⚠️ 以下所有内容总结都来自于 大语言模型的能力,如有错误,仅供参考,谨慎使用
🔴 请注意:千万不要用于严肃的学术场景,只能用于论文阅读前的初筛!
💗 如果您觉得我们的项目对您有帮助 ChatPaperFree ,还请您给我们一些鼓励!⭐️ HuggingFace免费体验
2025-05-15 更新
TT-DF: A Large-Scale Diffusion-Based Dataset and Benchmark for Human Body Forgery Detection
Authors:Wenkui Yang, Zhida Zhang, Xiaoqiang Zhou, Junxian Duan, Jie Cao
The emergence and popularity of facial deepfake methods spur the vigorous development of deepfake datasets and facial forgery detection, which to some extent alleviates the security concerns about facial-related artificial intelligence technologies. However, when it comes to human body forgery, there has been a persistent lack of datasets and detection methods, due to the later inception and complexity of human body generation methods. To mitigate this issue, we introduce TikTok-DeepFake (TT-DF), a novel large-scale diffusion-based dataset containing 6,120 forged videos with 1,378,857 synthetic frames, specifically tailored for body forgery detection. TT-DF offers a wide variety of forgery methods, involving multiple advanced human image animation models utilized for manipulation, two generative configurations based on the disentanglement of identity and pose information, as well as different compressed versions. The aim is to simulate any potential unseen forged data in the wild as comprehensively as possible, and we also furnish a benchmark on TT-DF. Additionally, we propose an adapted body forgery detection model, Temporal Optical Flow Network (TOF-Net), which exploits the spatiotemporal inconsistencies and optical flow distribution differences between natural data and forged data. Our experiments demonstrate that TOF-Net achieves favorable performance on TT-DF, outperforming current state-of-the-art extendable facial forgery detection models. For our TT-DF dataset, please refer to https://github.com/HashTAG00002/TT-DF.
面部深度伪造方法的出现和流行刺激了深度伪造数据集和面部伪造检测技术的蓬勃发展,这在一定程度上减轻了与面部相关的人工智能技术的安全担忧。然而,当涉及到人体伪造时,由于人体生成方法的起步较迟和复杂性,数据集和检测方法的缺乏一直存在。为了缓解这个问题,我们推出了TikTok-DeepFake(TT-DF),这是一个新型的大规模扩散基础数据集,包含6,120个伪造视频和1,378,857个合成帧,专门用于人体伪造检测。TT-DF提供了多种伪造方法,涉及多种先进的人体图像动画模型用于操作,两种基于身份和姿势信息解耦的生成配置,以及不同的压缩版本。我们的目标是尽可能全面地模拟野外任何潜在未见过的伪造数据,我们还为TT-DF提供了一个基准。此外,我们提出了一种适应性的人体伪造检测模型——Temporal Optical Flow Network(TOF-Net),它利用自然数据和伪造数据之间的时空不一致性和光流分布差异。我们的实验表明,TOF-Net在TT-DF上表现良好,优于当前先进的可扩展面部伪造检测模型。有关我们的TT-DF数据集,请参阅https://github.com/HashTAG00002/TT-DF。
论文及项目相关链接
PDF Accepted to PRCV 2024
Summary
本文介绍了TikTok-DeepFake数据集的出现以解决人体伪造缺乏数据集和检测方法的难题。该数据集包含大量基于扩散技术生成的人体伪造视频,模拟了各种潜在的未知伪造数据。此外,还提出了一种适用于该数据集的临时光学流网络(TOF-Net)检测模型,取得了良好效果。如需访问TT-DF数据集,请访问指定链接。
Key Takeaways
- TikTok-DeepFake(TT-DF)数据集解决了人体伪造缺乏数据集的问题,包含大量基于扩散技术生成的人体伪造视频。
- TT-DF数据集模拟了各种潜在的未知伪造数据,旨在提高检测模型的泛化能力。
- TOF-Net是一种适用于TT-DF数据集的检测模型,能够利用时空不一致性和光学流分布差异来检测伪造数据。
- TOF-Net在TT-DF数据集上的表现优于当前先进的人脸伪造检测模型。
- TT-DF数据集具有广泛的应用前景,可应用于数字取证、视频编辑、安全防护等领域。
- TT-DF数据集可免费访问和使用,具体的访问链接已在本文中给出。
点此查看论文截图
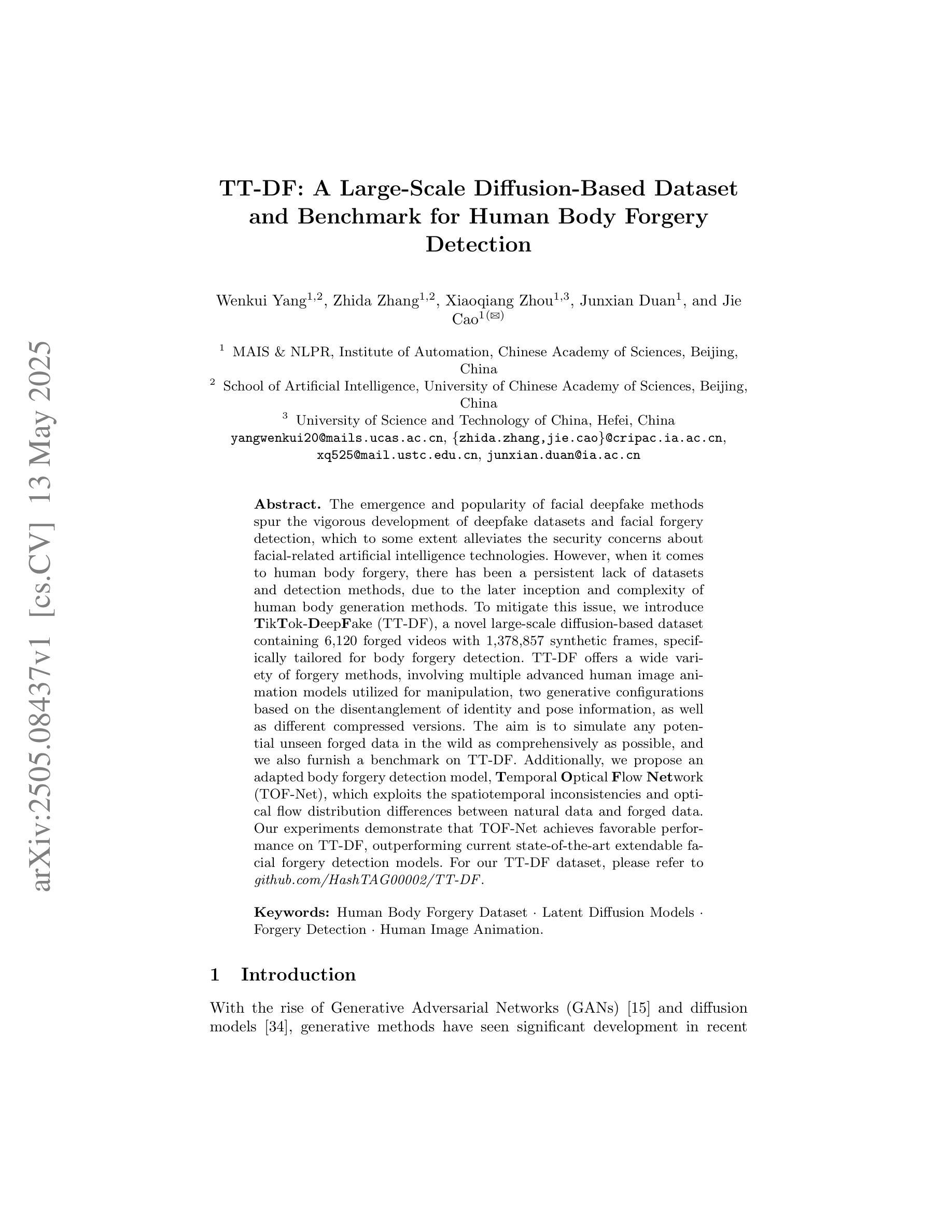
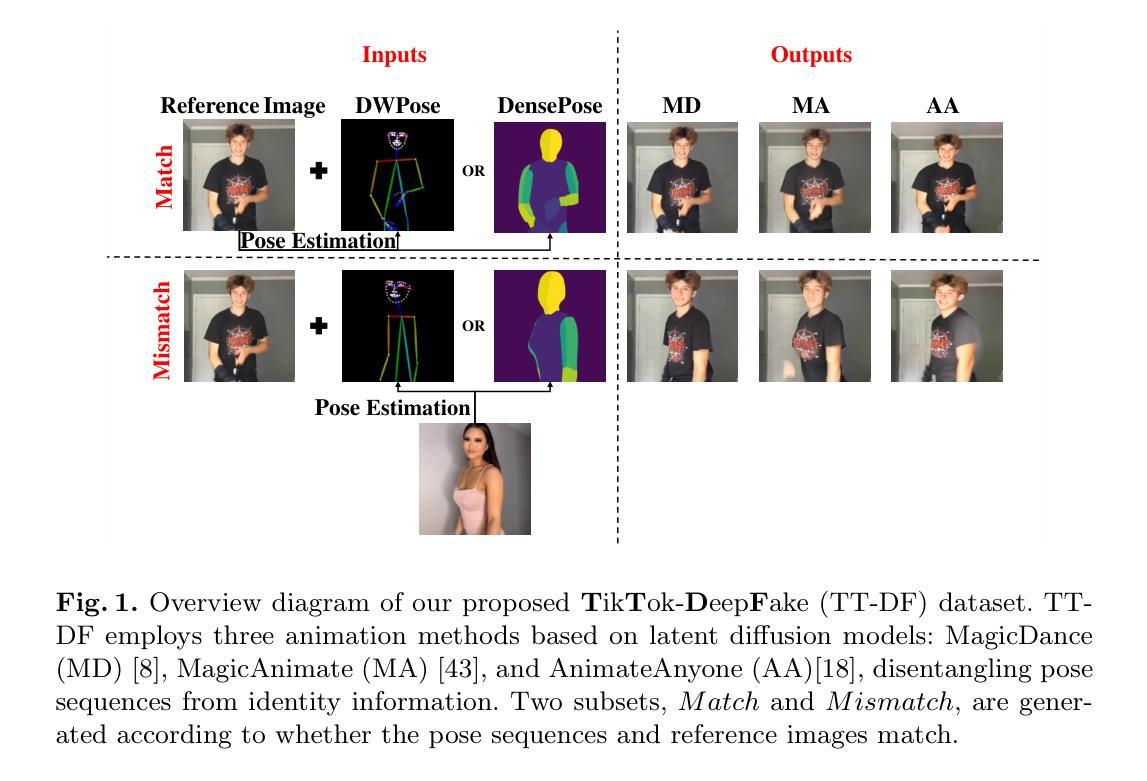
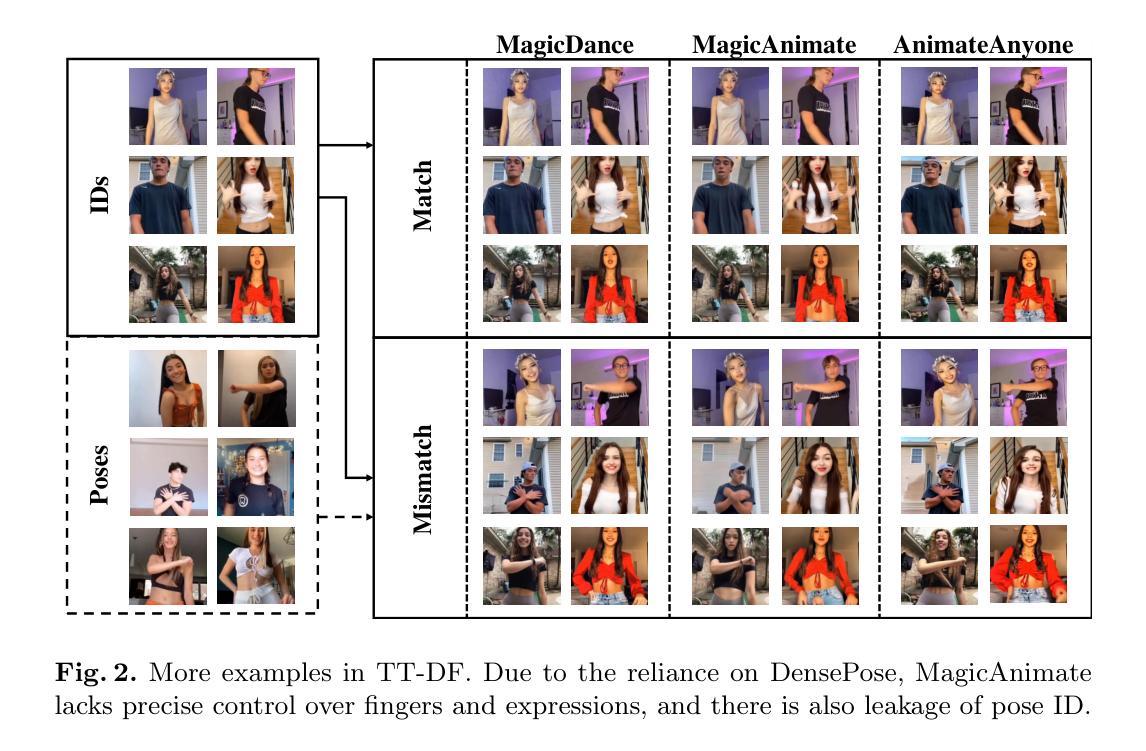
Investigating self-supervised features for expressive, multilingual voice conversion
Authors:Álvaro Martín-Cortinas, Daniel Sáez-Trigueros, Grzegorz Beringer, Iván Vallés-Pérez, Roberto Barra-Chicote, Biel Tura-Vecino, Adam Gabryś, Piotr Bilinski, Thomas Merritt, Jaime Lorenzo-Trueba
Voice conversion (VC) systems are widely used for several applications, from speaker anonymisation to personalised speech synthesis. Supervised approaches learn a mapping between different speakers using parallel data, which is expensive to produce. Unsupervised approaches are typically trained to reconstruct the input signal, which is composed of the content and the speaker information. Disentangling these components is a challenge and often leads to speaker leakage or prosodic information removal. In this paper, we explore voice conversion by leveraging the potential of self-supervised learning (SSL). A combination of the latent representations of SSL models, concatenated with speaker embeddings, is fed to a vocoder which is trained to reconstruct the input. Zero-shot voice conversion results show that this approach allows to keep the prosody and content of the source speaker while matching the speaker similarity of a VC system based on phonetic posteriorgrams (PPGs).
语音转换(VC)系统广泛应用于多个应用场景,从语音匿名化到个性化语音合成。有监督的方法使用平行数据学习不同说话者之间的映射,而平行数据的制作成本很高。无监督的方法通常被训练来重建输入信号,该信号由内容和说话者信息组成。解开这些组成部分是一个挑战,并且经常导致说话者泄露或韵律信息丢失。在本文中,我们通过对自监督学习(SSL)的潜力进行探索来实现语音转换。SSL模型的潜在表示与说话者嵌入相结合,输入到一个被训练用于重建输入的vocoder中。零样本语音转换结果表明,这种方法能够在保持源说话者的韵律和内容的同时,匹配基于语音后期语法(PPGs)的VC系统的说话者相似性。
论文及项目相关链接
PDF Published as a conference paper at ICASSP 2024
总结
本文探讨了基于自监督学习(SSL)的语音转换(VC)技术。文章指出,自监督学习模型能够结合潜表征和说话人嵌入信息,并训练vocoder进行输入重建。这种零样本语音转换方法能够在保持源说话人的语调和内容的同时,匹配基于音素后验概率图(PPGs)的VC系统的说话人相似性。
要点
- 语音转换(VC)系统广泛应用于多种应用,如说话人匿名化和个性化语音合成。
- 监督学习方法使用平行数据学习不同说话人之间的映射,但数据制作成本高昂。
- 无监督方法通常旨在重建输入信号,但面临将内容和说话人信息分解的挑战,可能导致说话人泄漏或语调信息移除。
- 本文利用自监督学习(SSL)的潜力进行语音转换。
- SSL模型的潜表征与说话人嵌入相结合,输入给受过训练的vocoder进行输入重建。
- 零样本语音转换结果能够在保持源说话人的语调和内容的同时,匹配基于音素后验图的VC系统的说话人相似性。
- 这种方法为提高语音转换技术的性能提供了新的思路。
点此查看论文截图
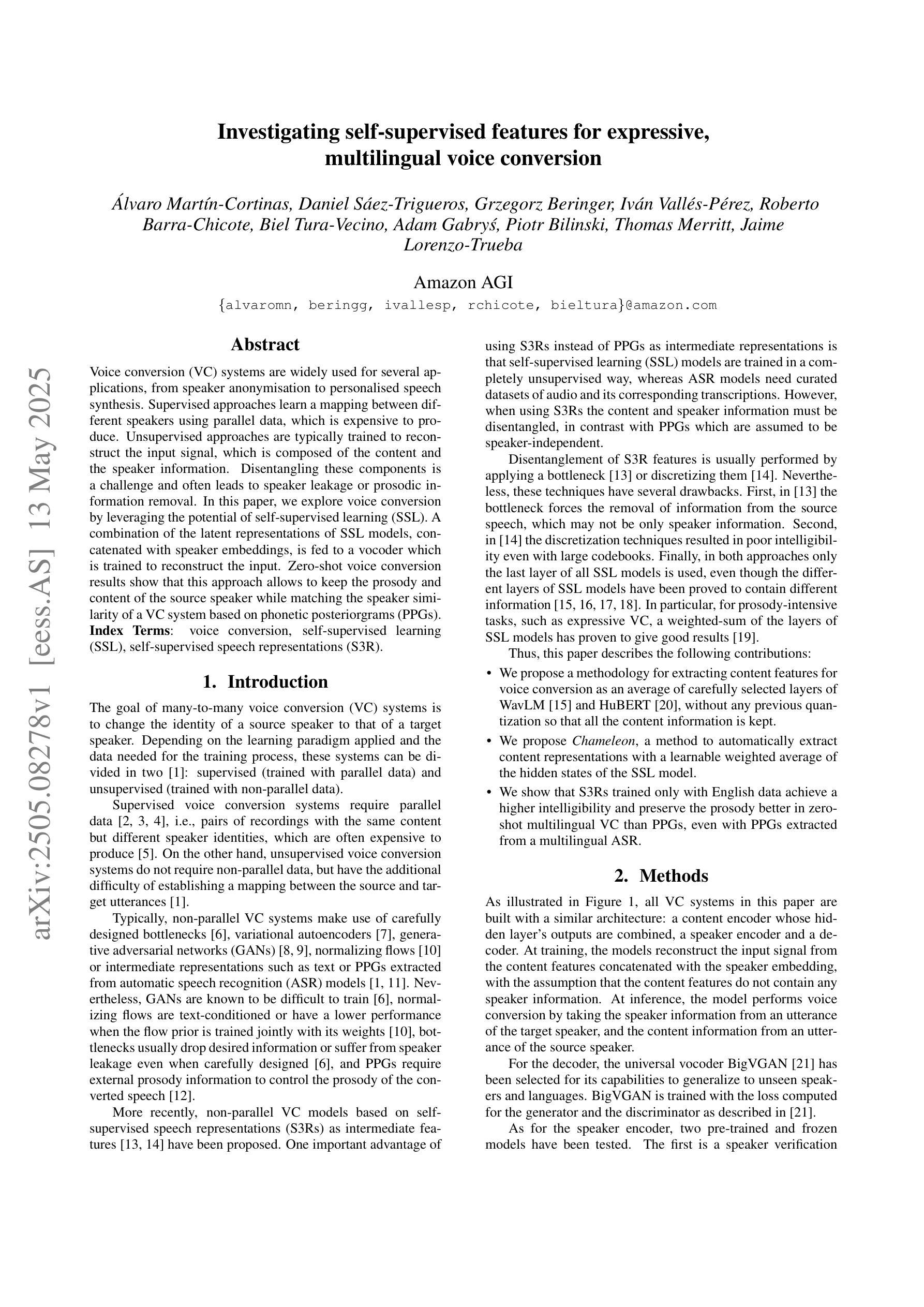
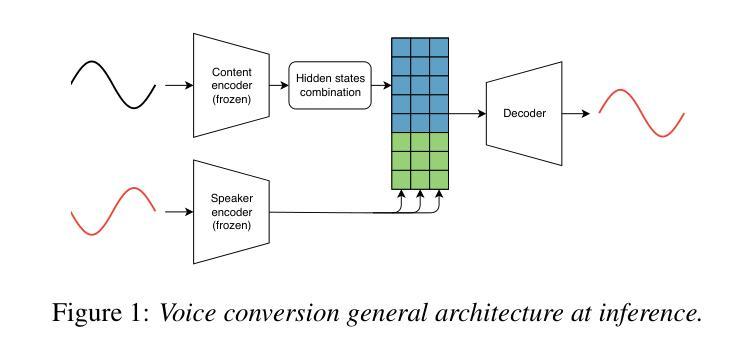
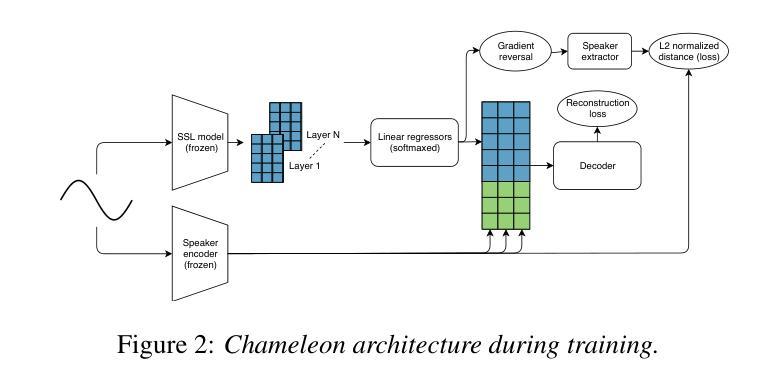
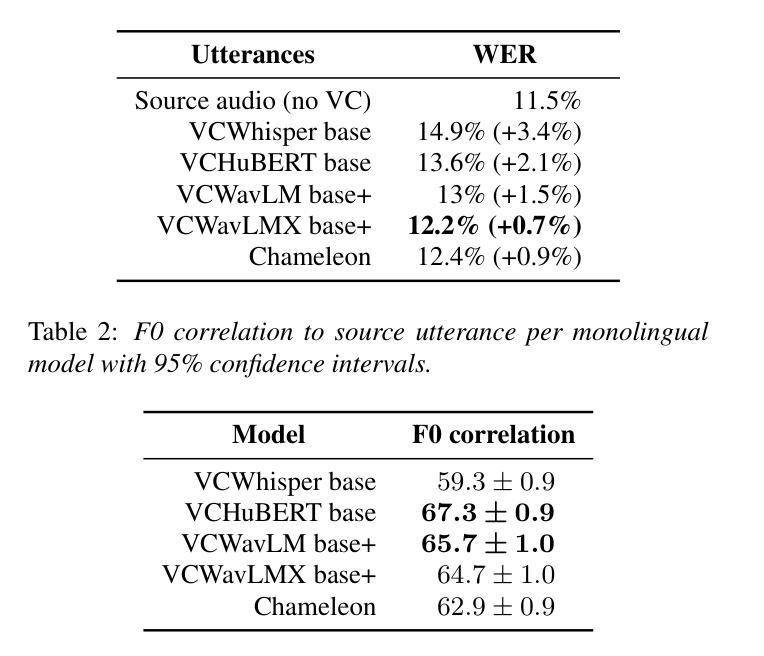
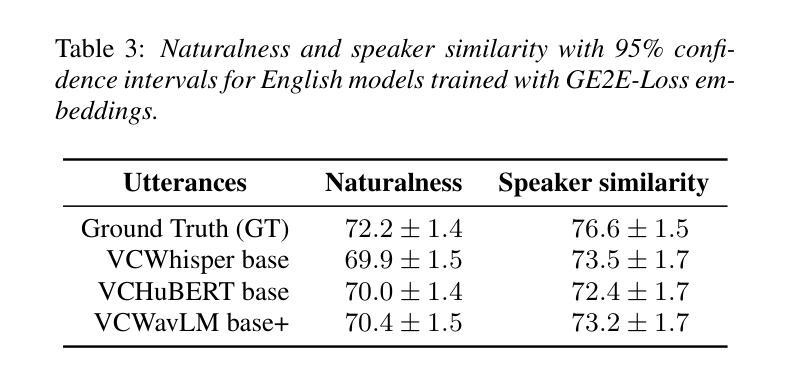
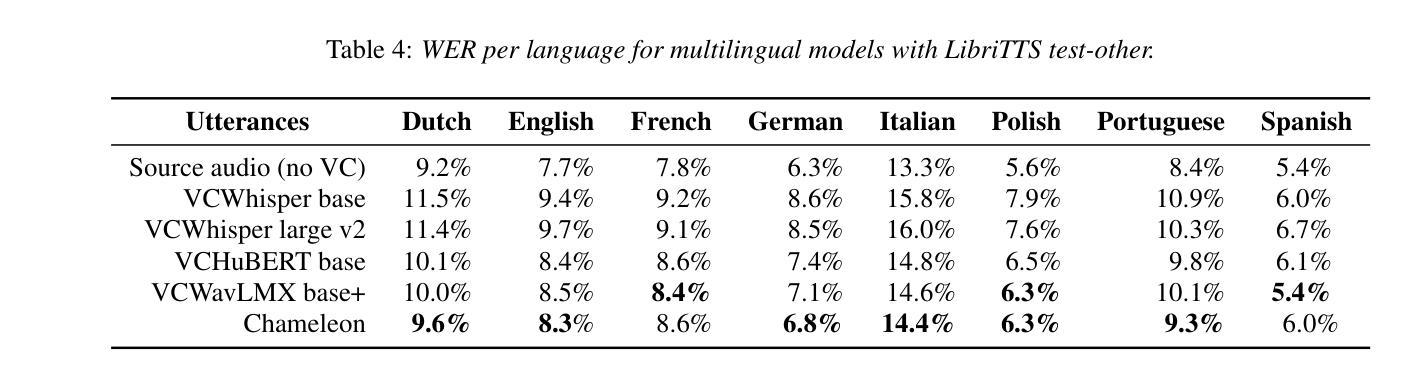
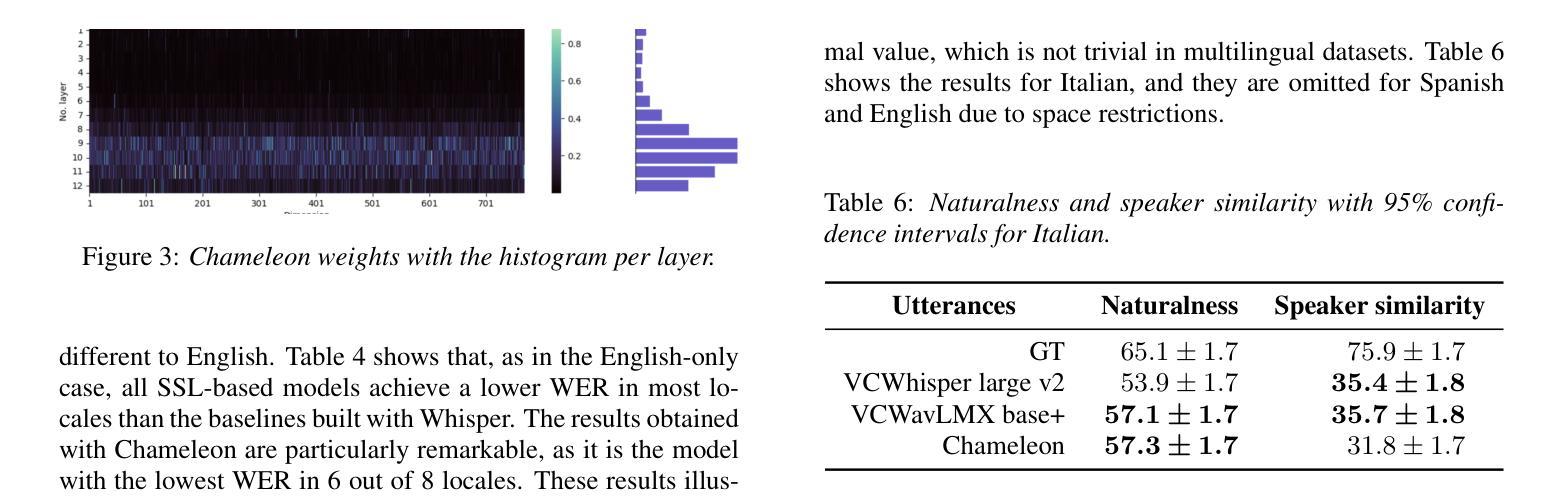
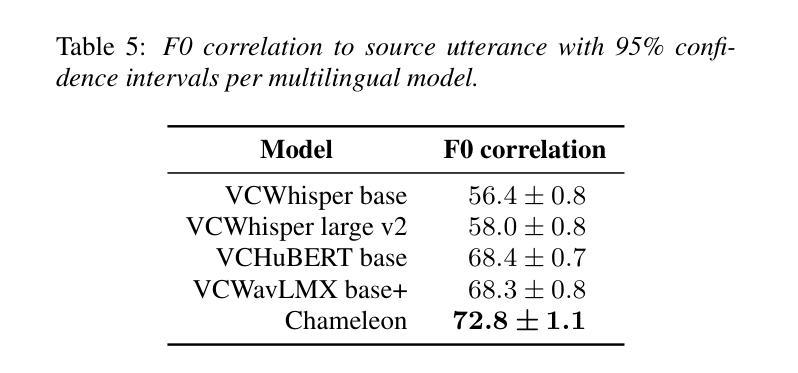
MiniMax-Speech: Intrinsic Zero-Shot Text-to-Speech with a Learnable Speaker Encoder
Authors:Bowen Zhang, Congchao Guo, Geng Yang, Hang Yu, Haozhe Zhang, Heidi Lei, Jialong Mai, Junjie Yan, Kaiyue Yang, Mingqi Yang, Peikai Huang, Ruiyang Jin, Sitan Jiang, Weihua Cheng, Yawei Li, Yichen Xiao, Yiying Zhou, Yongmao Zhang, Yuan Lu, Yucen He
We introduce MiniMax-Speech, an autoregressive Transformer-based Text-to-Speech (TTS) model that generates high-quality speech. A key innovation is our learnable speaker encoder, which extracts timbre features from a reference audio without requiring its transcription. This enables MiniMax-Speech to produce highly expressive speech with timbre consistent with the reference in a zero-shot manner, while also supporting one-shot voice cloning with exceptionally high similarity to the reference voice. In addition, the overall quality of the synthesized audio is enhanced through the proposed Flow-VAE. Our model supports 32 languages and demonstrates excellent performance across multiple objective and subjective evaluations metrics. Notably, it achieves state-of-the-art (SOTA) results on objective voice cloning metrics (Word Error Rate and Speaker Similarity) and has secured the top position on the public TTS Arena leaderboard. Another key strength of MiniMax-Speech, granted by the robust and disentangled representations from the speaker encoder, is its extensibility without modifying the base model, enabling various applications such as: arbitrary voice emotion control via LoRA; text to voice (T2V) by synthesizing timbre features directly from text description; and professional voice cloning (PVC) by fine-tuning timbre features with additional data. We encourage readers to visit https://minimax-ai.github.io/tts_tech_report for more examples.
我们介绍了MiniMax-Speech,这是一款基于自回归Transformer的文本到语音(TTS)模型,能够生成高质量语音。我们的主要创新之处在于可学习的说话人编码器,它可以从参考音频中提取音色特征,而无需其转录。这使得MiniMax-Speech能够以零样本的方式产生与参考音色一致的富有表现力的语音,同时支持单次语音克隆,与参考音色的相似度极高。此外,通过提出的Flow-VAE,合成音频的整体质量得到了提高。我们的模型支持32种语言,并在多个客观和主观评估指标上表现出卓越的性能。值得注意的是,它在客观语音克隆指标(词错误率和说话人相似性)上达到了最新水平,并在公共TTS Arena排行榜上获得了第一名。MiniMax-Speech的另一个关键优势在于其强大的说话人编码器提供的稳健和分离的表示形式,这使得其在不修改基础模型的情况下具有可扩展性,能够支持各种应用,例如通过LoRA实现任意语音情绪控制;通过直接从文本描述中合成音色特征来实现文本到语音(T2V);以及通过使用额外数据进行音色特征的微调来实现专业语音克隆(PVC)。更多示例请访问:https://minimax-ai.github.io/tts_tech_report。
论文及项目相关链接
摘要
本文介绍了基于Transformer的自回归文本到语音(TTS)模型——MiniMax-Speech。该模型能够生成高质量语音,主要创新点在于可学习的说话人编码器,能够从参考音频中提取音色特征而无需其转录。这使得MiniMax-Speech能够在零样本的情况下,产生与参考音色一致的具有高度表现力的语音,同时支持单样本声音克隆,与参考声音的相似度极高。此外,通过提出的Flow-VAE,增强了合成音频的整体质量。该模型支持32种语言,并在多个客观和主观评估指标上表现出卓越性能。在客观声音克隆指标(词错误率和说话人相似性)上实现了最新结果,并在公共TTS Arena排行榜上获得了第一名。MiniMax-Speech的另一关键优势在于其强大的说话人编码器提供的稳健和分离的表示形式,这使其无需修改基础模型即可扩展,支持多种应用,如通过LoRA实现任意语音情感控制;通过文本描述直接合成音色特征的文本到语音(T2V);以及通过额外数据微调音色特征的专业语音克隆(PVC)。更多示例可访问https://minimax-ai.github.io/tts_tech_report。
关键见解
- MiniMax-Speech是一个基于Transformer的自回归TTS模型,生成高质量语音。
- 模型的创新之处在于可学习的说话人编码器,可从参考音频提取音色特征。
- MiniMax-Speech支持零样本高度表现力的语音生成和单样本声音克隆。
- 通过Flow-VAE增强了合成音频质量。
- 模型支持32种语言,并在多项评估指标上表现优异。
- 在客观声音克隆指标上达到最新结果,并在TTS Arena排行榜上名列前茅。
- MiniMax-Speech具有多种应用,包括任意语音情感控制、文本到语音、以及专业语音克隆。
点此查看论文截图
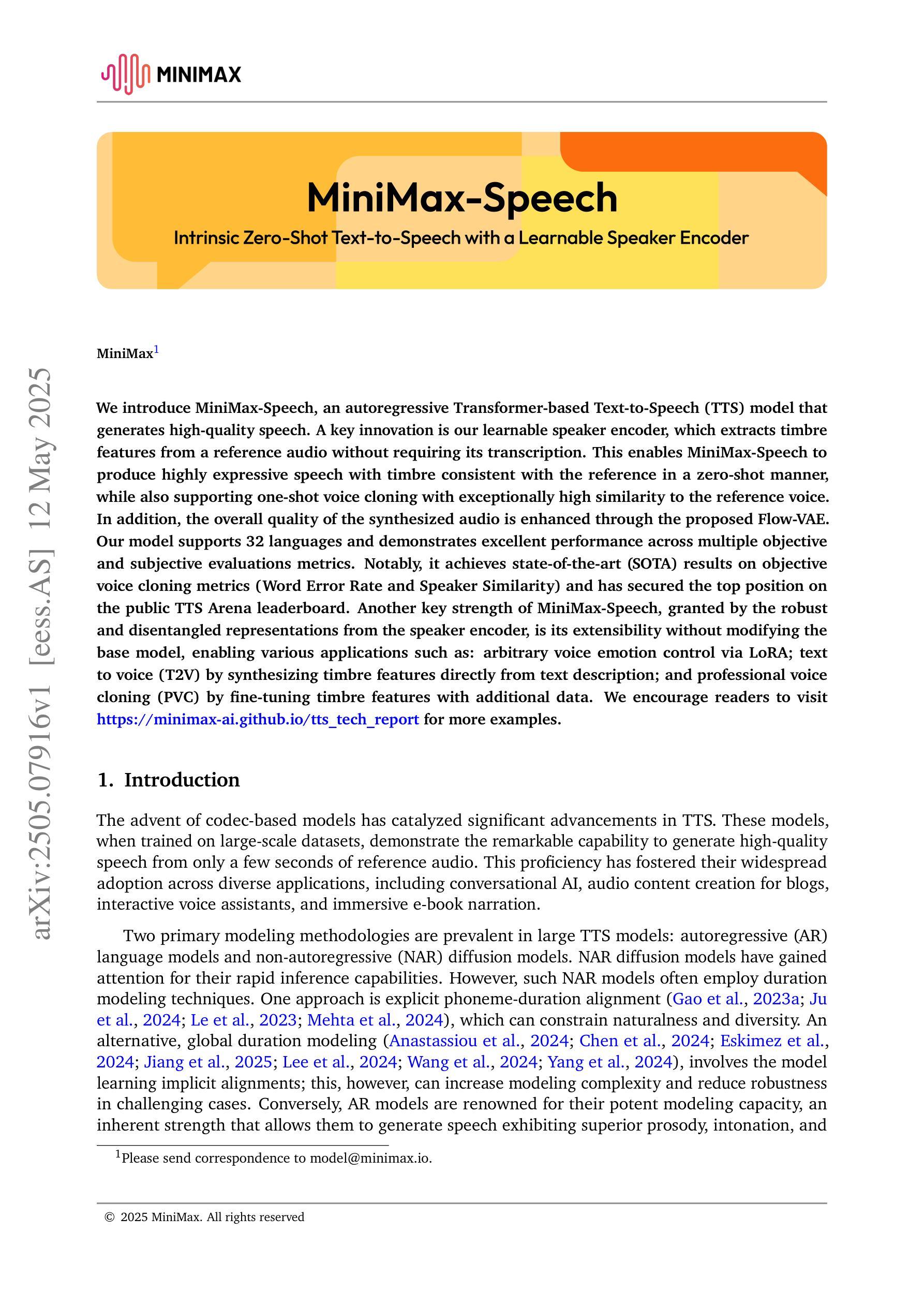
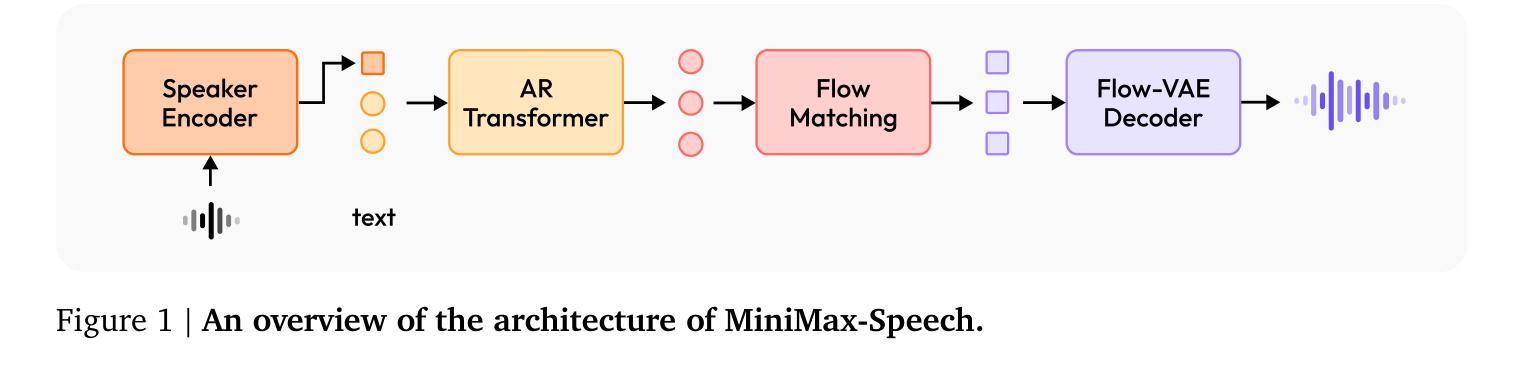
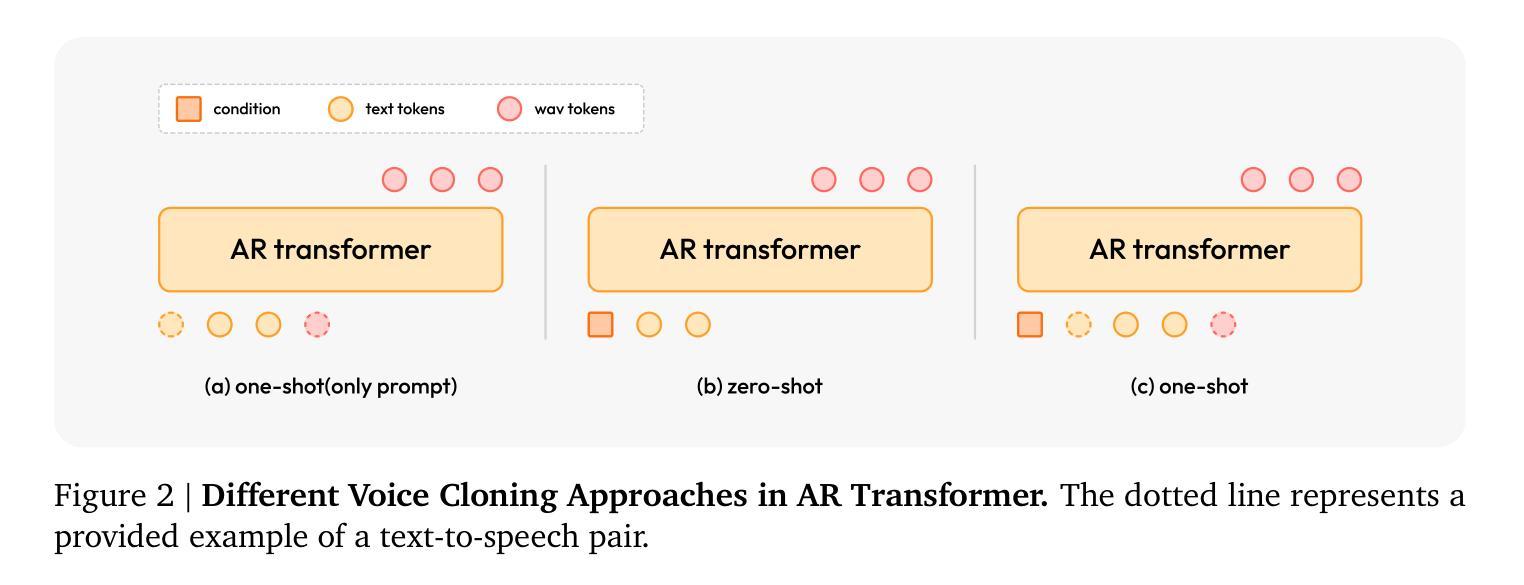
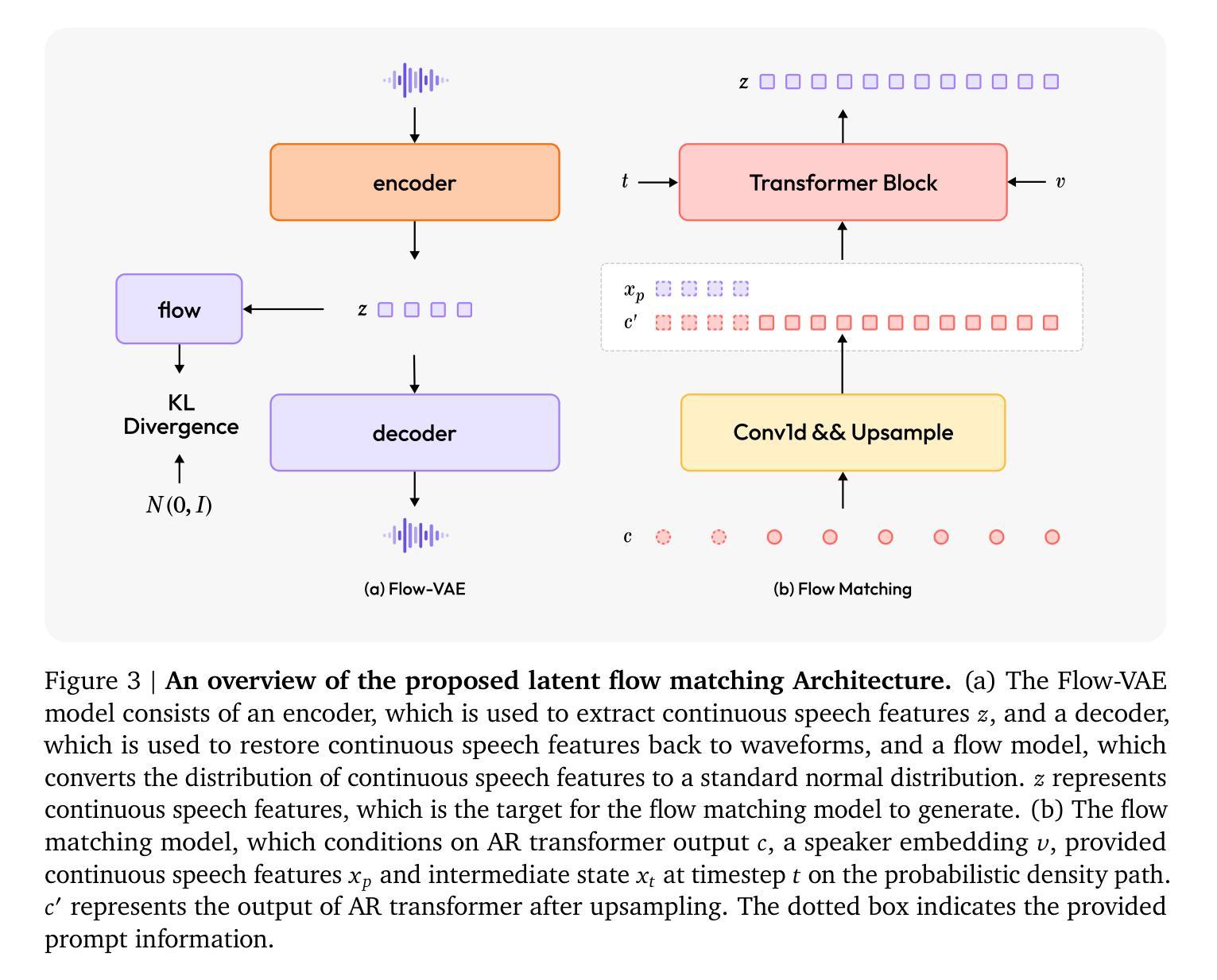