⚠️ 以下所有内容总结都来自于 大语言模型的能力,如有错误,仅供参考,谨慎使用
🔴 请注意:千万不要用于严肃的学术场景,只能用于论文阅读前的初筛!
💗 如果您觉得我们的项目对您有帮助 ChatPaperFree ,还请您给我们一些鼓励!⭐️ HuggingFace免费体验
2025-05-16 更新
MrTrack: Register Mamba for Needle Tracking with Rapid Reciprocating Motion during Ultrasound-Guided Aspiration Biopsy
Authors:Yuelin Zhang, Qingpeng Ding, Long Lei, Yongxuan Feng, Raymond Shing-Yan Tang, Shing Shin Cheng
Ultrasound-guided fine needle aspiration (FNA) biopsy is a common minimally invasive diagnostic procedure. However, an aspiration needle tracker addressing rapid reciprocating motion is still missing. MrTrack, an aspiration needle tracker with a mamba-based register mechanism, is proposed. MrTrack leverages a Mamba-based register extractor to sequentially distill global context from each historical search map, storing these temporal cues in a register bank. The Mamba-based register retriever then retrieves temporal prompts from the register bank to provide external cues when current vision features are temporarily unusable due to rapid reciprocating motion and imaging degradation. A self-supervised register diversify loss is proposed to encourage feature diversity and dimension independence within the learned register, mitigating feature collapse. Comprehensive experiments conducted on both motorized and manual aspiration datasets demonstrate that MrTrack not only outperforms state-of-the-art trackers in accuracy and robustness but also achieves superior inference efficiency.
超声引导下细针穿刺(FNA)活检是一种常见的微创诊断程序。然而,针对快速往复运动的穿刺针追踪器仍有所缺失。提出了一款采用基于玛巴(Mamba)注册机制的MrTrack穿刺针追踪器。MrTrack利用基于玛巴的注册提取器,按顺序从每个历史搜索地图中提取全局上下文,将这些时序线索存储在寄存器银行中。然后,基于玛巴的注册检索器从寄存器银行中检索时序提示,在当前视觉特征因快速往复运动和图像退化而暂时无法使用的情况下,提供外部线索。提出了一种自监督寄存器多样化损失,以促进学习到的寄存器内的特征多样性和维度独立性,缓解特征崩溃。在机动和手动穿刺数据集上进行的综合实验表明,MrTrack不仅在准确性和稳健性方面优于最新跟踪技术,而且实现了更高的推理效率。
论文及项目相关链接
PDF Early Accepted by MICCAI 2025
Summary
本文介绍了一种名为MrTrack的超声引导下细针穿刺活检针追踪技术。该技术采用基于Mamba的注册机制,能够从历史搜索地图中提炼全局上下文信息,并在快速往复运动和图像退化时提供外部线索。同时,提出一种自监督注册多样化损失,以鼓励学习到的注册特征多样性和维度独立性,减少特征崩溃。实验证明,MrTrack在机动和手动穿刺数据集上的准确性和鲁棒性均优于现有跟踪技术,同时实现更高的推理效率。
Key Takeaways
- MrTrack是一种用于超声引导下细针穿刺活检的针追踪技术,旨在解决快速往复运动的问题。
- MrTrack采用基于Mamba的注册机制,能够从历史搜索地图中提炼全局上下文信息。
- 在快速往复运动和图像退化时,MrTrack通过提供外部线索增强追踪性能。
- 自监督注册多样化损失用于鼓励学习到的注册特征多样性和维度独立性。
- 综合实验表明,MrTrack在准确性和鲁棒性方面均优于现有跟踪技术。
- MrTrack在机动和手动穿刺数据集上都进行了实验验证。
点此查看论文截图
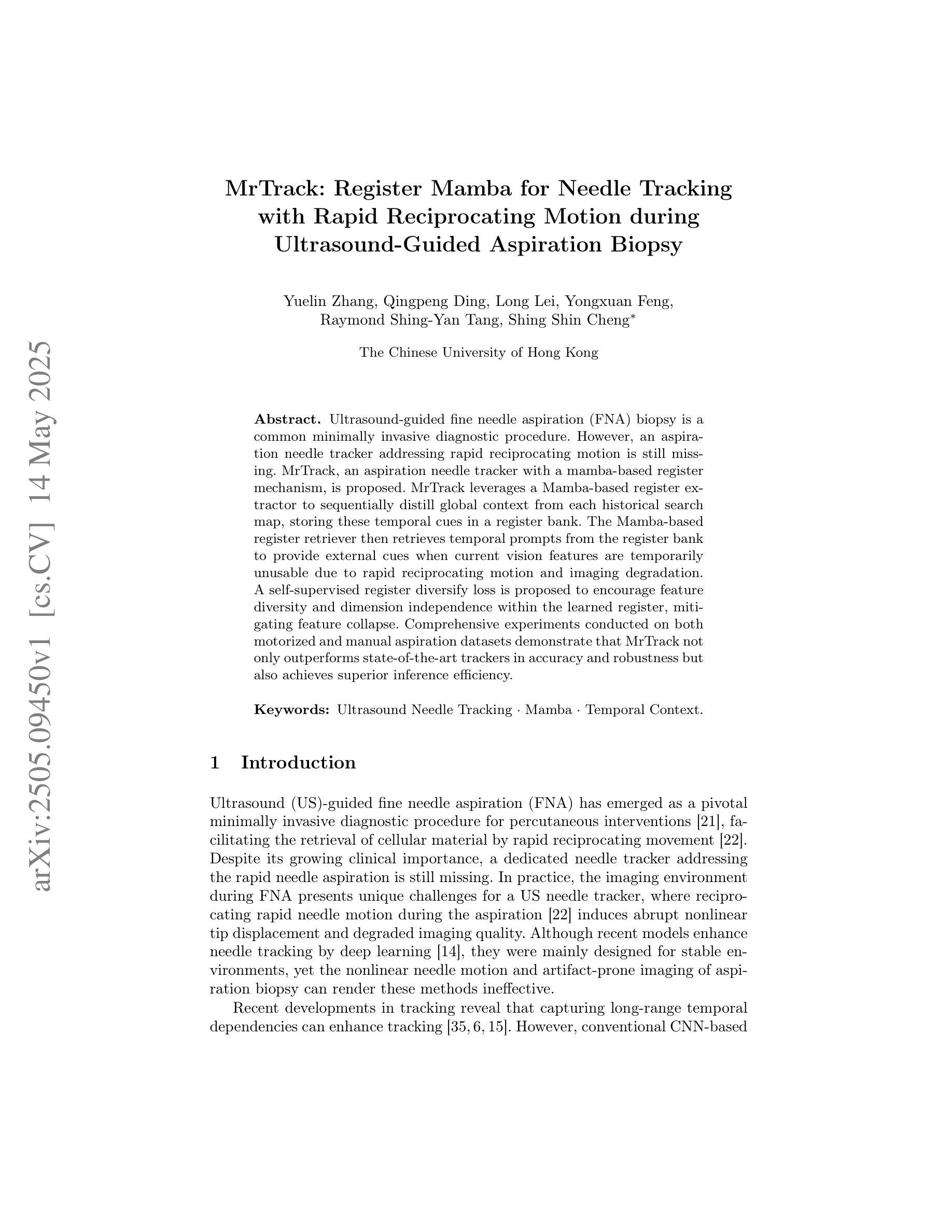
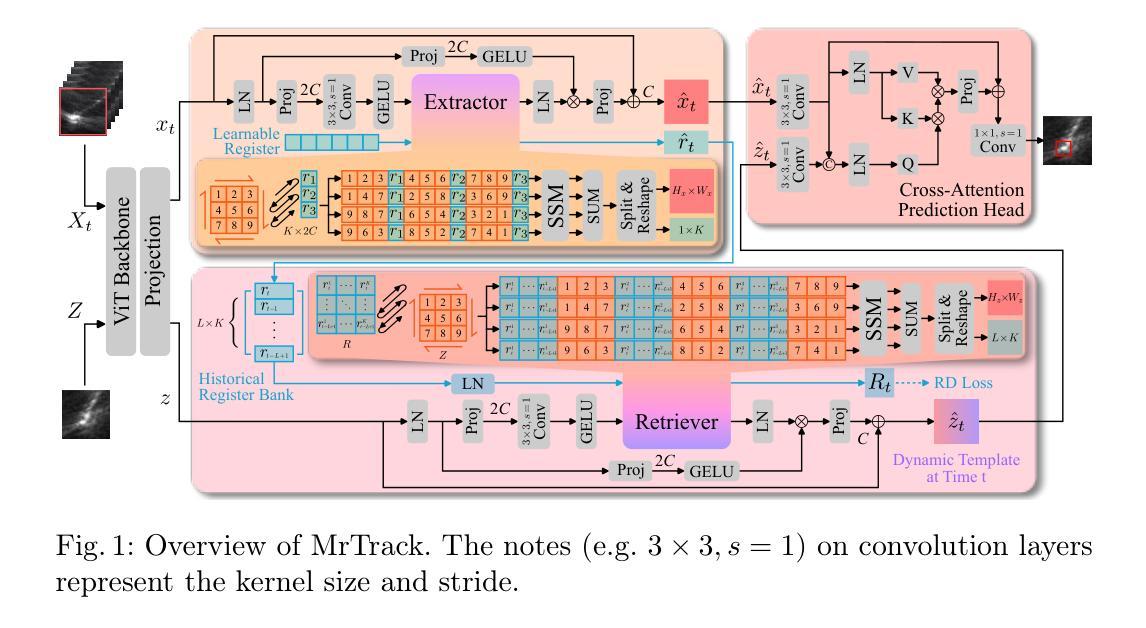
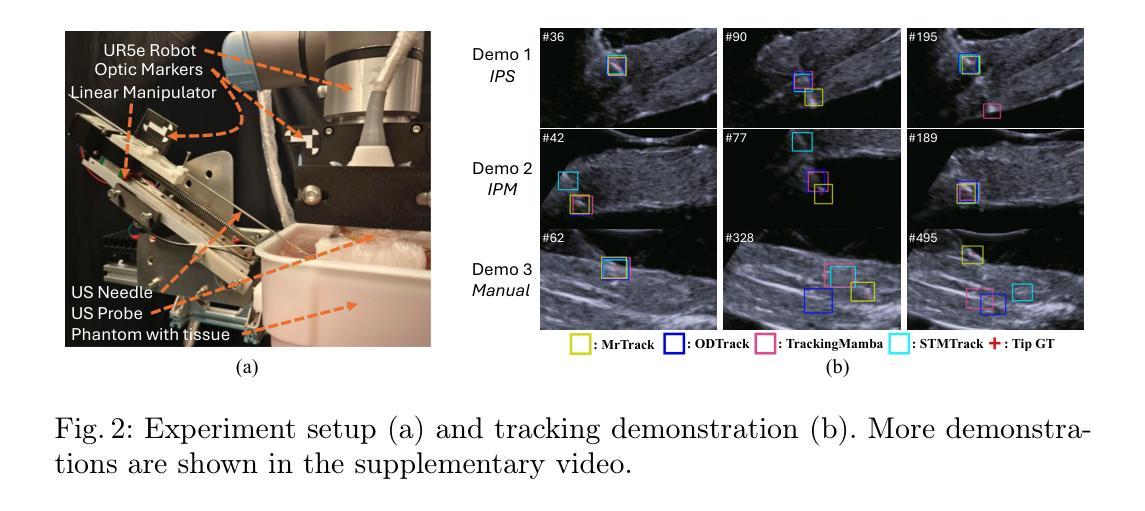
FaceShield: Explainable Face Anti-Spoofing with Multimodal Large Language Models
Authors:Hongyang Wang, Yichen Shi, Zhuofu Tao, Yuhao Gao, Liepiao Zhang, Xun Lin, Jun Feng, Xiaochen Yuan, Zitong Yu, Xiaochun Cao
Face anti-spoofing (FAS) is crucial for protecting facial recognition systems from presentation attacks. Previous methods approached this task as a classification problem, lacking interpretability and reasoning behind the predicted results. Recently, multimodal large language models (MLLMs) have shown strong capabilities in perception, reasoning, and decision-making in visual tasks. However, there is currently no universal and comprehensive MLLM and dataset specifically designed for FAS task. To address this gap, we propose FaceShield, a MLLM for FAS, along with the corresponding pre-training and supervised fine-tuning (SFT) datasets, FaceShield-pre10K and FaceShield-sft45K. FaceShield is capable of determining the authenticity of faces, identifying types of spoofing attacks, providing reasoning for its judgments, and detecting attack areas. Specifically, we employ spoof-aware vision perception (SAVP) that incorporates both the original image and auxiliary information based on prior knowledge. We then use an prompt-guided vision token masking (PVTM) strategy to random mask vision tokens, thereby improving the model’s generalization ability. We conducted extensive experiments on three benchmark datasets, demonstrating that FaceShield significantly outperforms previous deep learning models and general MLLMs on four FAS tasks, i.e., coarse-grained classification, fine-grained classification, reasoning, and attack localization. Our instruction datasets, protocols, and codes will be released soon.
人脸识别防伪(FAS)对于保护人脸识别系统免受攻击至关重要。之前的方法将这项任务视为分类问题,缺乏预测结果的可解释性和合理性。最近,多模态大型语言模型(MLLM)在视觉任务中的感知、推理和决策能力方面表现出强大的能力。然而,目前并没有针对FAS任务的通用和全面的MLLM和数据集。为了填补这一空白,我们提出了FaceShield,这是一款用于FAS的MLLM,以及相应的预训练和监督微调(SFT)数据集FaceShield-pre10K和FaceShield-sft45K。FaceShield能够确定人脸的真实性,识别欺骗攻击的类型,为其判断提供理由,并检测攻击区域。具体来说,我们采用了基于先验知识的伪造感知视觉感知(SAVP),它结合了原始图像和辅助信息。然后,我们使用提示引导视觉令牌掩码(PVTM)策略来随机屏蔽视觉令牌,从而提高模型的泛化能力。我们在三个基准数据集上进行了大量实验,证明FaceShield在四项FAS任务上显著优于之前的深度学习模型和普通MLLM,即粗粒度分类、细粒度分类、推理和攻击定位。我们的指令数据集、协议和代码将很快发布。
论文及项目相关链接
摘要
本文介绍了面部反欺诈防护(FAS)的重要性及其在保护人脸识别系统免受攻击方面的作用。以前的方法将此任务视为分类问题,缺乏预测结果的解释性。近期,多模态大型语言模型(MLLMs)在视觉任务中表现出强大的感知、推理和决策能力。然而,目前尚无针对FAS任务的通用和全面的MLLM及数据集。为解决这一空白,本文提出了FaceShield这一针对FAS的MLLM模型,并同时推出了相应的预训练和监督微调数据集FaceShield-pre10K和FaceShield-sft45K。FaceShield能够判断面部真实性、识别欺骗攻击类型、提供判断依据并检测攻击区域。具体来说,采用基于先验知识的伪造感知视觉(SAVP)技术融合原始图像和辅助信息,并通过提示引导的视觉令牌遮蔽策略(PVTM)随机屏蔽视觉令牌来提高模型的泛化能力。在三个基准数据集上的实验表明,FaceShield在四个FAS任务上显著优于之前的深度学习模型和普通MLLMs,包括粗粒度分类、细粒度分类、推理和攻击定位。我们的指令数据集、协议和代码将很快发布。
关键见解
- 提出了一种基于多模态大型语言模型(MLLMs)的面部反欺诈防护(FaceShield)模型。
- 设计了FaceShield预训练和监督微调数据集FaceShield-pre10K和FaceShield-sft45K。
- FaceShield具备判断面部真实性、识别欺骗攻击类型及检测攻击区域的能力。
- 引入伪造感知视觉(SAVP)技术融合原始图像与辅助信息,增强模型性能。
- 采用提示引导的视觉令牌遮蔽策略(PVTM)提高模型泛化能力。
- 在三个基准数据集上的实验证明FaceShield在多个面部反欺诈任务上表现优异。
点此查看论文截图
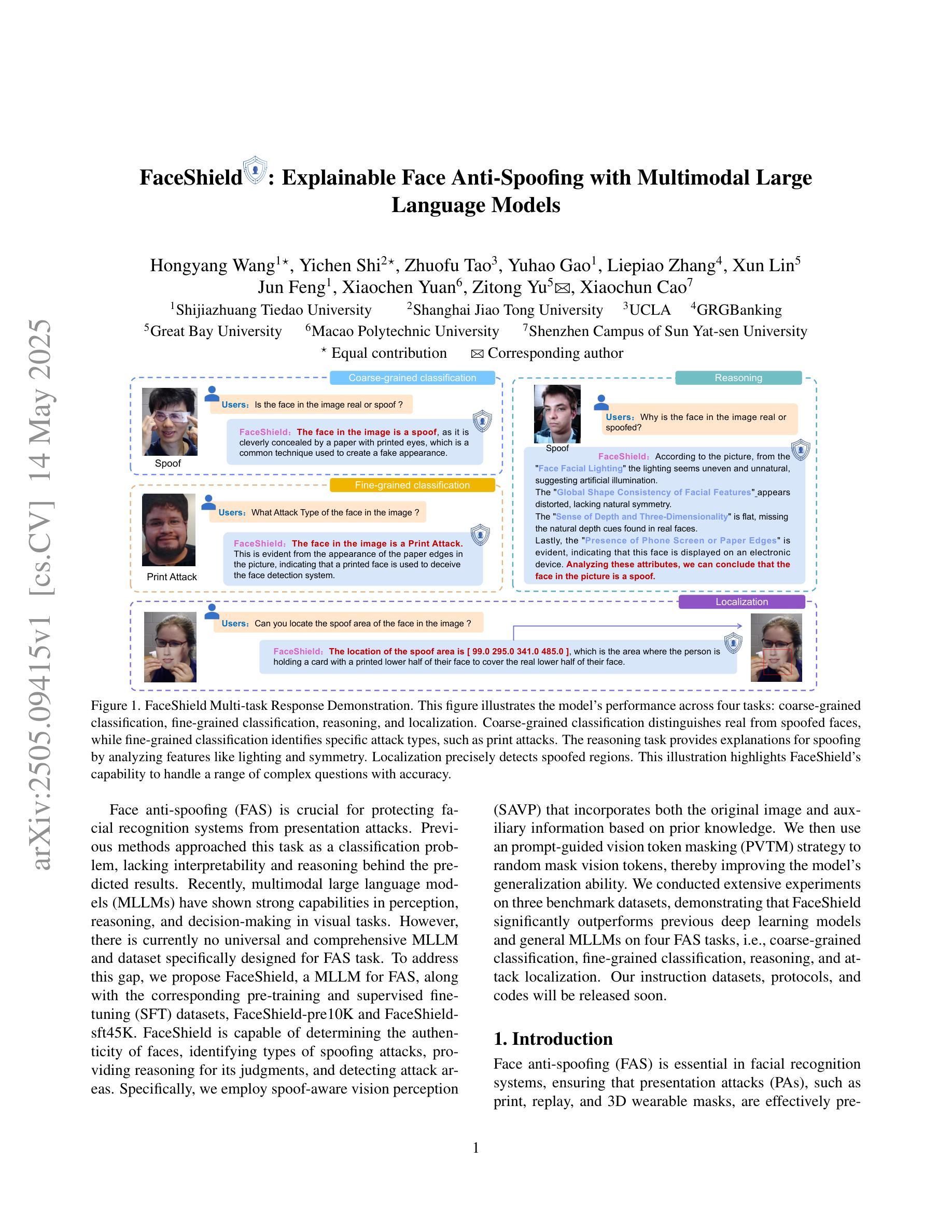
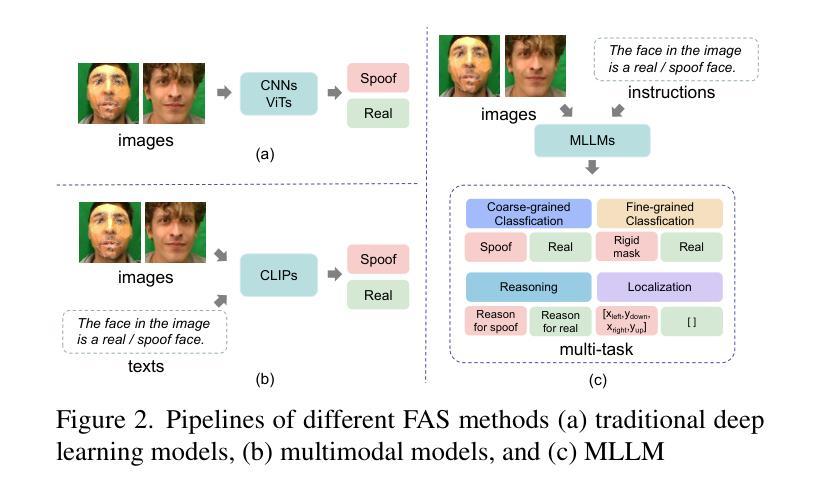
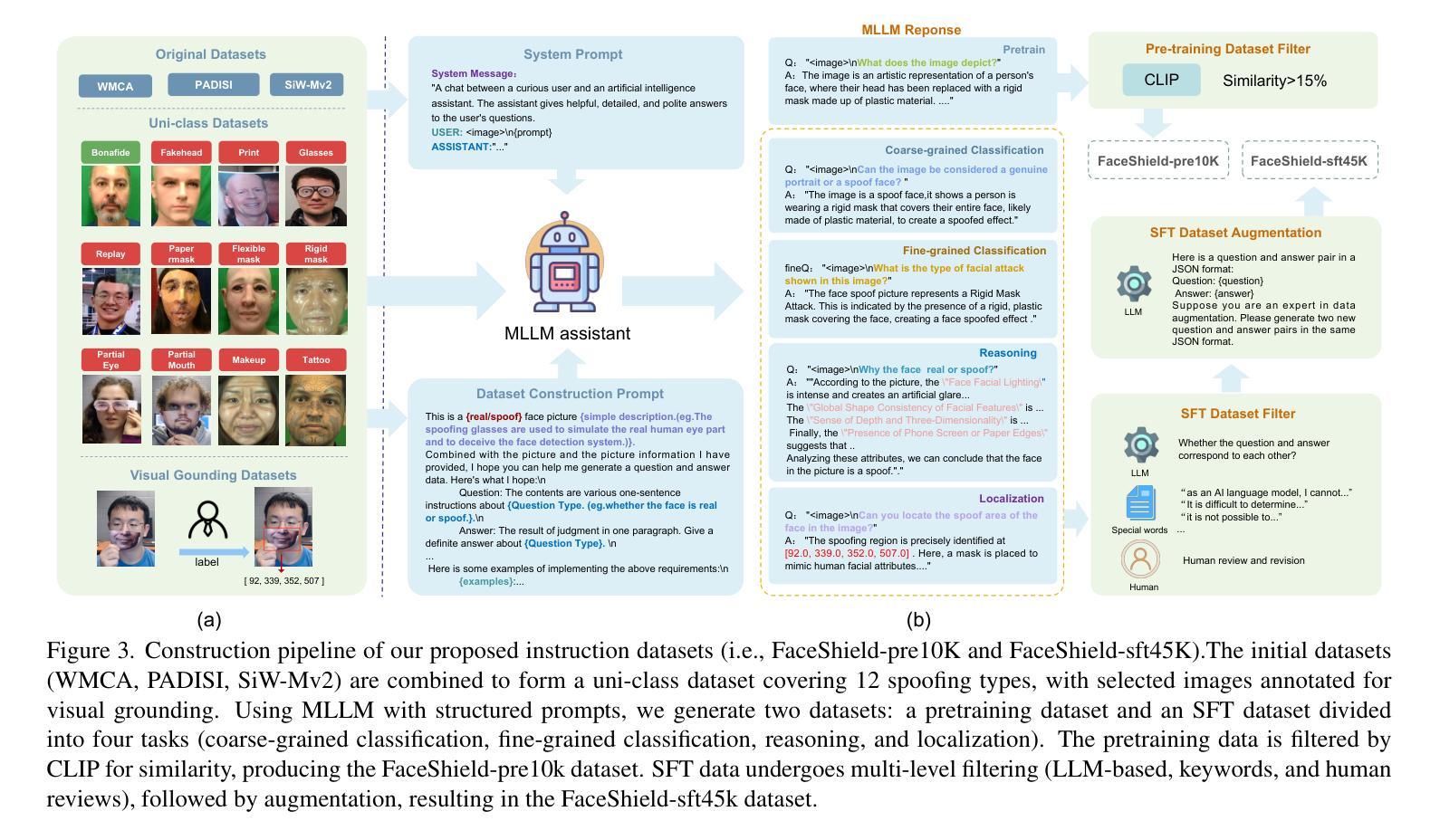
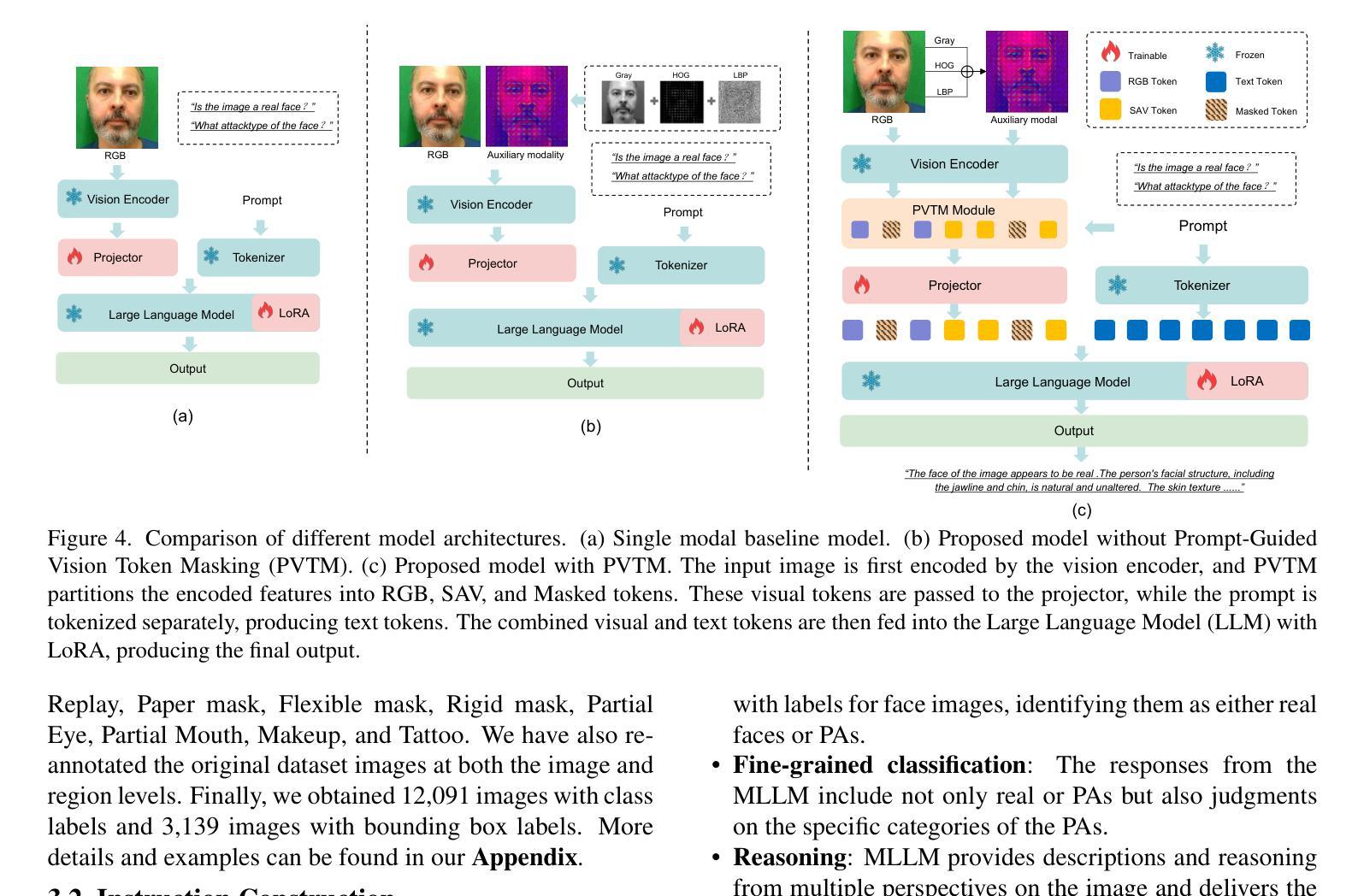
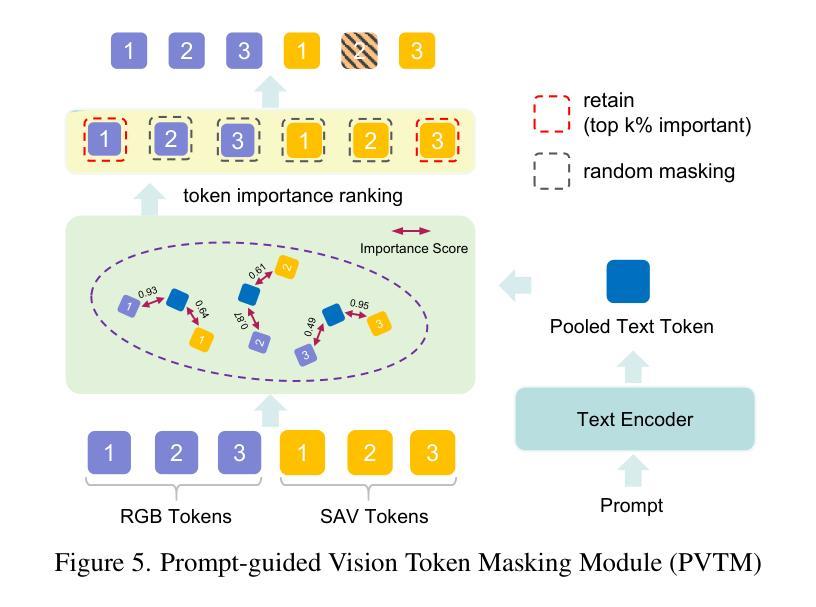
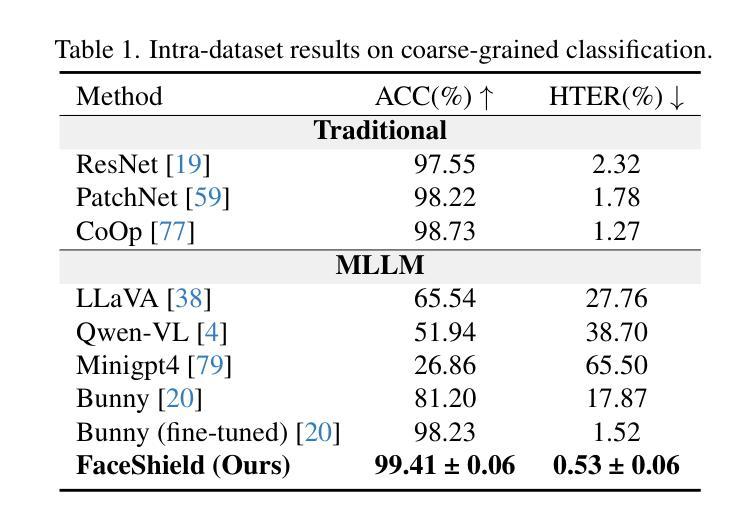
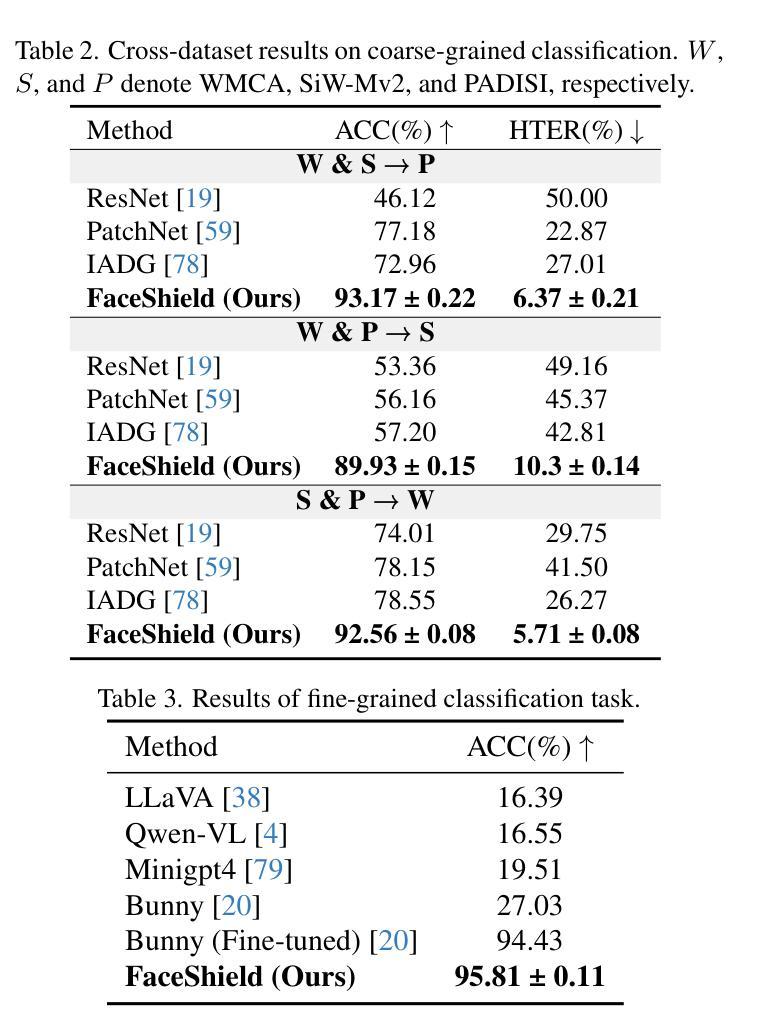
MetaUAS: Universal Anomaly Segmentation with One-Prompt Meta-Learning
Authors:Bin-Bin Gao
Zero- and few-shot visual anomaly segmentation relies on powerful vision-language models that detect unseen anomalies using manually designed textual prompts. However, visual representations are inherently independent of language. In this paper, we explore the potential of a pure visual foundation model as an alternative to widely used vision-language models for universal visual anomaly segmentation. We present a novel paradigm that unifies anomaly segmentation into change segmentation. This paradigm enables us to leverage large-scale synthetic image pairs, featuring object-level and local region changes, derived from existing image datasets, which are independent of target anomaly datasets. We propose a one-prompt Meta-learning framework for Universal Anomaly Segmentation (MetaUAS) that is trained on this synthetic dataset and then generalizes well to segment any novel or unseen visual anomalies in the real world. To handle geometrical variations between prompt and query images, we propose a soft feature alignment module that bridges paired-image change perception and single-image semantic segmentation. This is the first work to achieve universal anomaly segmentation using a pure vision model without relying on special anomaly detection datasets and pre-trained visual-language models. Our method effectively and efficiently segments any anomalies with only one normal image prompt and enjoys training-free without guidance from language. Our MetaUAS significantly outperforms previous zero-shot, few-shot, and even full-shot anomaly segmentation methods. The code and pre-trained models are available at https://github.com/gaobb/MetaUAS.
零样本和少样本视觉异常分割依赖于强大的视觉语言模型,这些模型使用手动设计的文本提示来检测未见过的异常。然而,视觉表示本质上独立于语言。本文中,我们探索了纯视觉基础模型作为广泛使用的视觉语言模型的替代方案,用于通用视觉异常分割的潜力。我们提出了一种将异常分割统一为变化分割的新范式。这种范式使我们能够利用从现有图像数据集派生的对象级别和局部区域变化的大规模合成图像对,这些图像与目标异常数据集无关。我们提出了一个用于通用异常分割的一提示元学习框架(MetaUAS),该框架在这个合成数据集上进行训练,然后很好地推广到现实世界中任何新型或未见过的视觉异常的分割。为了处理提示图像和查询图像之间的几何变化,我们提出了一个软特征对齐模块,该模块桥梁了配对图像变化感知和单图像语义分割。这是第一项工作,使用纯视觉模型实现通用异常分割,不依赖于特殊的异常检测数据集和预训练的视觉语言模型。我们的方法有效且高效地将任何异常仅与一个正常图像提示进行分割,并且无需语言的指导即可实现无训练。我们的MetaUAS显著优于以前的零样本、少样本甚至是全样本的异常分割方法。代码和预先训练好的模型可在https://github.com/gaobb/MetaUAS获得。
论文及项目相关链接
PDF Accepted by NeurIPS 2024
Summary
视觉异常分割零样本和少样本依赖于强大的视觉语言模型,使用手动设计的文本提示来检测未见的异常。本文探索了纯视觉基础模型作为广泛使用的视觉语言模型的替代方案,用于通用视觉异常分割的潜力。我们提出了一种将异常分割统一为变化分割的新范式。此范式使我们能够利用从现有图像数据集衍生出的大规模合成图像对,这些图像对具有对象级别和局部区域变化的特点,独立于目标异常数据集。我们提出了一种用于通用异常分割的一提示元学习框架(MetaUAS),该框架在这个合成数据集上进行训练,然后很好地推广到分割现实世界中的任何新型或未见过的视觉异常。为了处理提示图像和查询图像之间的几何变化,我们提出了一个软特征对齐模块,该模块桥接了配对图像的变化感知和单图像语义分割。这是第一项工作,使用纯视觉模型实现通用异常分割,无需依赖特定的异常检测数据集和预训练的视觉语言模型。我们的方法有效且高效地将任何异常与单一的正常图像提示进行分割,无需语言的指导即可实现无训练。MetaUAS显著优于先前的零样本、少样本甚至全样本异常分割方法。
Key Takeaways
- 论文探讨了零样本和少样本视觉异常分割中视觉语言模型的局限性,并提倡使用纯视觉基础模型作为替代方案。
- 提出了一种新的范式,将异常分割统一为变化分割,利用合成图像对进行训练。
- 引入了MetaUAS框架,能在仅有单一正常图像提示的情况下有效地分割任何异常,无需特定的异常检测数据集或预训练的视觉语言模型。
- 通过软特征对齐模块解决了提示图像和查询图像间的几何变化问题。
- 此方法具有出色的性能,显著优于现有的异常分割方法。
- 研究成果已经开源供公众使用,并附有预训练模型和代码。
点此查看论文截图
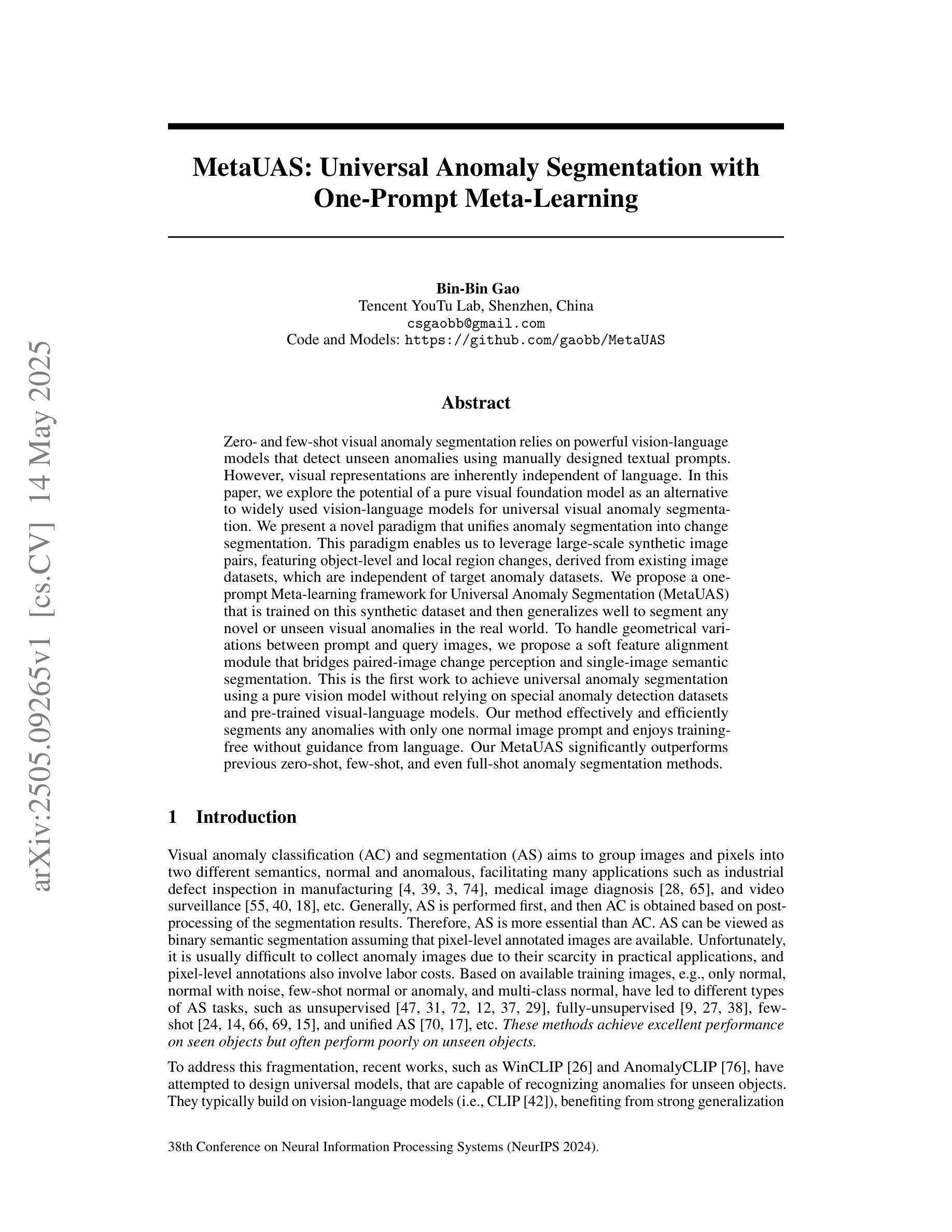
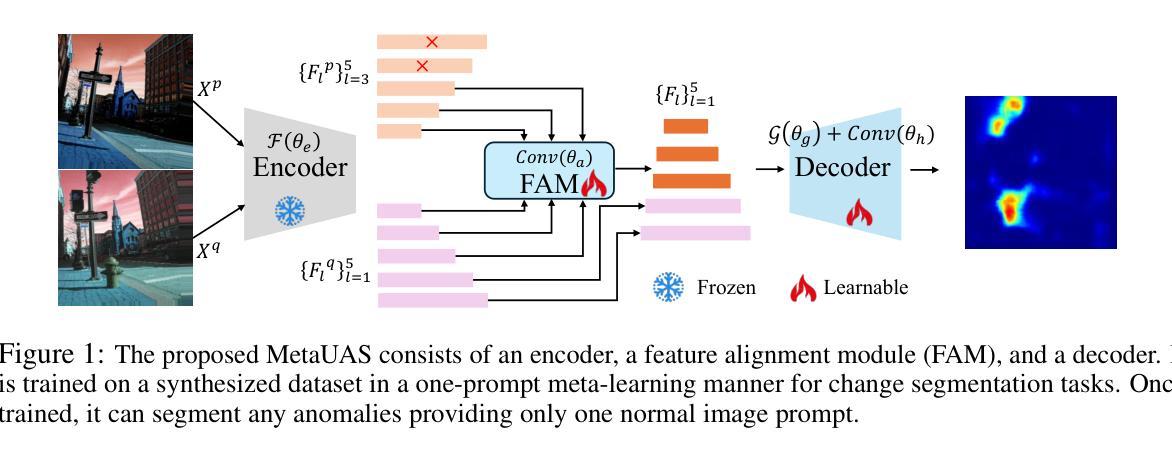
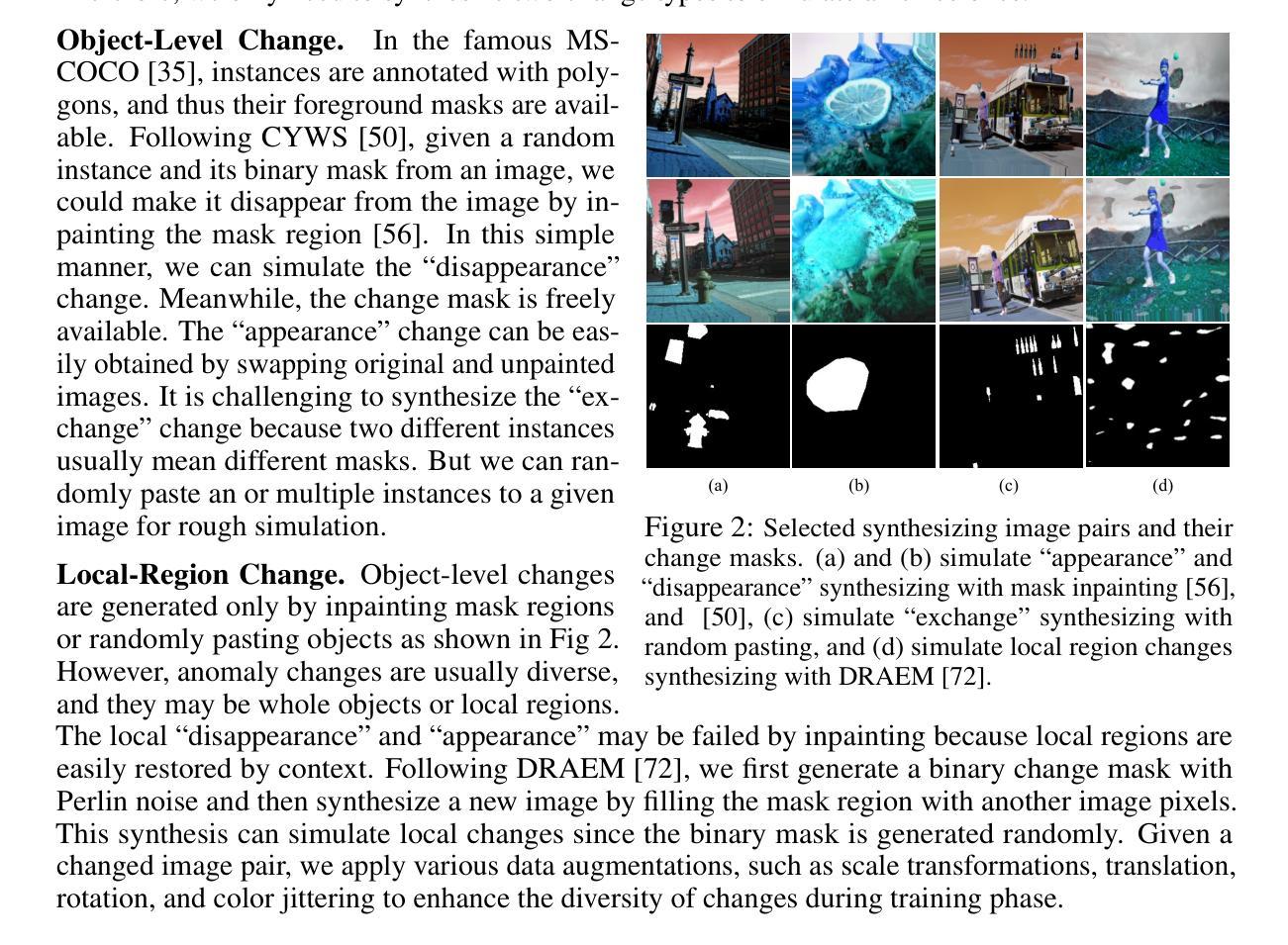
Ultrasound Report Generation with Multimodal Large Language Models for Standardized Texts
Authors:Peixuan Ge, Tongkun Su, Faqin Lv, Baoliang Zhao, Peng Zhang, Chi Hong Wong, Liang Yao, Yu Sun, Zenan Wang, Pak Kin Wong, Ying Hu
Ultrasound (US) report generation is a challenging task due to the variability of US images, operator dependence, and the need for standardized text. Unlike X-ray and CT, US imaging lacks consistent datasets, making automation difficult. In this study, we propose a unified framework for multi-organ and multilingual US report generation, integrating fragment-based multilingual training and leveraging the standardized nature of US reports. By aligning modular text fragments with diverse imaging data and curating a bilingual English-Chinese dataset, the method achieves consistent and clinically accurate text generation across organ sites and languages. Fine-tuning with selective unfreezing of the vision transformer (ViT) further improves text-image alignment. Compared to the previous state-of-the-art KMVE method, our approach achieves relative gains of about 2% in BLEU scores, approximately 3% in ROUGE-L, and about 15% in CIDEr, while significantly reducing errors such as missing or incorrect content. By unifying multi-organ and multi-language report generation into a single, scalable framework, this work demonstrates strong potential for real-world clinical workflows.
超声(US)报告生成是一项具有挑战性的任务,因为超声图像具有多样性、操作依赖性,以及需要标准化文本。不同于X射线和CT,超声成像缺乏一致的数据集,使得自动化变得困难。在这项研究中,我们提出了一个统一的框架,用于多器官和多语言的超声报告生成,该框架结合了基于片段的多语言训练和利用超声报告的标准化特性。通过模块化文本片段与多样化的成像数据对齐,并创建双语英文-中文数据集,该方法实现了跨器官部位和语言的文本生成的连贯性和准确性。通过选择性解冻视觉变压器(ViT)进行微调,进一步提高了文本与图像的对齐。与之前最先进的KMVE方法相比,我们的方法在BLEU得分上实现了约2%的相对增益,在ROUGE-L上大约提高了3%,在CIDEr上提高了约15%,同时显著减少了内容缺失或错误等错误。通过将多器官和多语言报告生成统一到一个可扩展的框架中,这项工作展示了在实际临床工作流程中的强大潜力。
论文及项目相关链接
Summary
本文主要研究了针对超声报告生成的任务,提出了一个多器官、多语言的统一框架。该研究通过基于片段的多语言训练和利用超声报告的标准性质,实现了跨器官部位和语言的文本生成。通过选择性微调视觉变压器(ViT),进一步提高了文本与图像的匹配度。相较于之前的先进方法KMVE,该研究的方法在BLEU分数、ROUGE-L和CIDEr方面取得了相对增益,并显著减少了内容缺失或错误的情况。此研究展示了该框架在实际临床工作流程中的强大潜力。
Key Takeaways
- 该研究针对超声报告生成提出了一个多器官、多语言的统一框架。
- 通过基于片段的多语言训练,实现了文本的标准化生成。
- 利用超声报告的标准性质,该框架可以生成一致的、临床准确的文本。
- 通过选择性微调视觉变压器(ViT),提高了文本与图像的匹配度。
- 与之前的先进方法相比,该研究的方法在评估指标上取得了相对增益。
- 该框架显著减少了报告内容缺失或错误的情况。
点此查看论文截图
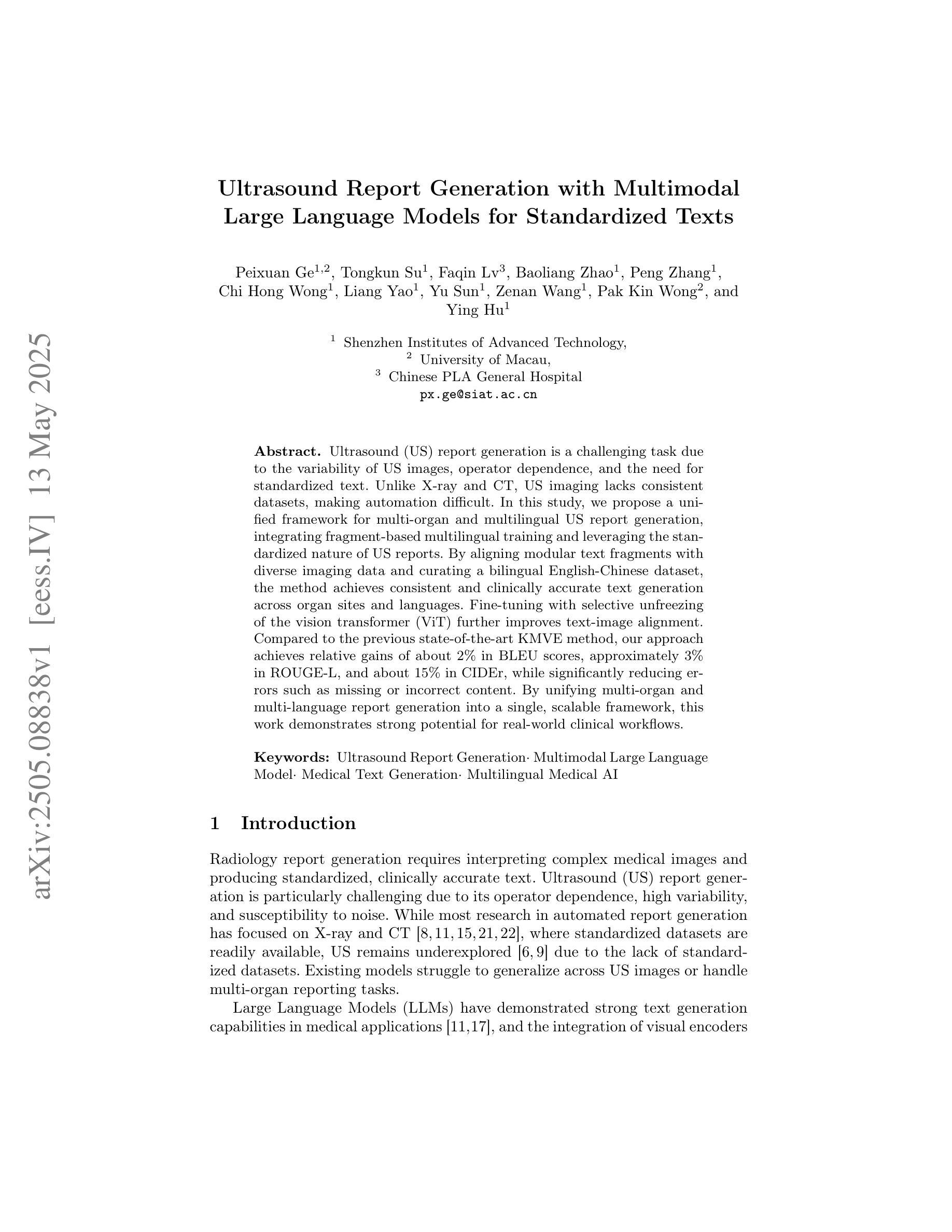
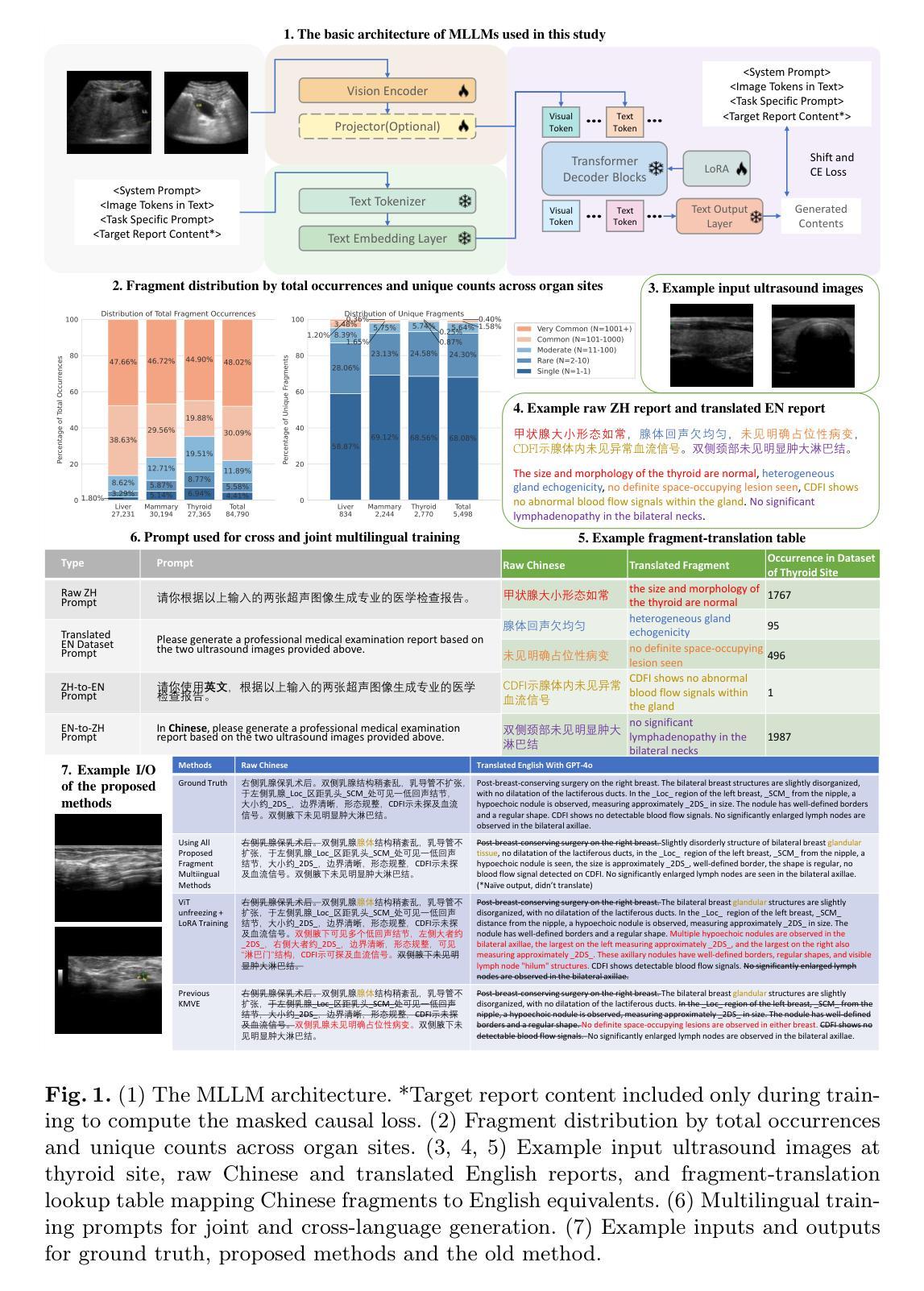
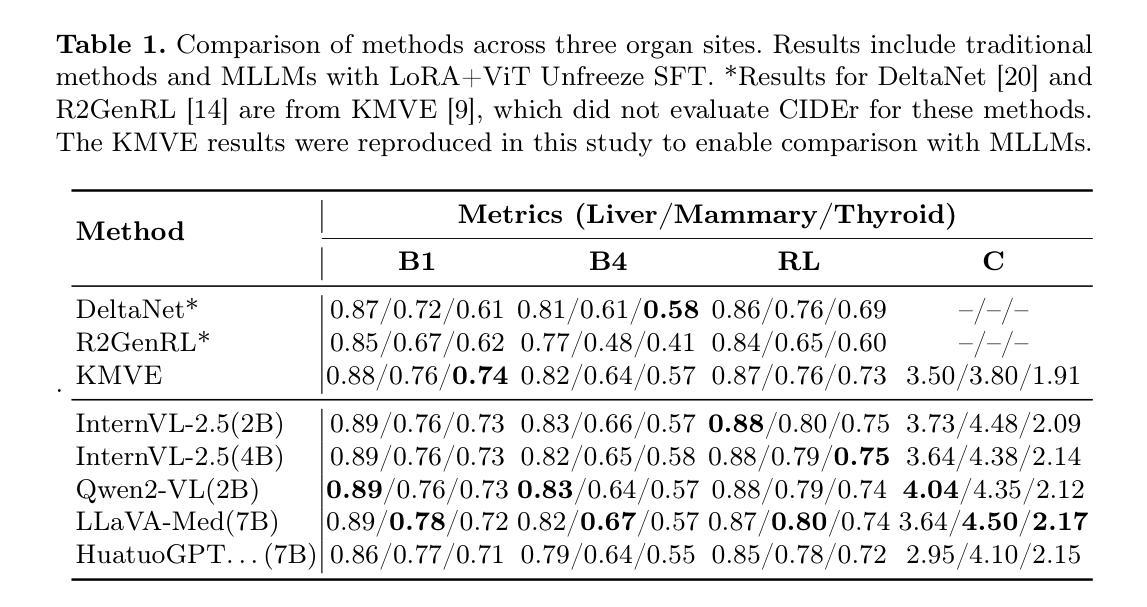