⚠️ 以下所有内容总结都来自于 大语言模型的能力,如有错误,仅供参考,谨慎使用
🔴 请注意:千万不要用于严肃的学术场景,只能用于论文阅读前的初筛!
💗 如果您觉得我们的项目对您有帮助 ChatPaperFree ,还请您给我们一些鼓励!⭐️ HuggingFace免费体验
2025-05-16 更新
WavReward: Spoken Dialogue Models With Generalist Reward Evaluators
Authors:Shengpeng Ji, Tianle Liang, Yangzhuo Li, Jialong Zuo, Minghui Fang, Jinzheng He, Yifu Chen, Zhengqing Liu, Ziyue Jiang, Xize Cheng, Siqi Zheng, Jin Xu, Junyang Lin, Zhou Zhao
End-to-end spoken dialogue models such as GPT-4o-audio have recently garnered significant attention in the speech domain. However, the evaluation of spoken dialogue models’ conversational performance has largely been overlooked. This is primarily due to the intelligent chatbots convey a wealth of non-textual information which cannot be easily measured using text-based language models like ChatGPT. To address this gap, we propose WavReward, a reward feedback model based on audio language models that can evaluate both the IQ and EQ of spoken dialogue systems with speech input. Specifically, 1) based on audio language models, WavReward incorporates the deep reasoning process and the nonlinear reward mechanism for post-training. By utilizing multi-sample feedback via the reinforcement learning algorithm, we construct a specialized evaluator tailored to spoken dialogue models. 2) We introduce ChatReward-30K, a preference dataset used to train WavReward. ChatReward-30K includes both comprehension and generation aspects of spoken dialogue models. These scenarios span various tasks, such as text-based chats, nine acoustic attributes of instruction chats, and implicit chats. WavReward outperforms previous state-of-the-art evaluation models across multiple spoken dialogue scenarios, achieving a substantial improvement about Qwen2.5-Omni in objective accuracy from 55.1$%$ to 91.5$%$. In subjective A/B testing, WavReward also leads by a margin of 83$%$. Comprehensive ablation studies confirm the necessity of each component of WavReward. All data and code will be publicly at https://github.com/jishengpeng/WavReward after the paper is accepted.
端到端的口语对话模型,如GPT-4o-audio,最近在语音领域引起了极大的关注。然而,对口语对话模型的会话性能评估却被大大忽视了。这主要是因为智能聊天机器人传递了大量的非文本信息,这些非文本信息无法轻易使用基于文本的模型进行评估,如ChatGPT。为了弥补这一空白,我们提出了WavReward,一种基于音频语言模型的奖励反馈模型,它可以评估口语对话系统通过语音输入的智商和情商。具体来说,1)WavReward基于音频语言模型,结合了深度推理过程和用于后训练的非线性奖励机制。通过利用强化学习算法的多个样本反馈,我们构建了一个专为口语对话模型量身定制的评估器。2)我们引入了用于训练WavReward的偏好数据集ChatReward-30K。ChatReward-30K涵盖了口语对话模型的理解和生成方面。这些场景涵盖了各种任务,如文本聊天、指令聊天的九个声音特征以及隐式聊天。WavReward在多个口语对话场景中表现出超越先前最先进的评估模型的能力,在客观准确性方面实现了从55.1%到91.5%的实质性改进。在主观的A/B测试中,WavReward也以83%的优势领先。全面的消融研究证实了WavReward每个组件的必要性。论文被接受后,所有数据和代码将在https://github.com/jishengpeng/WavReward上公开。
论文及项目相关链接
Summary
本文介绍了针对语音对话模型评估的新的挑战,提出了基于音频语言模型的奖励反馈模型WavReward。该模型可以评估语音对话系统的智商和情商,通过深度推理和非线性奖励机制进行后训练。同时引入了用于训练WavReward的ChatReward-30K偏好数据集,并在多个语音对话场景中表现出优于现有评价模型的性能。
Key Takeaways
- 当前语音对话模型如GPT-4o-audio备受关注,但模型的对话性能评估却被忽视。
- 语音对话系统传递的非文本信息无法用基于文本的模型如ChatGPT来评估。
- WavReward是一个基于音频语言模型的奖励反馈模型,旨在评估语音对话系统的智商和情商。
- WavReward采用深度推理和非线性奖励机制进行后训练。
- 引入了ChatReward-30K数据集,包含对话模型的理解与生成方面。
- WavReward在多个语音对话场景中表现出卓越性能,客观准确率从55.1%提高到91.5%。
点此查看论文截图
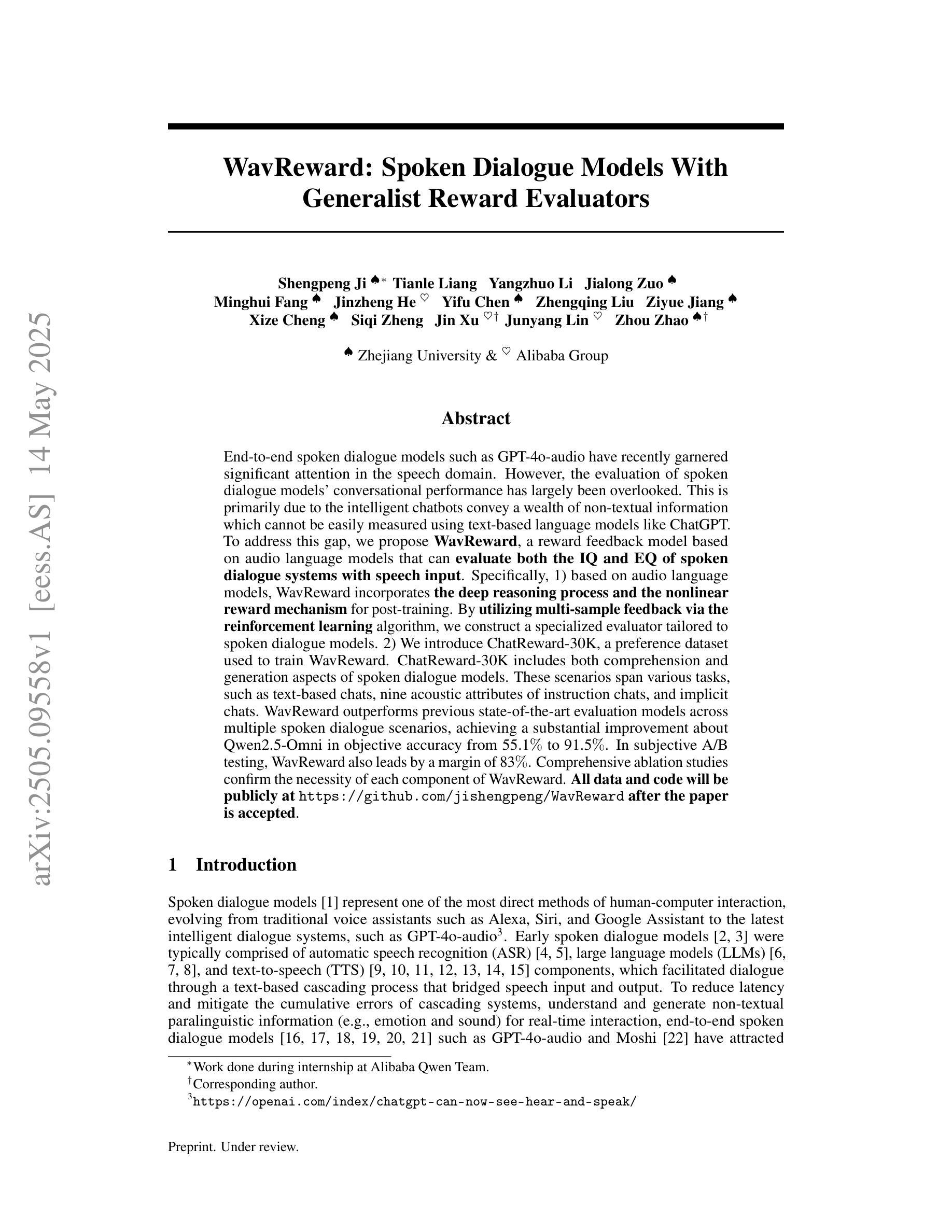
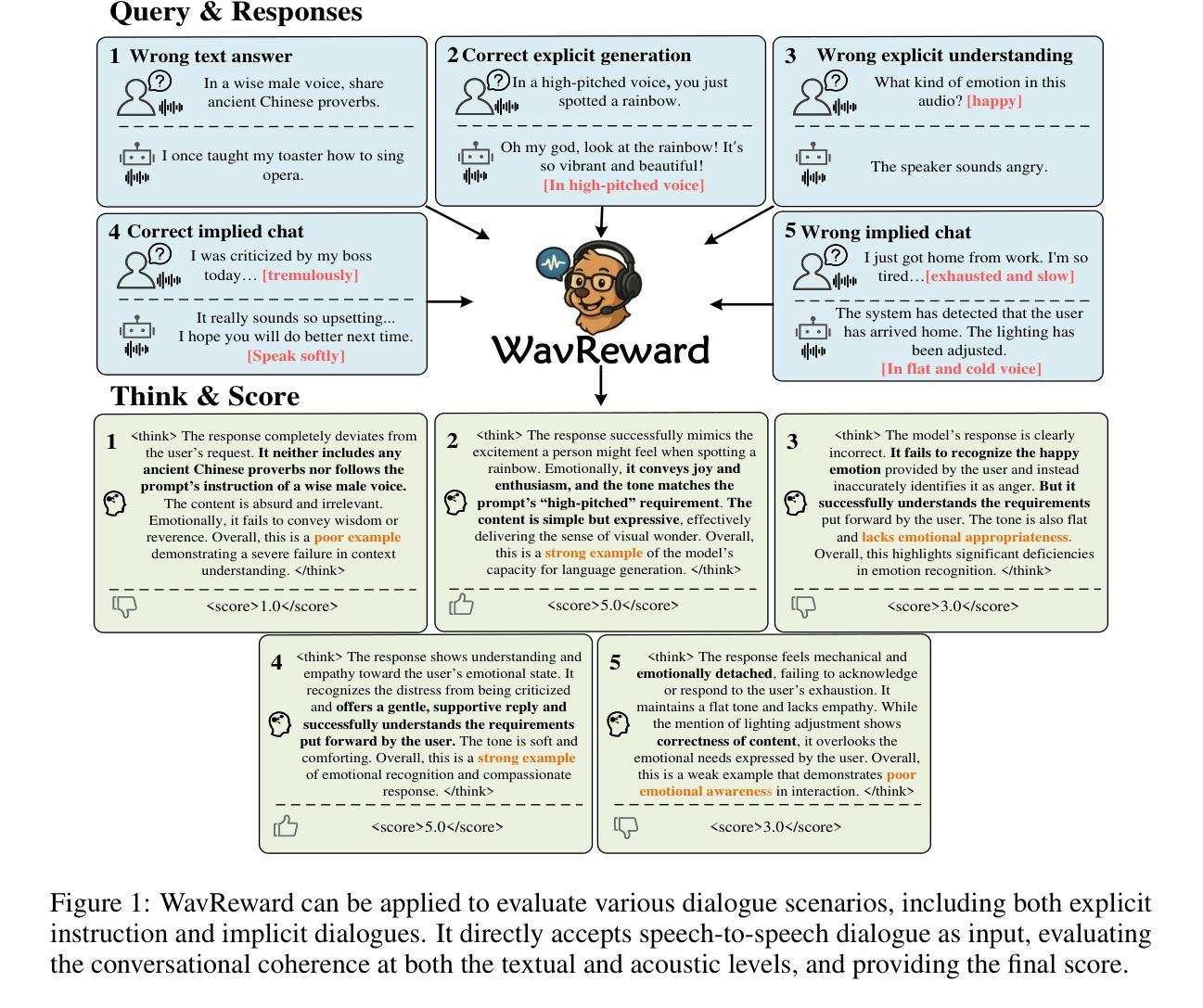
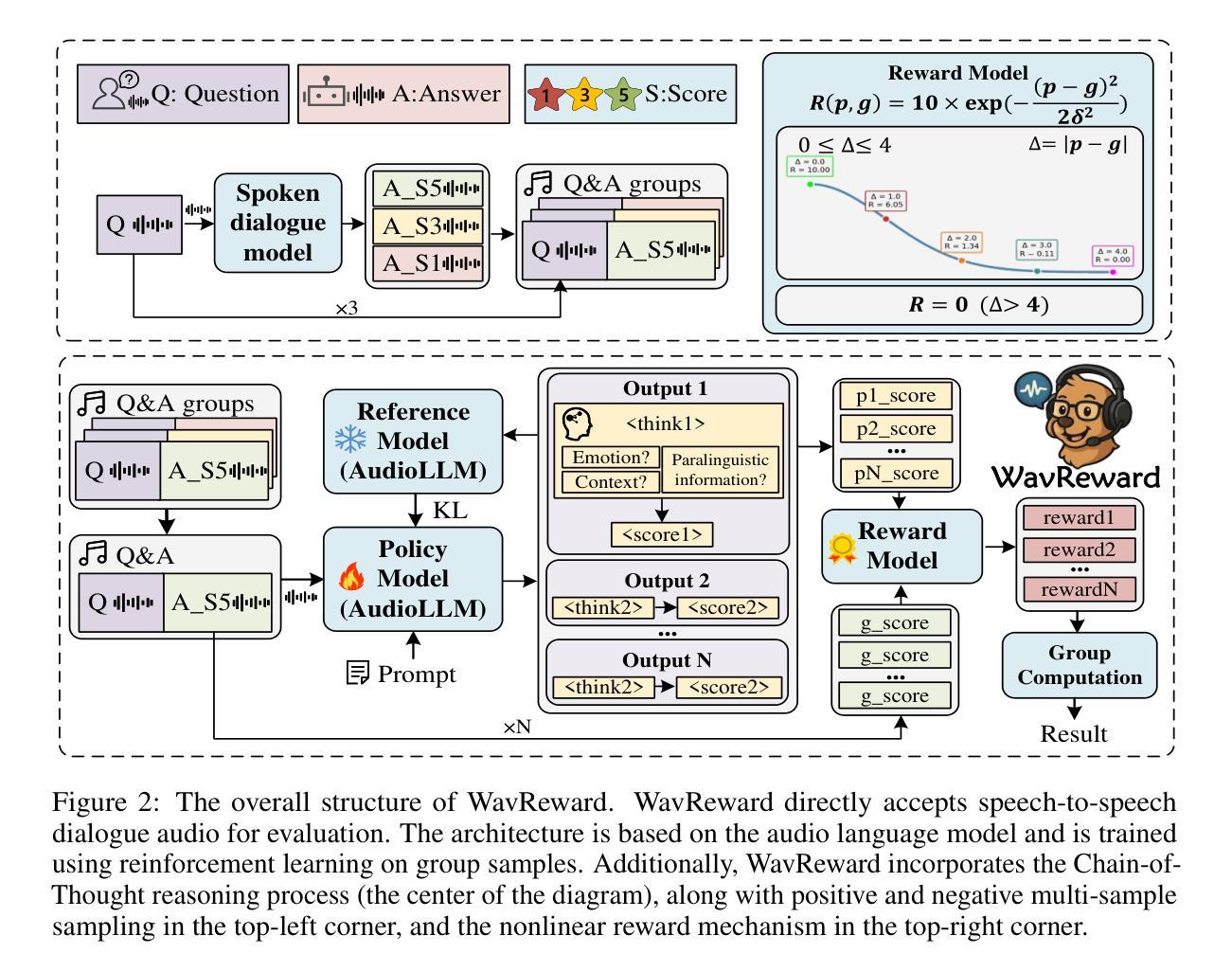