⚠️ 以下所有内容总结都来自于 大语言模型的能力,如有错误,仅供参考,谨慎使用
🔴 请注意:千万不要用于严肃的学术场景,只能用于论文阅读前的初筛!
💗 如果您觉得我们的项目对您有帮助 ChatPaperFree ,还请您给我们一些鼓励!⭐️ HuggingFace免费体验
2025-05-15 更新
DArFace: Deformation Aware Robustness for Low Quality Face Recognition
Authors:Sadaf Gulshad, Abdullah Aldahlawi Thakaa
Facial recognition systems have achieved remarkable success by leveraging deep neural networks, advanced loss functions, and large-scale datasets. However, their performance often deteriorates in real-world scenarios involving low-quality facial images. Such degradations, common in surveillance footage or standoff imaging include low resolution, motion blur, and various distortions, resulting in a substantial domain gap from the high-quality data typically used during training. While existing approaches attempt to address robustness by modifying network architectures or modeling global spatial transformations, they frequently overlook local, non-rigid deformations that are inherently present in real-world settings. In this work, we introduce DArFace, a Deformation-Aware robust Face recognition framework that enhances robustness to such degradations without requiring paired high- and low-quality training samples. Our method adversarially integrates both global transformations (e.g., rotation, translation) and local elastic deformations during training to simulate realistic low-quality conditions. Moreover, we introduce a contrastive objective to enforce identity consistency across different deformed views. Extensive evaluations on low-quality benchmarks including TinyFace, IJB-B, and IJB-C demonstrate that DArFace surpasses state-of-the-art methods, with significant gains attributed to the inclusion of local deformation modeling.
人脸识别系统通过利用深度神经网络、先进的损失函数和大规模数据集取得了显著的成功。然而,在处理涉及低质量面部图像的现实场景时,它们的性能往往会下降。这种退化在监控录像或远距离成像中很常见,包括低分辨率、运动模糊和各种失真,与训练期间通常使用的高质量数据之间存在很大的领域差距。虽然现有的方法试图通过修改网络架构或建立全局空间变换来提高稳健性,但它们往往忽略了现实环境中固有的局部非刚性变形。在这项工作中,我们引入了DArFace,一个基于变形的稳健人脸识别框架,它提高了对这种退化的稳健性,而无需配对的高质量低质量训练样本。我们的方法通过模拟真实的低质量条件,在训练中对抗性地结合了全局变换(如旋转、平移)和局部弹性变形。此外,我们引入了一个对比目标,以在不同变形的视图中强制执行身份一致性。在包括TinyFace、IJB-B和IJB.C在内的低质量基准测试上的广泛评估表明,DArFace超越了最先进的方法,其显著的提升归因于局部变形建模的引入。
论文及项目相关链接
Summary
人脸识别系统在实际应用中面临低质量图像的问题,如监控视频或远距离成像中的分辨率低、运动模糊和各种失真。为提高对这类问题的鲁棒性,DArFace框架通过集成全局变换和局部弹性变形,模拟真实低质量条件,并在不同变形视图中强制执行身份一致性。其在低质量基准测试上的表现超过了现有技术。
Key Takeaways
- 人脸识别系统在实际应用中可能遇到低质量图像问题,如分辨率低、运动模糊和失真等。
- DArFace框架旨在提高人脸识别系统对这些问题的鲁棒性。
- DArFace通过集成全局变换和局部弹性变形,模拟真实低质量条件。
- 该框架使用对比目标来强制执行不同变形视图中的身份一致性。
- 在低质量基准测试上,DArFace的表现超过了现有技术。
- DArFace的优势主要来自于对局部变形建模的纳入。
点此查看论文截图
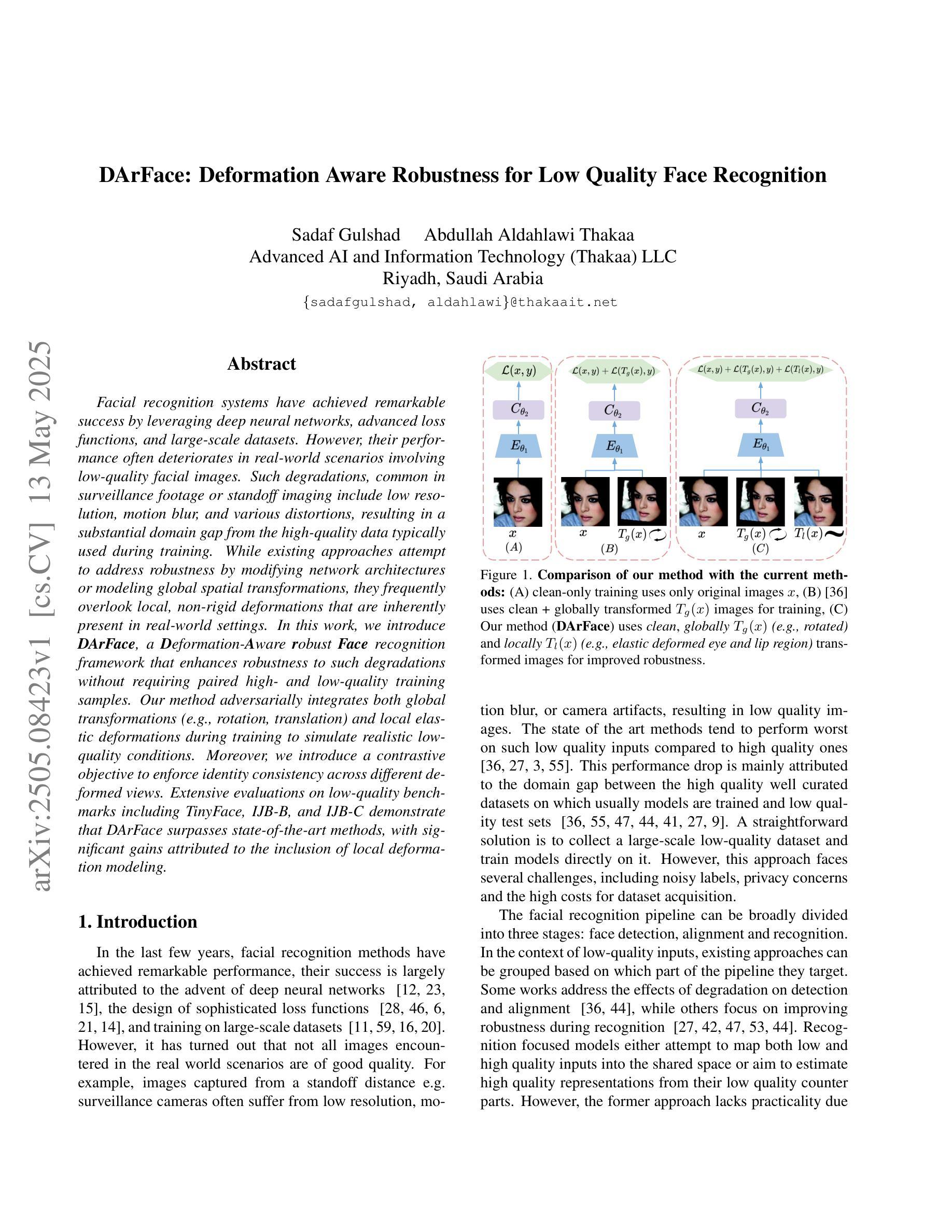
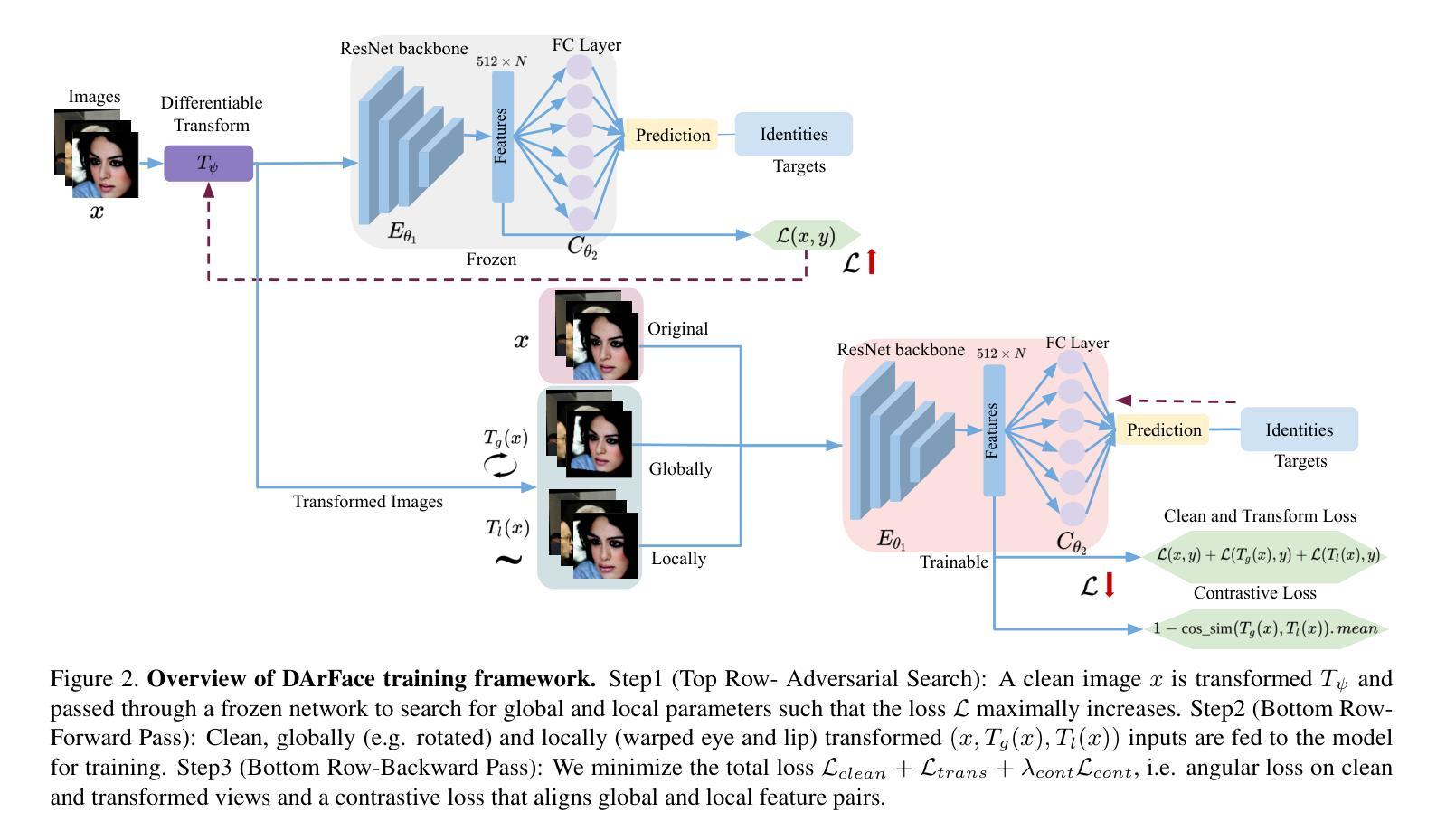
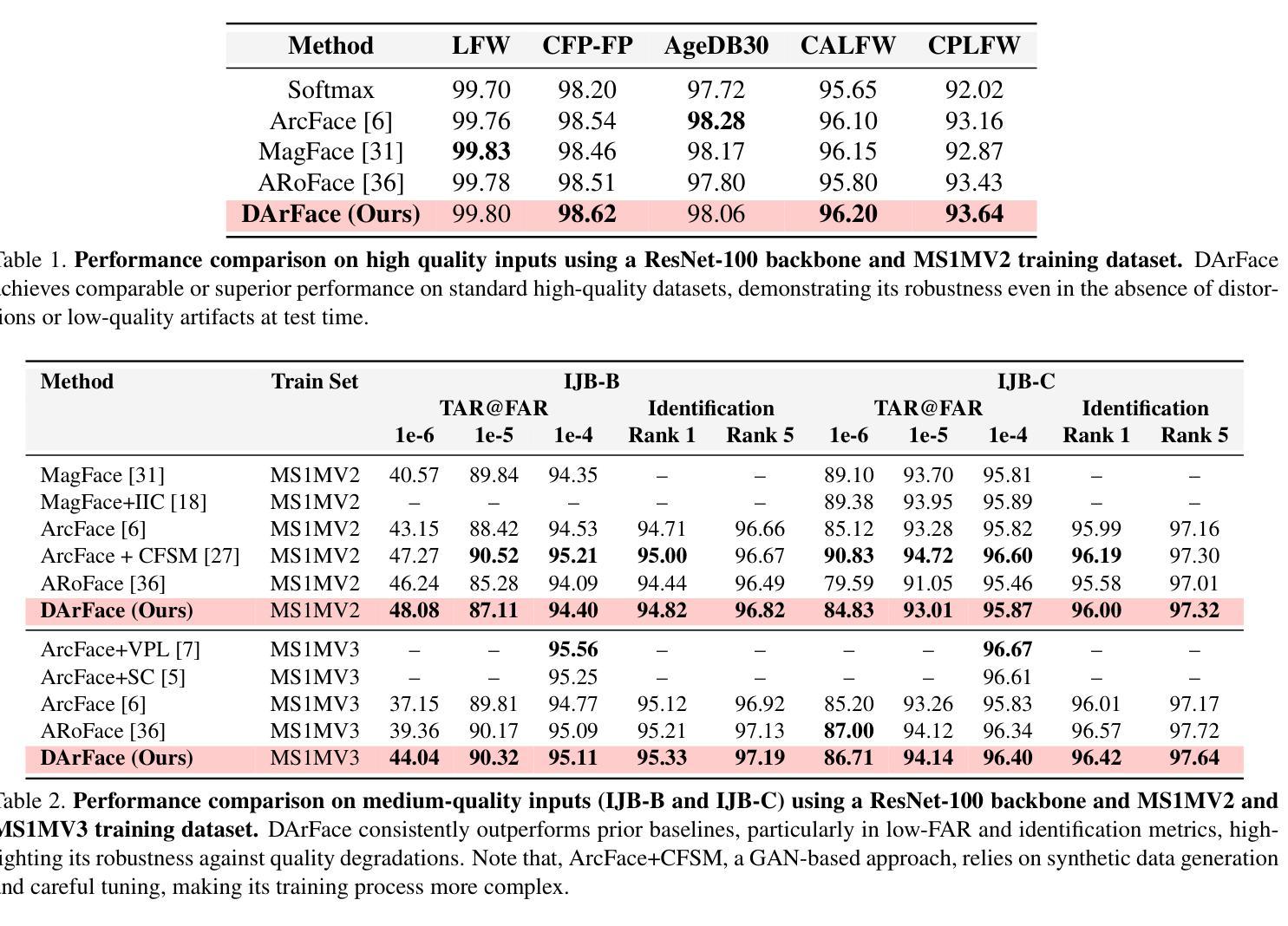