⚠️ 以下所有内容总结都来自于 大语言模型的能力,如有错误,仅供参考,谨慎使用
🔴 请注意:千万不要用于严肃的学术场景,只能用于论文阅读前的初筛!
💗 如果您觉得我们的项目对您有帮助 ChatPaperFree ,还请您给我们一些鼓励!⭐️ HuggingFace免费体验
2025-05-16 更新
DPN-GAN: Inducing Periodic Activations in Generative Adversarial Networks for High-Fidelity Audio Synthesis
Authors:Zeeshan Ahmad, Shudi Bao, Meng Chen
In recent years, generative adversarial networks (GANs) have made significant progress in generating audio sequences. However, these models typically rely on bandwidth-limited mel-spectrograms, which constrain the resolution of generated audio sequences, and lead to mode collapse during conditional generation. To address this issue, we propose Deformable Periodic Network based GAN (DPN-GAN), a novel GAN architecture that incorporates a kernel-based periodic ReLU activation function to induce periodic bias in audio generation. This innovative approach enhances the model’s ability to capture and reproduce intricate audio patterns. In particular, our proposed model features a DPN module for multi-resolution generation utilizing deformable convolution operations, allowing for adaptive receptive fields that improve the quality and fidelity of the synthetic audio. Additionally, we enhance the discriminator network using deformable convolution to better distinguish between real and generated samples, further refining the audio quality. We trained two versions of the model: DPN-GAN small (38.67M parameters) and DPN-GAN large (124M parameters). For evaluation, we use five different datasets, covering both speech synthesis and music generation tasks, to demonstrate the efficiency of the DPN-GAN. The experimental results demonstrate that DPN-GAN delivers superior performance on both out-of-distribution and noisy data, showcasing its robustness and adaptability. Trained across various datasets, DPN-GAN outperforms state-of-the-art GAN architectures on standard evaluation metrics, and exhibits increased robustness in synthesized audio.
近年来,生成对抗网络(GANs)在生成音频序列方面取得了显著进展。然而,这些模型通常依赖于带宽有限的梅尔频谱图,这限制了生成音频序列的分辨率,并在条件生成过程中导致模式崩溃。为了解决这一问题,我们提出了基于可变形周期网络的可变形周期网络GAN(DPN-GAN),这是一种新型的GAN架构,它引入了一种基于内核的周期ReLU激活函数,从而在音频生成中引入周期偏差。这种创新的方法提高了模型捕捉和再现复杂音频模式的能力。特别是,我们提出的模型具有使用可变形卷积操作进行多分辨率生成的DPN模块,允许自适应的感受野,提高了合成音频的质量和保真度。此外,我们还通过增强判别网络使用可变形卷积来更好地区分真实和生成的样本,进一步提高了音频质量。我们训练了两个版本的模型:DPN-GAN小型(含3867万个参数)和DPN-GAN大型(含约一亿两千四百万个参数)。为了评估模型性能,我们使用了五个不同的数据集,涵盖了语音合成和音乐生成任务,以证明DPN-GAN的有效性。实验结果表明,DPN-GAN在处理离群点和噪声数据时表现出卓越的性能,证明了其稳健性和适应性。在各种数据集上训练的DPN-GAN在标准评估指标上优于最新的GAN架构,并在合成音频中展现出更高的稳健性。
论文及项目相关链接
Summary
本文提出一种基于可变形周期网络(DPN)的生成对抗网络(GAN)架构,用于生成音频序列。通过引入基于内核的周期ReLU激活函数,提高模型捕捉和复现复杂音频模式的能力。采用DPN模块实现多分辨率生成,结合可变形卷积操作,提高合成音频的质量和保真度。此外,改进判别器网络,使其能更好地区分真实和生成样本,进一步改善音频质量。实验结果显示,DPN-GAN在合成音频的稳健性和适应性方面表现优越,优于现有GAN架构。
Key Takeaways
- 提出一种新型的基于可变形周期网络的生成对抗网络(DPN-GAN),用于音频生成。
- 引入周期ReLU激活函数,增加模型在音频生成中的周期性偏差。
- DPN模块用于多分辨率生成,结合可变形卷积操作,提高音频质量和保真度。
- 改进判别器网络,使其能更好地分辨真实和生成的音频样本。
- DPN-GAN模型训练了两种版本:小型(38.67M参数)和大型(124M参数)。
- 在语音合成和音乐生成任务上的五个数据集上进行了评估,证明了DPN-GAN的高效性。
点此查看论文截图
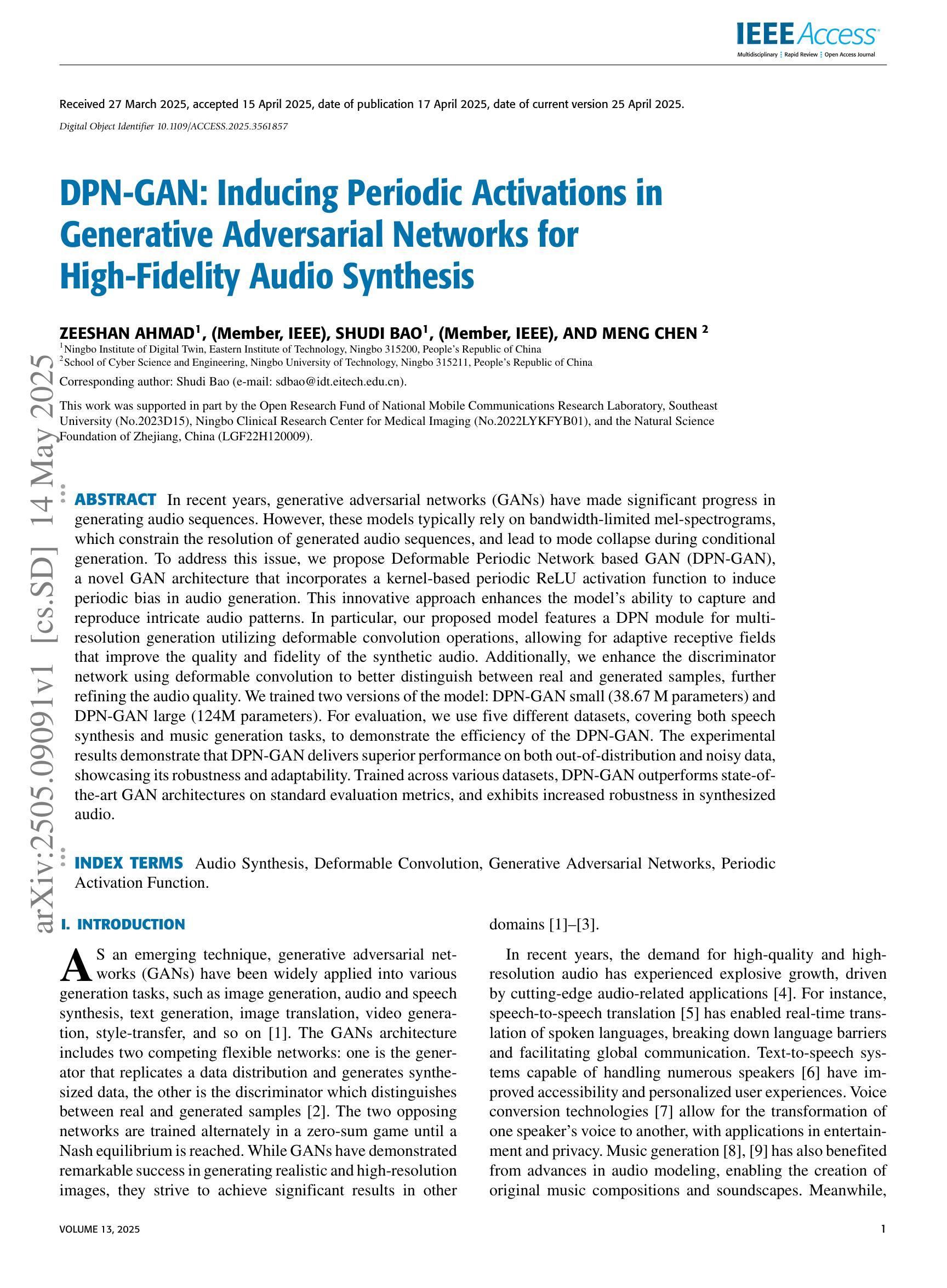
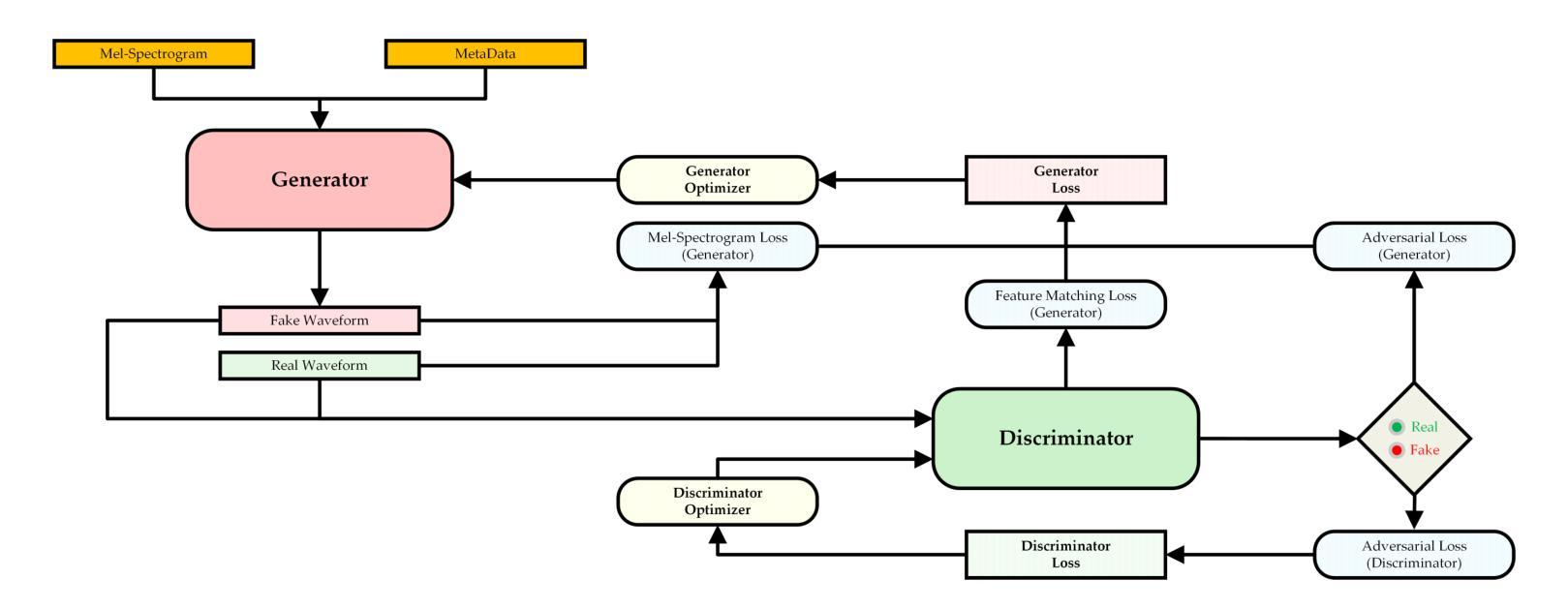
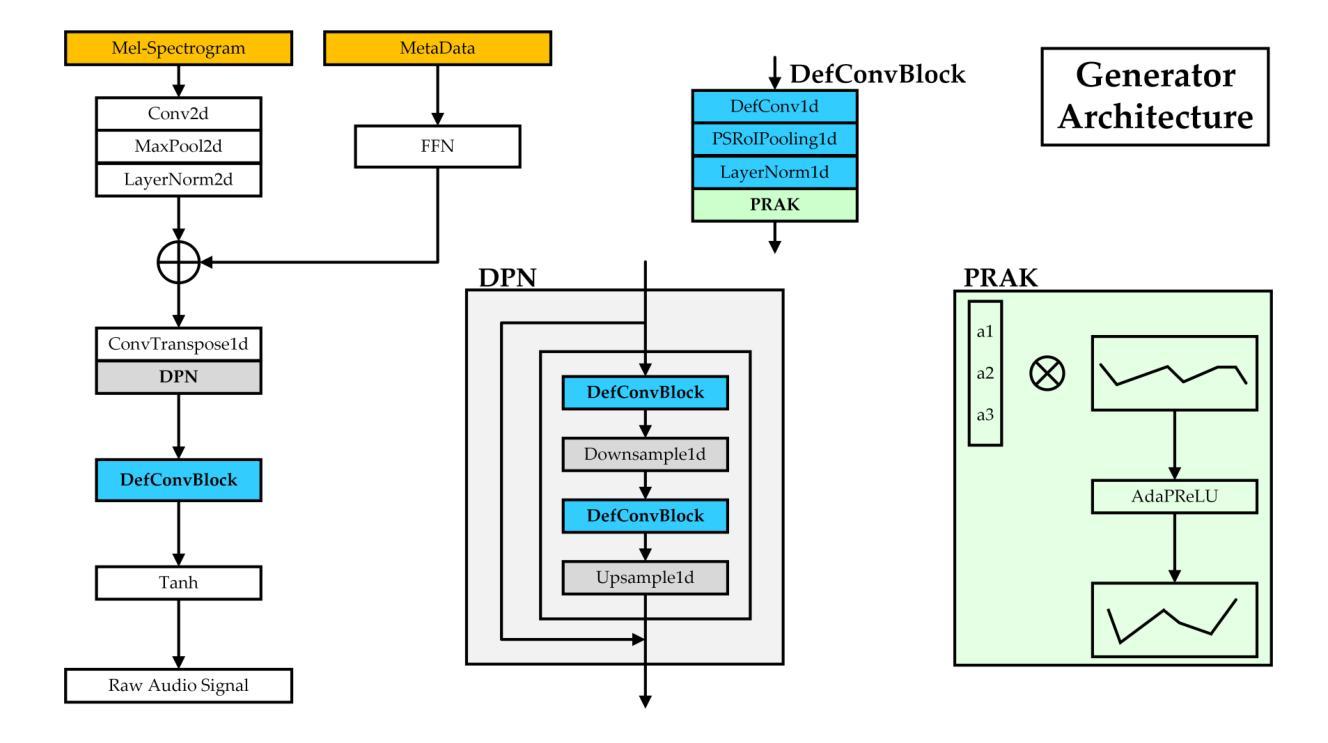