⚠️ 以下所有内容总结都来自于 大语言模型的能力,如有错误,仅供参考,谨慎使用
🔴 请注意:千万不要用于严肃的学术场景,只能用于论文阅读前的初筛!
💗 如果您觉得我们的项目对您有帮助 ChatPaperFree ,还请您给我们一些鼓励!⭐️ HuggingFace免费体验
2025-05-17 更新
Introducing voice timbre attribute detection
Authors:Jinghao He, Zhengyan Sheng, Liping Chen, Kong Aik Lee, Zhen-Hua Ling
This paper focuses on explaining the timbre conveyed by speech signals and introduces a task termed voice timbre attribute detection (vTAD). In this task, voice timbre is explained with a set of sensory attributes describing its human perception. A pair of speech utterances is processed, and their intensity is compared in a designated timbre descriptor. Moreover, a framework is proposed, which is built upon the speaker embeddings extracted from the speech utterances. The investigation is conducted on the VCTK-RVA dataset. Experimental examinations on the ECAPA-TDNN and FACodec speaker encoders demonstrated that: 1) the ECAPA-TDNN speaker encoder was more capable in the seen scenario, where the testing speakers were included in the training set; 2) the FACodec speaker encoder was superior in the unseen scenario, where the testing speakers were not part of the training, indicating enhanced generalization capability. The VCTK-RVA dataset and open-source code are available on the website https://github.com/vTAD2025-Challenge/vTAD.
本文重点解释语音信号所传递的音色,并介绍了一项称为音色属性检测(vTAD)的任务。在该任务中,音色通过一系列描述人类感知的感官属性来解释。对一对语音片段进行处理,并在指定的音色描述符中比较它们的强度。此外,提出了一个基于从语音片段中提取的说话者嵌入的框架。该研究在VCTK-RVA数据集上进行。对ECAPA-TDNN和FACodec说话人编码器的实验表明:1)在可见场景下,ECAPA-TDNN说话人编码器更具能力,其中测试说话人包含在训练集中;2)FACodec说话人编码器在未见过的情况下表现更好,其中测试说话人不是训练的一部分,表明其增强了泛化能力。VCTK-RVA数据集和开源代码可在网站https://github.com/vTAD2025-Challenge/vTAD上找到。
论文及项目相关链接
总结
本文介绍了语音信号所传递的音色,并提出了声音音色属性检测(vTAD)任务。该任务通过一组描述人类感知的感官属性来解释声音音色。对一对语音发音进行处理,并在指定的音色描述符中比较其强度。此外,提出了一个基于从语音发音中提取的说话者嵌入的框架。在VCTK-RVA数据集上进行调查,通过对ECAPA-TDNN和FACodec说话人编码器的实验考察发现:1)ECAPA-TDNN说话人编码器在测试说话人包含在训练集中的情况下表现更好;2)FACodec说话人编码器在测试说话人未参与训练的情况下表现更优,显示出增强的泛化能力。VCTK-RVA数据集和开源代码可在网站https://github.com/vTAD2025-Challenge/vTAD上找到。
关键见解
- 论文介绍了声音音色属性检测(vTAD)任务,该任务旨在通过一系列感官属性来解释声音的音色特征。
- 在对比研究中使用了两种主流的说话人编码器:ECAPA-TDNN和FACodec。
- 实验发现ECAPA-TDNN在已知说话人的场景下表现更好,适合用于识别已训练的说话人群体。
- FACodec在未知说话人的场景下表现优越,显示出更强的泛化能力,适用于识别未参与训练的说话人。
- VCTK-RVA数据集用于实验考察,为语音音色研究提供了丰富的数据资源。
- 开源代码及数据集资源链接提供,便于后续研究者进行进一步的探索和研究。
- vTAD任务的研究对于语音识别、情感分析和语音合成等语音领域应用具有重要意义。
点此查看论文截图
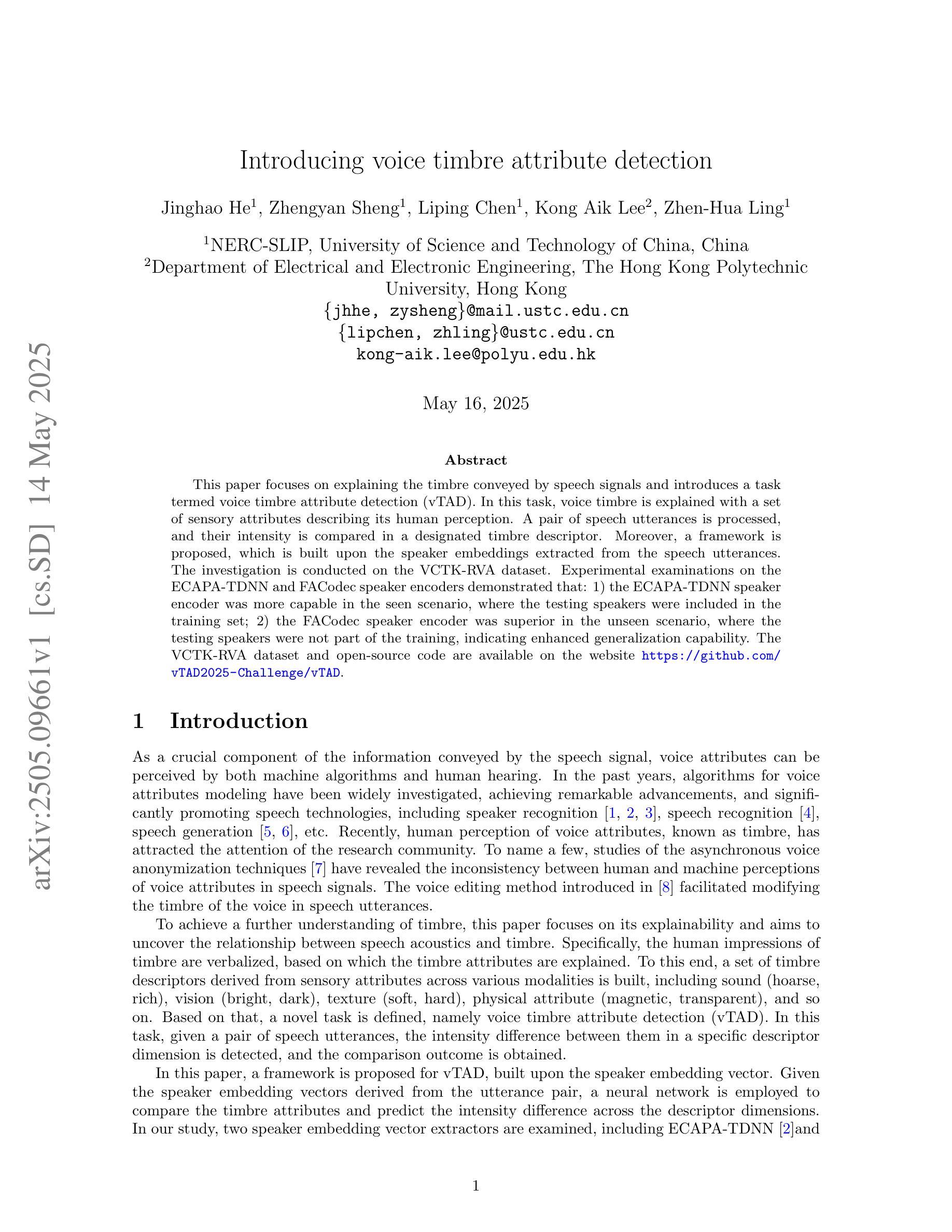
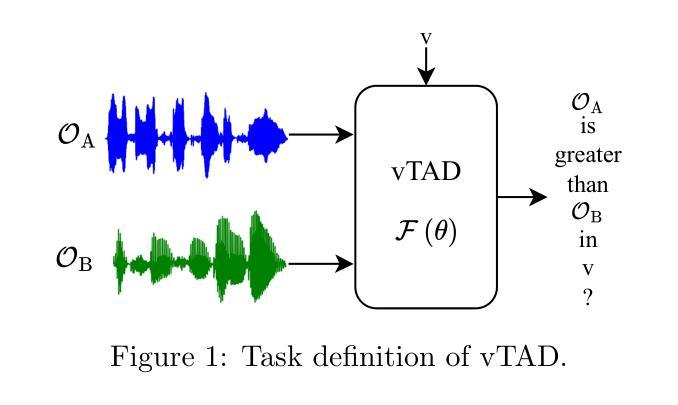
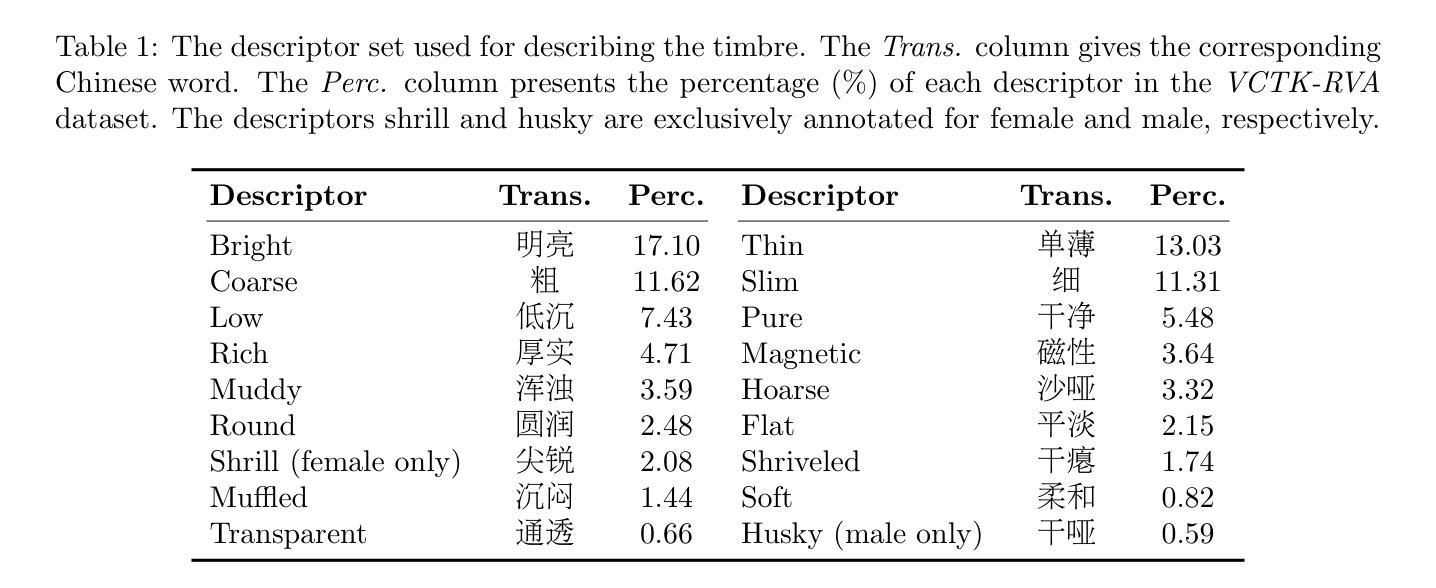
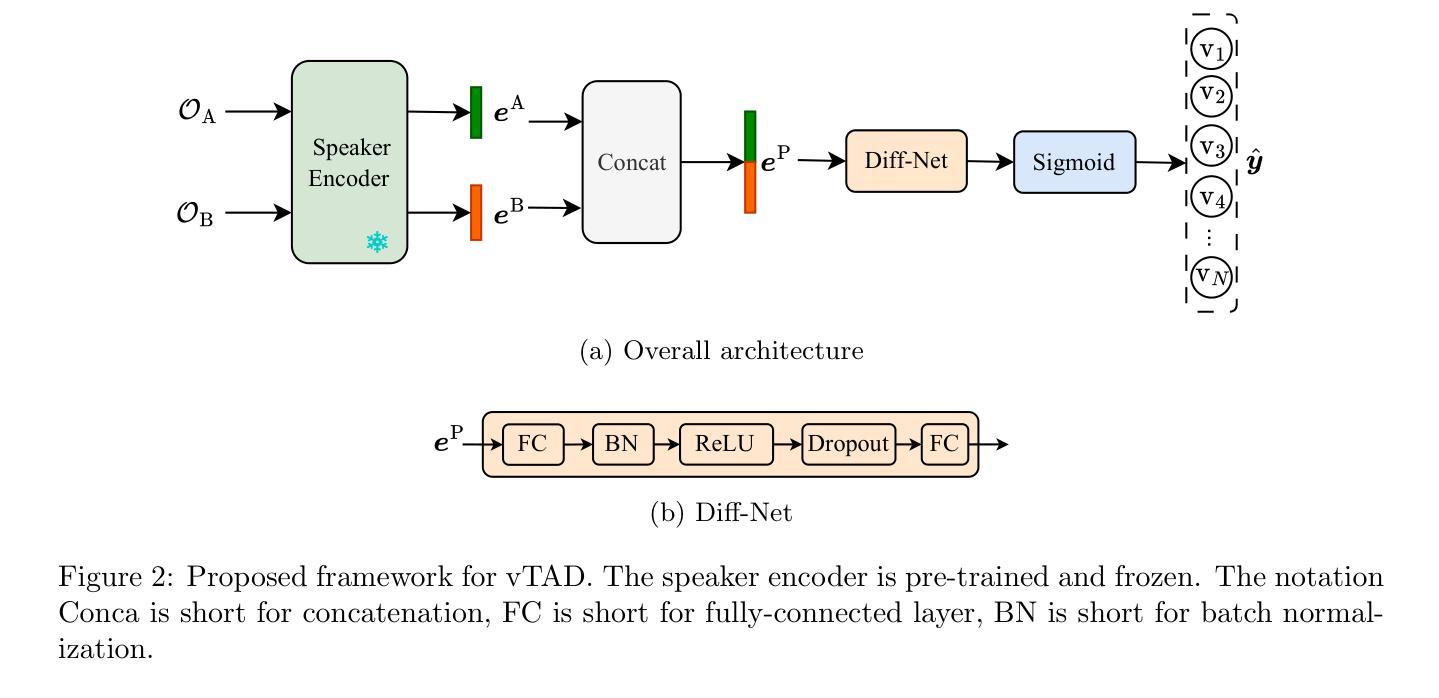
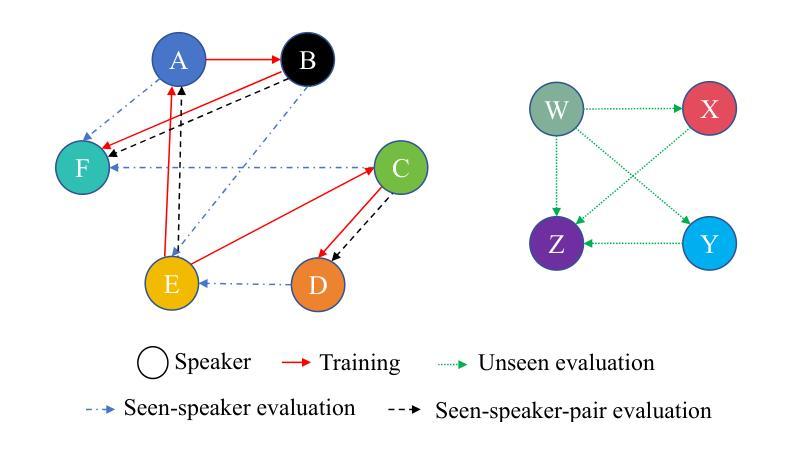
CoGenAV: Versatile Audio-Visual Representation Learning via Contrastive-Generative Synchronization
Authors:Detao Bai, Zhiheng Ma, Xihan Wei, Liefeng Bo
The inherent synchronization between a speaker’s lip movements, voice, and the underlying linguistic content offers a rich source of information for improving speech processing tasks, especially in challenging conditions where traditional audio-only systems falter. We introduce CoGenAV, a powerful and data-efficient model designed to learn versatile audio-visual representations applicable across a wide range of speech and audio-visual tasks. CoGenAV is trained by optimizing a dual objective derived from natural audio-visual synchrony, contrastive feature alignment and generative text prediction, using only 223 hours of labeled data from the LRS2 dataset. This contrastive-generative synchronization strategy effectively captures fundamental cross-modal correlations. We showcase the effectiveness and versatility of the learned CoGenAV representations on multiple benchmarks. When utilized for Audio-Visual Speech Recognition (AVSR) on LRS2, these representations contribute to achieving a state-of-the-art Word Error Rate (WER) of 1.27. They also enable strong performance in Visual Speech Recognition (VSR) with a WER of 20.5 on LRS2, and significantly improve performance in noisy environments by over 70%. Furthermore, CoGenAV representations benefit speech reconstruction tasks, boosting performance in Speech Enhancement and Separation, and achieve competitive results in audio-visual synchronization tasks like Active Speaker Detection (ASD). Our model will be open-sourced to facilitate further development and collaboration within both academia and industry.
说话者的嘴唇动作、声音与底层语言内容之间的固有同步性,为改进语音识别任务提供了丰富的信息来源,特别是在传统仅依赖音频的系统表现不佳的困难条件下。我们引入了CoGenAV,这是一个强大且数据高效的模型,旨在学习适用于各种语音和视听任务的通用视听表示。CoGenAV通过优化从自然视听同步中派生的双重目标、对比特征对齐和生成文本预测来进行训练,仅使用LRS2数据集的223小时标记数据进行训练。这种对比生成同步策略有效地捕捉了跨模式的基本相关性。我们在多个基准测试上展示了CoGenAV表示的有效性和通用性。在LRS2上的视听语音识别(AVSR)中利用CoGenAV表示,取得了词错误率(WER)达到1.27的最新水平。在LRS2的视觉语音识别(VSR)中,其WER达到了20.5,并且在噪声环境中的性能提高了70%以上。此外,CoGenAV表示对于语音重建任务有益,提高了语音增强和分离的性能,并在视听同步任务如活跃说话者检测(ASD)上取得了有竞争力的结果。我们的模型将开源,以促进学术界和工业界的进一步发展和合作。
论文及项目相关链接
Summary
本文介绍了CoGenAV模型,该模型通过优化自然视听同步、对比特征对齐和生成文本预测的双目标,学习适用于多种语音和视听任务的视听表示。CoGenAV模型使用LRS2数据集仅223小时的标记数据进行训练,采用对比生成同步策略,有效捕捉跨模态关联。在多个基准测试中,验证了CoGenAV表示的有效性、通用性,对于视听语音识别任务在LRS2数据集上实现词错误率为1.27的业界领先水平。此外,在噪声环境下性能提升超过70%,并有助于语音重建任务以及音频视觉同步任务,如主动说话人检测等。我们的模型将开源,以促进学术界和工业界的进一步发展和合作。
Key Takeaways
- CoGenAV模型利用视听信息的同步性,为改进语音处理任务提供了丰富的信息来源。
- 该模型通过优化自然视听同步、对比特征对齐和生成文本预测的双目标来训练数据高效的多功能模型。
- 仅使用LRS2数据集的223小时标记数据进行训练,对比生成同步策略有助于捕捉跨模态关联。
- CoGenAV表示在多个基准测试中表现出优异性能,实现了先进的语音识别效果。
- 在噪声环境下性能显著提升超过70%,证明了其强大的稳健性。
- CoGenAV表示对语音重建任务有益,如语音增强和分离等。
点此查看论文截图
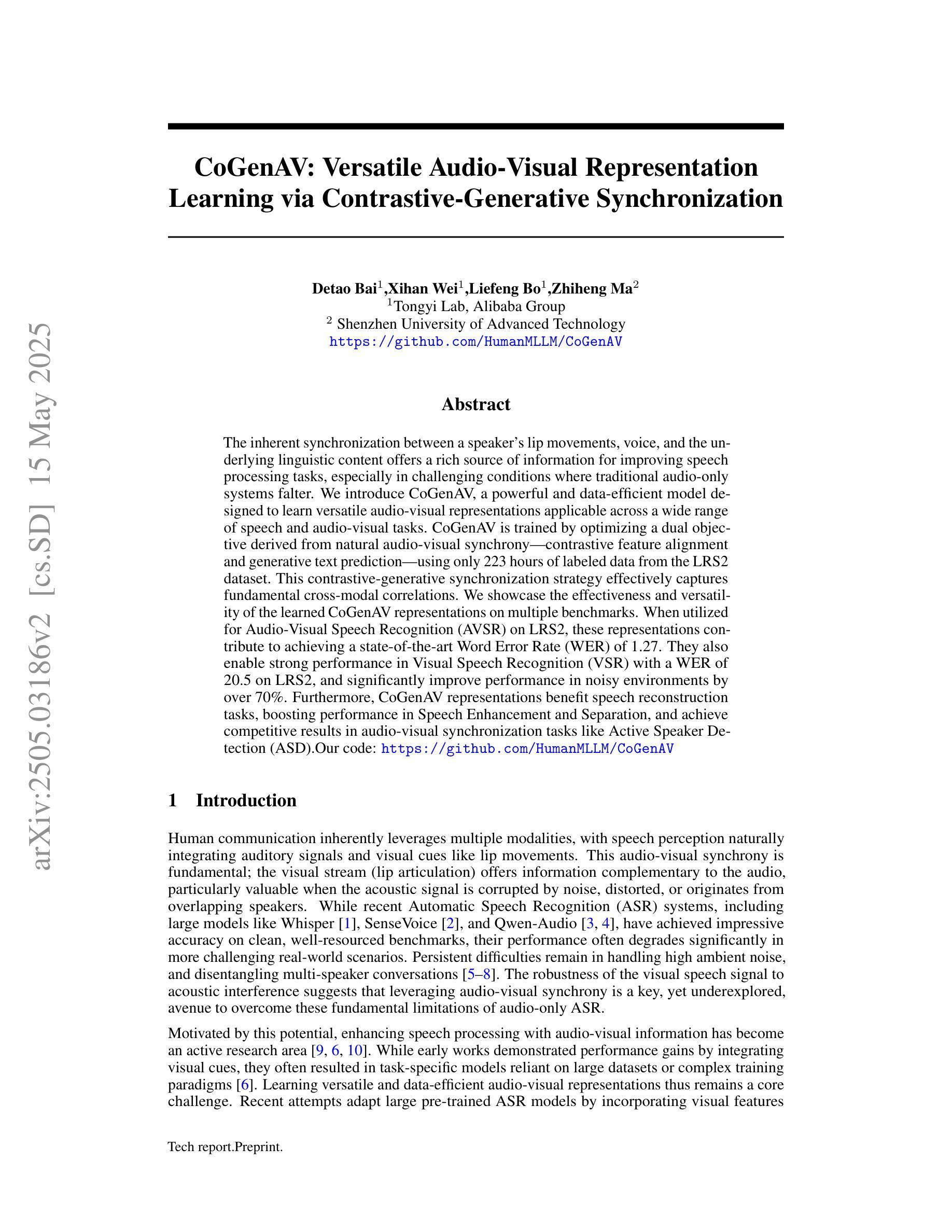
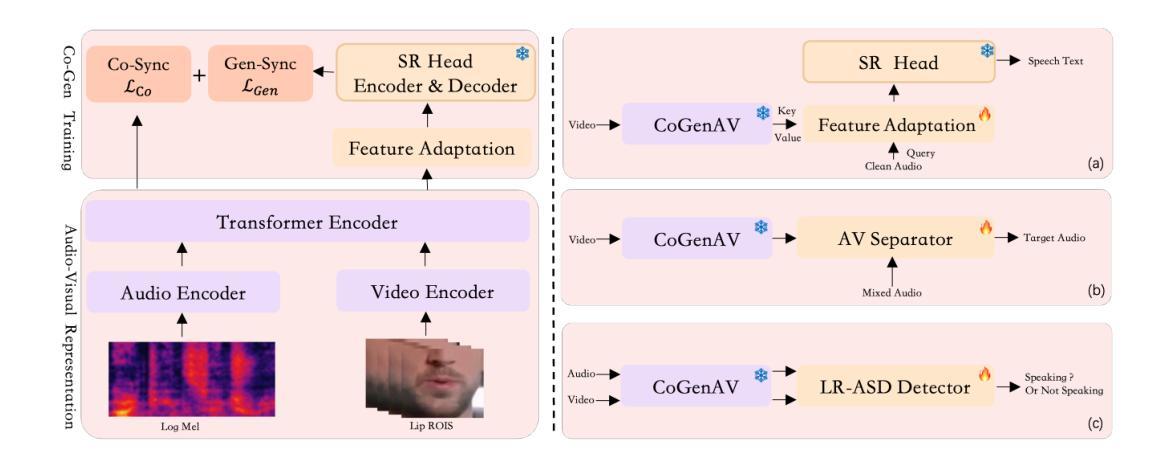
MultiMed: Multilingual Medical Speech Recognition via Attention Encoder Decoder
Authors:Khai Le-Duc, Phuc Phan, Tan-Hanh Pham, Bach Phan Tat, Minh-Huong Ngo, Chris Ngo, Thanh Nguyen-Tang, Truong-Son Hy
Multilingual automatic speech recognition (ASR) in the medical domain serves as a foundational task for various downstream applications such as speech translation, spoken language understanding, and voice-activated assistants. This technology improves patient care by enabling efficient communication across language barriers, alleviating specialized workforce shortages, and facilitating improved diagnosis and treatment, particularly during pandemics. In this work, we introduce MultiMed, the first multilingual medical ASR dataset, along with the first collection of small-to-large end-to-end medical ASR models, spanning five languages: Vietnamese, English, German, French, and Mandarin Chinese. To our best knowledge, MultiMed stands as the world’s largest medical ASR dataset across all major benchmarks: total duration, number of recording conditions, number of accents, and number of speaking roles. Furthermore, we present the first multilinguality study for medical ASR, which includes reproducible empirical baselines, a monolinguality-multilinguality analysis, Attention Encoder Decoder (AED) vs Hybrid comparative study and a linguistic analysis. We present practical ASR end-to-end training schemes optimized for a fixed number of trainable parameters that are common in industry settings. All code, data, and models are available online: https://github.com/leduckhai/MultiMed/tree/master/MultiMed.
在医疗领域,多语言自动语音识别(ASR)作为各种下游应用的基础任务,如语音识别翻译、口语理解和语音助手等。通过消除语言障碍、缓解专业劳动力短缺和促进诊断与治疗改善,特别是疫情期间,该技术提升了患者的护理质量。在这项工作中,我们介绍了MultiMed,首个多语言医疗ASR数据集,以及涵盖越南语、英语、德语、法语和普通话的端到端小型至大型医疗ASR模型集合。据我们所知,MultiMed在所有主要基准测试方面都是全球最大的医疗ASR数据集:总时长、记录条件数量、口音数量和说话角色数量。此外,我们对医疗ASR进行了首次多语言性研究,包括可重复使用的实证基准、单语-多语分析、注意力编码器解码器(AED)与混合技术的比较研究以及语言学分析。我们提出了适用于工业环境中常见固定可训练参数数量的实用ASR端到端训练方案。所有代码、数据和模型均可在网上找到:https://github.com/leduckhai/MultiMed/tree/master/MultiMed。
论文及项目相关链接
PDF ACL 2025, 38 pages
Summary
本文介绍了医疗领域中的多语言自动语音识别(ASR)技术及其重要性。研究人员推出了MultiMed数据集,包含五种语言的医疗ASR模型。该数据集是目前全球最大的医疗ASR数据集,涵盖了多种录音条件、口音和说话角色。此外,文章还进行了多语言性研究,包括可复现的实证基准、单语言与多语言分析、AED与Hybrid的比较分析以及语言分析。同时,提出了针对工业环境中常见固定参数数量的实用ASR端到端训练方案。
Key Takeaways
- 医疗领域中的多语言自动语音识别(ASR)对于多种下游应用如语音翻译、自然语言理解和语音助手至关重要。
- MultiMed数据集是首个多语言医疗ASR数据集,涵盖越南语、英语、德语、法语和普通话五种语言。
- MultiMed数据集是目前全球最大的医疗ASR数据集,包含多种录音条件、口音和说话角色。
- 文章进行了关于医疗ASR的多语言性研究,包括实证基准、单语言与多语言分析、AED与Hybrid模型的比较分析以及语言分析。
- 提出了针对工业环境的实用ASR端到端训练方案,这些方案针对固定数量的可训练参数进行优化。
- 所有代码、数据和模型均可在网上找到。
点此查看论文截图
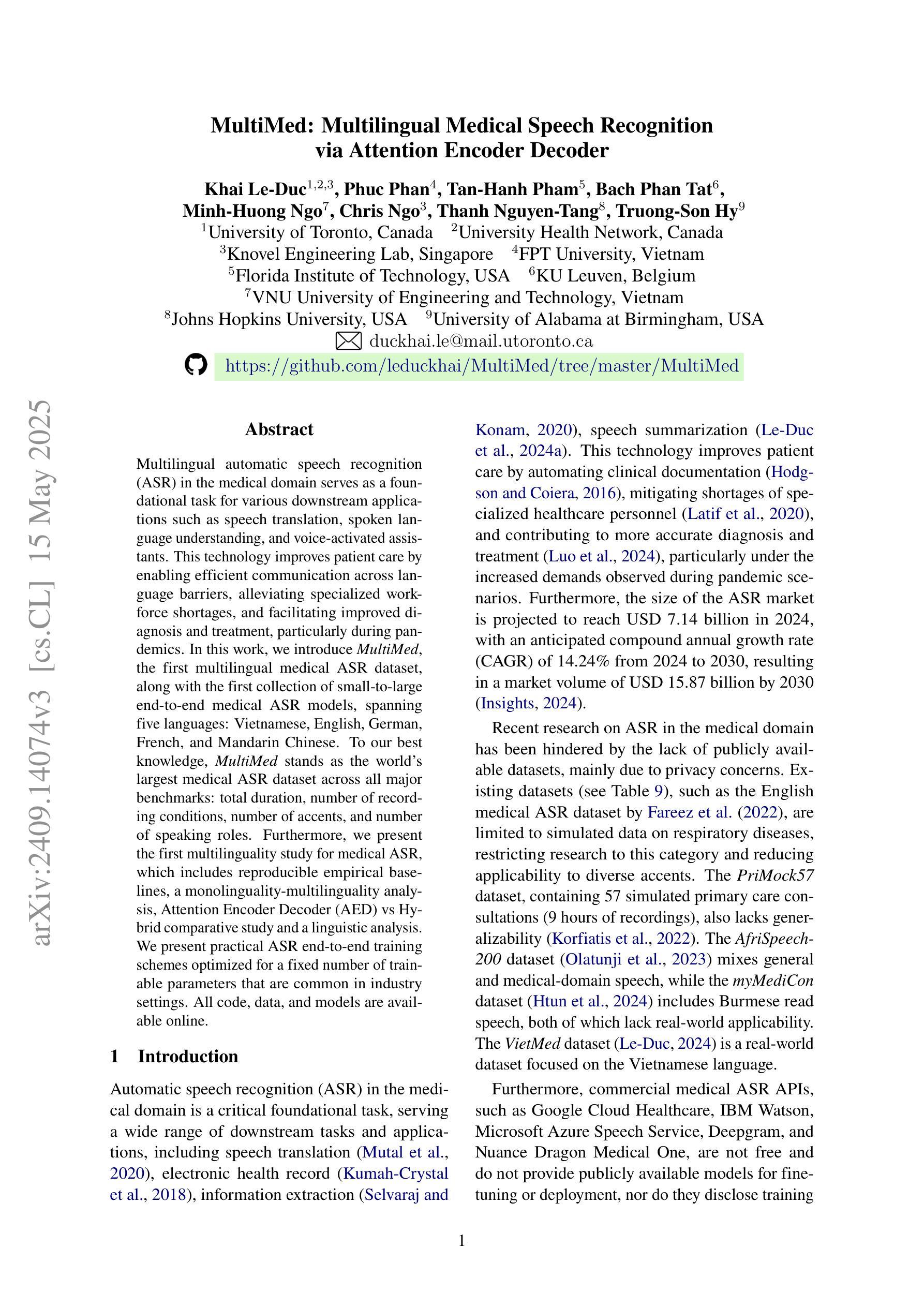
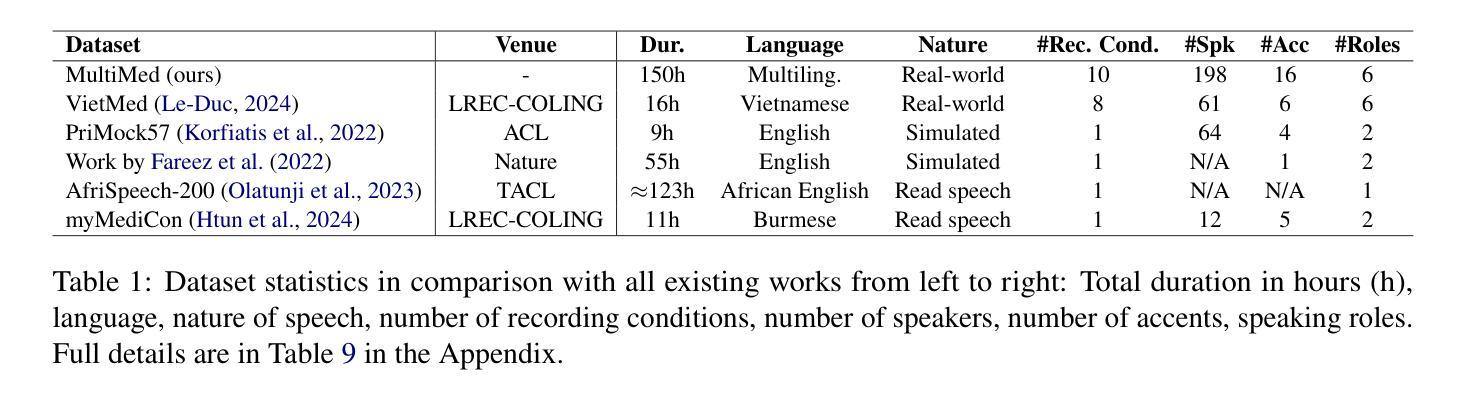
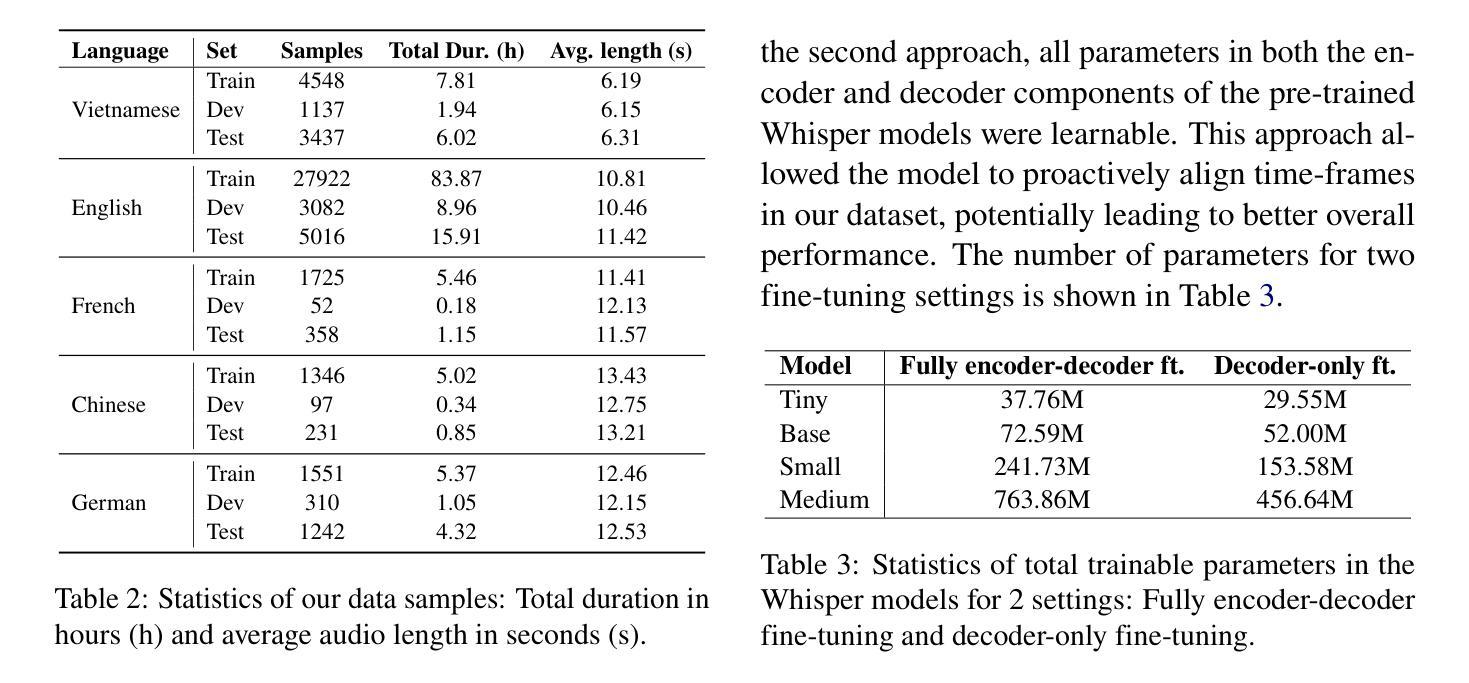
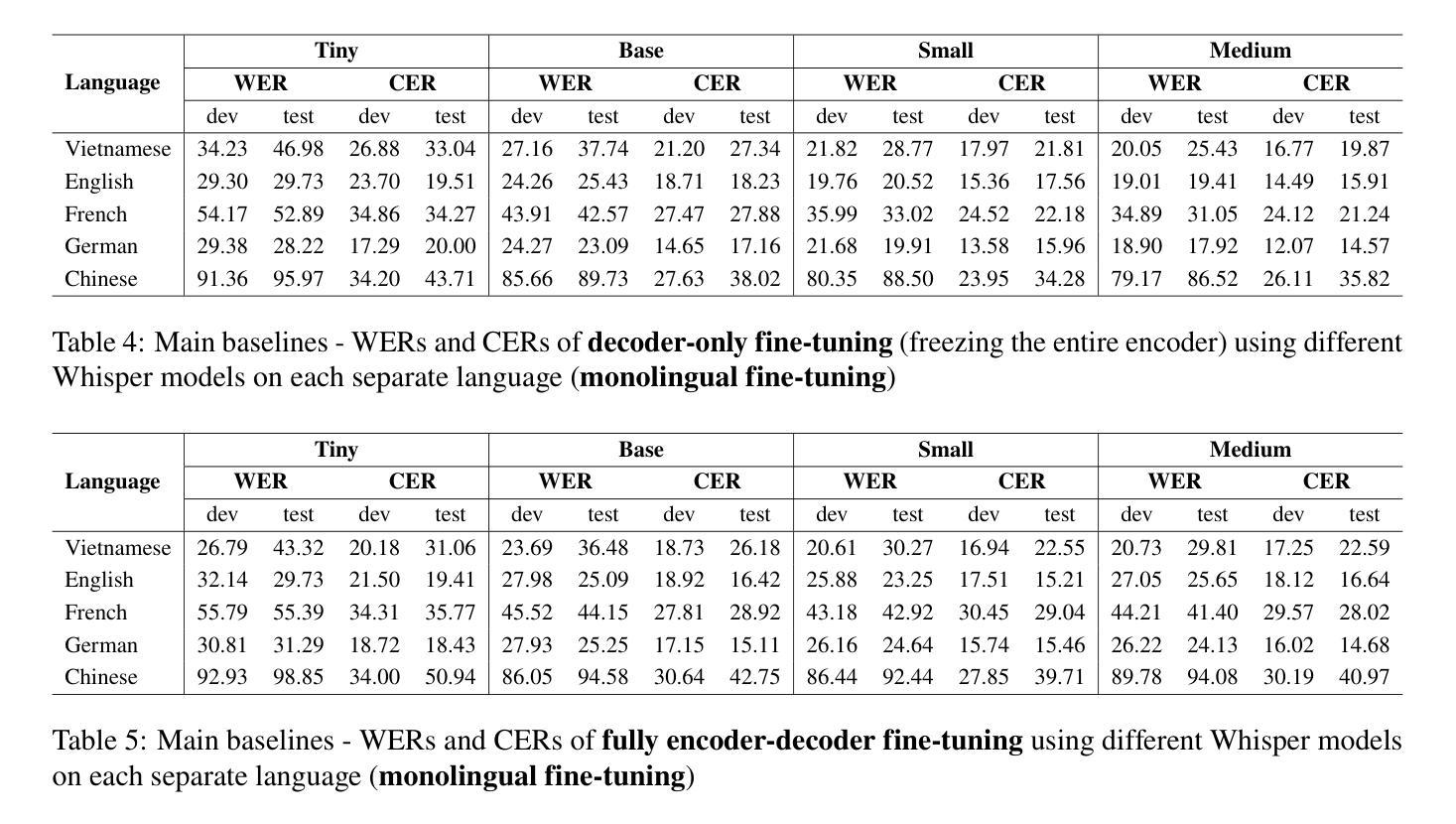
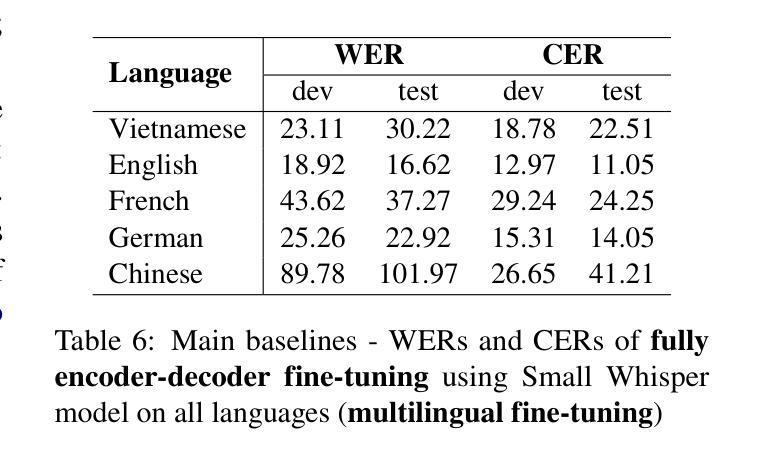
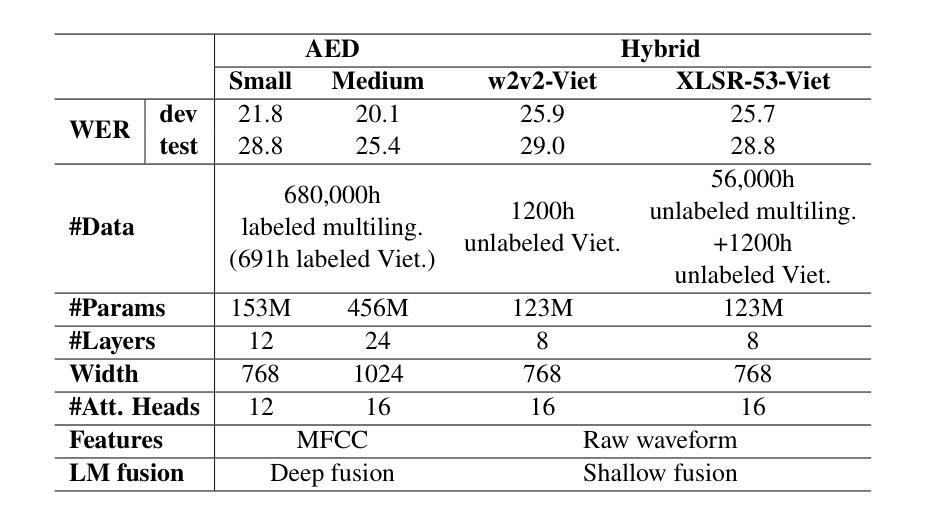
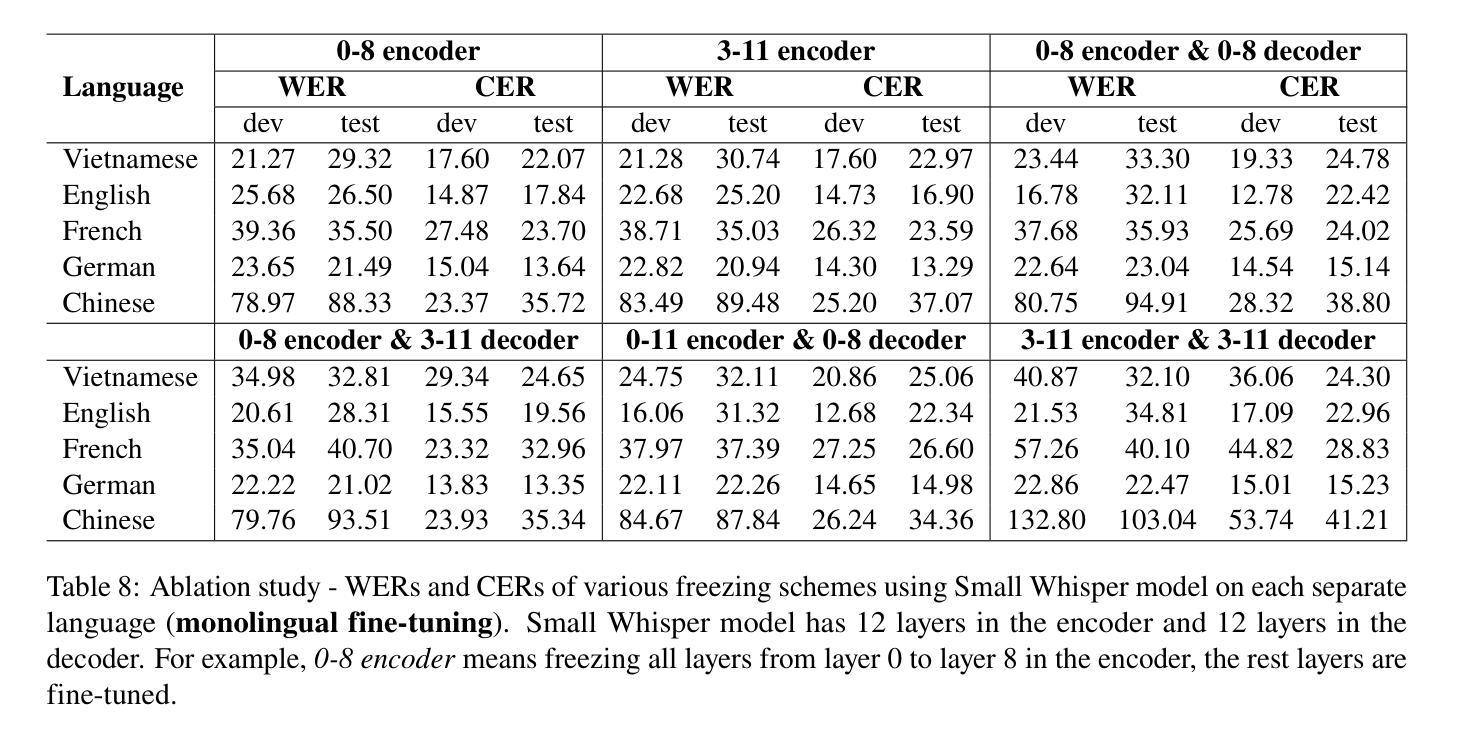