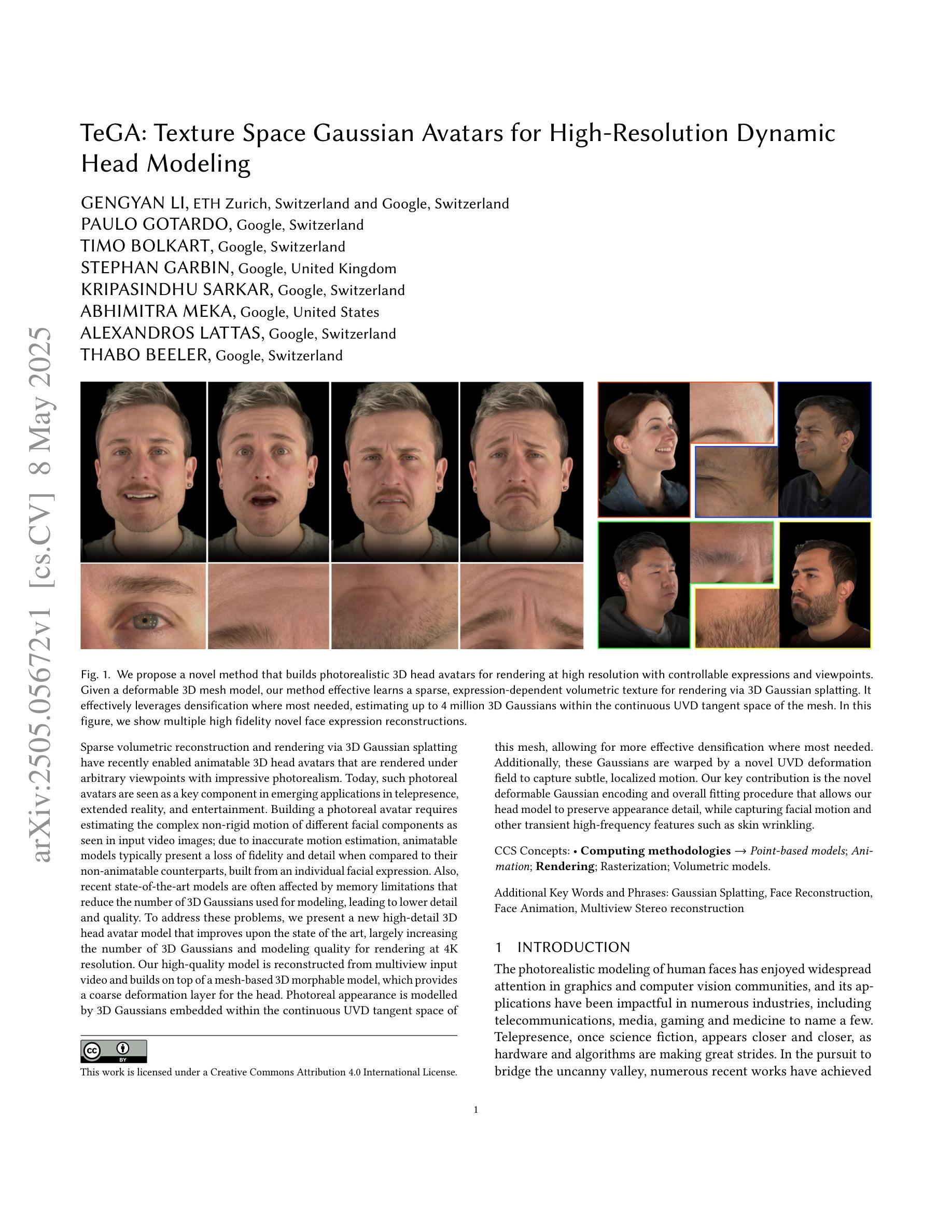
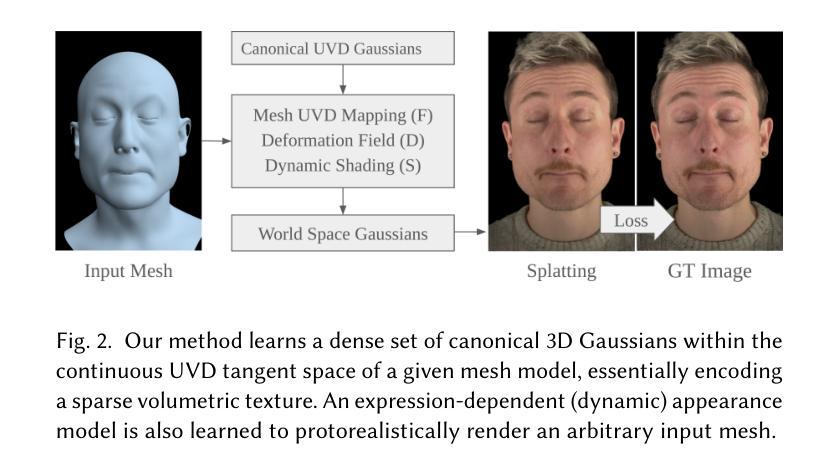
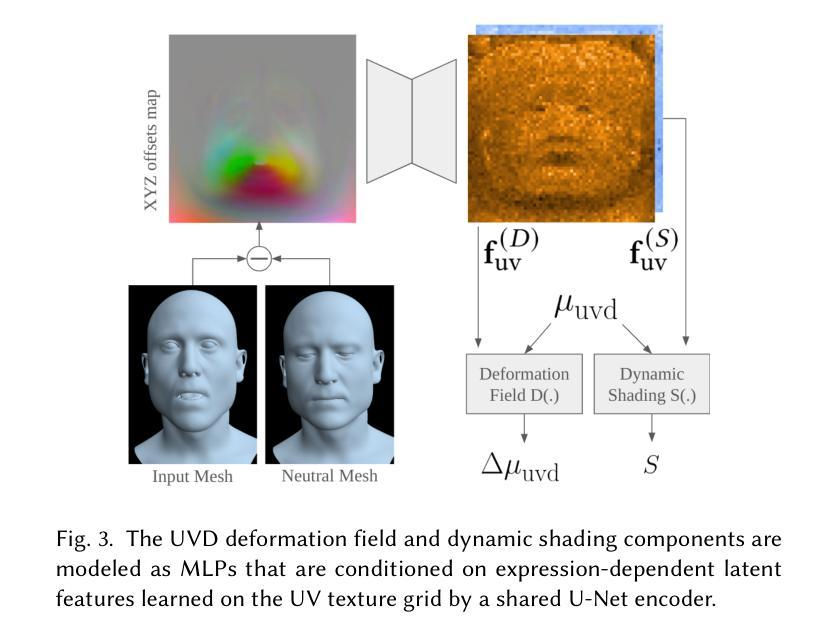