⚠️ 以下所有内容总结都来自于 大语言模型的能力,如有错误,仅供参考,谨慎使用
🔴 请注意:千万不要用于严肃的学术场景,只能用于论文阅读前的初筛!
💗 如果您觉得我们的项目对您有帮助 ChatPaperFree ,还请您给我们一些鼓励!⭐️ HuggingFace免费体验
2025-05-14 更新
RealRAG: Retrieval-augmented Realistic Image Generation via Self-reflective Contrastive Learning
Authors:Yuanhuiyi Lyu, Xu Zheng, Lutao Jiang, Yibo Yan, Xin Zou, Huiyu Zhou, Linfeng Zhang, Xuming Hu
Recent text-to-image generative models, e.g., Stable Diffusion V3 and Flux, have achieved notable progress. However, these models are strongly restricted to their limited knowledge, a.k.a., their own fixed parameters, that are trained with closed datasets. This leads to significant hallucinations or distortions when facing fine-grained and unseen novel real-world objects, e.g., the appearance of the Tesla Cybertruck. To this end, we present the first real-object-based retrieval-augmented generation framework (RealRAG), which augments fine-grained and unseen novel object generation by learning and retrieving real-world images to overcome the knowledge gaps of generative models. Specifically, to integrate missing memory for unseen novel object generation, we train a reflective retriever by self-reflective contrastive learning, which injects the generator’s knowledge into the sef-reflective negatives, ensuring that the retrieved augmented images compensate for the model’s missing knowledge. Furthermore, the real-object-based framework integrates fine-grained visual knowledge for the generative models, tackling the distortion problem and improving the realism for fine-grained object generation. Our Real-RAG is superior in its modular application to all types of state-of-the-art text-to-image generative models and also delivers remarkable performance boosts with all of them, such as a gain of 16.18% FID score with the auto-regressive model on the Stanford Car benchmark.
最近出现的文本到图像生成模型,如Stable Diffusion V3和Flux,已经取得了显著的进步。然而,这些模型受到其有限知识的强烈限制,即使用封闭数据集训练的固定参数。这导致在面对细粒度和未见的新现实世界对象(例如特斯拉Cybertruck的外观)时,会出现明显的幻觉或失真。为此,我们提出了首个基于真实对象的检索增强生成框架(RealRAG),通过学习和检索真实世界的图像,来克服生成模型的知识空白,从而增强细粒度和未见新对象的生成。具体来说,为了整合未见新对象生成的缺失记忆,我们通过自我反思对比学习训练了一个反射检索器,将生成器的知识注入到自我反思的否定样本中,确保检索到的增强图像能够弥补模型的缺失知识。此外,基于真实对象的框架为生成模型整合了细粒度视觉知识,解决了失真问题,提高了细粒度对象生成的逼真度。我们的RealRAG在模块化应用方面优于所有最先进的文本到图像生成模型,并且与它们结合都带来了显著的性能提升,例如在Stanford Car基准测试上,与自回归模型相比,FID得分提高了16.18%。
论文及项目相关链接
PDF Accepted to ICML2025
Summary
近期文本到图像的生成模型,如Stable Diffusion V3和Flux,取得了显著进展。然而,这些模型受限于固定参数和封闭数据集,面对细粒度和未见的新现实对象时,会产生幻觉或失真。为此,我们提出了基于真实对象的检索增强生成框架(RealRAG),通过学习和检索真实世界图像来克服生成模型的知识空白。该框架采用自我反思对比学习训练反射检索器,将生成器的知识注入自我反思的负样本中,确保检索到的增强图像补偿模型的缺失知识。此外,该框架还结合了细粒度视觉知识,解决生成模型的失真问题,提高细粒度对象生成的逼真度。RealRAG可应用于所有先进文本到图像生成模型,并带来显著性能提升,如在Stanford Car基准测试上,与自回归模型相比,FID得分提高了16.18%。
Key Takeaways
- 近期文本到图像的生成模型取得显著进展,但仍受限于固定参数和封闭数据集。
- 面对细粒度和未见的新现实对象时,这些模型会产生幻觉或失真。
- 提出了基于真实对象的检索增强生成框架(RealRAG)来克服生成模型的知识空白。
- RealRAG采用自我反思对比学习训练反射检索器,确保检索到的图像能补偿模型的缺失知识。
- RealRAG结合了细粒度视觉知识,提高生成模型的逼真度,并解决失真问题。
- RealRAG可应用于所有先进的文本到图像生成模型,并带来性能提升。
点此查看论文截图
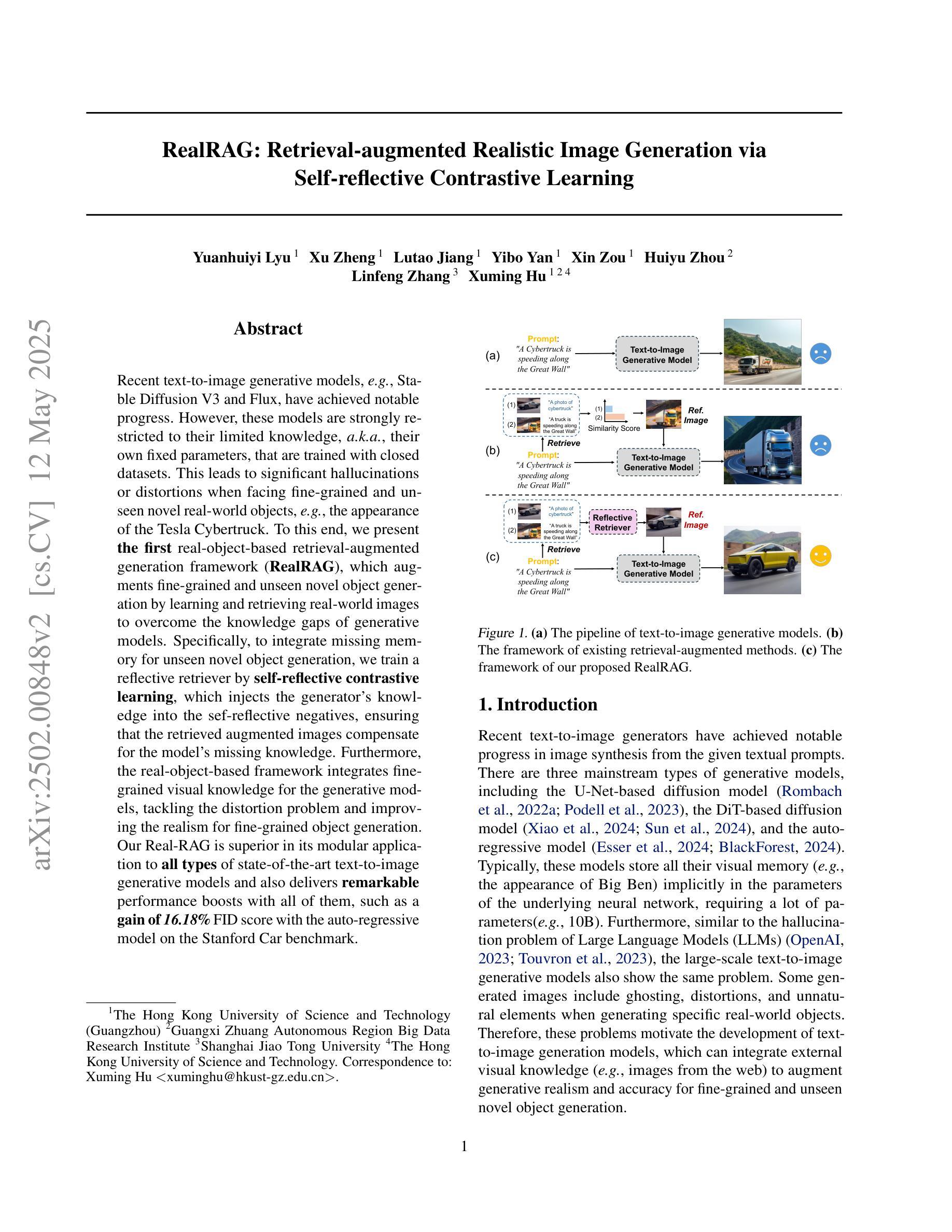
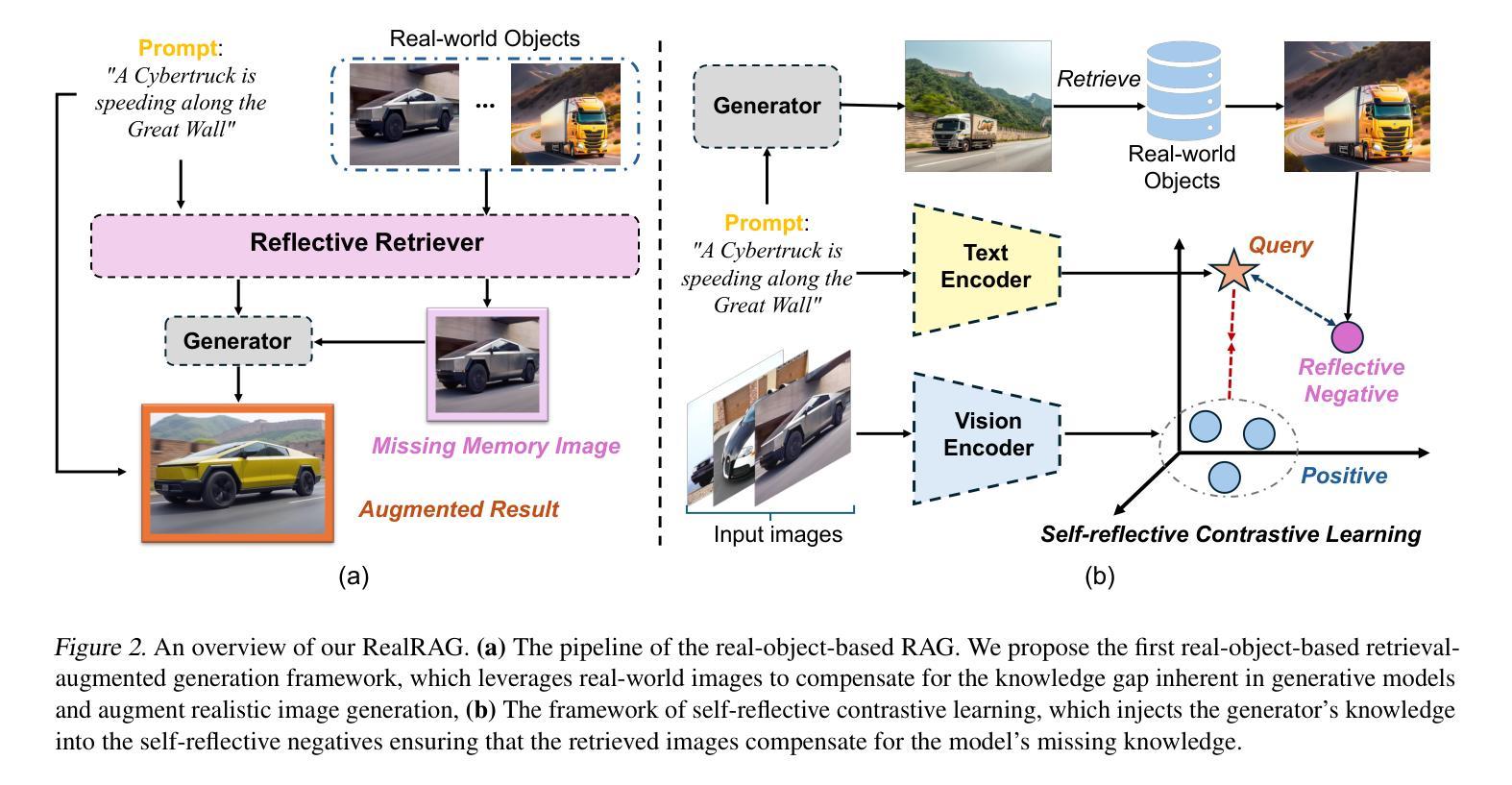
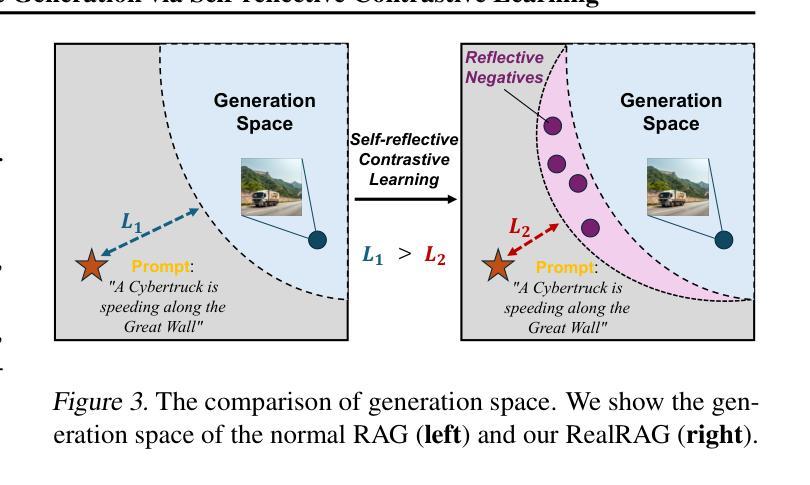
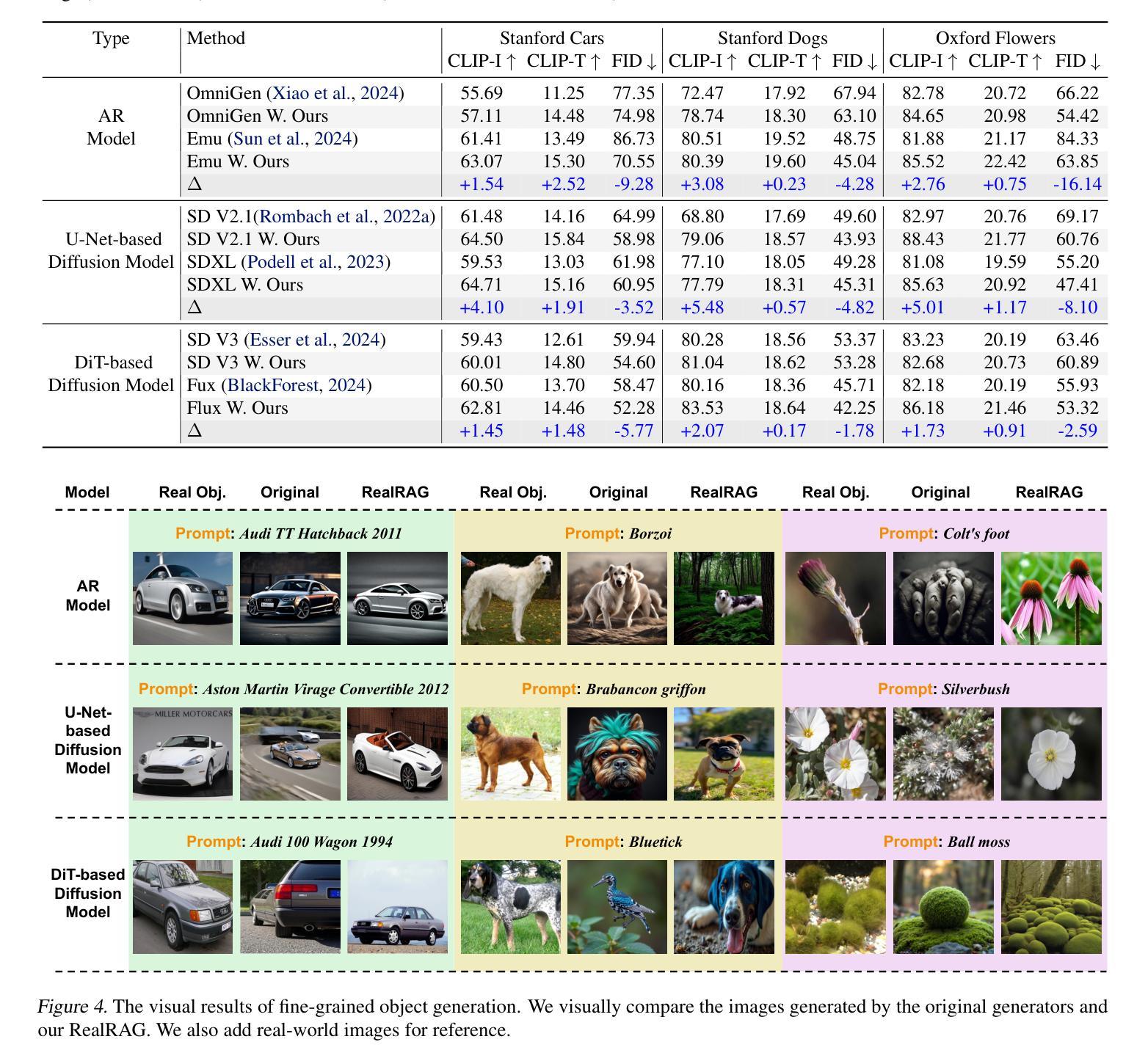
Similarity-Dissimilarity Loss for Multi-label Supervised Contrastive Learning
Authors:Guangming Huang, Yunfei Long, Cunjin Luo
Supervised contrastive learning has achieved remarkable success by leveraging label information; however, determining positive samples in multi-label scenarios remains a critical challenge. In multi-label supervised contrastive learning (MSCL), multi-label relations are not yet fully defined, leading to ambiguity in identifying positive samples and formulating contrastive loss functions to construct the representation space. To address these challenges, we: (i) first define five distinct multi-label relations in MSCL to systematically identify positive samples, (ii) introduce a novel Similarity-Dissimilarity Loss that dynamically re-weights samples through computing the similarity and dissimilarity factors between positive samples and given anchors based on multi-label relations, and (iii) further provide theoretical grounded proofs for our method through rigorous mathematical analysis that supports the formulation and effectiveness of the proposed loss function. We conduct the experiments across both image and text modalities, and extend the evaluation to medical domain. The results demonstrate that our method consistently outperforms baselines in a comprehensive evaluation, confirming its effectiveness and robustness. Code is available at: https://github.com/guangminghuang/similarity-dissimilarity-loss.
有监督对比学习通过利用标签信息取得了显著的成功,但在多标签场景中确定正样本仍然是一个关键挑战。在多标签有监督对比学习(MSCL)中,多标签关系尚未明确定义,导致正样本的识别以及对比损失函数的制定存在模糊性,从而构建表示空间。为了应对这些挑战,我们:(i)首先在MSCL中定义了五种不同的多标签关系,以系统地识别正样本;(ii)引入了一种新的相似性-差异性损失,通过计算正样本与给定锚点之间的相似性和差异性因素来动态地重新加权样本,这基于多标签关系;(iii)通过严格的数学分析为我们的方法提供了理论上的证明,支持所提出的损失函数的制定和有效性。我们在图像和文本模态上进行了实验,并将评估扩展到医疗领域。结果表明,我们的方法在综合评估中始终优于基线,证实了其有效性和稳健性。代码可用:https://github.com/guangminghuang/similarity-dissimilarity-loss。
论文及项目相关链接
Summary
该文本主要介绍了针对多标签监督对比学习(MSCL)中面临的正样本确定问题,通过定义五种多标签关系来系统地识别正样本,引入了一种新的相似度-差异度损失函数,并通过严格的数学分析证明了该方法的有效性。实验结果显示,该方法在图像和文本模态以及医疗领域均表现优异,优于基线方法。
Key Takeaways
- 多标签监督对比学习(MSCL)在确定正样本时面临挑战,因为多标签关系尚未明确定义。
- 提出了五种多标签关系的定义,以系统地识别正样本。
- 引入了一种新的相似度-差异度损失函数,通过计算正样本与给定锚点之间的相似度和差异度因子来动态重新加权样本。
- 通过严格的数学分析证明了该方法的有效性。
- 实验结果显示,该方法在图像和文本模态以及医疗领域均表现优异。
- 该方法在各种评估中均优于基线方法。
点此查看论文截图
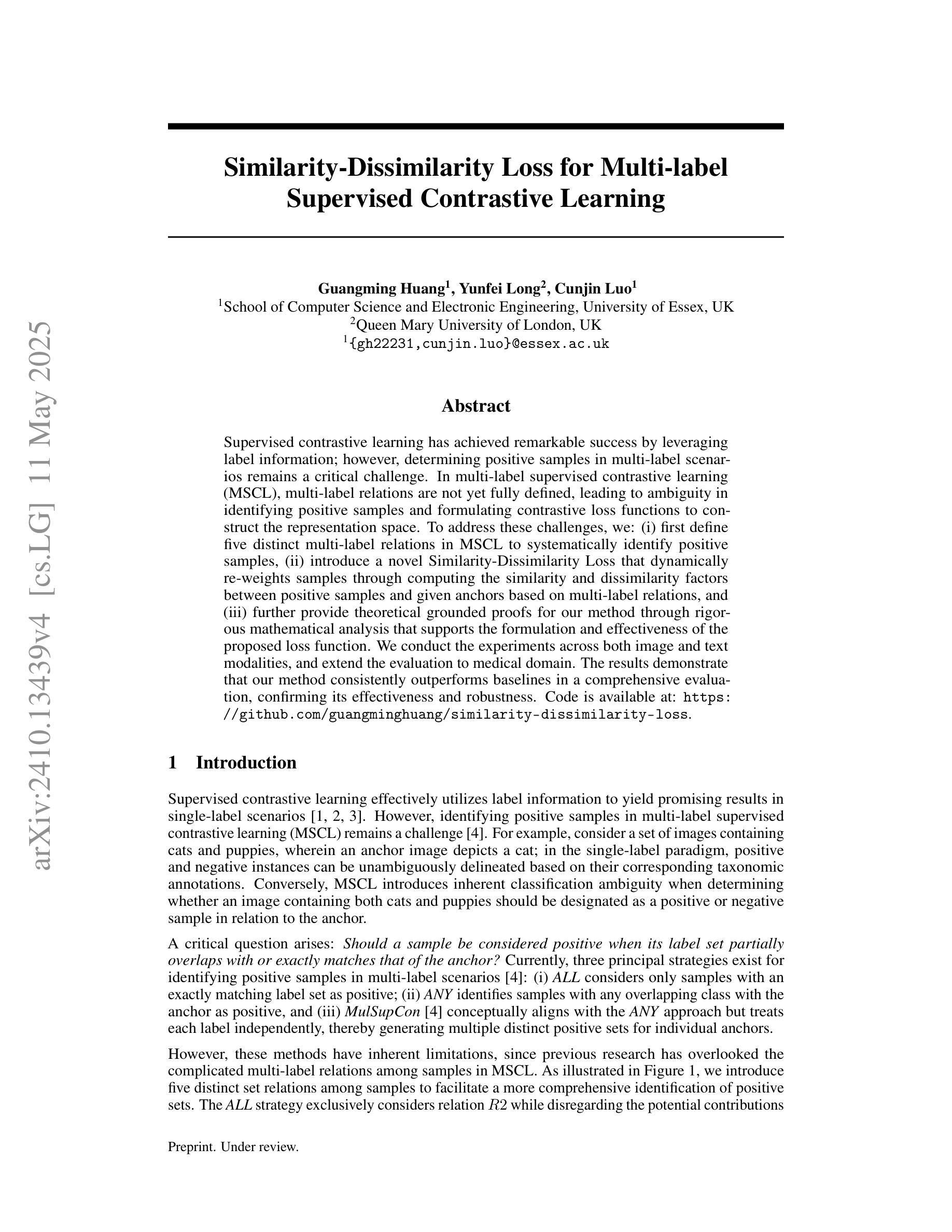
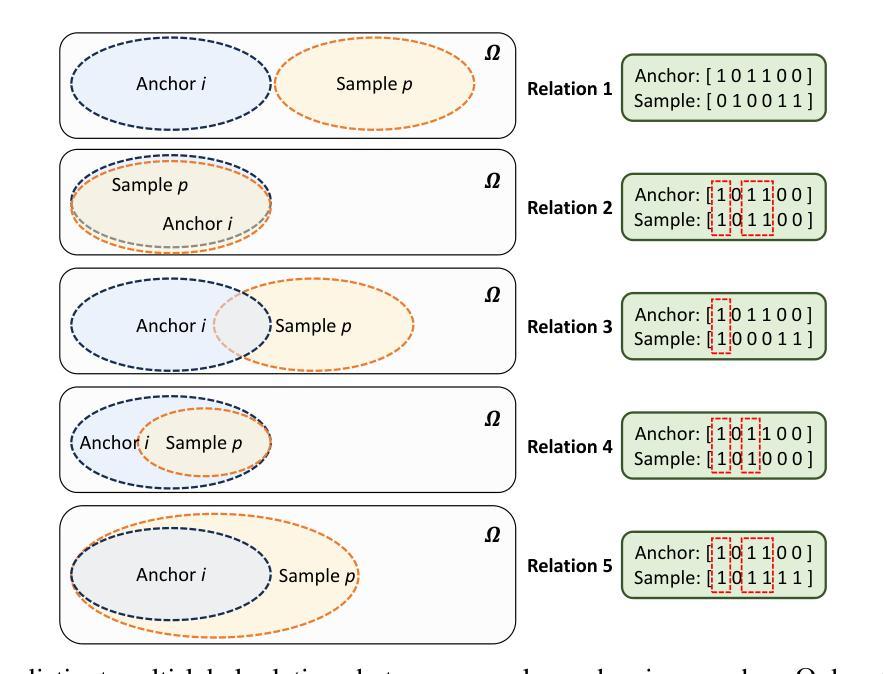