⚠️ 以下所有内容总结都来自于 大语言模型的能力,如有错误,仅供参考,谨慎使用
🔴 请注意:千万不要用于严肃的学术场景,只能用于论文阅读前的初筛!
💗 如果您觉得我们的项目对您有帮助 ChatPaperFree ,还请您给我们一些鼓励!⭐️ HuggingFace免费体验
2025-05-14 更新
Less is More: Improving Motion Diffusion Models with Sparse Keyframes
Authors:Jinseok Bae, Inwoo Hwang, Young Yoon Lee, Ziyu Guo, Joseph Liu, Yizhak Ben-Shabat, Young Min Kim, Mubbasir Kapadia
Recent advances in motion diffusion models have led to remarkable progress in diverse motion generation tasks, including text-to-motion synthesis. However, existing approaches represent motions as dense frame sequences, requiring the model to process redundant or less informative frames. The processing of dense animation frames imposes significant training complexity, especially when learning intricate distributions of large motion datasets even with modern neural architectures. This severely limits the performance of generative motion models for downstream tasks. Inspired by professional animators who mainly focus on sparse keyframes, we propose a novel diffusion framework explicitly designed around sparse and geometrically meaningful keyframes. Our method reduces computation by masking non-keyframes and efficiently interpolating missing frames. We dynamically refine the keyframe mask during inference to prioritize informative frames in later diffusion steps. Extensive experiments show that our approach consistently outperforms state-of-the-art methods in text alignment and motion realism, while also effectively maintaining high performance at significantly fewer diffusion steps. We further validate the robustness of our framework by using it as a generative prior and adapting it to different downstream tasks.
近期动作扩散模型的进展在多种运动生成任务中取得了显著进展,包括文本到运动的合成。然而,现有方法将运动表示为密集帧序列,要求模型处理冗余或信息较少的帧。密集动画帧的处理带来了很大的训练复杂性,尤其是当使用现代神经网络架构学习大型运动数据集复杂分布时更是如此。这严重限制了生成运动模型在下游任务中的性能。受到专注于稀疏关键帧的专业动画师的启发,我们提出了一种围绕稀疏和几何意义明确的关键帧明确设计的新型扩散框架。我们的方法通过屏蔽非关键帧来减少计算量,并有效地插值缺失帧。我们在推理过程中动态调整关键帧掩码,以在后续的扩散步骤中优先处理信息帧。大量实验表明,我们的方法在文本对齐和运动逼真度方面始终优于最先进的方法,同时在显著减少的扩散步骤中保持高性能。我们还通过将其作为生成先验并适应不同的下游任务来验证我们框架的稳健性。
论文及项目相关链接
Summary
近期运动扩散模型的进步在多种运动生成任务中取得了显著成效,包括文本到运动的合成。然而,现有方法将运动表示为密集帧序列,要求模型处理冗余或信息较少的帧,增加了训练复杂度,尤其是在学习大型运动数据集复杂分布时,即使使用现代神经网络架构亦是如此。这严重限制了生成运动模型在下游任务中的表现。本研究受专业动画师专注于稀疏关键帧的启发,提出一个围绕稀疏和几何意义关键帧设计的新型扩散框架。该方法通过掩去非关键帧来减少计算量,并有效插值缺失帧。在推理过程中,我们动态调整关键帧掩膜以优先处理后续扩散步骤中的信息帧。实验表明,我们的方法在文本对齐和运动真实感方面始终优于现有先进技术,同时在显著减少扩散步骤的情况下保持高性能。我们还通过将其作为生成先验并适应不同的下游任务来验证我们框架的稳健性。
Key Takeaways
- 现有运动生成模型处理密集帧序列存在冗余和信息不全面的问题。
- 冗余帧增加了训练复杂度和计算成本,影响模型性能。
- 扩散框架现以稀疏关键帧为基础,提高效率和性能。
- 框架通过掩去非关键帧和插值缺失帧来减少计算量。
- 动态调整关键帧掩膜可优先处理信息帧,提高推理性能。
- 实验表明新方法在文本对齐和运动真实感方面超越现有技术。
点此查看论文截图
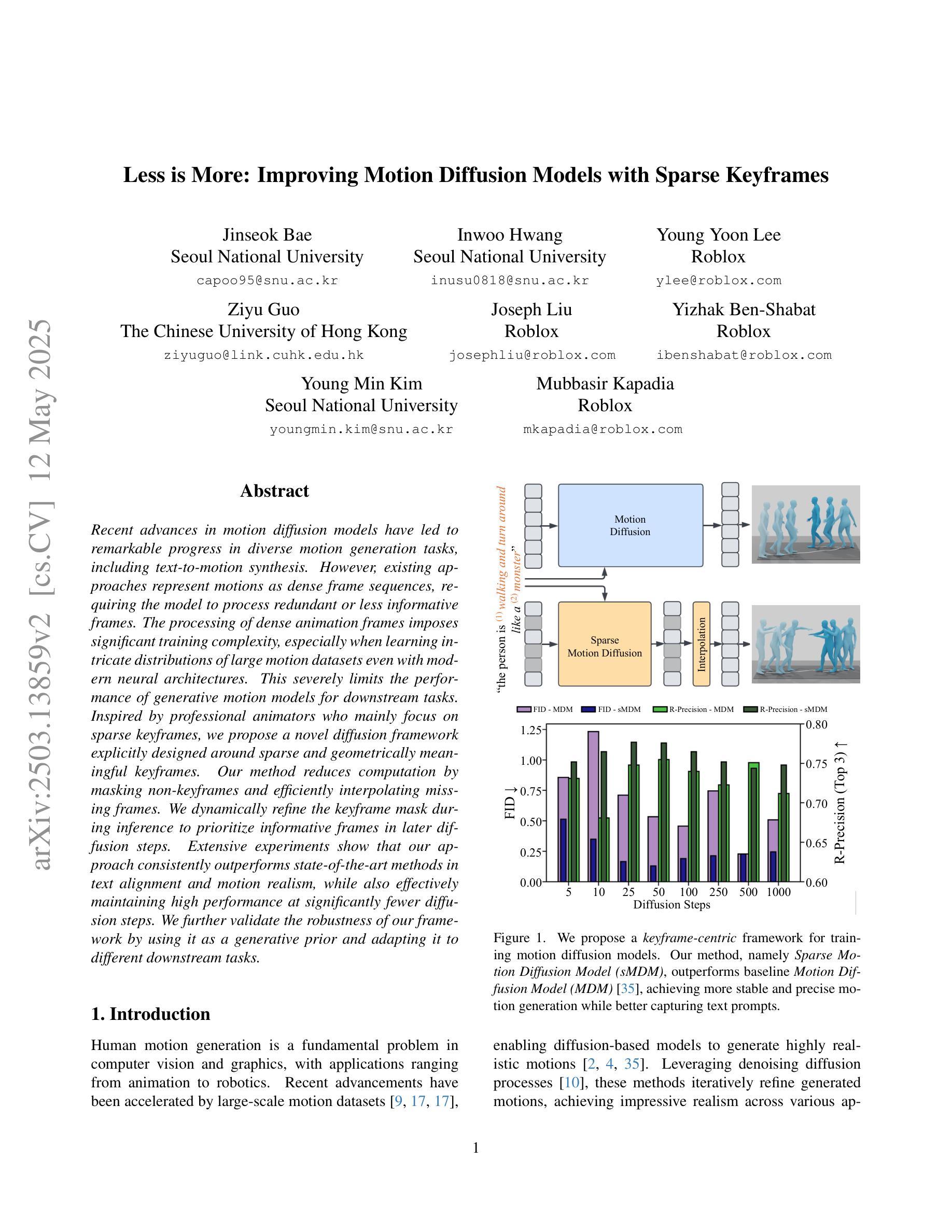
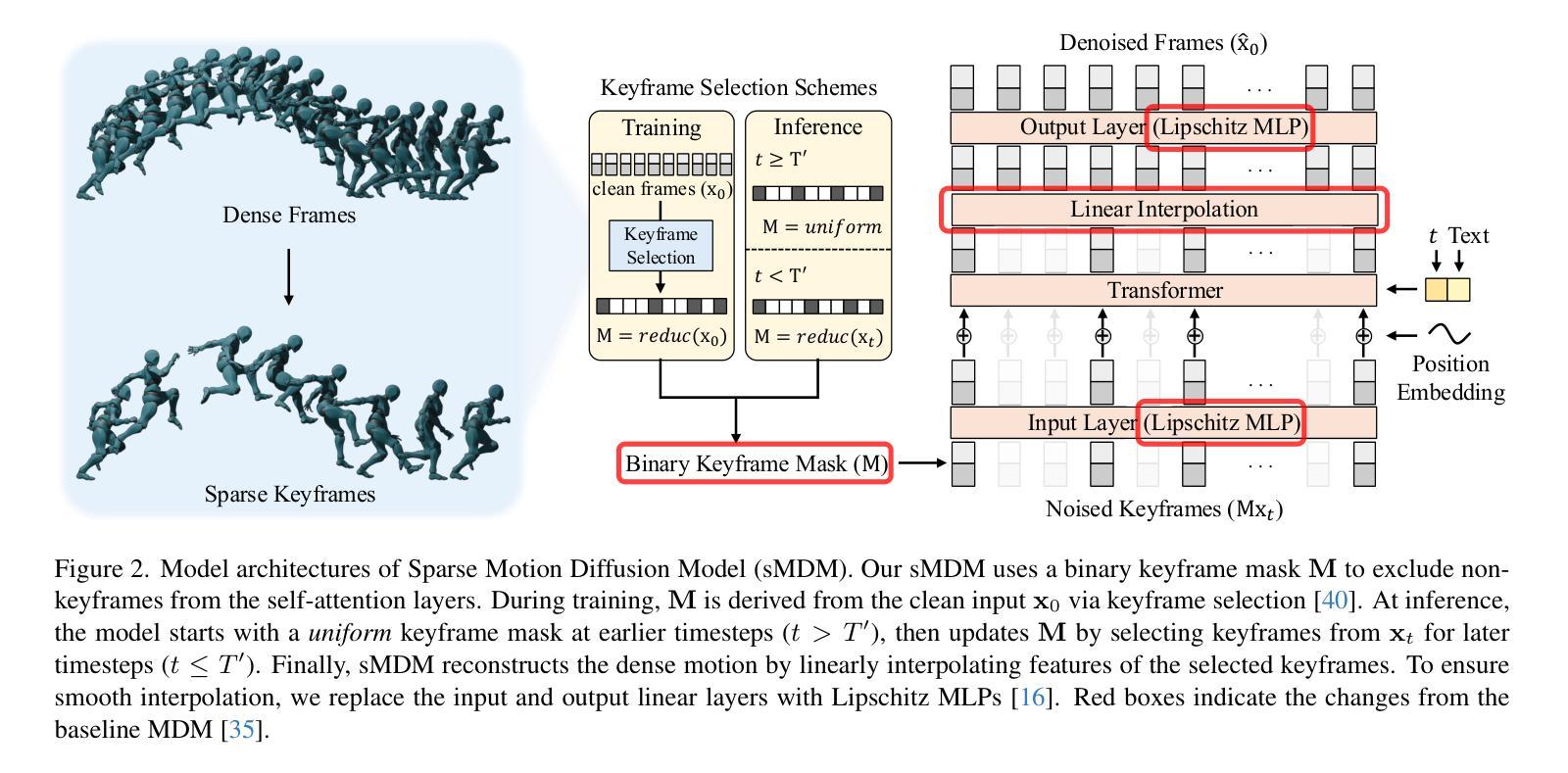
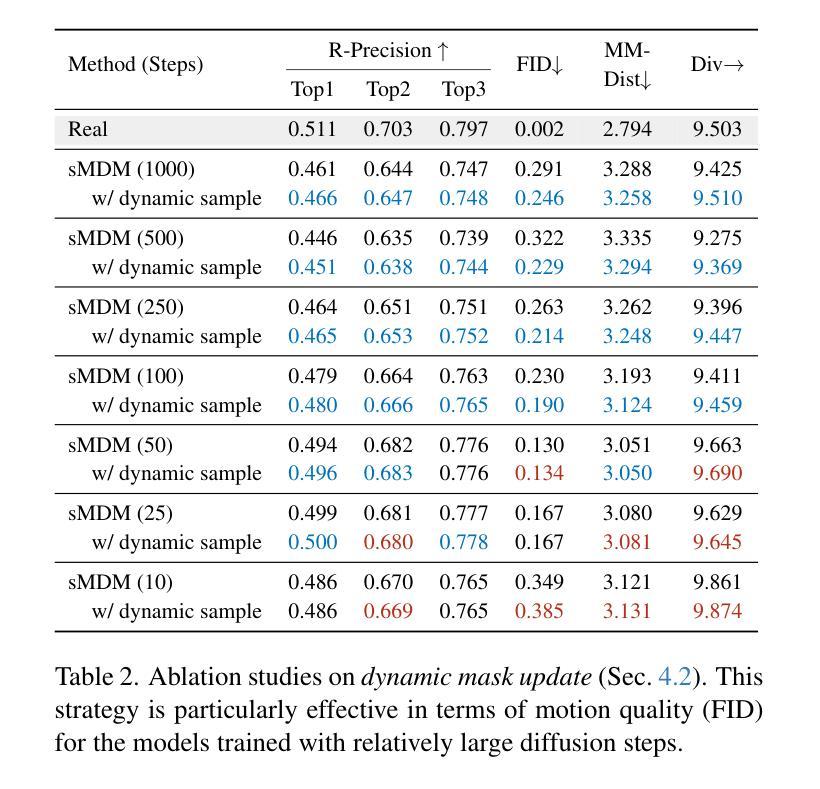
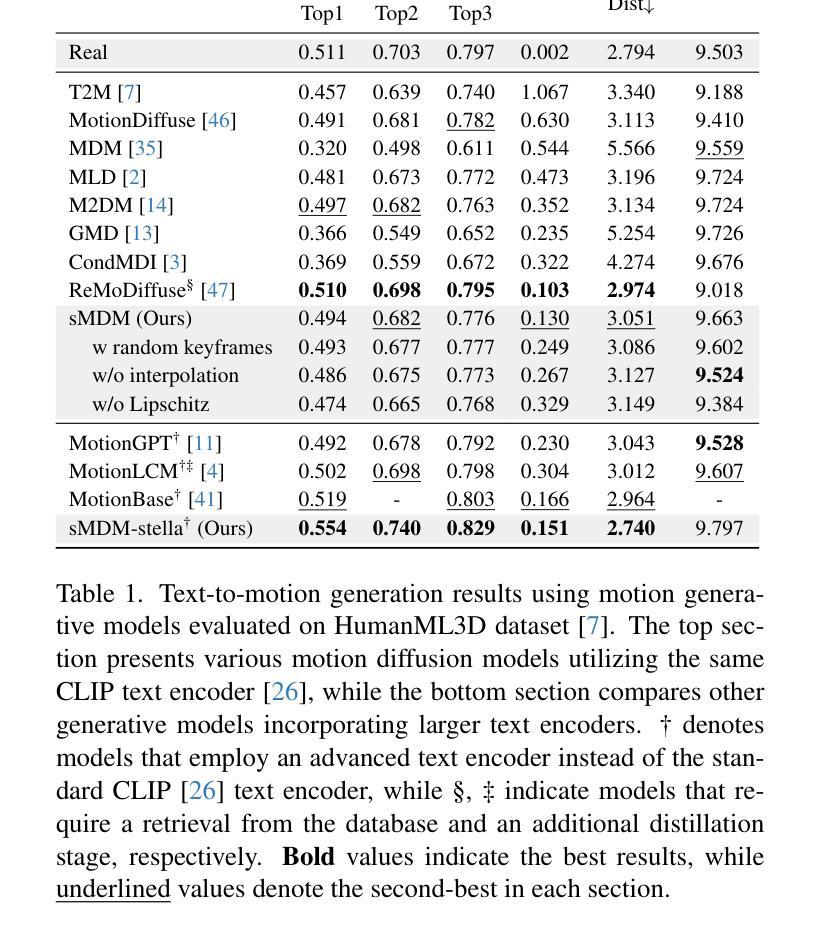
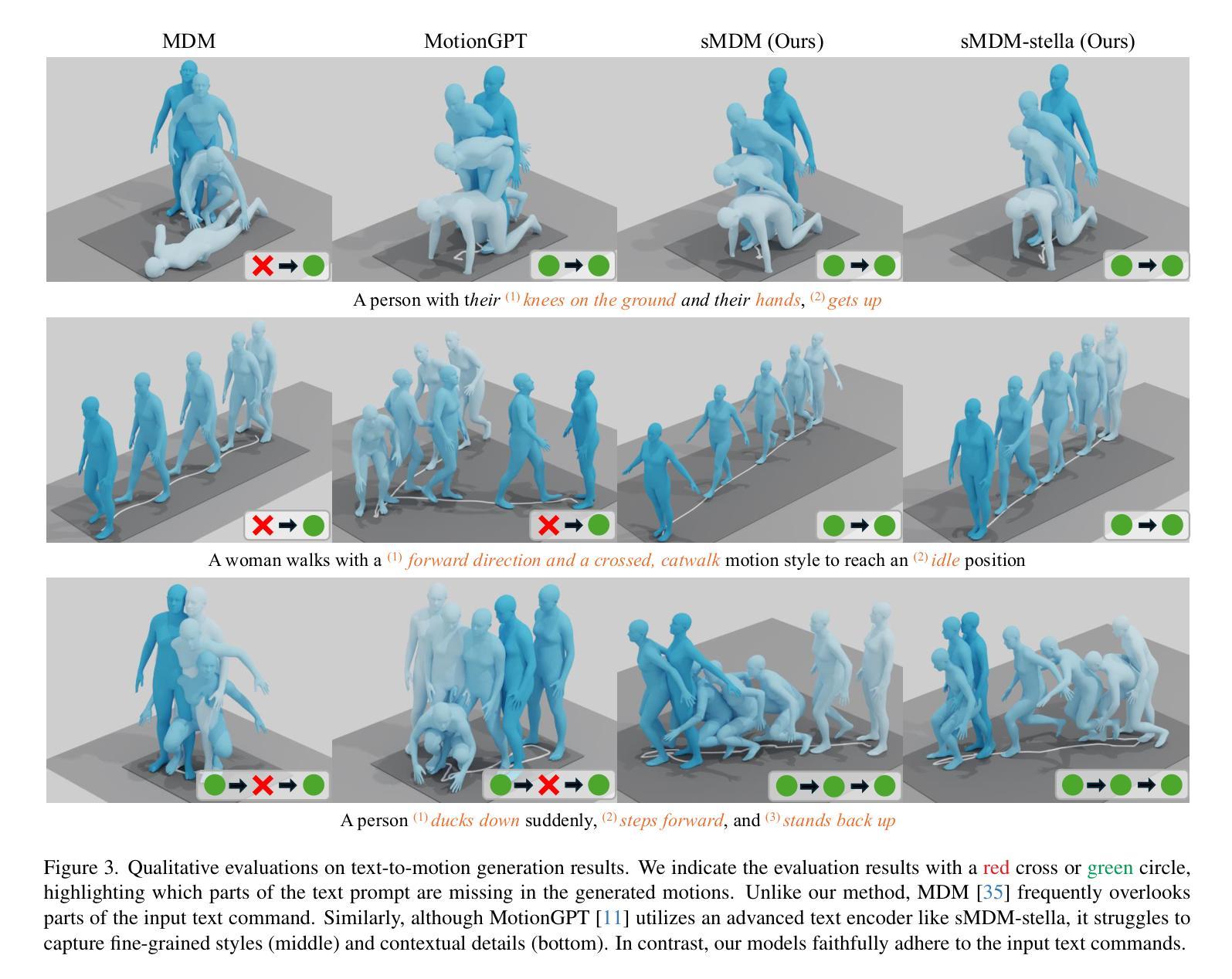
CrowdMoGen: Zero-Shot Text-Driven Collective Motion Generation
Authors:Yukang Cao, Xinying Guo, Mingyuan Zhang, Haozhe Xie, Chenyang Gu, Ziwei Liu
While recent advances in text-to-motion generation have shown promising results, they typically assume all individuals are grouped as a single unit. Scaling these methods to handle larger crowds and ensuring that individuals respond appropriately to specific events remains a significant challenge. This is primarily due to the complexities of scene planning, which involves organizing groups, planning their activities, and coordinating interactions, and controllable motion generation. In this paper, we present CrowdMoGen, the first zero-shot framework for collective motion generation, which effectively groups individuals and generates event-aligned motion sequences from text prompts. 1) Being limited by the available datasets for training an effective scene planning module in a supervised manner, we instead propose a crowd scene planner that leverages pre-trained large language models (LLMs) to organize individuals into distinct groups. While LLMs offer high-level guidance for group divisions, they lack the low-level understanding of human motion. To address this, we further propose integrating an SMPL-based joint prior to generate context-appropriate activities, which consists of both joint trajectories and textual descriptions. 2) Secondly, to incorporate the assigned activities into the generative network, we introduce a collective motion generator that integrates the activities into a transformer-based network in a joint-wise manner, maintaining the spatial constraints during the multi-step denoising process. Extensive experiments demonstrate that CrowdMoGen significantly outperforms previous approaches, delivering realistic, event-driven motion sequences that are spatially coherent. As the first framework of collective motion generation, CrowdMoGen has the potential to advance applications in urban simulation, crowd planning, and other large-scale interactive environments.
虽然文本到运动的生成技术最近取得了令人瞩目的进展,但它们通常假设所有个体都被视为一个单一单位。将这些方法扩展到处理更大的群体,并确保个体对特定事件做出适当反应,仍然是一个巨大的挑战。这主要是因为场景规划的复杂性,它涉及到组织群体、规划活动和协调交互以及可控的运动生成。在本文中,我们提出了CrowdMoGen,这是第一个用于集体运动生成的零样本框架,它可以有效地将个体分组,并根据文本提示生成与事件相对应的运动序列。首先,由于可用数据集的限制,我们无法以监督的方式训练有效的场景规划模块,因此我们提出了一种利用预训练的大型语言模型(LLM)对个体进行分组的群众场景规划器。虽然LLM为分组提供了高级指导,但它们缺乏对人类运动的低级理解。为了解决这一问题,我们进一步提出结合SMPL的关节先验来生成与上下文相适应的活动,这些活动既包括关节轨迹也包括文本描述。其次,为了将分配的活动融入生成网络,我们引入了一个集体运动生成器,它以关节方式将活动融入基于变压器的网络,在多步去噪过程中保持空间约束。大量实验表明,CrowdMoGen显著优于以前的方法,能够生成逼真的、事件驱动的运动序列,这些序列在空间上是连贯的。作为第一个集体运动生成框架,CrowdMoGen在都市模拟、人群规划以及其他大规模交互环境中具有广泛的应用潜力。
论文及项目相关链接
PDF Project page: https://yukangcao.github.io/CrowdMoGen
摘要
本文提出了一种名为CrowdMoGen的零样本集体运动生成框架,该框架可根据文本提示有效分组个体并生成与事件对齐的运动序列。为解决训练有效场景规划模块受限于可用数据集的问题,研究提出了利用预训练的大型语言模型(LLM)对个体进行分组。为解决LLM缺乏人类运动低级理解的问题,结合了SMPL基础关节先验来生成与上下文相适应的活动。同时,为了将分配的活动融入生成网络,研究引入了集体运动生成器,以关节方式将活动融入基于变压器的网络,并在多步去噪过程中保持空间约束。实验表明,CrowdMoGen显著优于先前的方法,能够生成逼真的、事件驱动且空间连贯的运动序列。作为集体运动生成的首个框架,CrowdMoGen在都市模拟、人群规划以及其他大规模交互环境中具有广泛的应用潜力。
关键见解
- CrowdMoGen是首个零样本集体运动生成框架,能够实现根据文本提示分组个体并生成与事件对齐的运动序列。
2.研究提出了利用预训练的大型语言模型(LLM)对个体进行分组的方案,解决了训练场景规划模块受限于数据集的问题。
3.结合SMPL基础关节先验生成与上下文相适应的活动,解决大型语言模型缺乏人类运动低级理解的问题。
4.引入集体运动生成器,以关节方式将活动融入基于变压器的网络,并保持空间约束。
5.CrowdMoGen生成的运动序列具有逼真性、事件驱动性以及空间连贯性。
6.与先前的方法相比,CrowdMoGen表现出显著优势。
点此查看论文截图
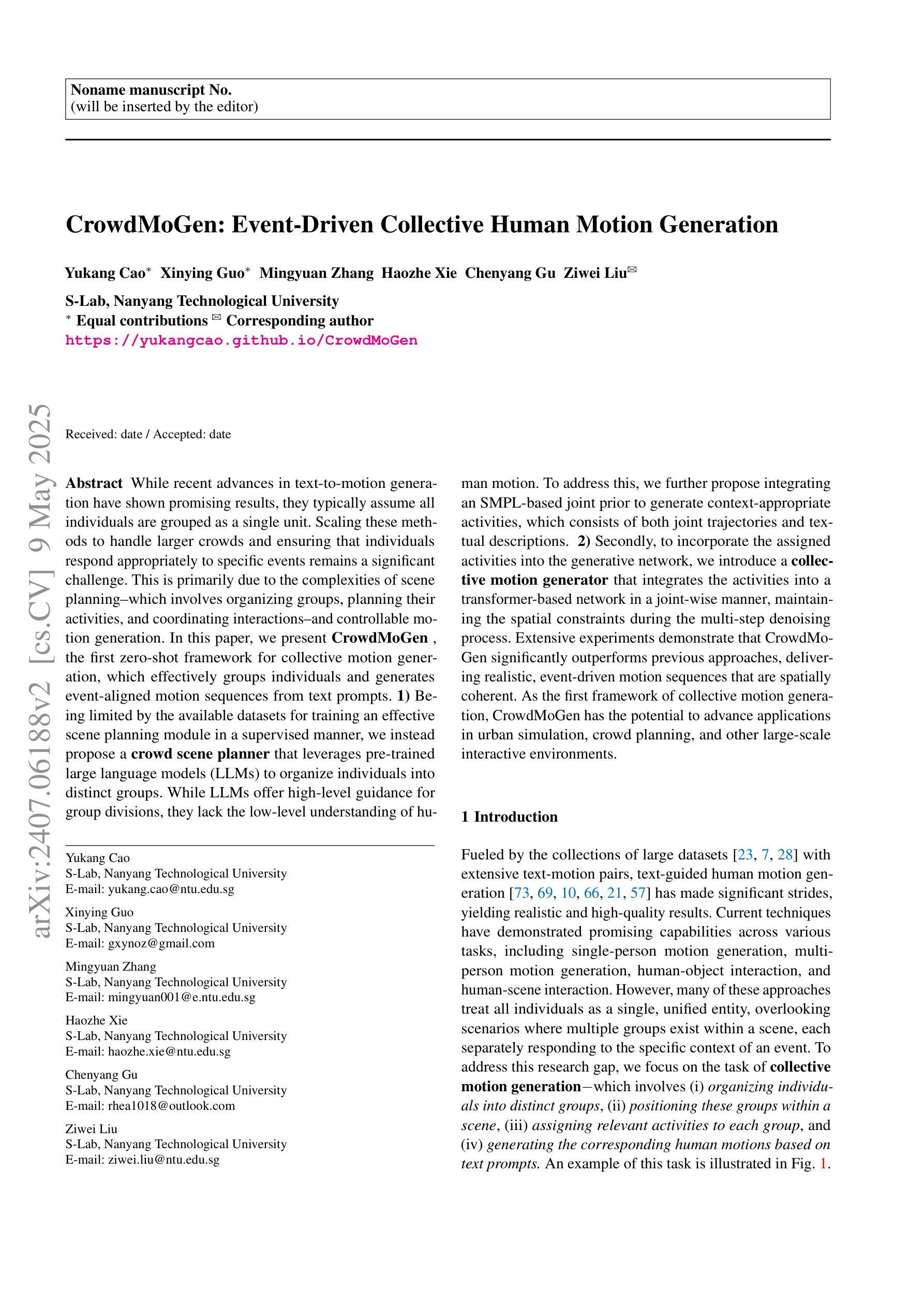
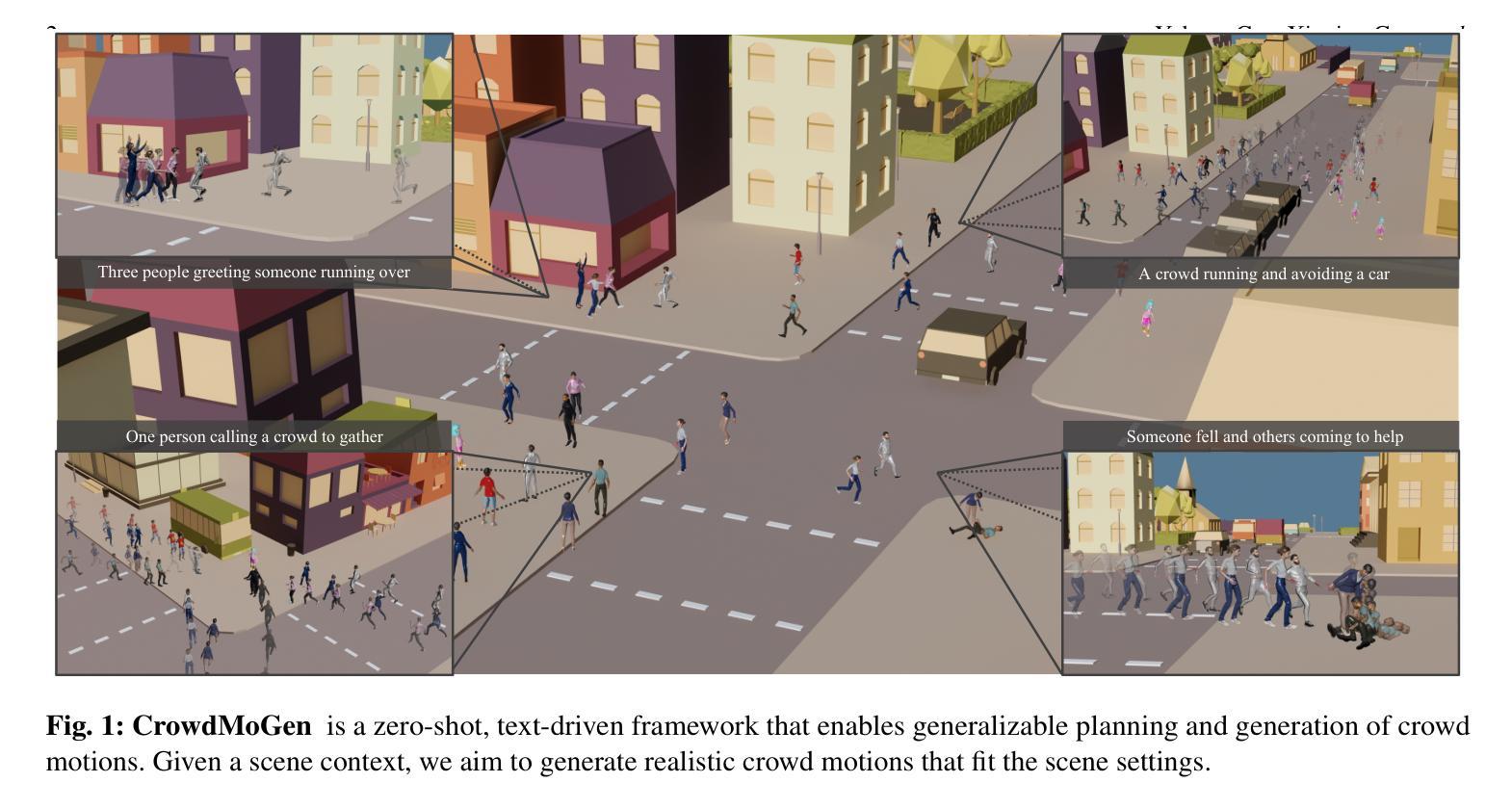
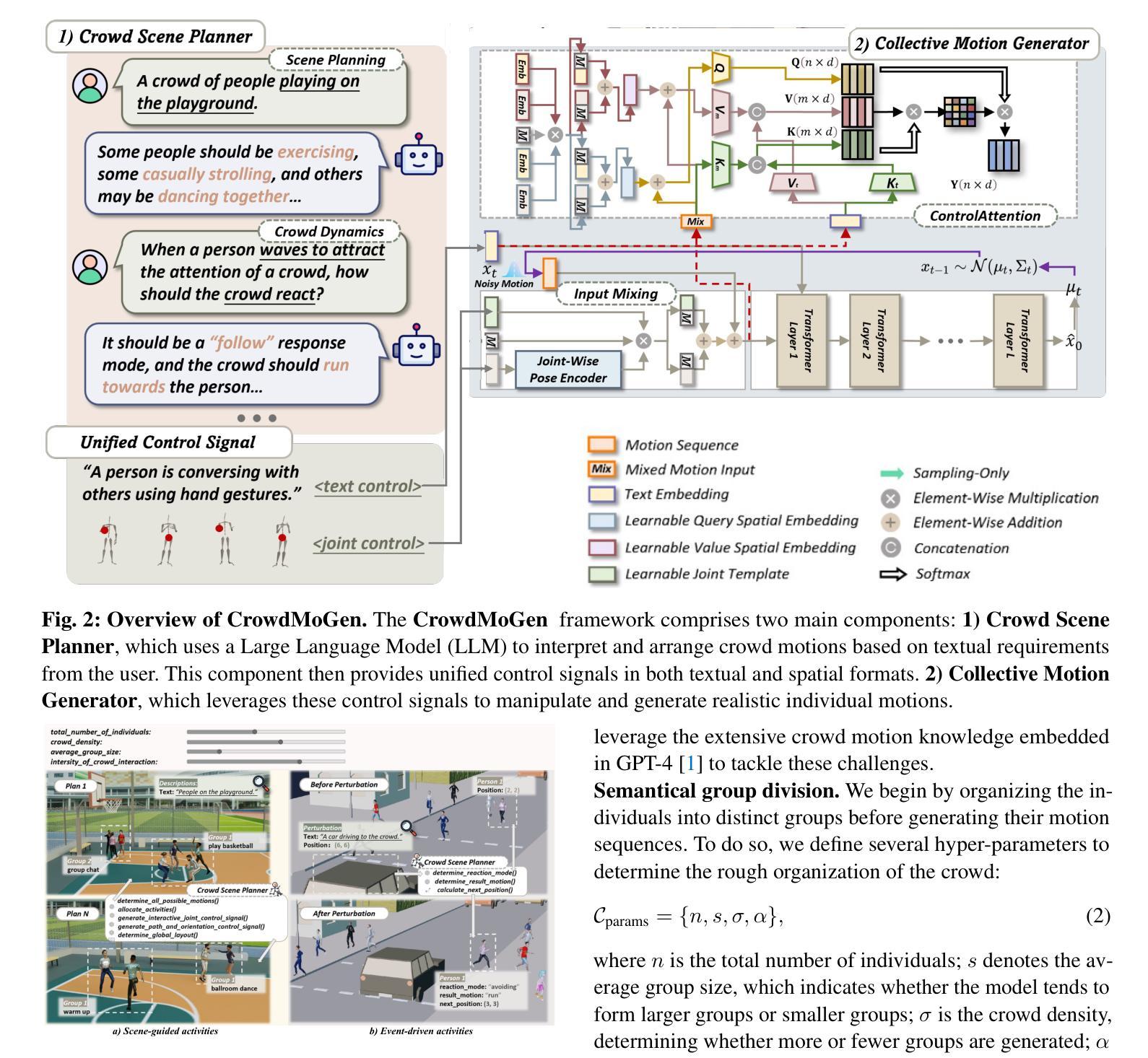
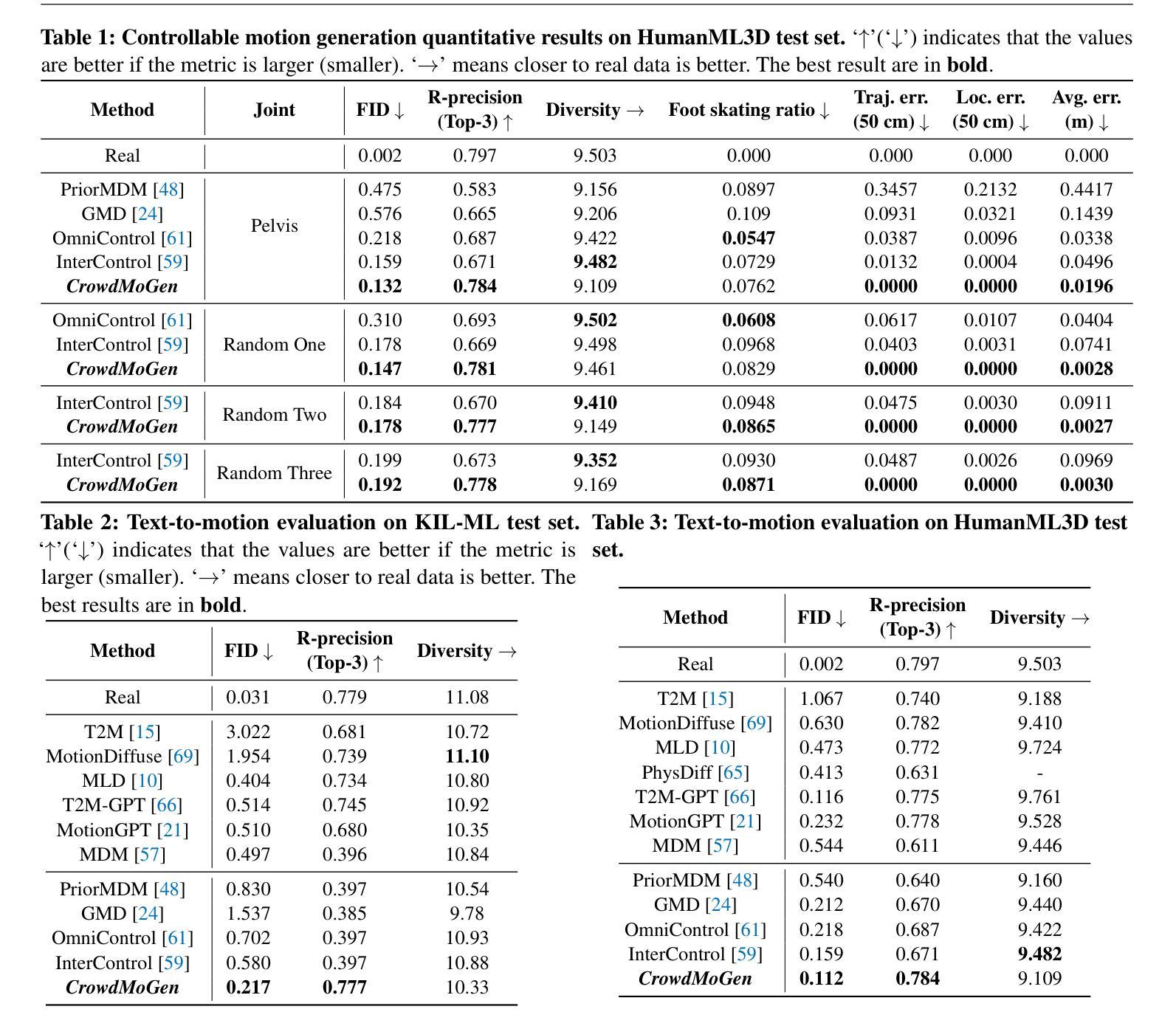