⚠️ 以下所有内容总结都来自于 大语言模型的能力,如有错误,仅供参考,谨慎使用
🔴 请注意:千万不要用于严肃的学术场景,只能用于论文阅读前的初筛!
💗 如果您觉得我们的项目对您有帮助 ChatPaperFree ,还请您给我们一些鼓励!⭐️ HuggingFace免费体验
2025-05-20 更新
Towards Robust and Controllable Text-to-Motion via Masked Autoregressive Diffusion
Authors:Zongye Zhang, Bohan Kong, Qingjie Liu, Yunhong Wang
Generating 3D human motion from text descriptions remains challenging due to the diverse and complex nature of human motion. While existing methods excel within the training distribution, they often struggle with out-of-distribution motions, limiting their applicability in real-world scenarios. Existing VQVAE-based methods often fail to represent novel motions faithfully using discrete tokens, which hampers their ability to generalize beyond seen data. Meanwhile, diffusion-based methods operating on continuous representations often lack fine-grained control over individual frames. To address these challenges, we propose a robust motion generation framework MoMADiff, which combines masked modeling with diffusion processes to generate motion using frame-level continuous representations. Our model supports flexible user-provided keyframe specification, enabling precise control over both spatial and temporal aspects of motion synthesis. MoMADiff demonstrates strong generalization capability on novel text-to-motion datasets with sparse keyframes as motion prompts. Extensive experiments on two held-out datasets and two standard benchmarks show that our method consistently outperforms state-of-the-art models in motion quality, instruction fidelity, and keyframe adherence.
从文本描述生成3D人类运动由于人类运动的多样性和复杂性仍然是一个挑战。现有方法在训练分布内表现卓越,但在超出分布的运动上经常遇到困难,限制了它们在现实场景中的应用。基于VQVAE的方法往往无法用离散令牌忠实表示新型运动,这阻碍了它们超越可见数据的泛化能力。同时,基于连续表示的操作的扩散方法通常缺乏对单个帧的精细控制。为了应对这些挑战,我们提出了一个稳健的运动生成框架MoMADiff,它将掩模建模与扩散过程相结合,利用帧级连续表示生成运动。我们的模型支持用户提供的灵活关键帧规格,能够对运动合成的空间和时间方面实现精确控制。MoMADiff在具有稀疏关键帧的新型文本到运动数据集上展示了强大的泛化能力。在两个独立数据集和两个标准基准测试上的大量实验表明,我们的方法在动作质量、指令保真度和关键帧依从性方面始终优于最新模型。
论文及项目相关链接
PDF 10 pages, 6 figures, 5 tables
摘要
文本描述了从文本描述生成3D人类运动所面临的挑战,包括人类运动的多样性和复杂性。现有方法在训练分布内表现良好,但在处理超出训练分布的运动时常常遇到困难,限制了它们在现实场景中的应用。本文提出了一种稳健的运动生成框架MoMADiff,它将掩模建模与扩散过程相结合,使用帧级连续表示生成运动。该模型支持用户提供的关键帧规格,实现对运动合成的空间和时间方面的精确控制。MoMADiff在带有稀疏关键帧的新文本到运动数据集上表现出强大的泛化能力。在两项独立数据集和两项标准基准测试上的大量实验表明,我们的方法在动作质量、指令保真度和关键帧遵循方面始终优于最新模型。
关键见解
- 文本到3D人类运动的生成面临多样性和复杂性的挑战。
- 现有方法在处理超出训练分布的运动时存在困难。
- MoMADiff框架结合了掩模建模和扩散过程来生成运动。
- MoMADiff使用帧级连续表示,支持用户定义的关键帧规格。
- MoMADiff在新型文本到运动数据集上表现出强大的泛化能力。
- MoMADiff在动作质量、指令保真度和关键帧遵循方面优于现有方法。
- MoMADiff框架为处理复杂和多样化的文本到运动生成任务提供了一种有效和灵活的解决方案。
点此查看论文截图
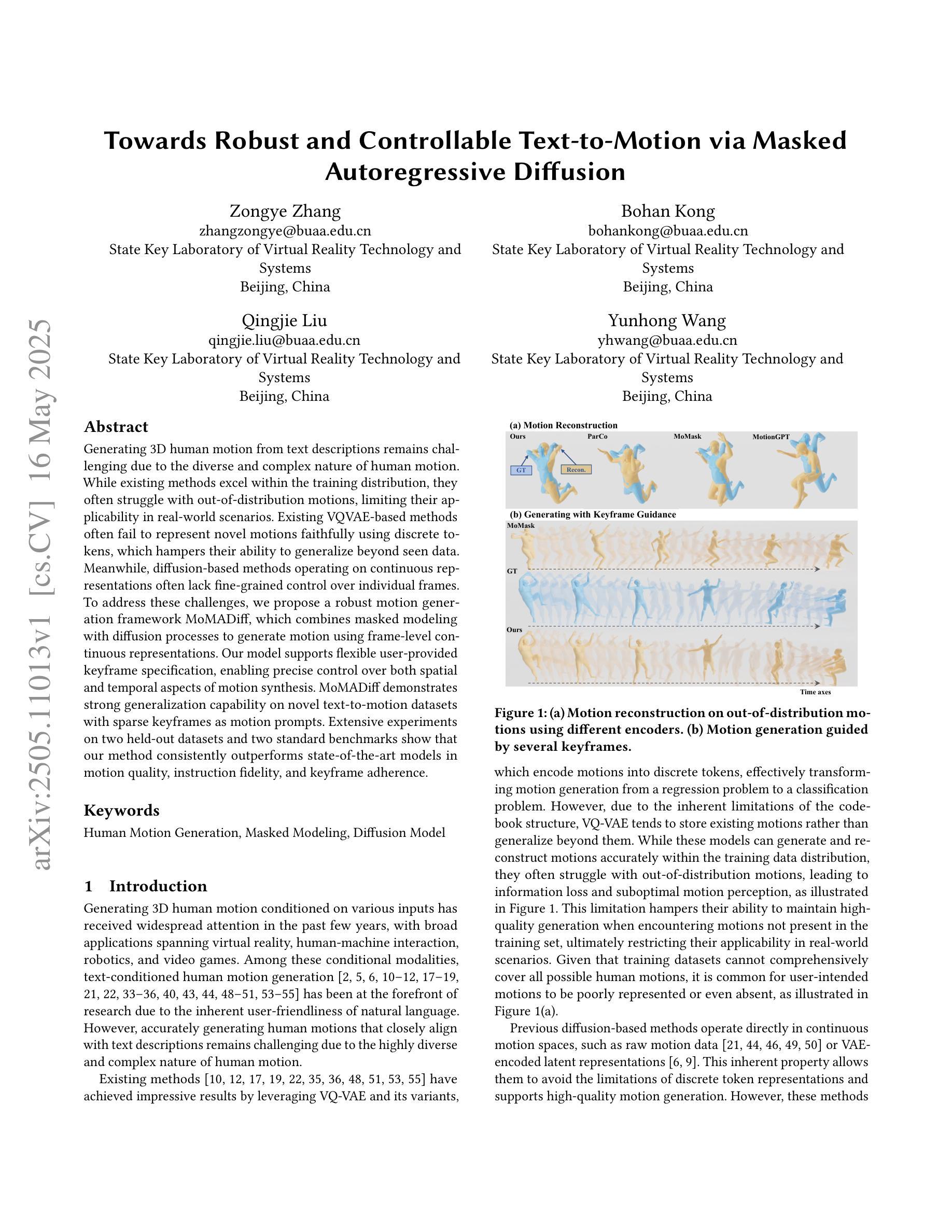
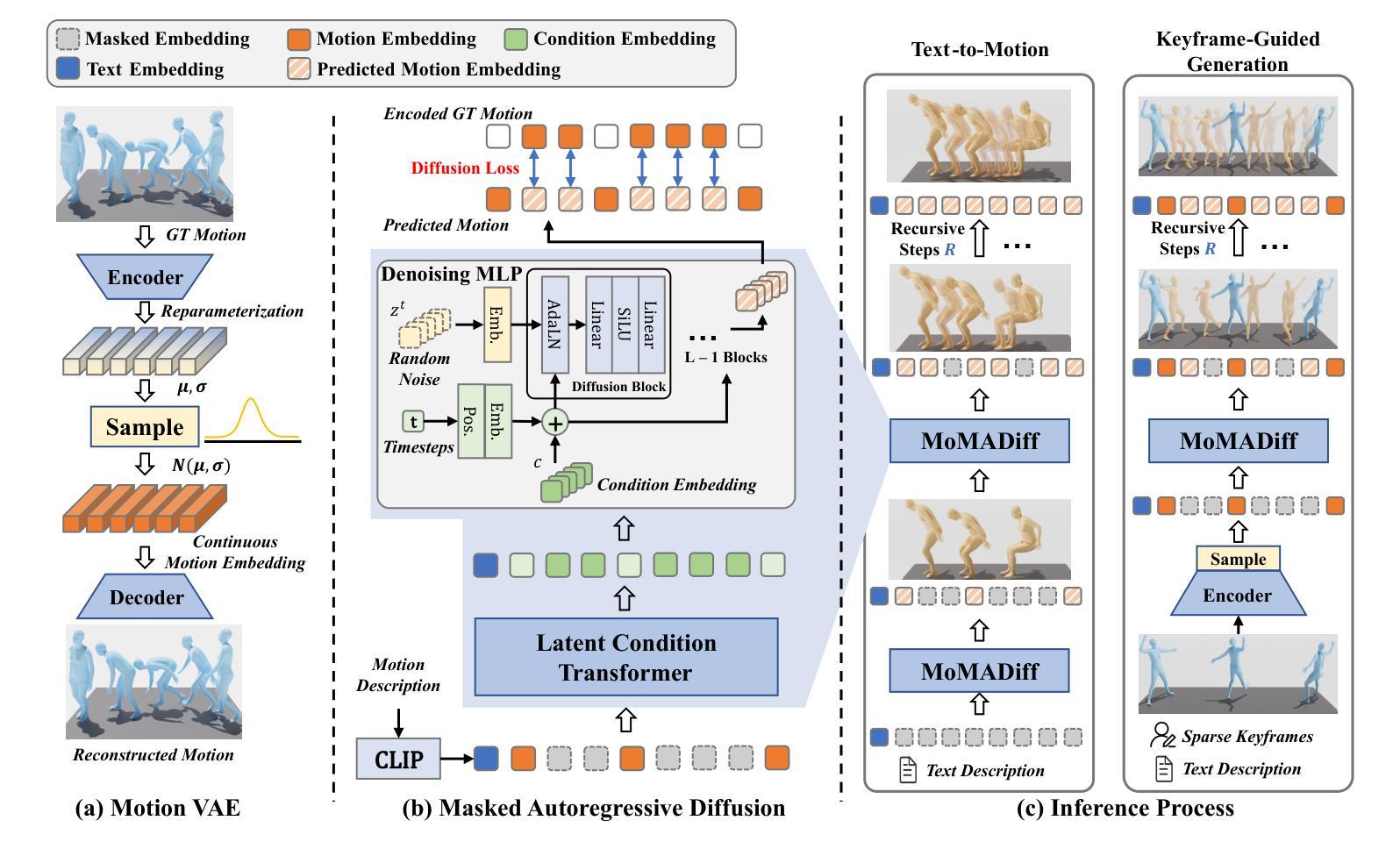
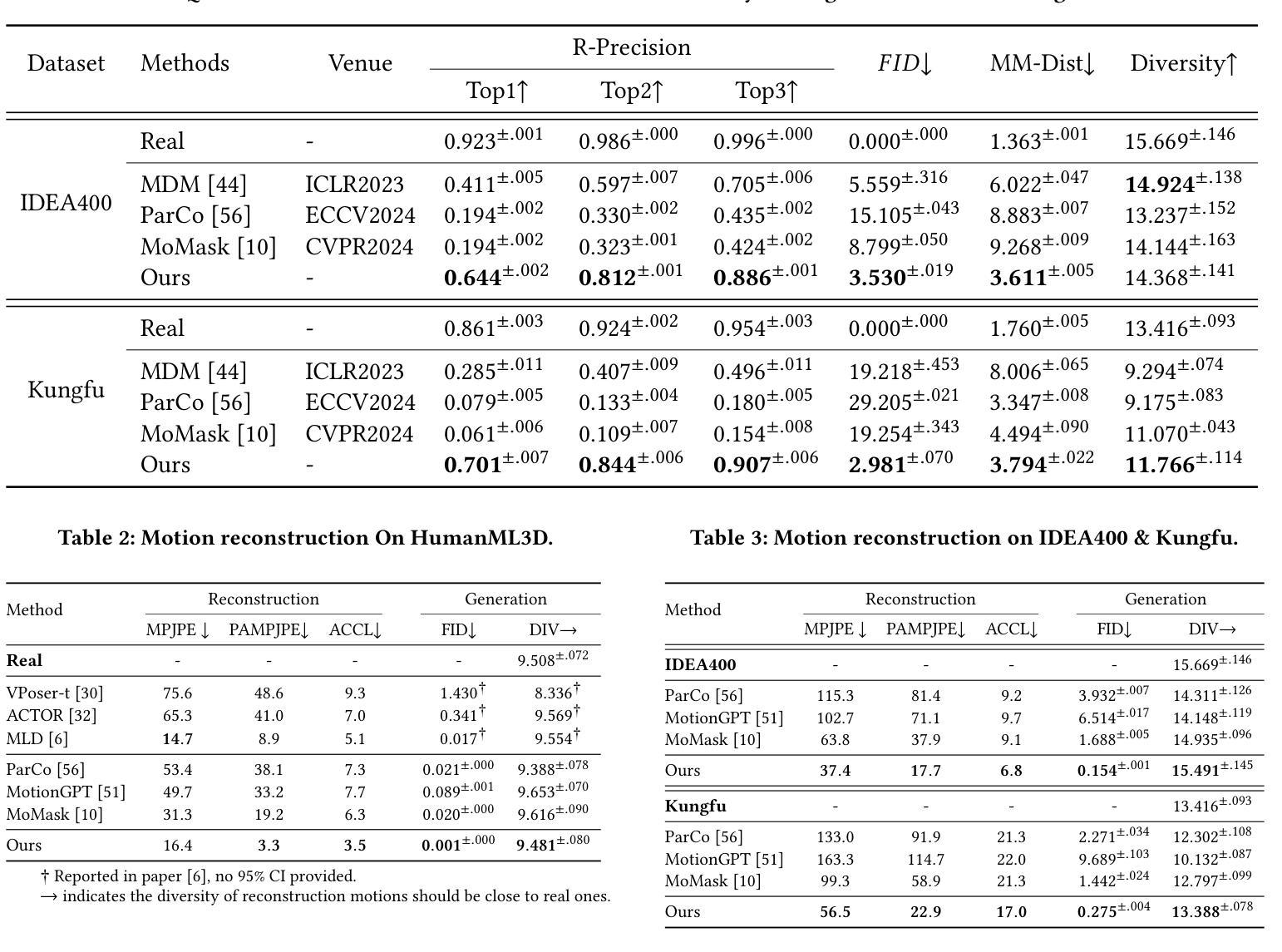
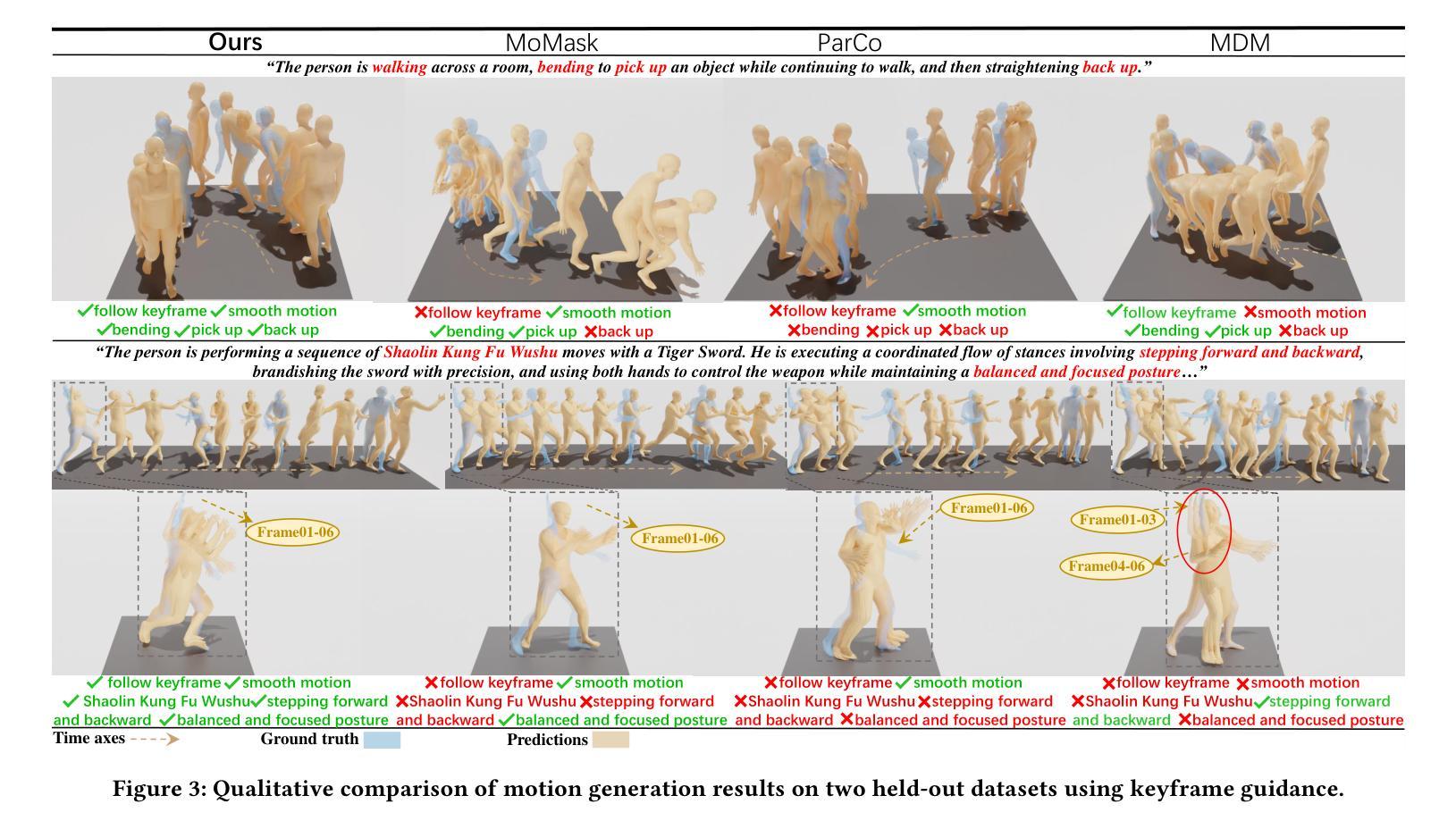
MoCLIP: Motion-Aware Fine-Tuning and Distillation of CLIP for Human Motion Generation
Authors:Gabriel Maldonado, Armin Danesh Pazho, Ghazal Alinezhad Noghre, Vinit Katariya, Hamed Tabkhi
Human motion generation is essential for fields such as animation, robotics, and virtual reality, requiring models that effectively capture motion dynamics from text descriptions. Existing approaches often rely on Contrastive Language-Image Pretraining (CLIP)-based text encoders, but their training on text-image pairs constrains their ability to understand temporal and kinematic structures inherent in motion and motion generation. This work introduces MoCLIP, a fine-tuned CLIP model with an additional motion encoding head, trained on motion sequences using contrastive learning and tethering loss. By explicitly incorporating motion-aware representations, MoCLIP enhances motion fidelity while remaining compatible with existing CLIP-based pipelines and seamlessly integrating into various CLIP-based methods. Experiments demonstrate that MoCLIP improves Top-1, Top-2, and Top-3 accuracy while maintaining competitive FID, leading to improved text-to-motion alignment results. These results highlight MoCLIP’s versatility and effectiveness, establishing it as a robust framework for enhancing motion generation.
人类动作生成对于动画、机器人技术和虚拟现实等领域至关重要,需要模型能够有效地从文本描述中捕捉动作动态。现有方法常常依赖于基于CLIP(Contrastive Language-Image Pretraining)的文本编码器,但其在文本图像对上的训练限制了其理解动作和动作生成中固有的时间性和运动学结构的能力。本研究引入了MoCLIP,这是一个经过精细调整的CLIP模型,配备了一个额外的运动编码头,使用对比学习和锚定损失在动作序列上进行训练。通过明确地引入运动感知表示,MoCLIP提高了运动保真度,同时兼容现有的CLIP基础管道,并能无缝地融入各种CLIP基础方法。实验表明,MoCLIP在提高Top-1、Top-2和Top-3准确率的同时,保持了竞争力的FID,从而提高了文本到动作对齐的结果。这些结果突出了MoCLIP的通用性和有效性,将其确立为一个增强动作生成的稳健框架。
论文及项目相关链接
PDF 11 pages, 5 figures, 2 tables. Presented at the CVPR 2025 Human Motion Generation (HuMoGen) Workshop. Introduces MoCLIP, a CLIP-based fine-tuning strategy for motion generation, with results on HumanML3D dataset and ablation studies
Summary
本文介绍了一种基于CLIP模型的改进方法MoCLIP,该方法通过引入运动编码头并使用对比学习和tethering损失进行训练,以更好地理解和生成运动序列。MoCLIP提高了运动保真度,同时兼容现有的CLIP管道,并能无缝集成到各种CLIP方法中。实验表明,MoCLIP在保持竞争力的FID的同时,提高了Top-1、Top-2和Top-3的准确性,实现了更好的文本到运动的对齐结果。
Key Takeaways
- MoCLIP是基于CLIP模型的改进方法,用于更有效地从文本描述中捕获运动动力学。
- MoCLIP通过引入运动编码头,增强了模型对运动序列的理解能力。
- 对比学习和tethering损失被用于训练MoCLIP,以提高运动序列的生成质量。
- MoCLIP提高了运动保真度,同时兼容现有的CLIP管道。
- MoCLIP能够无缝集成到各种CLIP方法中,提高了文本到运动的对齐结果。
- 实验结果表明,MoCLIP在保持竞争力的FID的同时,提高了Top-1、Top-2和Top-3的准确性。
点此查看论文截图
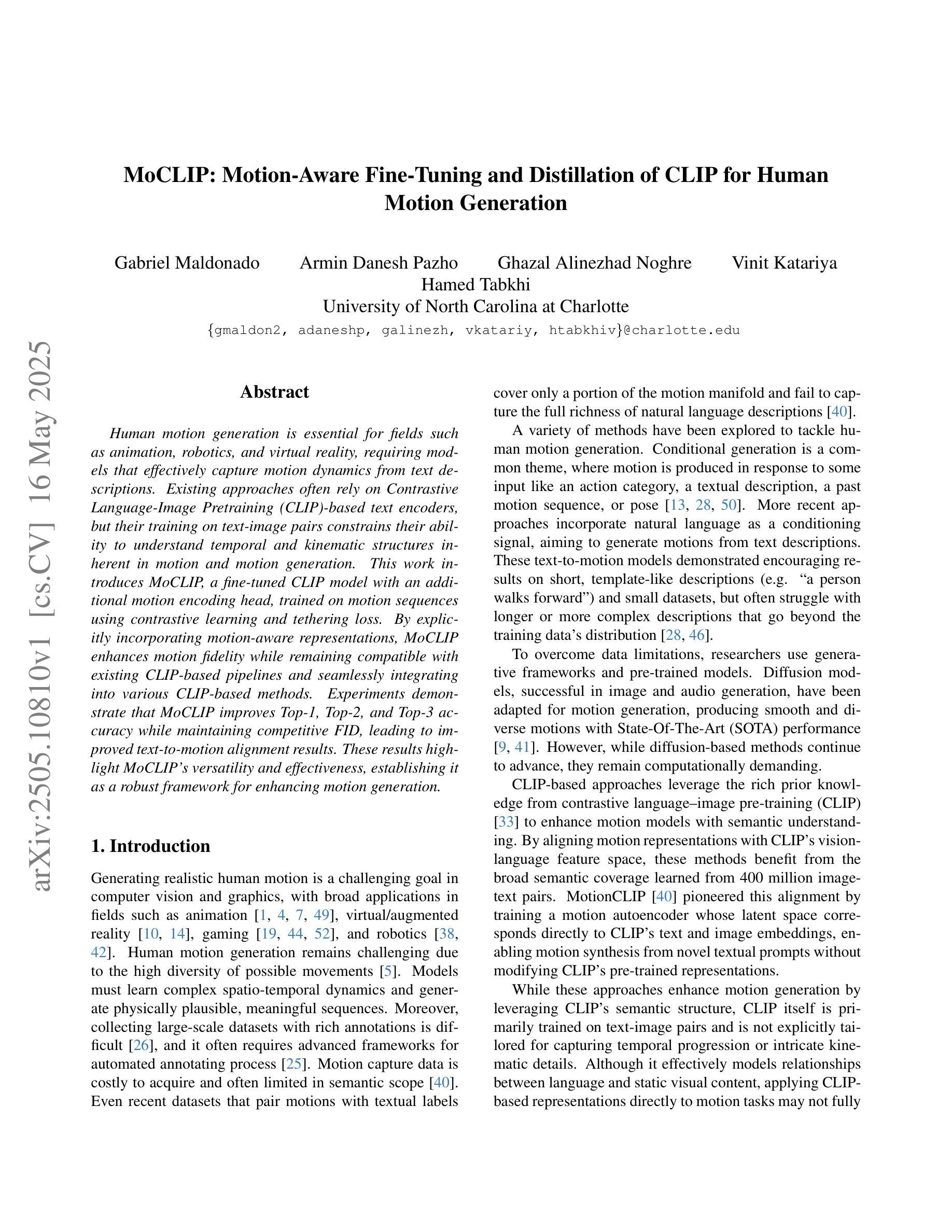
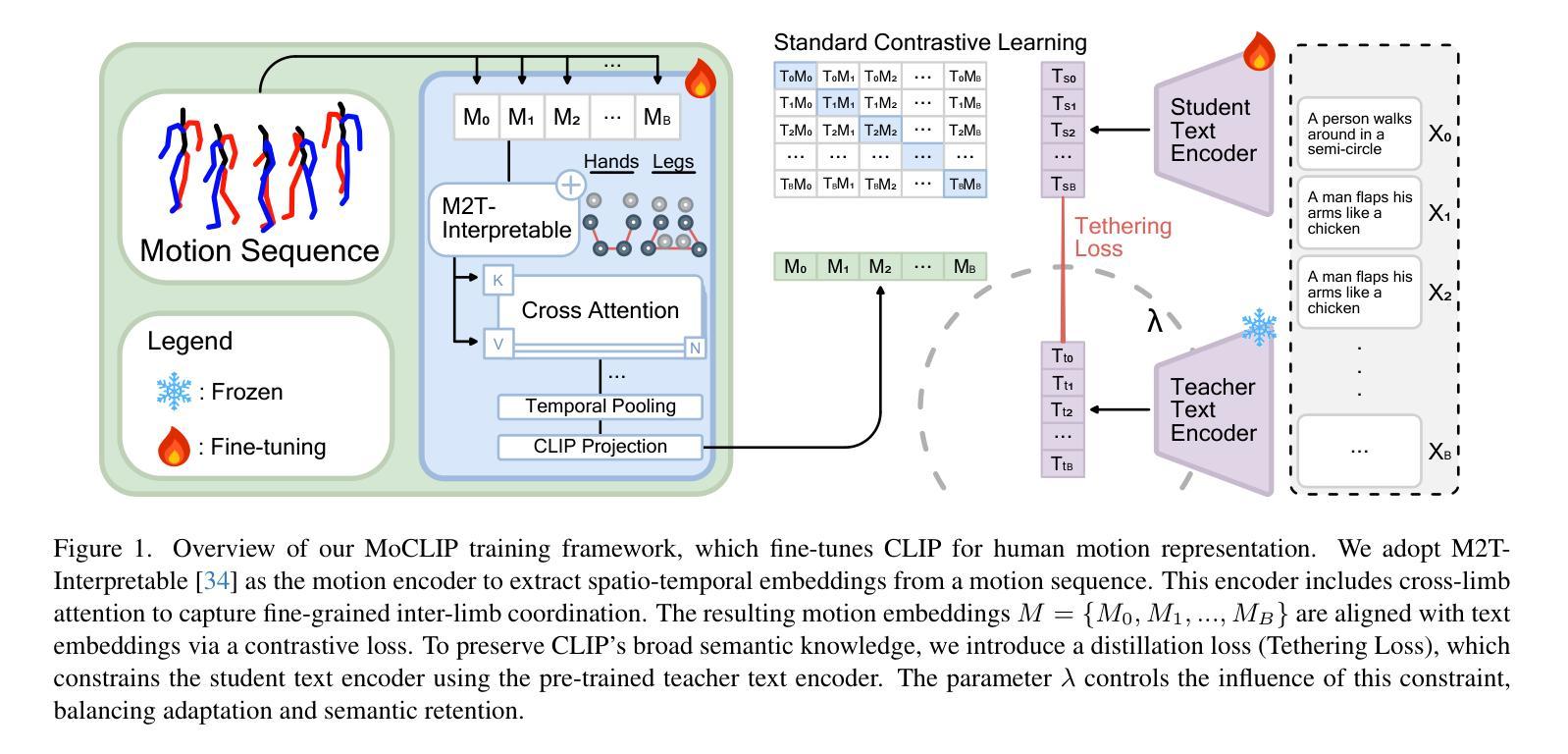
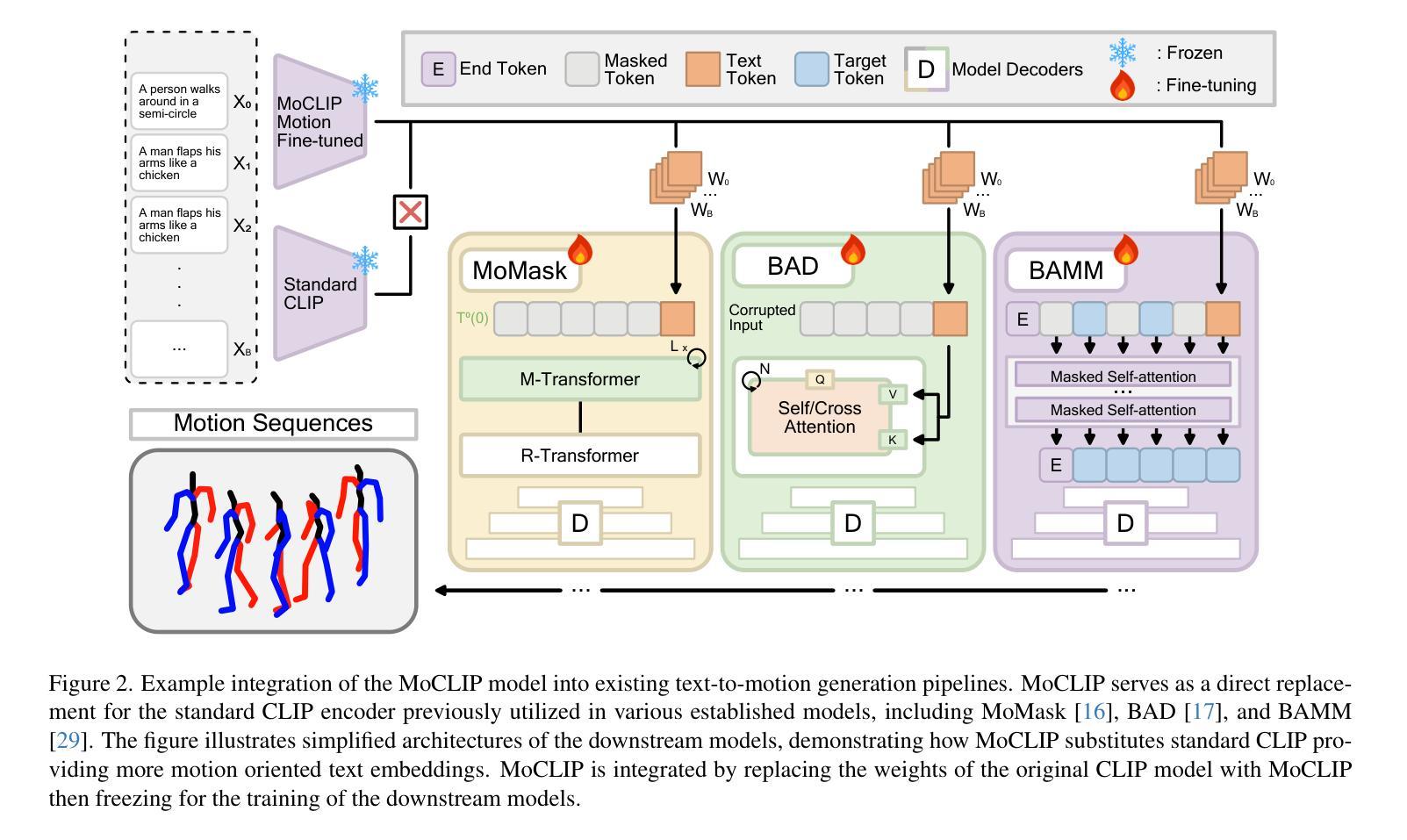
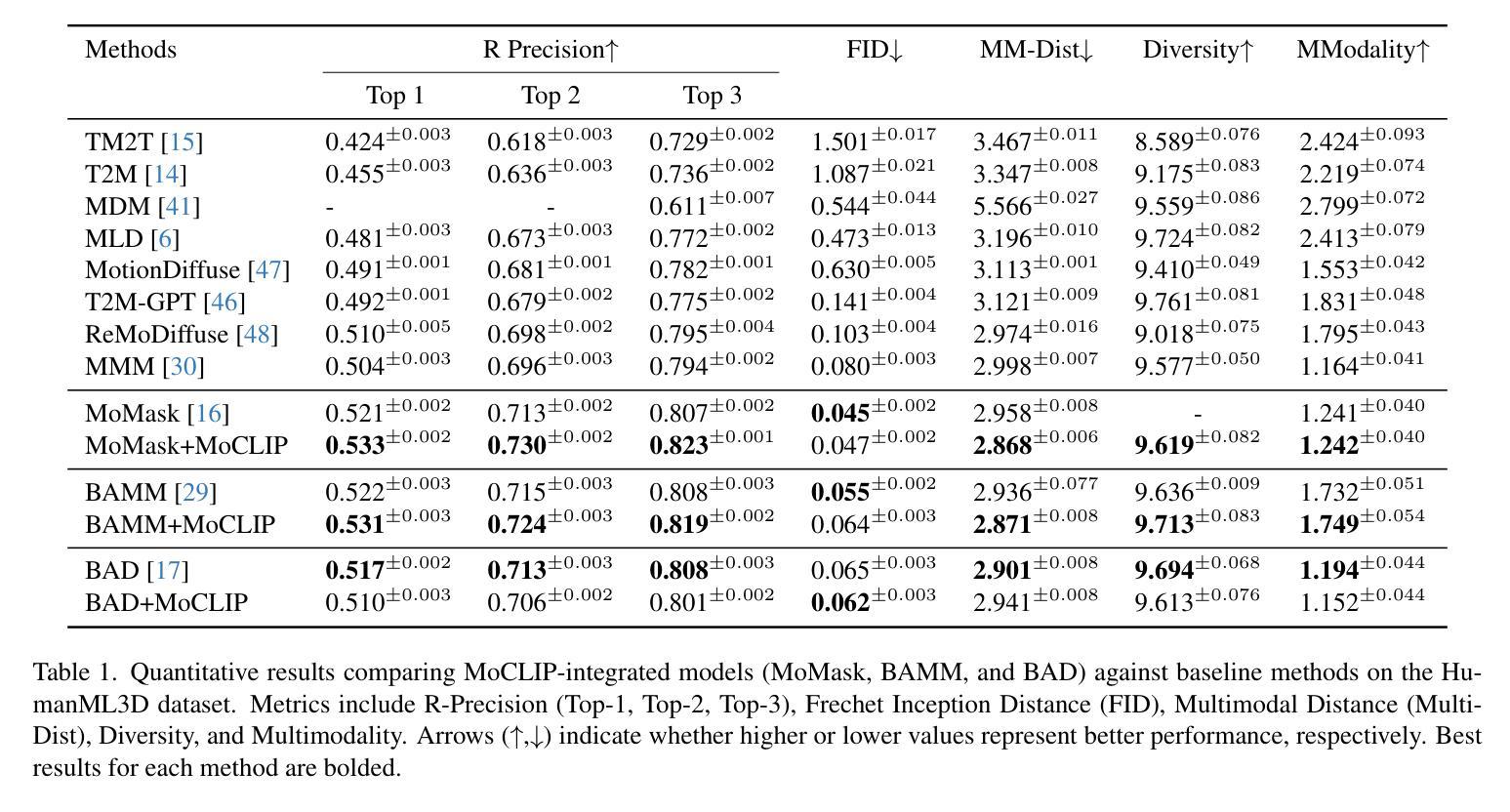