⚠️ 以下所有内容总结都来自于 大语言模型的能力,如有错误,仅供参考,谨慎使用
🔴 请注意:千万不要用于严肃的学术场景,只能用于论文阅读前的初筛!
💗 如果您觉得我们的项目对您有帮助 ChatPaperFree ,还请您给我们一些鼓励!⭐️ HuggingFace免费体验
2025-05-16 更新
Spec2VolCAMU-Net: A Spectrogram-to-Volume Model for EEG-to-fMRI Reconstruction based on Multi-directional Time-Frequency Convolutional Attention Encoder and Vision-Mamba U-Net
Authors:Dongyi He, Shiyang Li, Bin Jiang, He Yan
High-resolution functional magnetic resonance imaging (fMRI) is essential for mapping human brain activity; however, it remains costly and logistically challenging. If comparable volumes could be generated directly from widely available scalp electroencephalography (EEG), advanced neuroimaging would become significantly more accessible. Existing EEG-to-fMRI generators rely on plain CNNs that fail to capture cross-channel time-frequency cues or on heavy transformer/GAN decoders that strain memory and stability. We propose Spec2VolCAMU-Net, a lightweight spectrogram-to-volume generator that confronts these issues via a Multi-directional Time-Frequency Convolutional Attention Encoder, stacking temporal, spectral and joint convolutions with self-attention, and a Vision-Mamba U-Net decoder whose linear-time state-space blocks enable efficient long-range spatial modelling. Trained end-to-end with a hybrid SSI-MSE loss, Spec2VolCAMU-Net achieves state-of-the-art fidelity on three public benchmarks, recording SSIMs of 0.693 on NODDI, 0.725 on Oddball and 0.788 on CN-EPFL, representing improvements of 14.5%, 14.9%, and 16.9% respectively over previous best SSIM scores. Furthermore, it achieves competitive PSNR scores, particularly excelling on the CN-EPFL dataset with a 4.6% improvement over the previous best PSNR, thus striking a better balance in reconstruction quality. The proposed model is lightweight and efficient, making it suitable for real-time applications in clinical and research settings. The code is available at https://github.com/hdy6438/Spec2VolCAMU-Net.
高清晰度功能磁共振成像(fMRI)对于绘制人类大脑活动图至关重要,然而,它仍然成本高昂且后勤挑战重重。如果能够从广泛使用的头皮脑电图(EEG)直接生成相当数量的数据,那么先进的神经成像将变得更加易于获取。现有的EEG-to-fMRI生成器依赖于普通的CNN,无法捕捉跨通道的时空频率线索,或者依赖于沉重的transformer/GAN解码器,这会给内存和稳定性带来压力。我们提出了Spec2VolCAMU-Net,这是一种轻量级的频谱图生成器,通过多方向时空频率卷积注意力编码器来解决这些问题,该编码器堆叠了时间、频谱和联合卷积与自注意力机制,以及Vision-Mamba U-Net解码器,其线性时间状态空间块可实现高效的长程空间建模。使用混合SSI-MSE损失进行端到端训练,Spec2VolCAMU-Net在三个公共基准测试上达到了最先进的保真度,在NODDI上的SSIM为0.693,Oddball上的SSIM为0.725,CN-EPFL上的SSIM为0.788,分别比之前的最佳SSIM得分提高了14.5%、14.9%和16.9%。此外,它还实现了具有竞争力的PSNR分数,特别是在CN-EPFL数据集上比之前的最佳PSNR提高了4.6%,从而在重建质量上达到了更好的平衡。该模型轻巧高效,适合在临床和研究环境中进行实时应用。代码可在https://github.com/hdy6438/Spec2VolCAMU-Net获取。
论文及项目相关链接
Summary
本文提出了一种基于脑电图(EEG)的高分辨率功能磁共振成像(fMRI)映射方法,通过Spec2VolCAMU-Net模型实现EEG到fMRI的转换。该模型采用轻量级设计,解决了现有方法难以捕捉跨通道时间频率线索以及内存和稳定性问题。模型在三个公共基准测试上实现了最高保真度,并提供了实时应用在临床和研究环境中的可能性。代码已公开。
Key Takeaways
- 高分辨率功能磁共振成像(fMRI)在映射人类大脑活动方面至关重要,但其成本高昂且操作具有挑战性。
- 直接从广泛可用的头皮脑电图(EEG)生成可比体积数据可使高级神经成像更加普及。
- 现有EEG-to-fMRI生成器依赖于简单的CNN,无法捕捉跨通道的时间频率线索,或使用沉重的transformer/GAN解码器,存在内存和稳定性问题。
- 提出了一种名为Spec2VolCAMU-Net的轻量级光谱图生成器,解决了上述问题。采用多方向时间频率卷积注意力编码器与带有自注意力的时间、光谱和联合卷积相结合,以及Vision-Mamba U-Net解码器进行高效的长程空间建模。
- Spec2VolCAMU-Net采用混合SSI-MSE损失进行端到端训练,并在三个公共基准测试中达到最佳保真度,如NODDI、Oddball和CN-EPFL上的SSIM分数显著提高。
- 模型在保持高保真度的同时实现了竞争的PSNR分数,特别是在CN-EPFL数据集上取得了显著改进。
点此查看论文截图
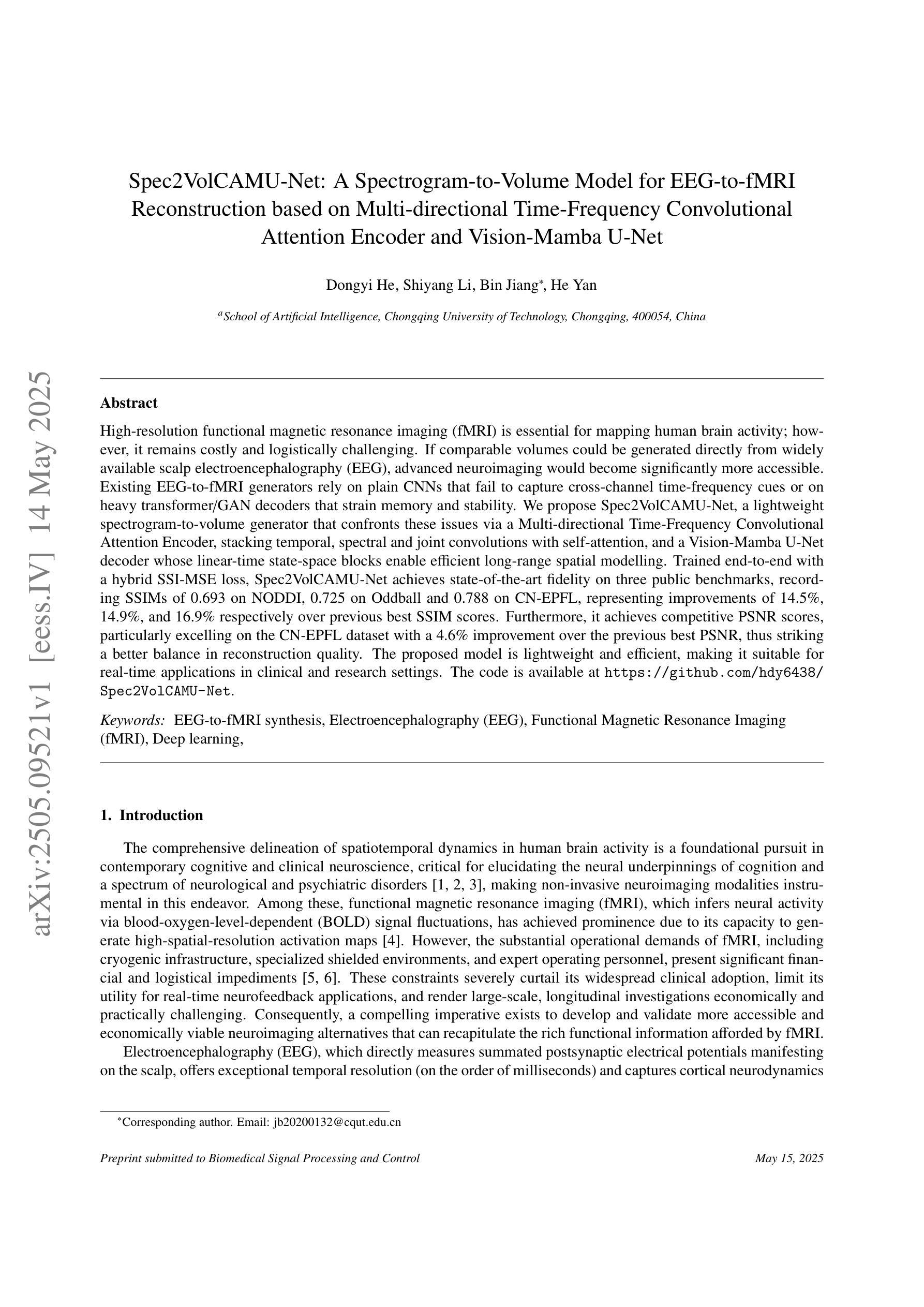
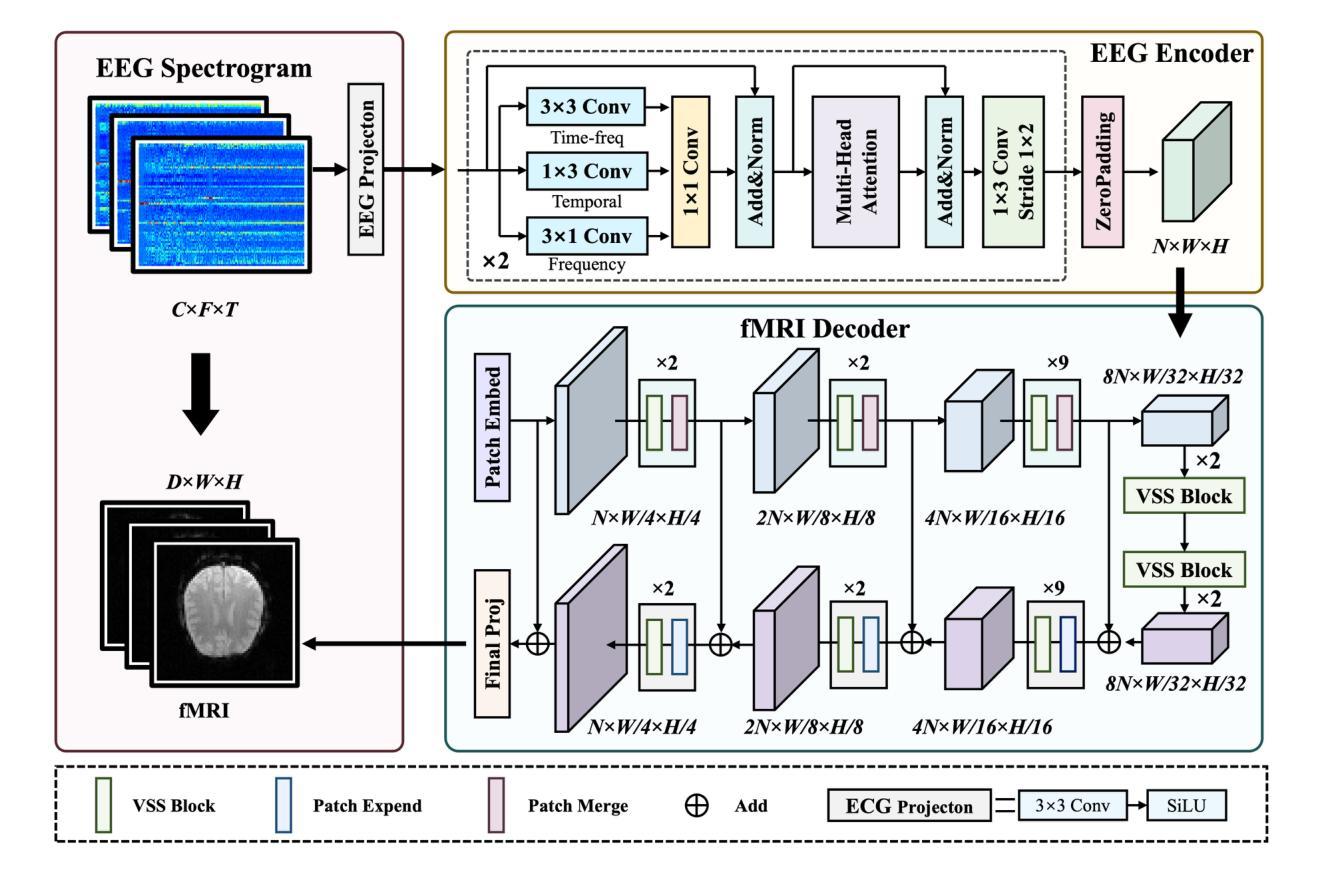
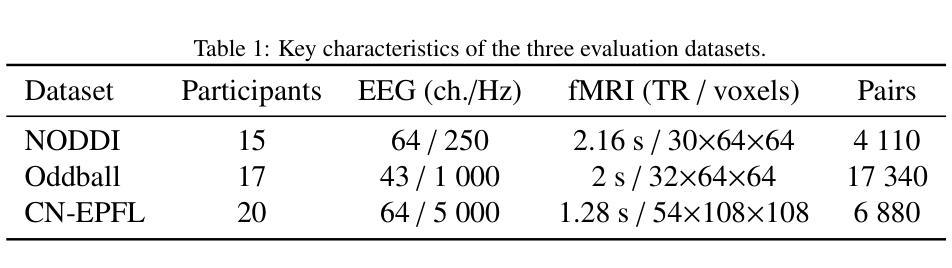
Generative AI for Autonomous Driving: Frontiers and Opportunities
Authors:Yuping Wang, Shuo Xing, Cui Can, Renjie Li, Hongyuan Hua, Kexin Tian, Zhaobin Mo, Xiangbo Gao, Keshu Wu, Sulong Zhou, Hengxu You, Juntong Peng, Junge Zhang, Zehao Wang, Rui Song, Mingxuan Yan, Walter Zimmer, Xingcheng Zhou, Peiran Li, Zhaohan Lu, Chia-Ju Chen, Yue Huang, Ryan A. Rossi, Lichao Sun, Hongkai Yu, Zhiwen Fan, Frank Hao Yang, Yuhao Kang, Ross Greer, Chenxi Liu, Eun Hak Lee, Xuan Di, Xinyue Ye, Liu Ren, Alois Knoll, Xiaopeng Li, Shuiwang Ji, Masayoshi Tomizuka, Marco Pavone, Tianbao Yang, Jing Du, Ming-Hsuan Yang, Hua Wei, Ziran Wang, Yang Zhou, Jiachen Li, Zhengzhong Tu
Generative Artificial Intelligence (GenAI) constitutes a transformative technological wave that reconfigures industries through its unparalleled capabilities for content creation, reasoning, planning, and multimodal understanding. This revolutionary force offers the most promising path yet toward solving one of engineering’s grandest challenges: achieving reliable, fully autonomous driving, particularly the pursuit of Level 5 autonomy. This survey delivers a comprehensive and critical synthesis of the emerging role of GenAI across the autonomous driving stack. We begin by distilling the principles and trade-offs of modern generative modeling, encompassing VAEs, GANs, Diffusion Models, and Large Language Models (LLMs). We then map their frontier applications in image, LiDAR, trajectory, occupancy, video generation as well as LLM-guided reasoning and decision making. We categorize practical applications, such as synthetic data workflows, end-to-end driving strategies, high-fidelity digital twin systems, smart transportation networks, and cross-domain transfer to embodied AI. We identify key obstacles and possibilities such as comprehensive generalization across rare cases, evaluation and safety checks, budget-limited implementation, regulatory compliance, ethical concerns, and environmental effects, while proposing research plans across theoretical assurances, trust metrics, transport integration, and socio-technical influence. By unifying these threads, the survey provides a forward-looking reference for researchers, engineers, and policymakers navigating the convergence of generative AI and advanced autonomous mobility. An actively maintained repository of cited works is available at https://github.com/taco-group/GenAI4AD.
生成式人工智能(GenAI)构成了一场变革性的技术浪潮,它通过无与伦比的内容创建、推理、规划和多模式理解能力,重新配置各行业。这场革命性的力量提供了解决工程领域最伟大挑战的最有前途的路径:实现可靠、完全自动驾驶,特别是对实现L5级别自动驾驶的追求。这篇综述全面而深入地探讨了GenAI在自动驾驶堆栈中的新兴角色。我们首先提炼了现代生成建模的原理和权衡,包括VAEs、GANs、扩散模型和大语言模型(LLM)。然后,我们将它们的前沿应用映射到图像、激光雷达、轨迹、占用、视频生成以及大语言模型指导的推理和决策制定。我们将分类实际应用,如合成数据流、端到端驾驶策略、高保真数字孪生系统、智能交通网络以及跨域转移到实体人工智能。我们确定了关键障碍和可能性,如全面概括罕见情况、评估和安全检查、预算有限实施、法规合规、道德关切和环境影响,同时就理论保证、信任指标、交通集成和社会技术影响提出研究计划。通过统一这些线索,这篇综述为研究人员、工程师和政策制定者提供了前瞻性参考,导航生成式人工智能和先进自动驾驶技术的融合。可在此处找到所引著作的活跃维护仓库:https://github.com/taco-group/GenAI4AD。
论文及项目相关链接
Summary
生成式人工智能(GenAI)通过其在内容创建、推理、规划和多模式理解方面的独特能力,为各行各业带来前所未有的变革。GenAI为自动驾驶的实现提供了最具前景的道路,尤其是实现第五级别的完全自动驾驶。该调查对GenAI在自动驾驶堆栈中的新兴角色进行了全面而关键的综述,并指出了其关键应用领域和未来研究方向。它为研究员、工程师和政策制定者提供了前瞻性的参考。更多详细内容请访问 https://github.com/taco-group/GenAI4AD。
Key Takeaways
- 生成式人工智能(GenAI)具有内容创建、推理、规划和多模式理解的独特能力,推动行业变革。
- GenAI为实现第五级别的完全自动驾驶提供了最具前景的道路。
- GenAI在自动驾驶堆栈中的新兴角色涉及多个领域,包括图像、LiDAR、轨迹、占用率、视频生成以及基于大型语言模型的推理和决策。
- GenAI的实际应用包括合成数据工作流程、端到端驾驶策略、高保真数字双胞胎系统和智能交通网络等。
- 实现GenAI面临诸多挑战,如全面泛化能力、评估和安全检查、预算限制的实施、法规合规性、道德关切和环境影响等。
点此查看论文截图
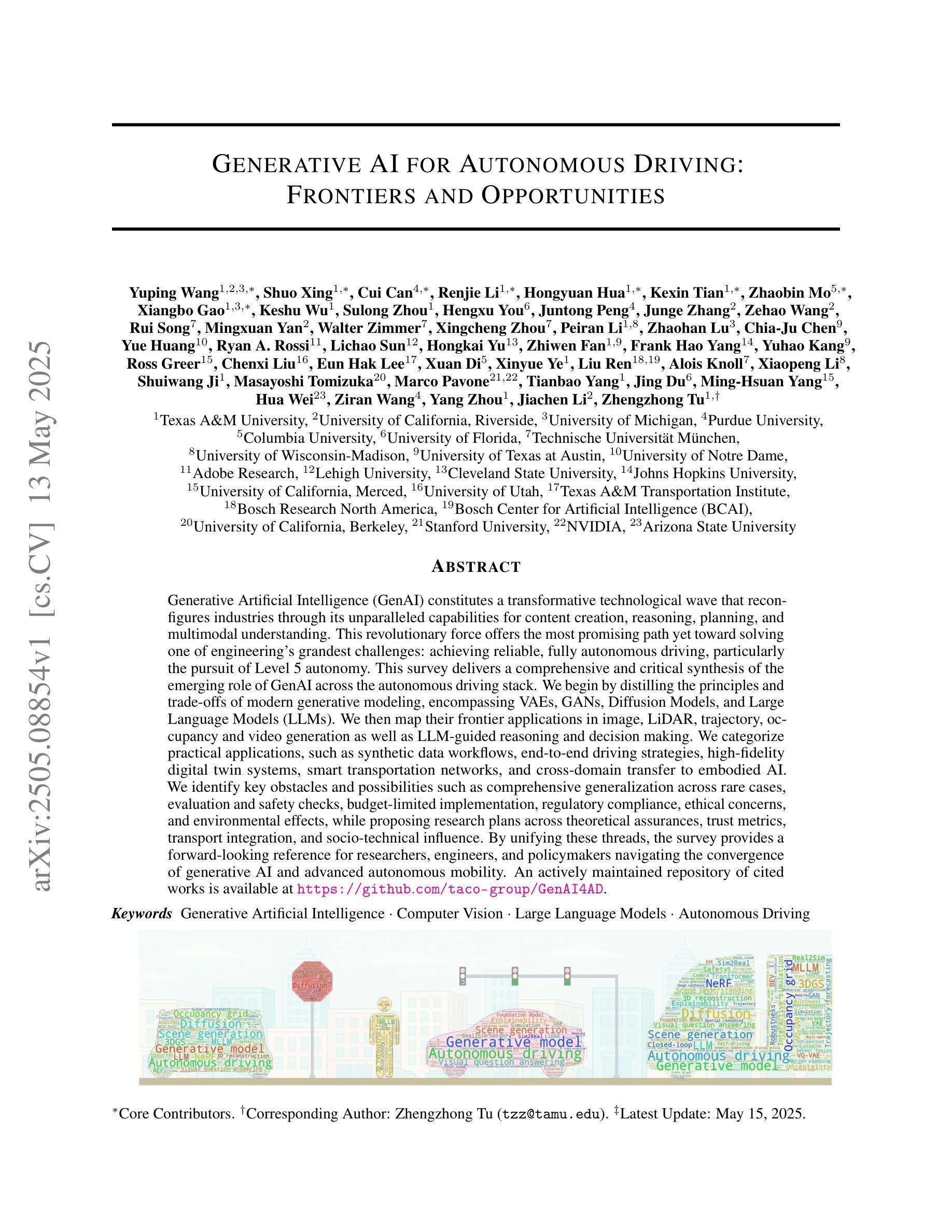