⚠️ 以下所有内容总结都来自于 大语言模型的能力,如有错误,仅供参考,谨慎使用
🔴 请注意:千万不要用于严肃的学术场景,只能用于论文阅读前的初筛!
💗 如果您觉得我们的项目对您有帮助 ChatPaperFree ,还请您给我们一些鼓励!⭐️ HuggingFace免费体验
2025-05-15 更新
Aya Vision: Advancing the Frontier of Multilingual Multimodality
Authors:Saurabh Dash, Yiyang Nan, John Dang, Arash Ahmadian, Shivalika Singh, Madeline Smith, Bharat Venkitesh, Vlad Shmyhlo, Viraat Aryabumi, Walter Beller-Morales, Jeremy Pekmez, Jason Ozuzu, Pierre Richemond, Acyr Locatelli, Nick Frosst, Phil Blunsom, Aidan Gomez, Ivan Zhang, Marzieh Fadaee, Manoj Govindassamy, Sudip Roy, Matthias Gallé, Beyza Ermis, Ahmet Üstün, Sara Hooker
Building multimodal language models is fundamentally challenging: it requires aligning vision and language modalities, curating high-quality instruction data, and avoiding the degradation of existing text-only capabilities once vision is introduced. These difficulties are further magnified in the multilingual setting, where the need for multimodal data in different languages exacerbates existing data scarcity, machine translation often distorts meaning, and catastrophic forgetting is more pronounced. To address the aforementioned challenges, we introduce novel techniques spanning both data and modeling. First, we develop a synthetic annotation framework that curates high-quality, diverse multilingual multimodal instruction data, enabling Aya Vision models to produce natural, human-preferred responses to multimodal inputs across many languages. Complementing this, we propose a cross-modal model merging technique that mitigates catastrophic forgetting, effectively preserving text-only capabilities while simultaneously enhancing multimodal generative performance. Aya-Vision-8B achieves best-in-class performance compared to strong multimodal models such as Qwen-2.5-VL-7B, Pixtral-12B, and even much larger Llama-3.2-90B-Vision. We further scale this approach with Aya-Vision-32B, which outperforms models more than twice its size, such as Molmo-72B and LLaMA-3.2-90B-Vision. Our work advances multilingual progress on the multi-modal frontier, and provides insights into techniques that effectively bend the need for compute while delivering extremely high performance.
构建多模态语言模型具有根本挑战性:它需要对视觉和语言模态进行对齐,筛选高质量指令数据,并在引入视觉信息时避免文本功能的退化。在多语种环境中,这些困难进一步放大。多模态数据在不同语言中的需求加剧了现有数据稀缺的问题,机器翻译经常扭曲词义,灾难性遗忘的问题更为突出。为了解决上述挑战,我们引入了涵盖数据和建模的新技术。首先,我们开发了一个合成注释框架,该框架能够筛选高质量、多样化的多语种多模态指令数据,使Aya Vision模型能够为多模态输入生成自然、人性化的跨语言响应。作为补充,我们提出了一种跨模态模型融合技术,可以缓解灾难性遗忘问题,在保留文本功能的同时,有效提高多模态生成性能。Aya-Vision-8B在同类中表现最佳,相较于强大的多模态模型如Qwen-2.5-VL-7B、Pixtral-12B甚至更大的Llama-3.2-90B-Vision都表现优秀。我们进一步扩展了这种方法,推出了Aya-Vision-32B,其性能超过了规模是其两倍的模型,如Molmo-72B和LLaMA-3.2-90B-Vision。我们的工作在多语种的多模态前沿取得了进展,并为有效利用计算资源同时实现极高性能的技术提供了见解。
论文及项目相关链接
Summary
本文介绍了构建多模态语言模型所面临的挑战,包括跨模态对齐、高质量指令数据的需求以及引入视觉后文本能力可能退化的问题。针对这些挑战,文章提出了新型技术和方法,包括合成注释框架和多模态模型融合技术,以改善多语言环境下的多模态数据稀缺和灾难性遗忘问题。文章还介绍了Aya Vision模型在多模态输入方面的表现,以及其相较于其他模型的性能优势。最后,文章展望了未来多模态语言模型的发展前景。
Key Takeaways
- 构建多模态语言模型面临跨模态对齐、高质量指令数据需求和文本能力退化等挑战。
- 在多语言环境下,需要更多的多模态数据,机器翻译可能导致意义扭曲,灾难性遗忘问题更加突出。
- 引入合成注释框架,为Aya Vision模型提供高质量、多样化的多语言多模态指令数据。
- 提出跨模态模型融合技术,有效缓解灾难性遗忘问题,同时保留文本能力并提升多模态生成性能。
- Aya-Vision-8B模型在性能上达到最佳水平,超过其他强大的多模态模型,如Qwen-2.5-VL-7B和Pixtral-12B。
- 通过进一步扩大规模,Aya-Vision-32B模型表现出超越其规模两倍的其他模型的性能,如Molmo-72B和LLaMA-3.2-90B-Vision。
点此查看论文截图
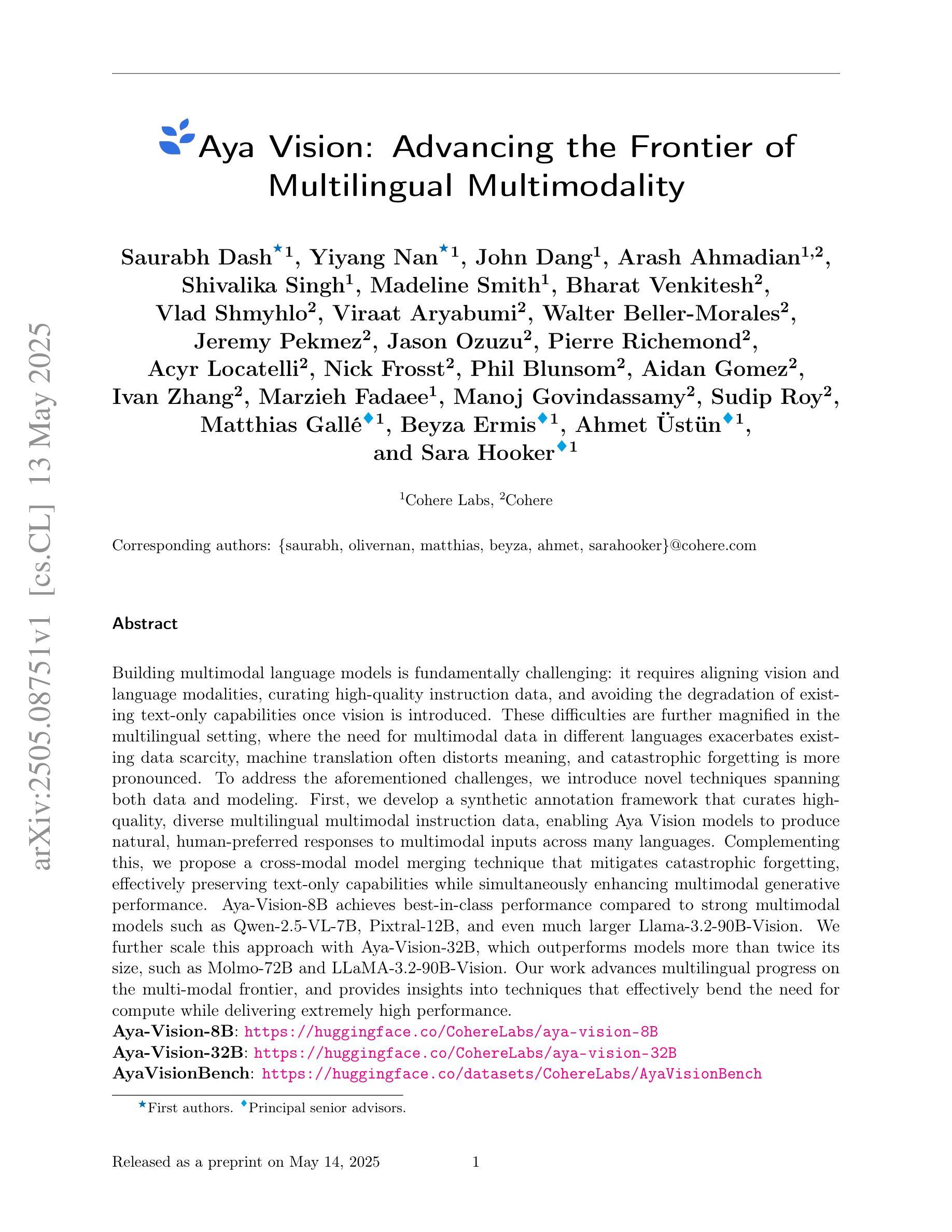
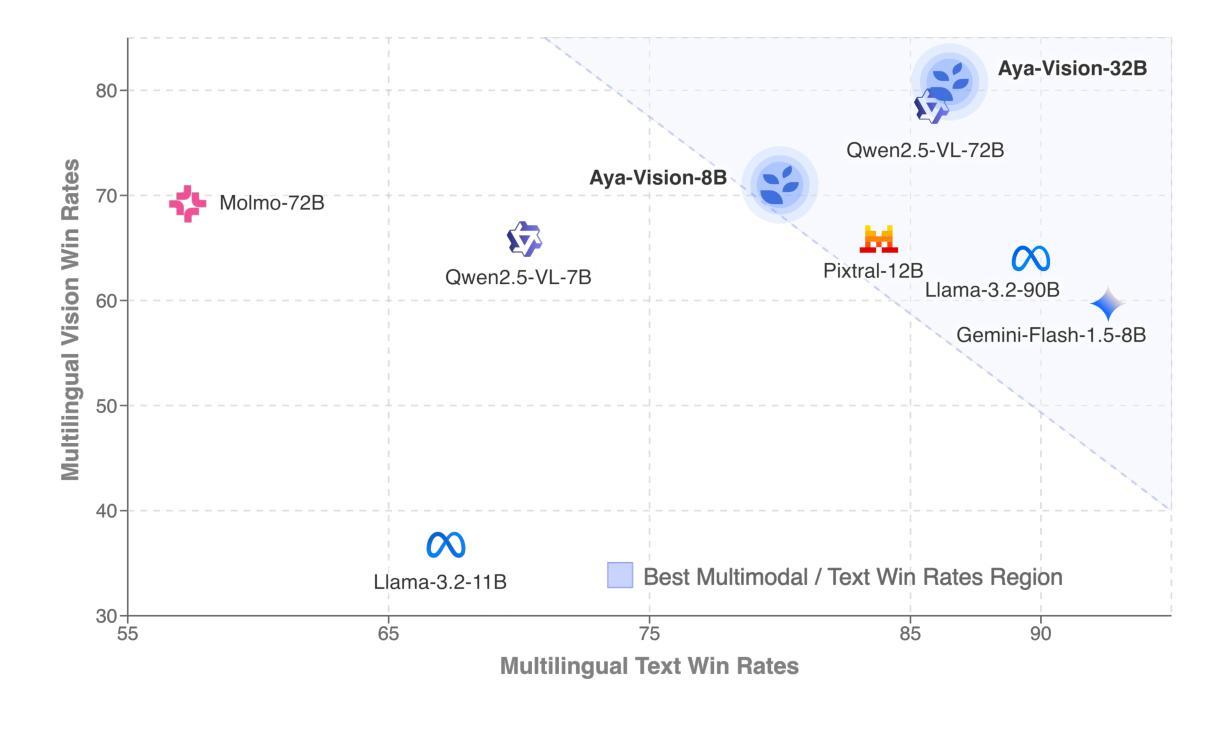
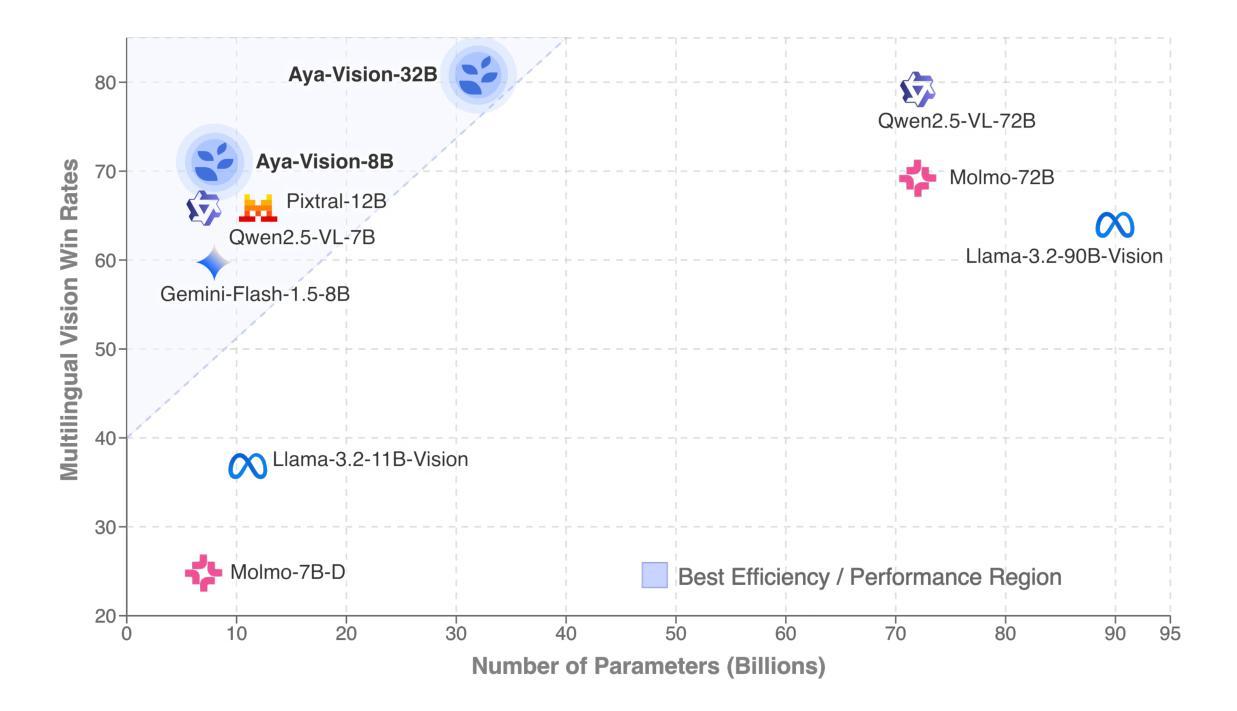
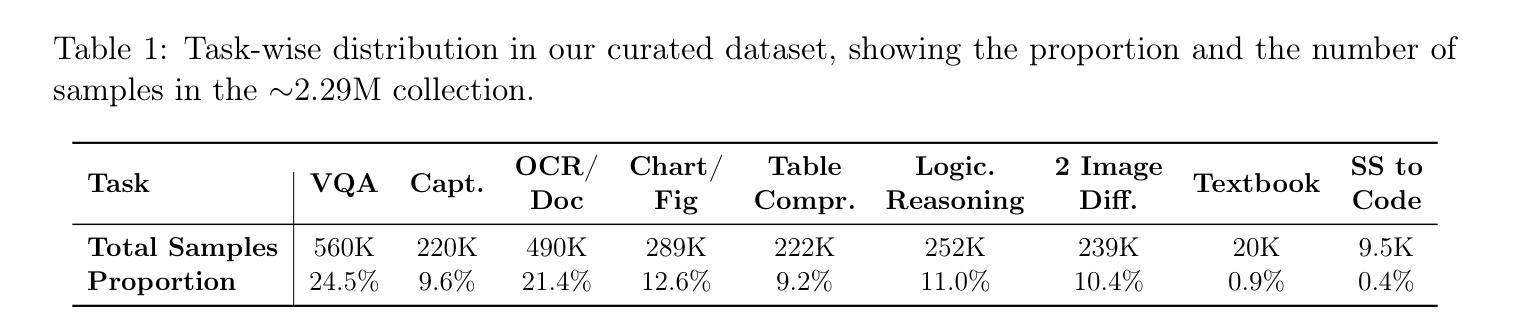
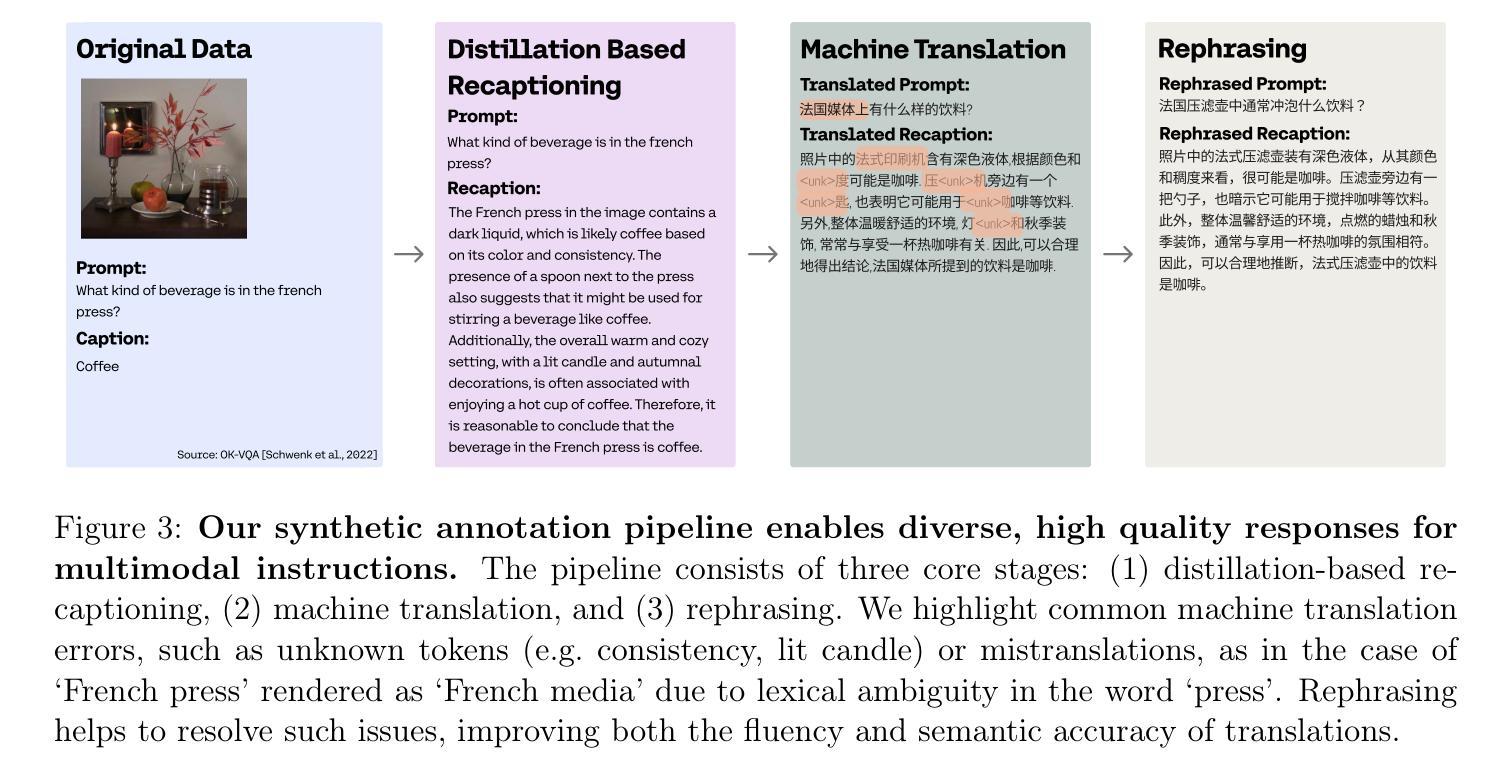