⚠️ 以下所有内容总结都来自于 大语言模型的能力,如有错误,仅供参考,谨慎使用
🔴 请注意:千万不要用于严肃的学术场景,只能用于论文阅读前的初筛!
💗 如果您觉得我们的项目对您有帮助 ChatPaperFree ,还请您给我们一些鼓励!⭐️ HuggingFace免费体验
2025-05-20 更新
VideoHallu: Evaluating and Mitigating Multi-modal Hallucinations on Synthetic Video Understanding
Authors:Zongxia Li, Xiyang Wu, Guangyao Shi, Yubin Qin, Hongyang Du, Tianyi Zhou, Dinesh Manocha, Jordan Lee Boyd-Graber
Synthetic video generation has gained significant attention for its realism and broad applications, but remains prone to violations of common sense and physical laws. This highlights the need for reliable abnormality detectors that understand such principles and are robust to hallucinations. To address this, we introduce VideoHallu, a benchmark of over 3,000 video QA pairs built from synthetic videos generated by models like Veo2, Sora, and Kling, paired with expert-crafted counterintuitive QA to evaluate the critical thinking abilities of Multi-modal Large Language Models (MLLMs) on abnormalities that are perceptually obvious to humans but often hallucinated due to language priors. VideoHallu evaluates MLLMs’ abnormality detection abilities with examples across alignment, consistency, commonsense, and physics. We benchmark SOTA MLLMs, including GPT-4o, Gemini-2.5-Pro, Qwen2.5-VL, Video-R1, and VideoChat-R1. We observe that these models perform well on many real-world benchmarks like MVBench and MovieChat, but still struggle with basic physics-based and commonsense reasoning in synthetic videos. We further show that post-training with Group Relative Policy Optimization (GRPO), using curriculum learning on datasets combining video QA with counterintuitive commonsense and physics reasoning over real and synthetic videos, improves MLLMs’ abnormality detection and critical thinking, demonstrating the value of targeted training for improving their understanding of commonsense and physical laws.
视频生成技术因其真实感和广泛应用而备受关注,但仍存在违反常识和物理定律的问题。这凸显了需要可靠的异常检测器,这些检测器需要理解这些原则,并对幻觉具有鲁棒性。针对这一问题,我们推出了VideoHallu,这是一个由超过3000个视频问答对组成的基准测试集,这些问答对是通过Veo2、Sora和Kling等模型生成的合成视频与专家制作的反直觉问答所构建的,旨在评估多模态大型语言模型(MLLMs)在异常检测方面的批判性思维能力,这些异常对人类来说是显而易见的,但由于语言先验知识往往会产生幻觉。VideoHallu通过示例评估MLLMs在对齐、一致性、常识和物理学方面的异常检测能力。我们对包括GPT-4o、Gemini-2.5-Pro、Qwen2.5-VL、Video-R1和VideoChat-R1在内的最新MLLMs进行了基准测试。我们发现这些模型在MVBench和MovieChat等现实世界的基准测试中表现良好,但在合成视频的基于物理和常识的推理方面仍存在困难。我们进一步表明,使用组合视频问答与反直觉常识和物理推理的数据集进行课程学习的集团相对策略优化(GRPO)后训练,可以改善MLLMs的异常检测和批判性思维,证明了有针对性的训练在提高其对常识和物理定律的理解方面的价值。
论文及项目相关链接
Summary
本文介绍了合成视频生成技术的现状与应用挑战,强调了对异常检测器的需求。为此,推出了VideoHallu基准测试,包含超过3000个视频问答对,旨在评估多模态大型语言模型在异常检测方面的能力。文章还探讨了当前顶尖模型在合成视频中的表现,并提出了通过课程学习及集团相对策略优化方法改进模型在异常检测和批判性思维方面的表现。
Key Takeaways
- 合成视频生成技术受到关注,但需应对常识和物理定律的违反问题。
- VideoHallu基准测试旨在评估多模态大型语言模型在异常检测方面的能力,包含超过3000个视频问答对。
- 当前顶尖模型在合成视频中的表现存在挑战,尤其在常识和物理推理方面。
- 多模态大型语言模型在真实世界基准测试中表现良好,但仍需改进在合成视频中的异常检测和批判性思维能力。
- 通过课程学习结合视频问答和具有挑战性的常识与物理推理数据集进行针对性训练,可提高模型的异常检测能力。
- Group Relative Policy Optimization(GRPO)方法有助于改进模型的性能。
点此查看论文截图
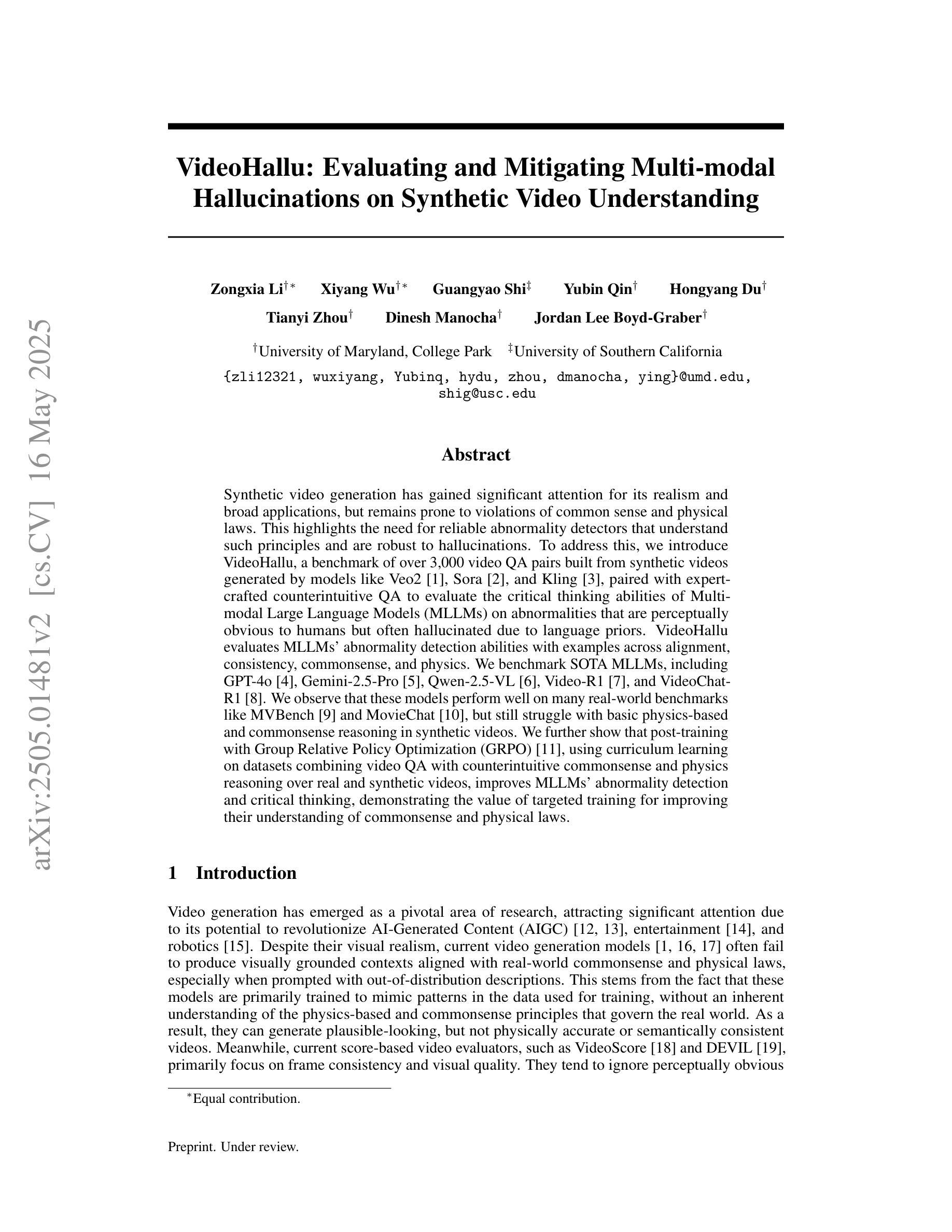
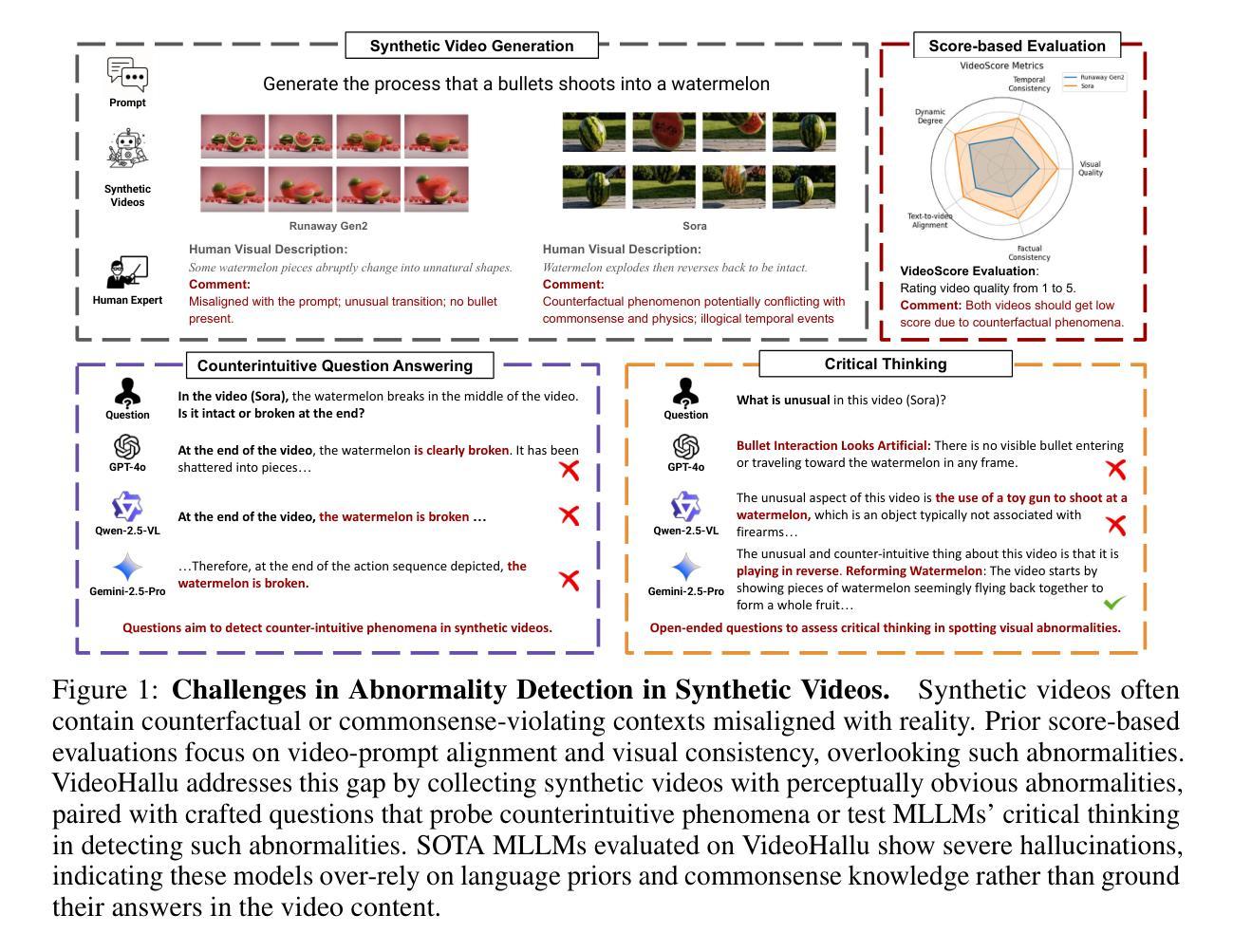
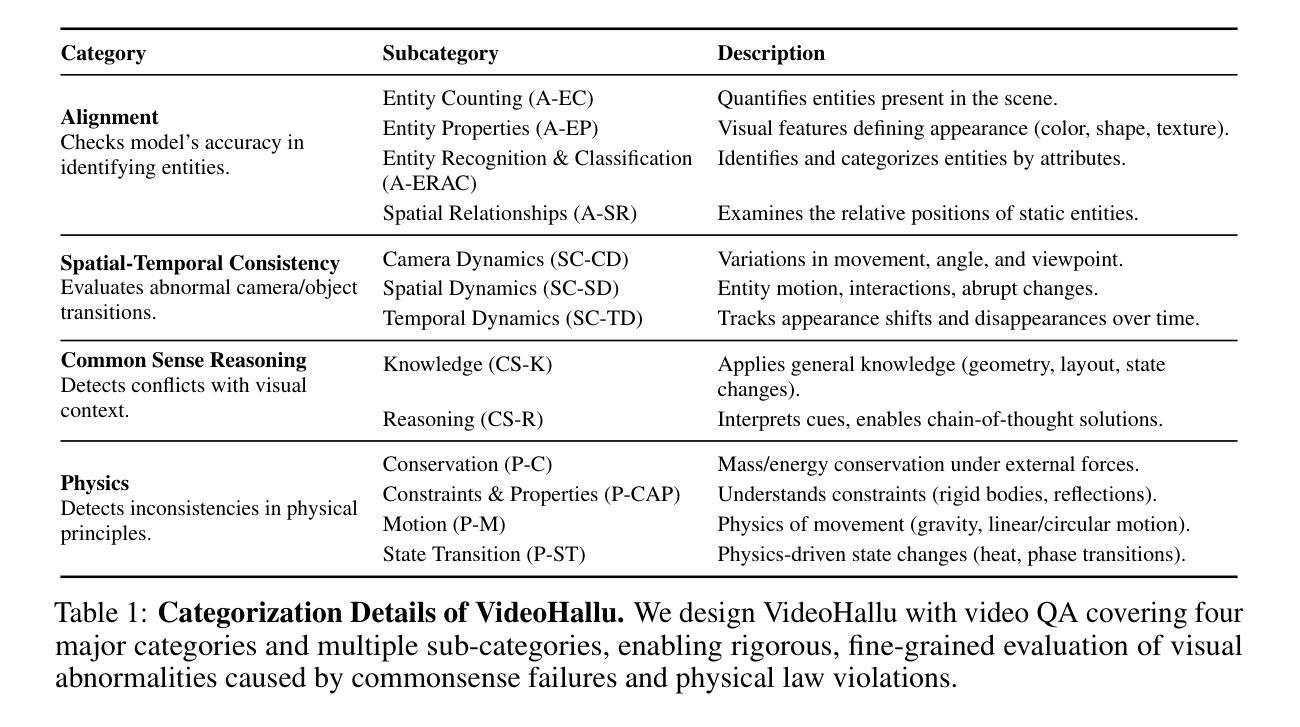
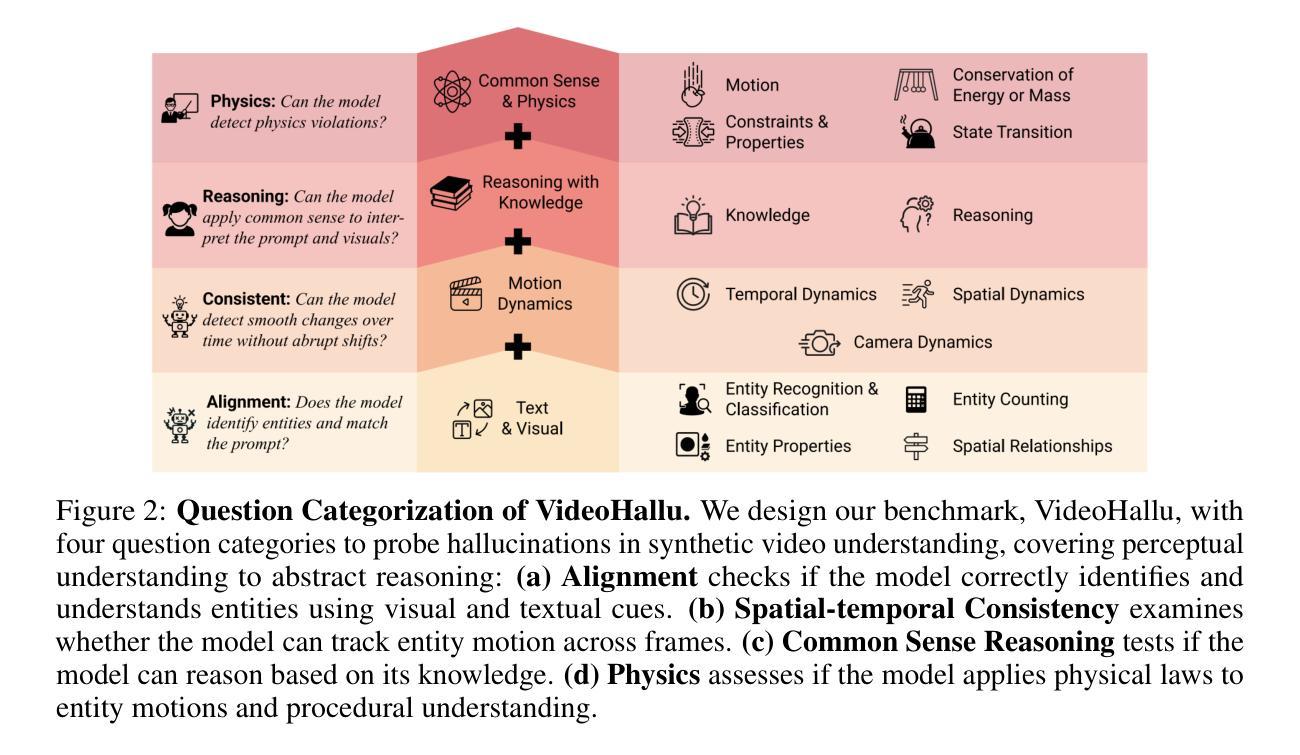
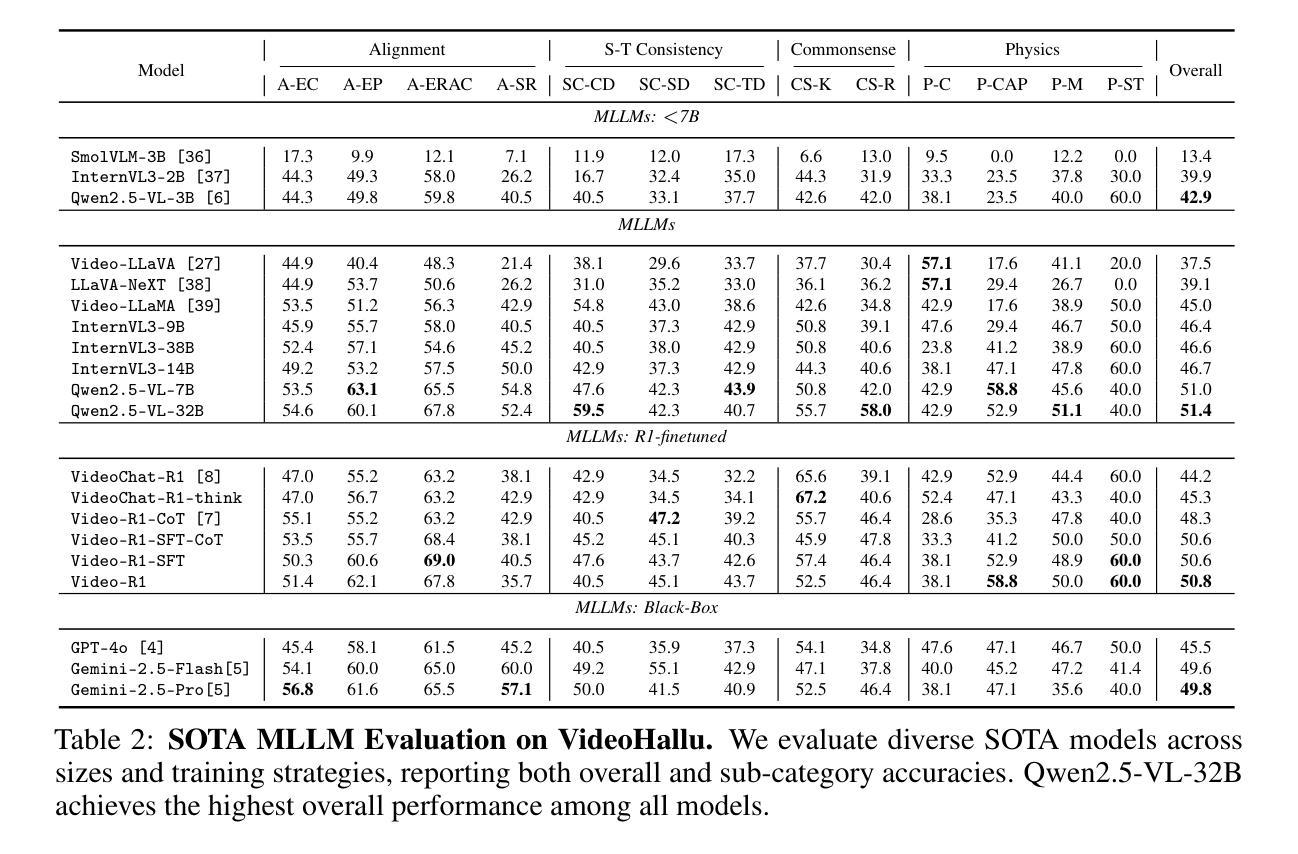
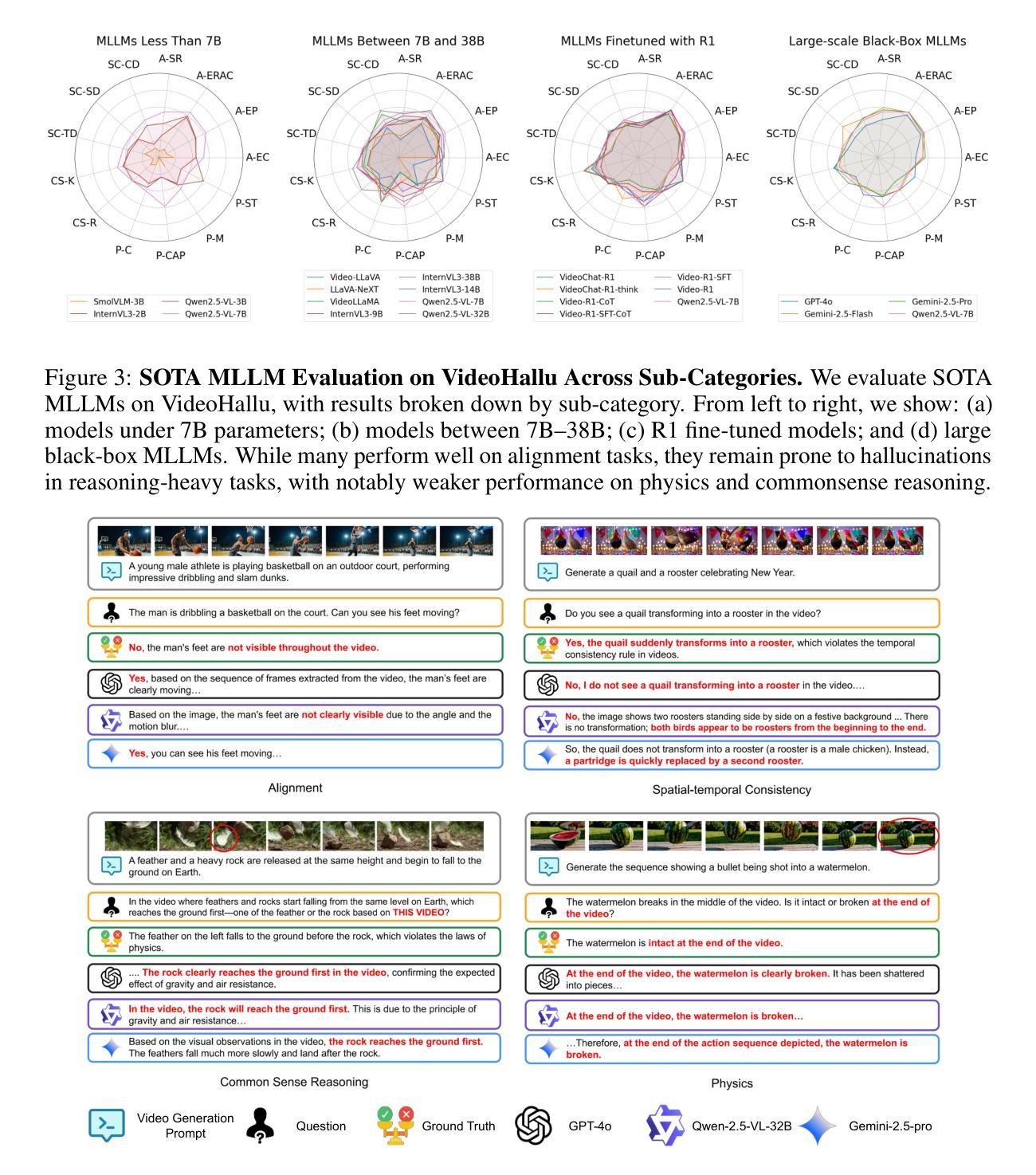