⚠️ 以下所有内容总结都来自于 大语言模型的能力,如有错误,仅供参考,谨慎使用
🔴 请注意:千万不要用于严肃的学术场景,只能用于论文阅读前的初筛!
💗 如果您觉得我们的项目对您有帮助 ChatPaperFree ,还请您给我们一些鼓励!⭐️ HuggingFace免费体验
2025-05-14 更新
TopicVD: A Topic-Based Dataset of Video-Guided Multimodal Machine Translation for Documentaries
Authors:Jinze Lv, Jian Chen, Zi Long, Xianghua Fu, Yin Chen
Most existing multimodal machine translation (MMT) datasets are predominantly composed of static images or short video clips, lacking extensive video data across diverse domains and topics. As a result, they fail to meet the demands of real-world MMT tasks, such as documentary translation. In this study, we developed TopicVD, a topic-based dataset for video-supported multimodal machine translation of documentaries, aiming to advance research in this field. We collected video-subtitle pairs from documentaries and categorized them into eight topics, such as economy and nature, to facilitate research on domain adaptation in video-guided MMT. Additionally, we preserved their contextual information to support research on leveraging the global context of documentaries in video-guided MMT. To better capture the shared semantics between text and video, we propose an MMT model based on a cross-modal bidirectional attention module. Extensive experiments on the TopicVD dataset demonstrate that visual information consistently improves the performance of the NMT model in documentary translation. However, the MMT model’s performance significantly declines in out-of-domain scenarios, highlighting the need for effective domain adaptation methods. Additionally, experiments demonstrate that global context can effectively improve translation performance. % Dataset and our implementations are available at https://github.com/JinzeLv/TopicVD
目前大多数多模态机器翻译(MMT)数据集主要由静态图像或短视频片段组成,缺乏跨不同领域和主题的大量视频数据。因此,它们无法满足现实世界中的MMT任务需求,如纪录片翻译。在本研究中,我们开发了TopicVD,这是一个用于视频支持的多模态机器翻译纪录片的基于主题的数据集,旨在推动该领域的研究。我们从纪录片中收集了视频字幕对,并将其分为经济、自然等八个主题,以促进视频指导的MMT领域适配研究。此外,我们保留了其上下文信息,以支持在视频指导的MMT中利用纪录片的全局上下文的研究。为了更好地捕捉文本和视频之间的共享语义,我们提出了一种基于跨模态双向注意模块的多模态翻译模型。在TopicVD数据集上的大量实验表明,视觉信息始终提高了自然语言翻译模型在纪录片翻译方面的性能。然而,在多模态翻译模型的性能在跨领域场景中显著下降,这凸显了有效领域适配方法的必要性。此外,实验表明全局上下文可以有效地提高翻译性能。数据集和我们的实现可在https://github.com/JinzeLv/TopicVD找到。
论文及项目相关链接
PDF NLDB 2025
Summary
本文介绍了多模态机器翻译(MMT)在纪录片翻译领域的研究现状。针对现有数据集缺乏跨领域和话题的丰富视频数据问题,研究者开发了一个基于话题的视频支持多模态机器翻译数据集——TopicVD。该数据集包含来自纪录片的视频字幕对,并按经济、自然等八个话题分类。研究还提出了一种基于跨模态双向注意力模块的多模态机器翻译模型,实验表明视觉信息能提高神经机器翻译模型在纪录片翻译中的性能,但跨领域场景性能显著下降,凸显出有效领域适应方法的重要性。全球语境信息可有效提升翻译性能。
Key Takeaways
- 当前MMT数据集主要局限于静态图像或短视频,缺乏跨领域的丰富视频数据。
- TopicVD数据集旨在解决这一问题,包含来自纪录片的视频字幕对,并按不同话题分类,有利于研究者在视频指导的多模态机器翻译中进行领域适应。
- TopicVD数据集保存了上下文信息,支持利用纪录片全局语境的研究。
- 提出了一种基于跨模态双向注意力模块的多模态机器翻译模型,能更好地捕捉文本和视频之间的共享语义。
- 实验显示视觉信息对提高神经机器翻译模型在纪录片翻译中的性能有积极作用。
- 在跨领域场景中,多模态机器翻译模型的性能显著下降,凸显出有效领域适应方法的重要性。
点此查看论文截图
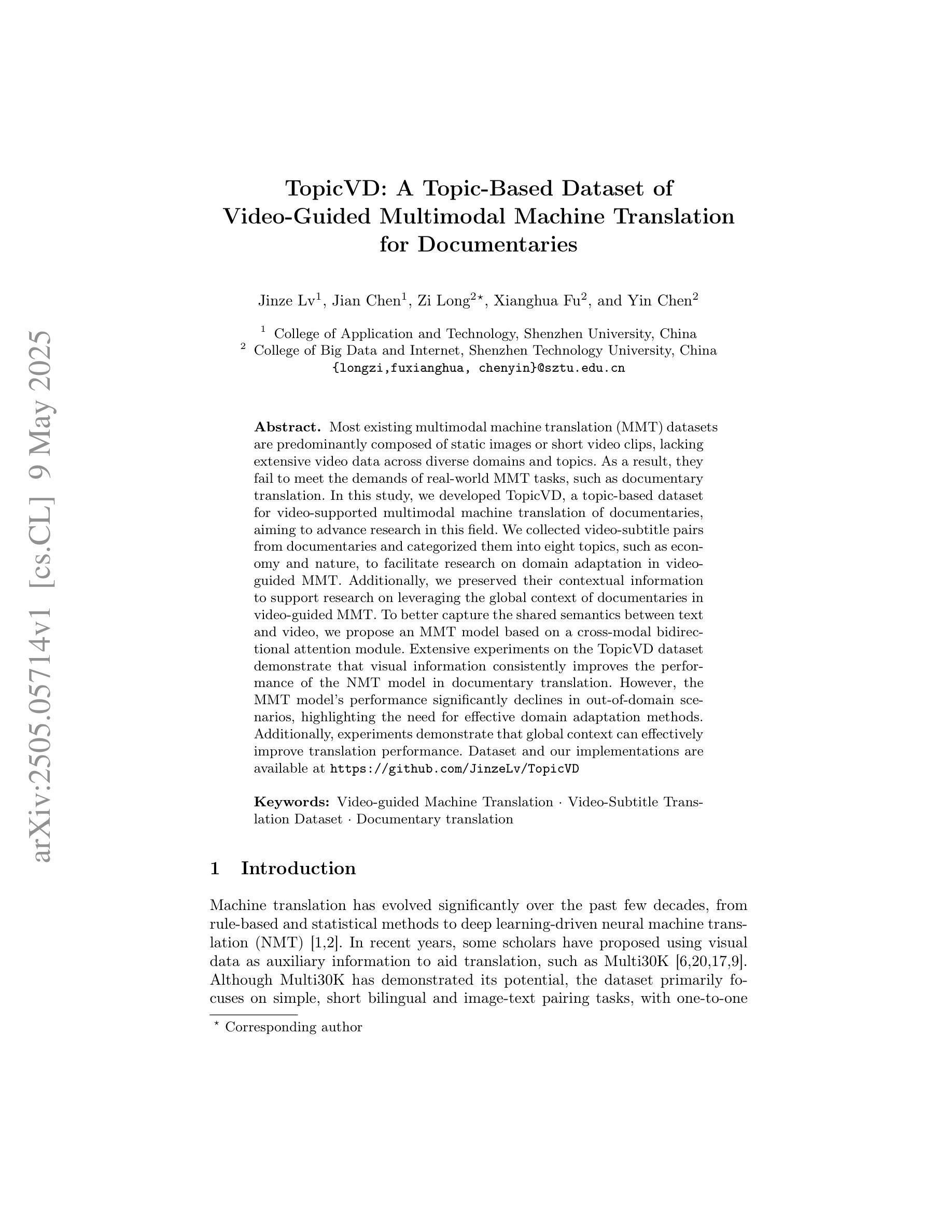
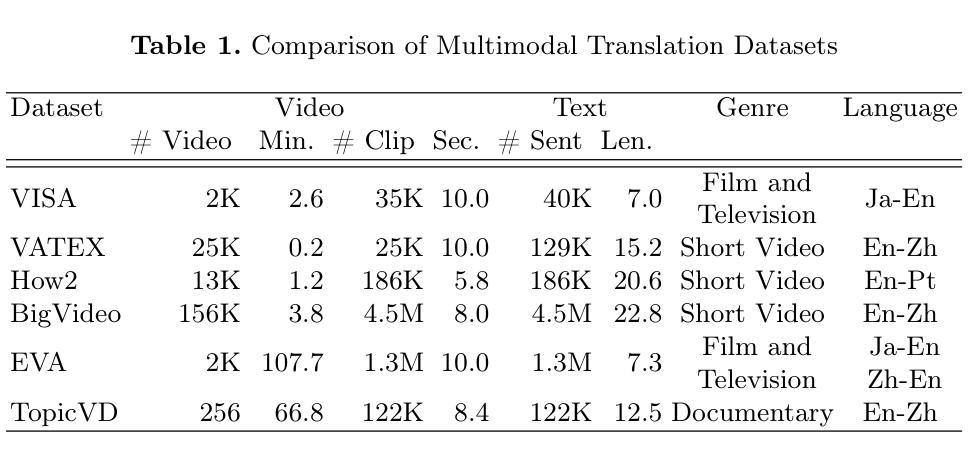
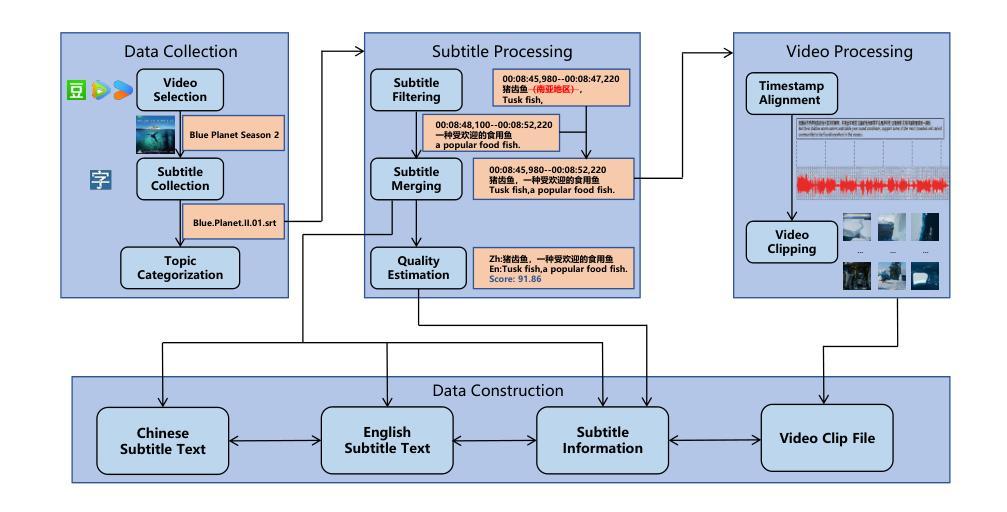