⚠️ 以下所有内容总结都来自于 大语言模型的能力,如有错误,仅供参考,谨慎使用
🔴 请注意:千万不要用于严肃的学术场景,只能用于论文阅读前的初筛!
💗 如果您觉得我们的项目对您有帮助 ChatPaperFree ,还请您给我们一些鼓励!⭐️ HuggingFace免费体验
2025-05-15 更新
M3G: Multi-Granular Gesture Generator for Audio-Driven Full-Body Human Motion Synthesis
Authors:Zhizhuo Yin, Yuk Hang Tsui, Pan Hui
Generating full-body human gestures encompassing face, body, hands, and global movements from audio is a valuable yet challenging task in virtual avatar creation. Previous systems focused on tokenizing the human gestures framewisely and predicting the tokens of each frame from the input audio. However, one observation is that the number of frames required for a complete expressive human gesture, defined as granularity, varies among different human gesture patterns. Existing systems fail to model these gesture patterns due to the fixed granularity of their gesture tokens. To solve this problem, we propose a novel framework named Multi-Granular Gesture Generator (M3G) for audio-driven holistic gesture generation. In M3G, we propose a novel Multi-Granular VQ-VAE (MGVQ-VAE) to tokenize motion patterns and reconstruct motion sequences from different temporal granularities. Subsequently, we proposed a multi-granular token predictor that extracts multi-granular information from audio and predicts the corresponding motion tokens. Then M3G reconstructs the human gestures from the predicted tokens using the MGVQ-VAE. Both objective and subjective experiments demonstrate that our proposed M3G framework outperforms the state-of-the-art methods in terms of generating natural and expressive full-body human gestures.
从音频生成包含面部、身体、手和全身动作的全套人体动作是一项在虚拟化身创建中非常有价值但又具有挑战性的任务。之前的系统侧重于逐帧标记人体动作,并从输入音频预测每帧的标记。然而,观察到不同人体动作模式的完整表达性动作所需的帧数(定义为粒度)是不同的。由于动作标记的固定粒度,现有系统无法对这些动作模式进行建模。为了解决这个问题,我们提出了一种名为Multi-Granular Gesture Generator(M3G)的音频驱动整体动作生成新型框架。在M3G中,我们提出了一种新型的多粒度VQ-VAE(MGVQ-VAE),用于标记动作模式并从不同的时间粒度重建动作序列。随后,我们提出了多粒度标记预测器,它从音频中提取多粒度信息并预测相应的动作标记。然后M3G使用MGVQ-VAE从预测的标记重建人体动作。客观和主观实验都表明,我们提出的M3G框架在生成自然和富有表现力的全身人体动作方面优于最新技术的方法。
论文及项目相关链接
PDF 9 Pages, 4 figures, submitted to NIPS 2025
Summary
本文探讨了音频驱动的全局动作生成问题,指出传统系统在固定粒度建模上的不足。为此,提出了一种名为Multi-Granular Gesture Generator(M3G)的新型框架,使用Multi-Granular VQ-VAE进行动作模式的令牌化和重建,并通过多粒度令牌预测器从音频中提取信息预测动作令牌。实验证明,M3G框架在生成自然、夸张的全身动作方面优于现有技术。
Key Takeaways
- 生成包含面部、身体、手和全局动作的全身人类动作是一个有价值但具有挑战性的任务。
- 现有系统主要基于固定粒度的动作令牌进行建模,无法适应不同动作模式所需的不同粒度。
- 提出了Multi-Granular Gesture Generator(M3G)框架,解决了上述问题。
- M3G使用Multi-Granular VQ-VAE进行动作模式的令牌化和重建。
- M3G通过多粒度令牌预测器从音频中提取信息并预测动作令牌。
- 实验证明M3G在生成自然、夸张的动作方面优于现有技术。
点此查看论文截图
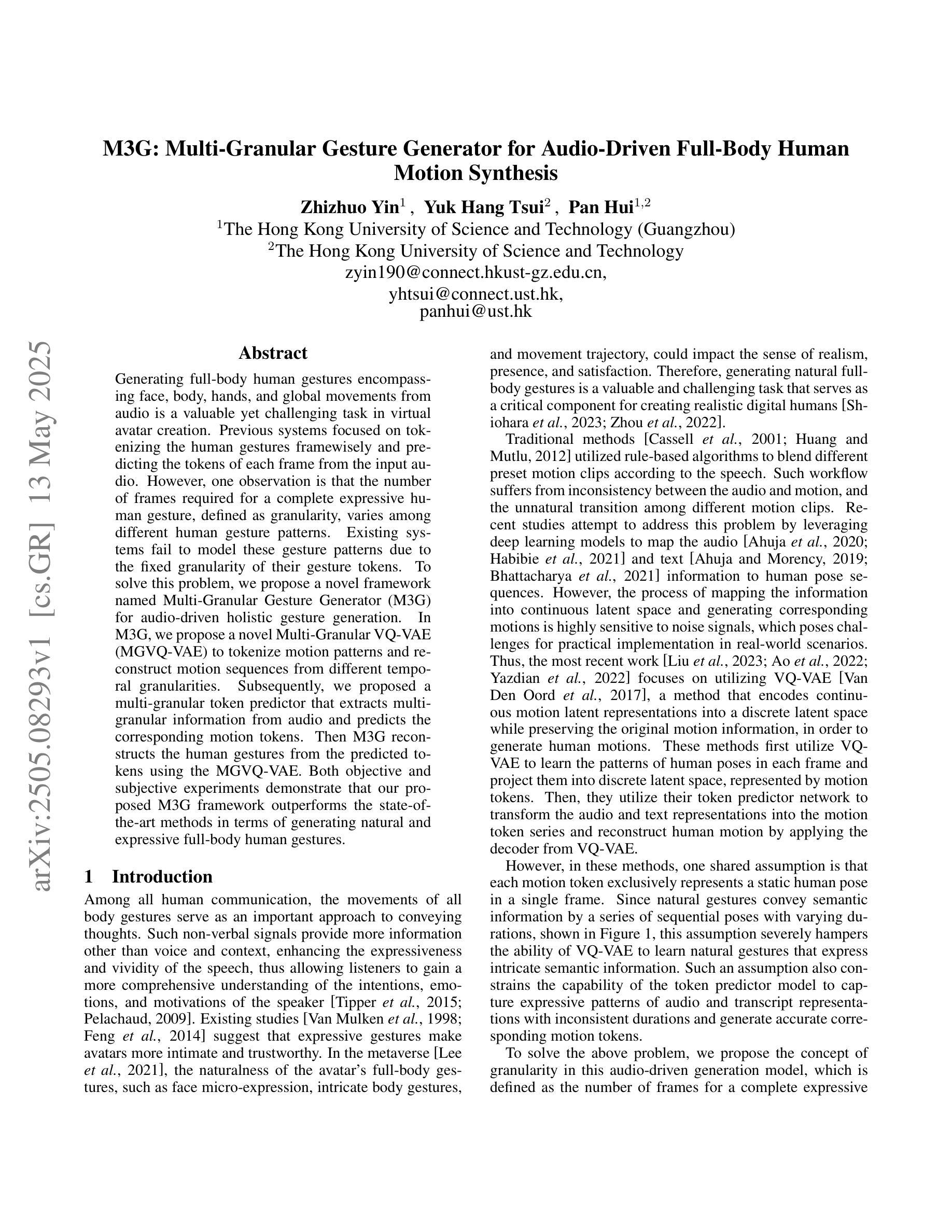
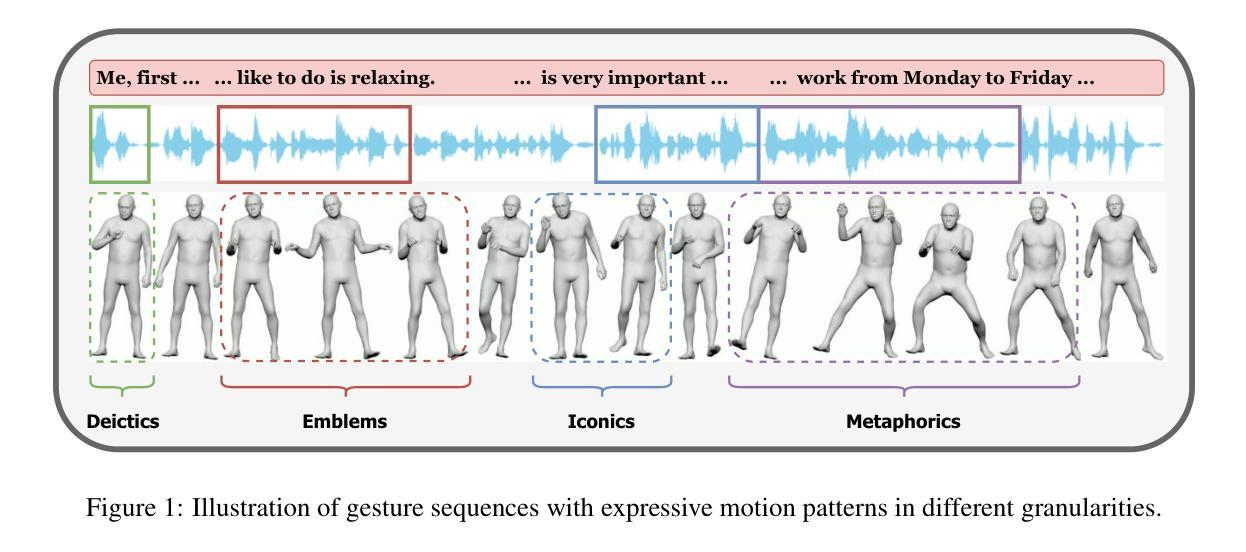
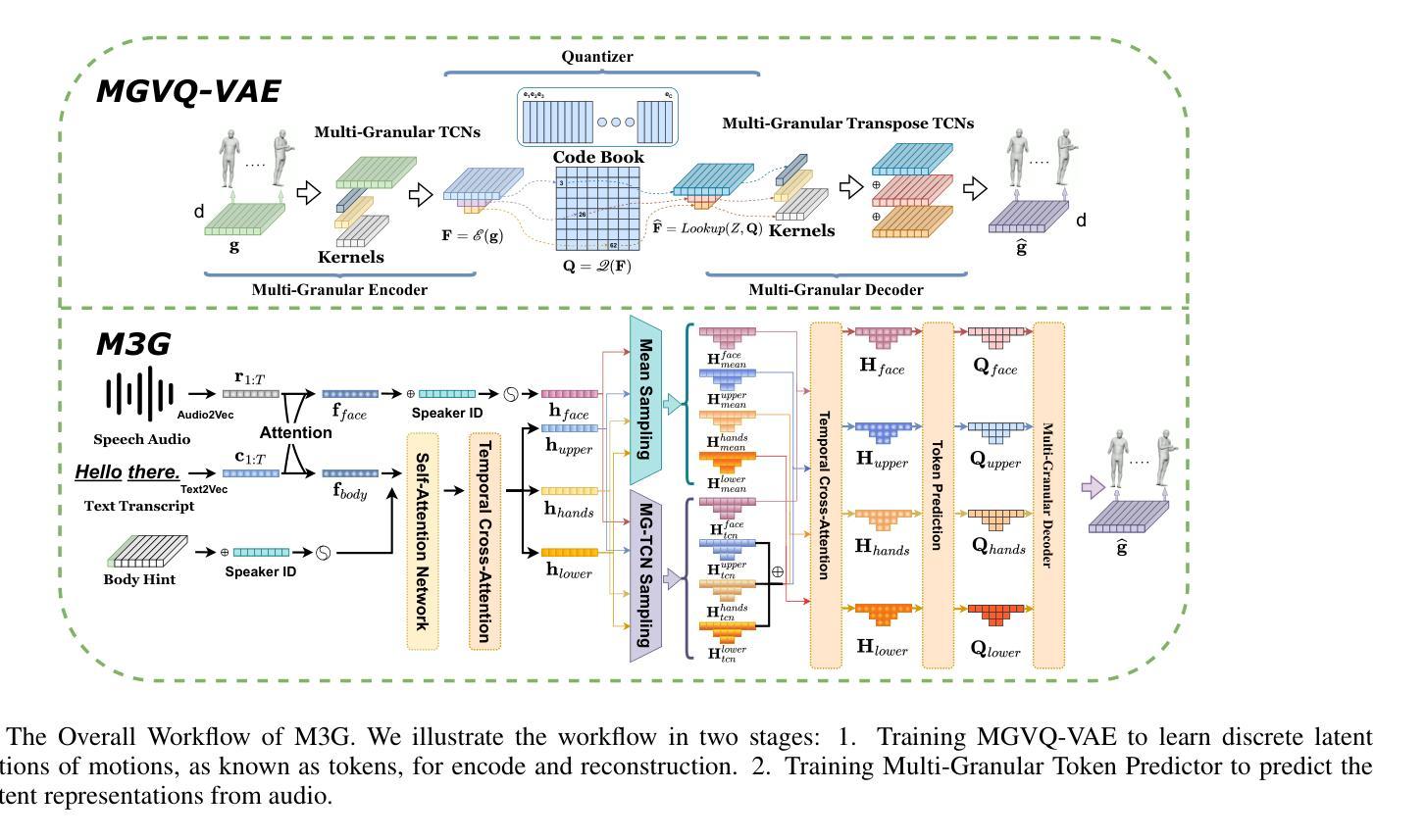
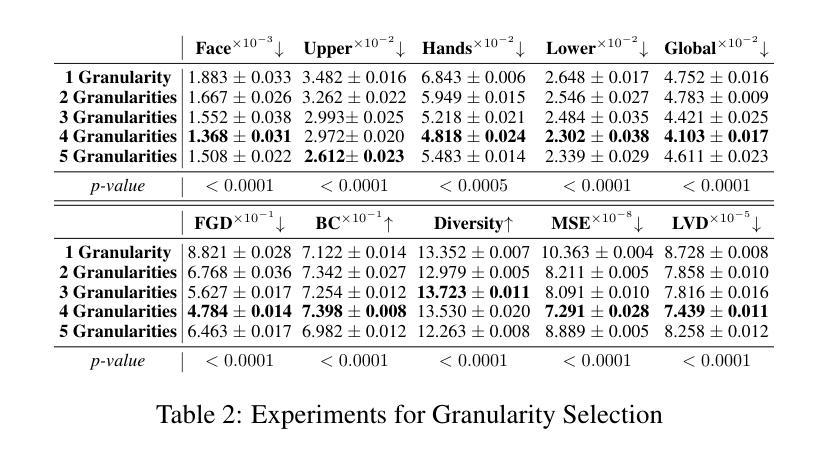
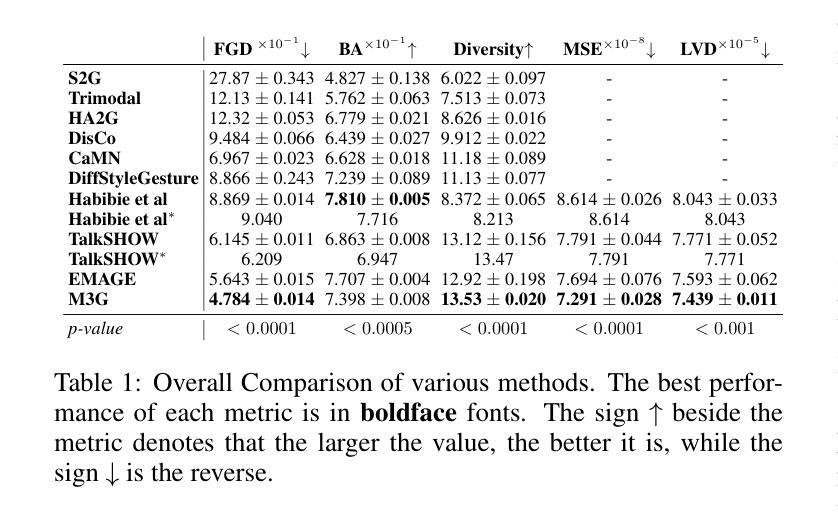