⚠️ 以下所有内容总结都来自于 大语言模型的能力,如有错误,仅供参考,谨慎使用
🔴 请注意:千万不要用于严肃的学术场景,只能用于论文阅读前的初筛!
💗 如果您觉得我们的项目对您有帮助 ChatPaperFree ,还请您给我们一些鼓励!⭐️ HuggingFace免费体验
2025-05-14 更新
DFEN: Dual Feature Equalization Network for Medical Image Segmentation
Authors:Jianjian Yin, Yi Chen, Chengyu Li, Zhichao Zheng, Yanhui Gu, Junsheng Zhou
Current methods for medical image segmentation primarily focus on extracting contextual feature information from the perspective of the whole image. While these methods have shown effective performance, none of them take into account the fact that pixels at the boundary and regions with a low number of class pixels capture more contextual feature information from other classes, leading to misclassification of pixels by unequal contextual feature information. In this paper, we propose a dual feature equalization network based on the hybrid architecture of Swin Transformer and Convolutional Neural Network, aiming to augment the pixel feature representations by image-level equalization feature information and class-level equalization feature information. Firstly, the image-level feature equalization module is designed to equalize the contextual information of pixels within the image. Secondly, we aggregate regions of the same class to equalize the pixel feature representations of the corresponding class by class-level feature equalization module. Finally, the pixel feature representations are enhanced by learning weights for image-level equalization feature information and class-level equalization feature information. In addition, Swin Transformer is utilized as both the encoder and decoder, thereby bolstering the ability of the model to capture long-range dependencies and spatial correlations. We conducted extensive experiments on Breast Ultrasound Images (BUSI), International Skin Imaging Collaboration (ISIC2017), Automated Cardiac Diagnosis Challenge (ACDC) and PH$^2$ datasets. The experimental results demonstrate that our method have achieved state-of-the-art performance. Our code is publicly available at https://github.com/JianJianYin/DFEN.
当前医学影像分割方法主要集中在从整个图像的角度提取上下文特征信息。虽然这些方法已经表现出有效的性能,但它们都没有考虑到边界处的像素以及具有少量类别像素的区域能够捕获来自其他类别的更多上下文特征信息,从而导致像素的误分类,因为不同类别的上下文特征信息是不平衡的。在本文中,我们提出了一种基于Swin Transformer和卷积神经网络混合架构的双特征均衡网络,旨在通过图像级均衡特征信息和类别级均衡特征信息来增强像素特征表示。首先,设计了图像级特征均衡模块,以均衡图像内像素的上下文信息。其次,我们通过类别级特征均衡模块聚合同一类别的区域,以均衡相应类别的像素特征表示。最后,通过图像级均衡特征信息和类别级均衡特征信息的权重学习来增强像素特征表示。此外,Swin Transformer同时作为编码器和解码器使用,从而增强了模型捕捉长期依赖性和空间关联性的能力。我们在乳腺超声图像(BUSI)、国际皮肤成像协作(ISIC2017)、自动心脏诊断挑战赛(ACDC)和PH数据集上进行了大量实验。实验结果表明,我们的方法达到了最先进的性能。我们的代码可在https://github.com/JianJianYin/DFEN公开访问。
论文及项目相关链接
Summary
本文提出了一种基于Swin Transformer和卷积神经网络混合架构的双特征均衡网络,用于增强像素特征表示。该网络通过图像级和类别级特征均衡模块设计,以均衡图像内像素的上下文信息和同类像素的特征表示。在乳腺超声图像等多个数据集上的实验结果表明,该方法达到了先进水平。
Key Takeaways
- 当前医疗图像分割方法主要关注从整个图像角度提取上下文特征信息。
- 像素边界和类别像素数量较少的区域能捕获更多来自其他类别的上下文特征信息。
- 提出的双特征均衡网络旨在通过图像级和类别级特征均衡模块增强像素特征表示。
- 图像级特征均衡模块设计用于均衡图像内像素的上下文信息。
- 类别级特征均衡模块通过聚合同类区域来均衡相应类别的像素特征表示。
- 该网络通过Swin Transformer同时作为编码器和解码器,提高了模型捕捉长期依赖关系和空间关联的能力。
点此查看论文截图
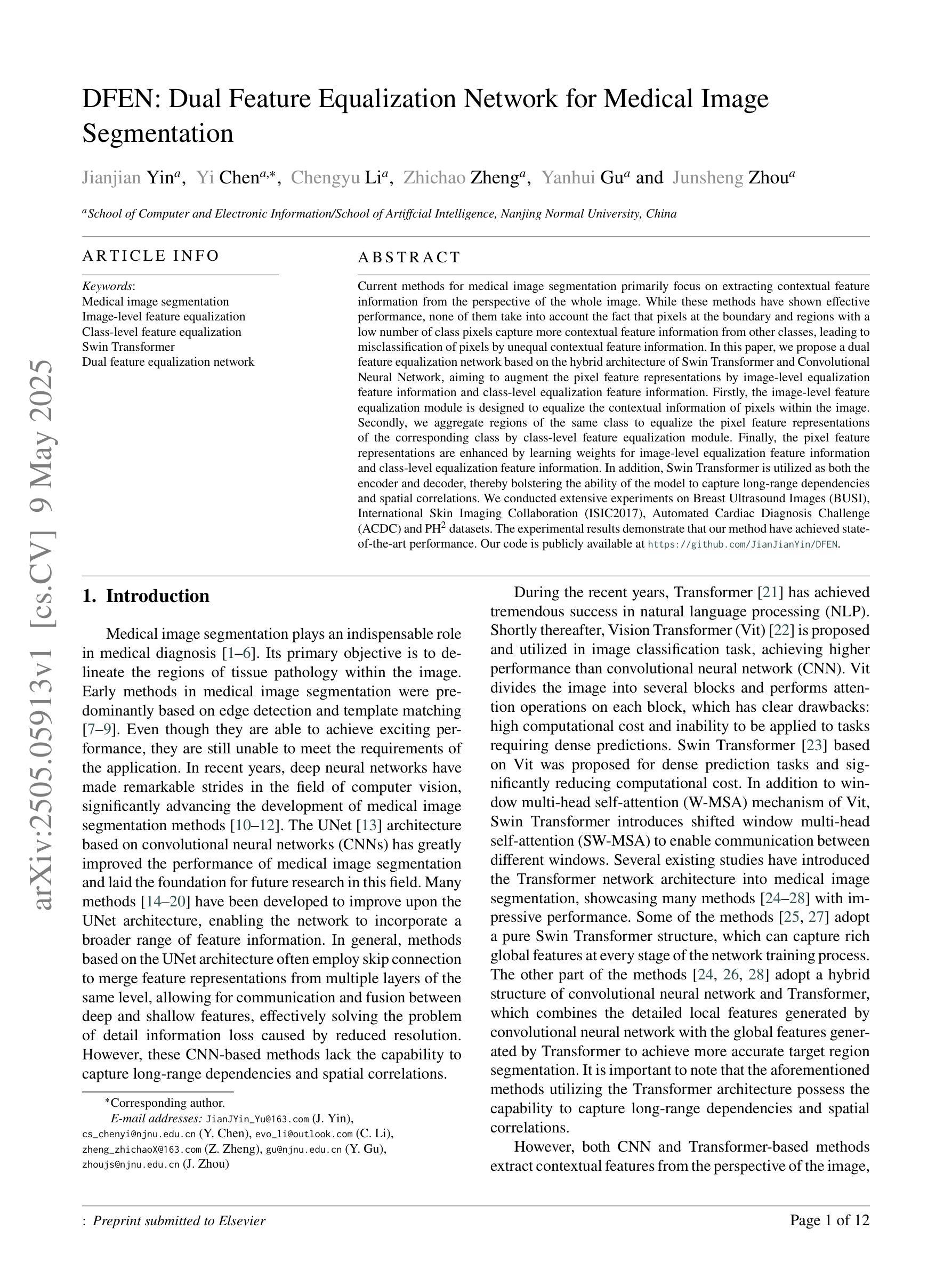
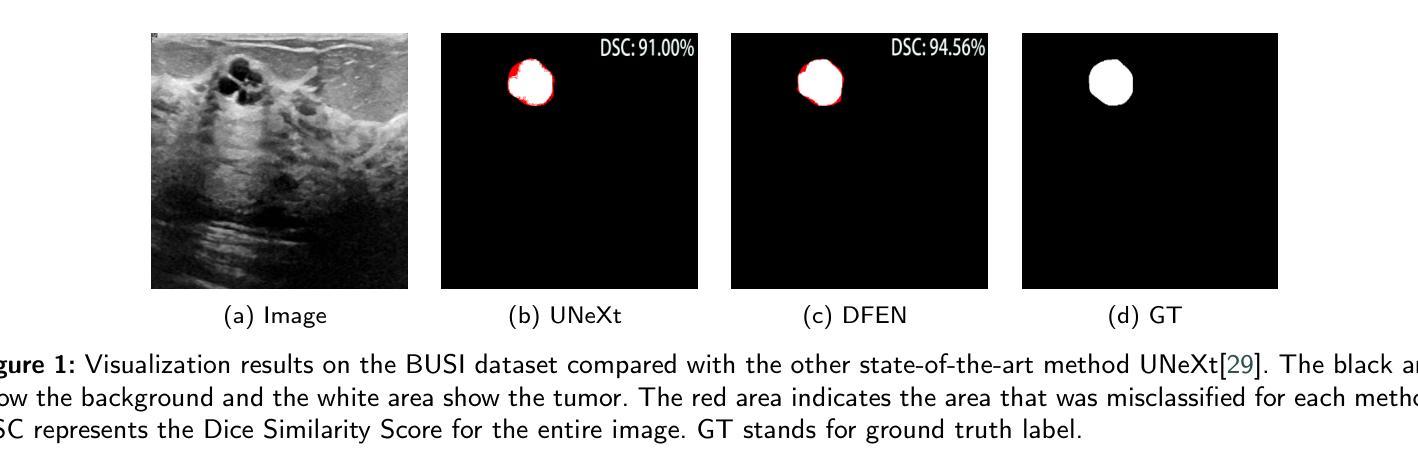
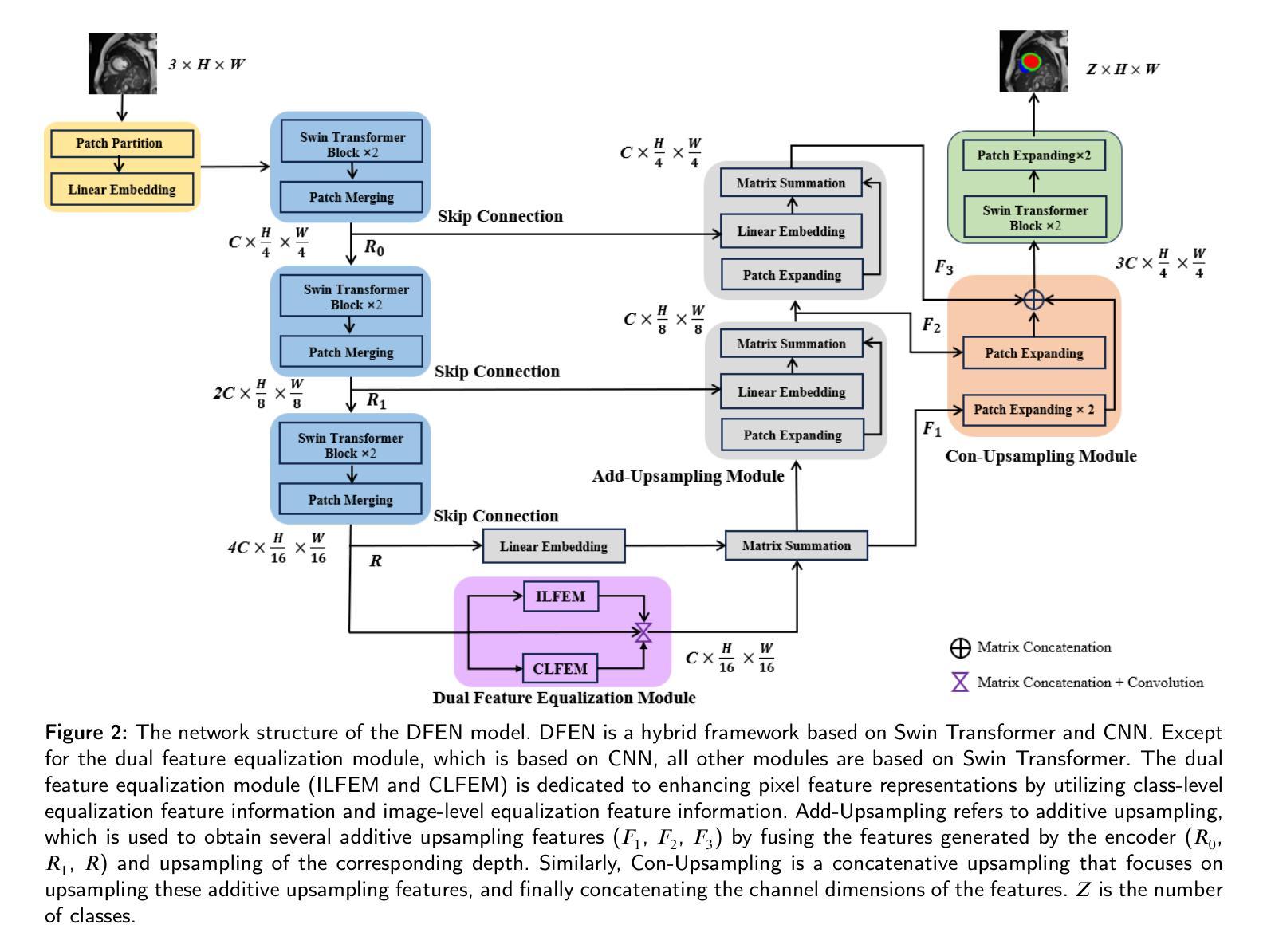
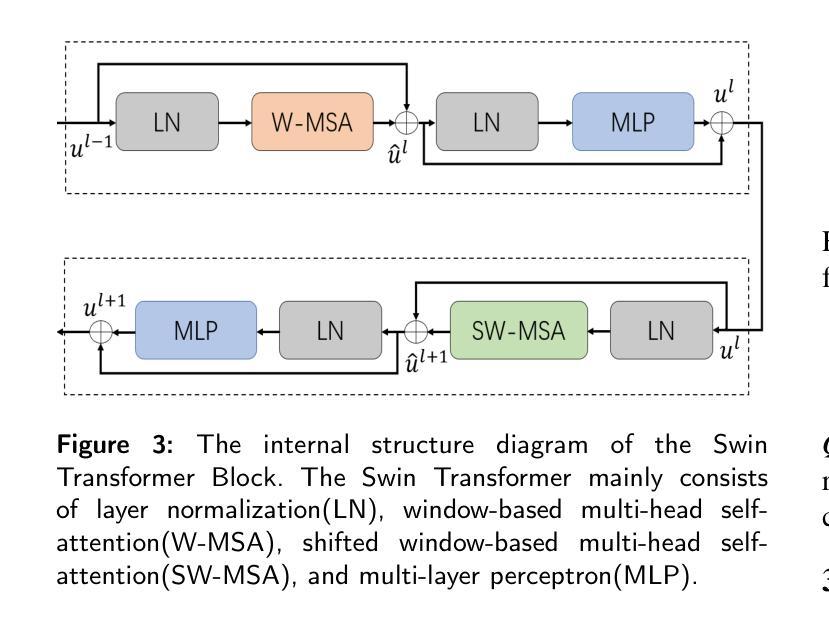
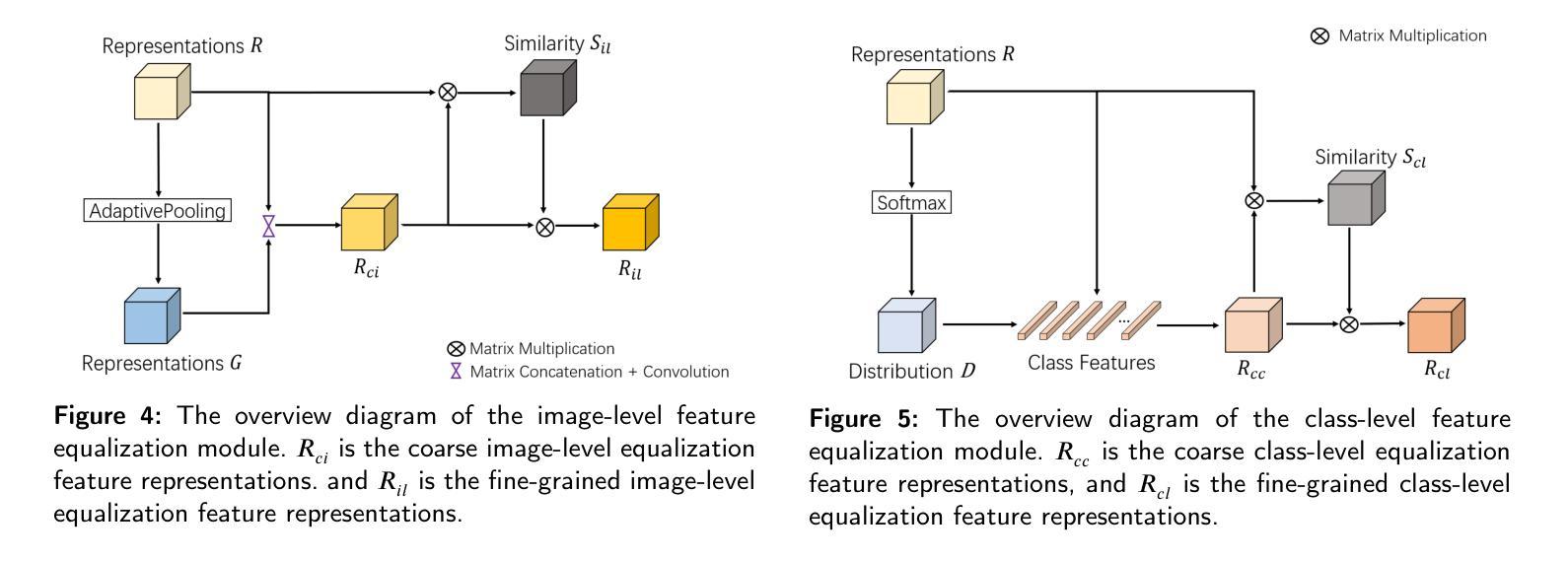
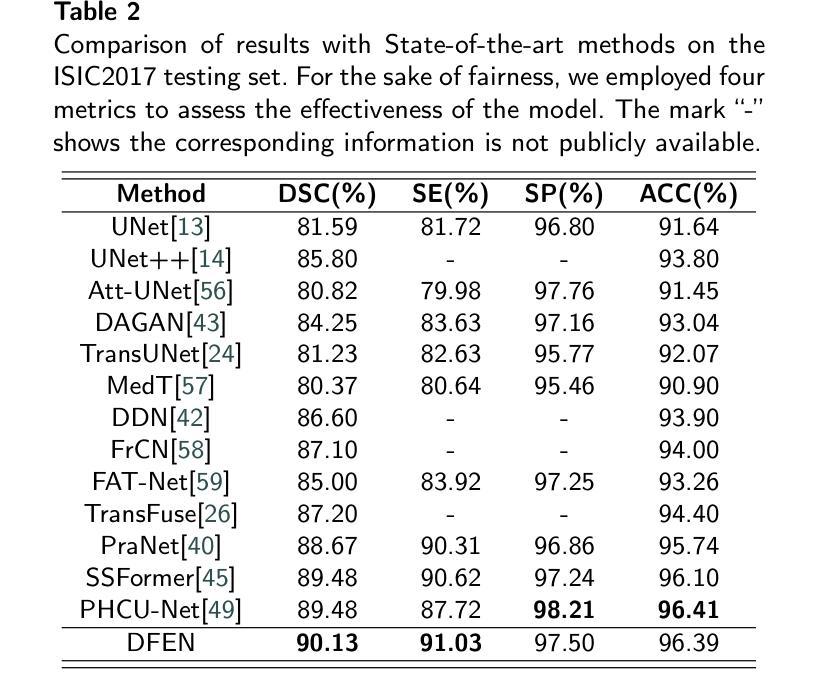
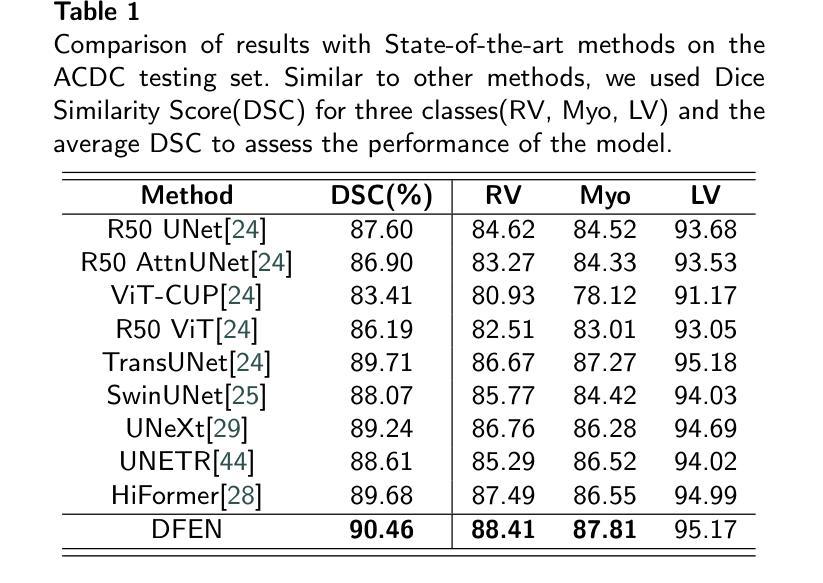