⚠️ 以下所有内容总结都来自于 大语言模型的能力,如有错误,仅供参考,谨慎使用
🔴 请注意:千万不要用于严肃的学术场景,只能用于论文阅读前的初筛!
💗 如果您觉得我们的项目对您有帮助 ChatPaperFree ,还请您给我们一些鼓励!⭐️ HuggingFace免费体验
2025-05-21 更新
HiERO: understanding the hierarchy of human behavior enhances reasoning on egocentric videos
Authors:Simone Alberto Peirone, Francesca Pistilli, Giuseppe Averta
Human activities are particularly complex and variable, and this makes challenging for deep learning models to reason about them. However, we note that such variability does have an underlying structure, composed of a hierarchy of patterns of related actions. We argue that such structure can emerge naturally from unscripted videos of human activities, and can be leveraged to better reason about their content. We present HiERO, a weakly-supervised method to enrich video segments features with the corresponding hierarchical activity threads. By aligning video clips with their narrated descriptions, HiERO infers contextual, semantic and temporal reasoning with an hierarchical architecture. We prove the potential of our enriched features with multiple video-text alignment benchmarks (EgoMCQ, EgoNLQ) with minimal additional training, and in zero-shot for procedure learning tasks (EgoProceL and Ego4D Goal-Step). Notably, HiERO achieves state-of-the-art performance in all the benchmarks, and for procedure learning tasks it outperforms fully-supervised methods by a large margin (+12.5% F1 on EgoProceL) in zero shot. Our results prove the relevance of using knowledge of the hierarchy of human activities for multiple reasoning tasks in egocentric vision.
人类活动特别复杂且多变,这给深度学习模型对其进行分析带来了挑战。然而,我们注意到这种变化其实具有一种底层结构,由相关行为的层次模式组成。我们认为这种结构可以自然地从人类活动的非脚本视频中浮现出来,并可以利用它来更好地分析视频内容。我们提出了HiERO,一种弱监督方法,用于通过相应的层次活动线索来丰富视频片段的特征。通过将视频片段与它们的叙述描述进行对齐,HiERO使用层次结构进行上下文、语义和时间推理。我们通过多个视频文本对齐基准测试(EgoMCQ、EgoNLQ)验证了丰富特征的潜力,在最少的额外训练下,以及在零样本学习程序任务中(EgoProceL和Ego4D Goal-Step)。值得注意的是,HiERO在所有基准测试中均达到了最先进的性能,对于程序学习任务,它在零样本情况下大幅超越了全监督方法(EgoProceL上的F1分数提高了12.5%)。我们的结果证明了在多个以自我为中心视角的推理任务中,利用人类活动层次结构知识的重要性。
论文及项目相关链接
PDF Project page https://github.com/sapeirone/hiero
Summary
人类活动复杂多变,深度学习模型理解起来有难度。但人类活动存在潜在的结构性,即一系列相关动作的层次结构。我们主张这种结构可以自然地从未经脚本的人类活动视频中产生并利用它来更好地理解视频内容。我们提出了HiERO方法,这是一种弱监督方法,能够丰富视频片段特征,对应层次化的活动线索。通过视频剪辑与叙述描述的对应,HiERO在层次架构中推断上下文、语义和时间推理。我们在多个视频文本对齐基准测试(EgoMCQ、EgoNLQ)上验证了丰富特征的潜力,并且在零样本学习任务(EgoProceL和Ego4D Goal-Step)上也有所表现。值得注意的是,HiERO在所有基准测试中均达到了最佳性能,并且在零样本学习任务的程序学习中大幅超越了全监督方法(EgoProceL上的F1得分提高了12.5%)。我们的结果证明了利用人类活动层次结构知识对于多个第一人称视角的任务推理的重要性。
Key Takeaways
- 人类活动的复杂性和多变性给深度学习模型的理解带来挑战。
- 人类活动存在潜在的结构性,即一系列相关动作的层次结构。
- HiERO方法是一种弱监督方法,能够从未经脚本的视频中推断层次化的活动线索。
- HiERO通过视频剪辑与叙述描述的对应,在层次架构中整合上下文、语义和时间推理。
- 在多个视频文本对齐基准测试中,HiERO表现出卓越性能。
- 在零样本学习任务的程序学习中,HiERO大幅超越了全监督方法。
点此查看论文截图
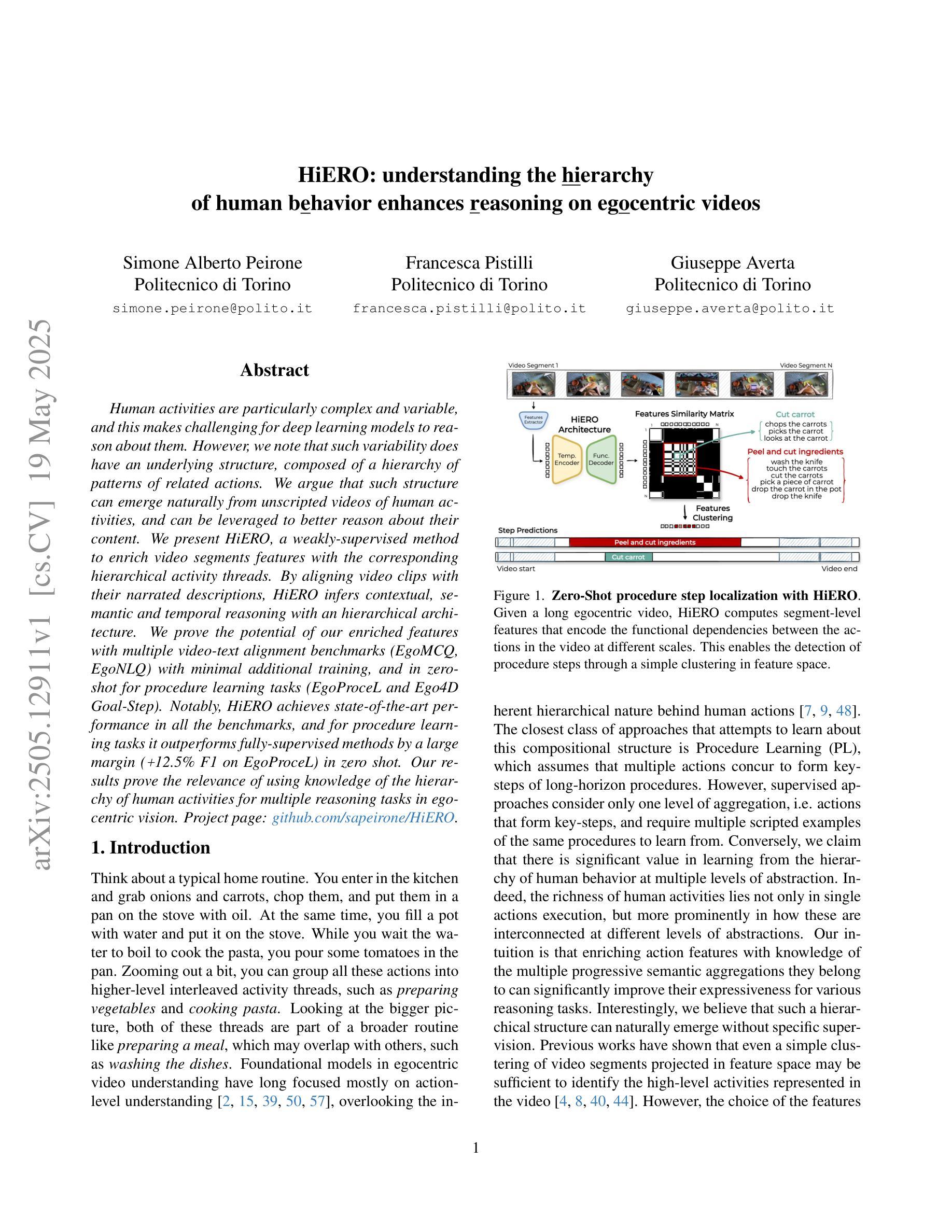
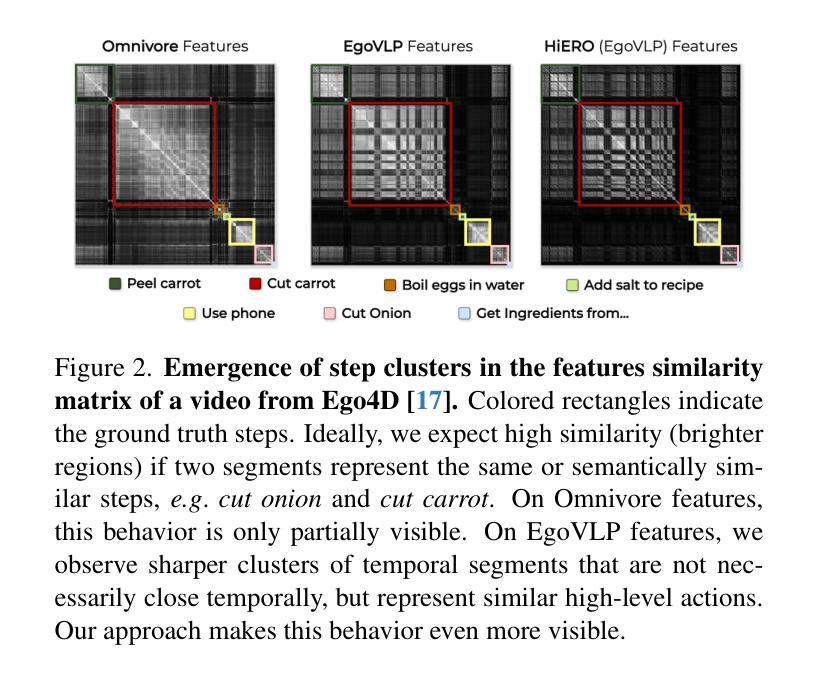
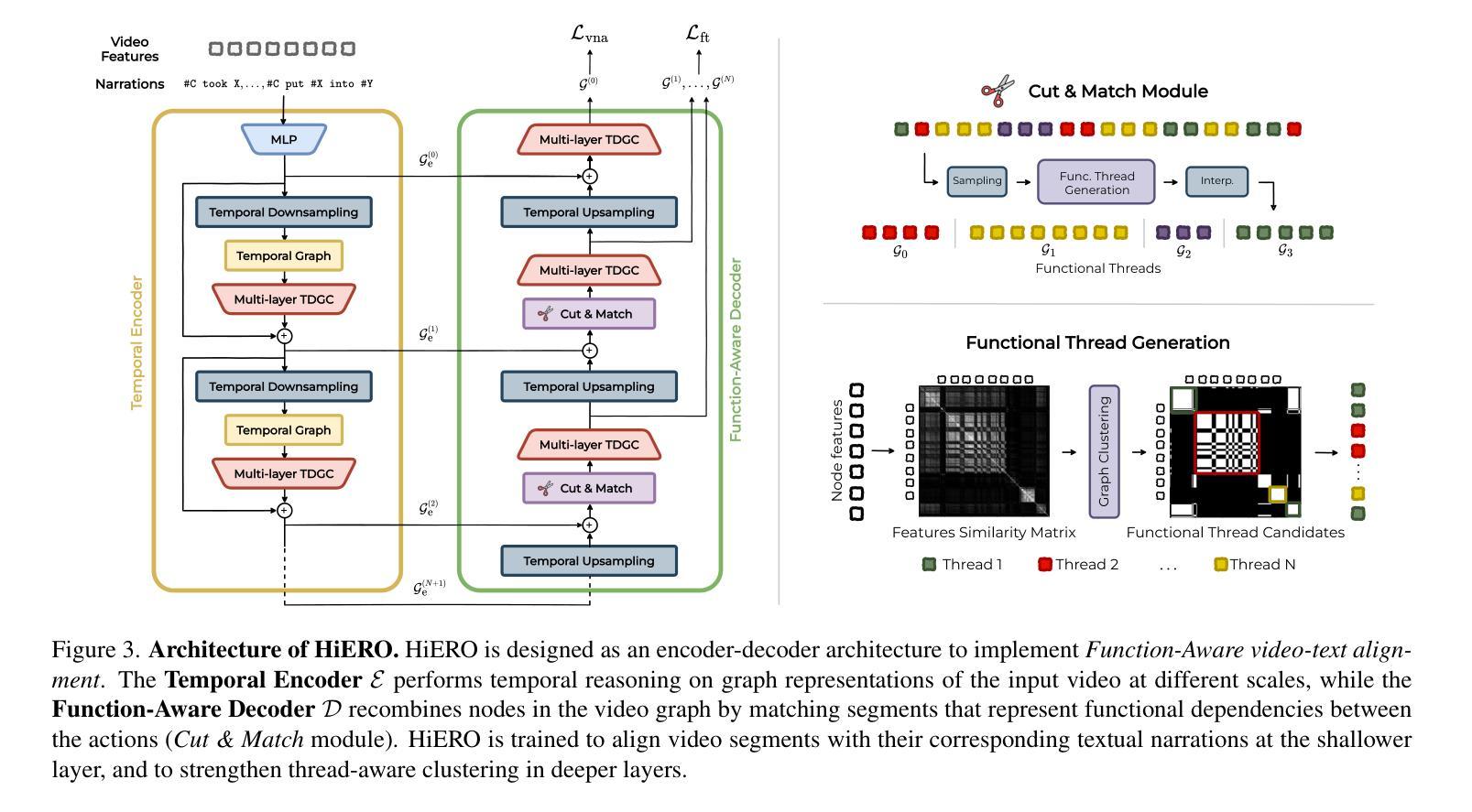
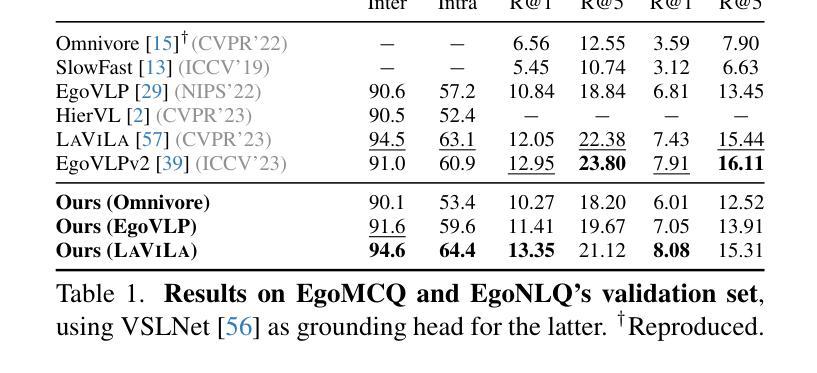
Temporal-Oriented Recipe for Transferring Large Vision-Language Model to Video Understanding
Authors:Thong Nguyen, Zhiyuan Hu, Xu Lin, Cong-Duy Nguyen, See-Kiong Ng, Luu Anh Tuan
Recent years have witnessed outstanding advances of large vision-language models (LVLMs). In order to tackle video understanding, most of them depend upon their implicit temporal understanding capacity. As such, they have not deciphered important components that contribute to temporal understanding ability, which might limit the potential of these LVLMs for video understanding. In this work, we conduct a thorough empirical study to demystify crucial components that influence the temporal understanding of LVLMs. Our empirical study reveals that significant impacts are centered around the intermediate interface between the visual encoder and the large language model. Building on these insights, we propose a temporal-oriented recipe that encompasses temporal-oriented training schemes and an upscaled interface. Our final model developed using our recipe significantly enhances previous LVLMs on standard video understanding tasks.
近年来,大型视觉语言模型(LVLMs)取得了突出的进展。为了处理视频理解,它们大多依赖于隐式的时序理解能。因此,它们尚未解密对时序理解能力做出贡献的重要组件,这可能会限制这些LVLMs在视频理解方面的潜力。在这项工作中,我们对影响LVLMs时序理解的关键组件进行了彻底的实证研究。我们的实证研究揭示,影响主要集中在视觉编码器与大型语言模型之间的中间接口。基于这些见解,我们提出了一个面向时序的配方,包括面向时序的训练方案和扩展接口。使用我们的配方开发的最终模型在标准视频理解任务上显著提高了之前的LVLMs性能。
论文及项目相关链接
PDF In Progress
Summary
本文探讨了大型视觉语言模型(LVLMs)在视频理解方面的进展。虽然这些模型具有隐式的时序理解能力,但它们尚未解析对时序理解能力至关重要的组件,这限制了它们在视频理解方面的潜力。本研究通过实证研究揭示了影响LVLMs时序理解的关键因素,并提出了一种面向时序的训练方案和扩展接口。使用此方案开发的最终模型在标准视频理解任务上显著提高了之前的LVLMs性能。
Key Takeaways
- 大型视觉语言模型(LVLMs)在视频理解方面取得显著进展。
- 大多数LVLMs依赖隐式的时序理解能力。
- 实证研究揭示了影响LVLMs时序理解的关键因素。
- 关键因素集中在视觉编码器与大型语言模型之间的中间接口。
- 提出了一种面向时序的训练方案和扩展接口。
- 使用此方案开发的模型在标准视频理解任务上表现优异。
点此查看论文截图
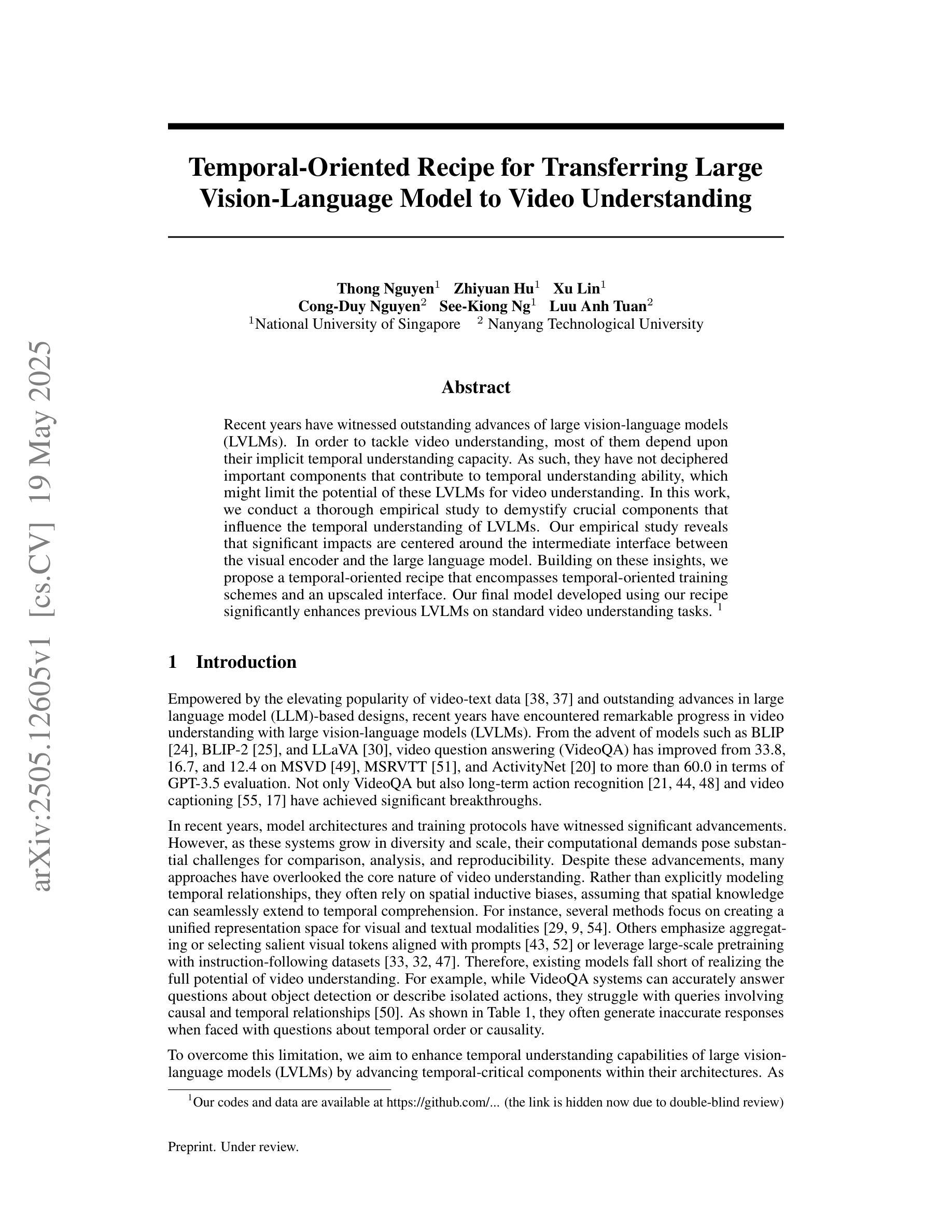
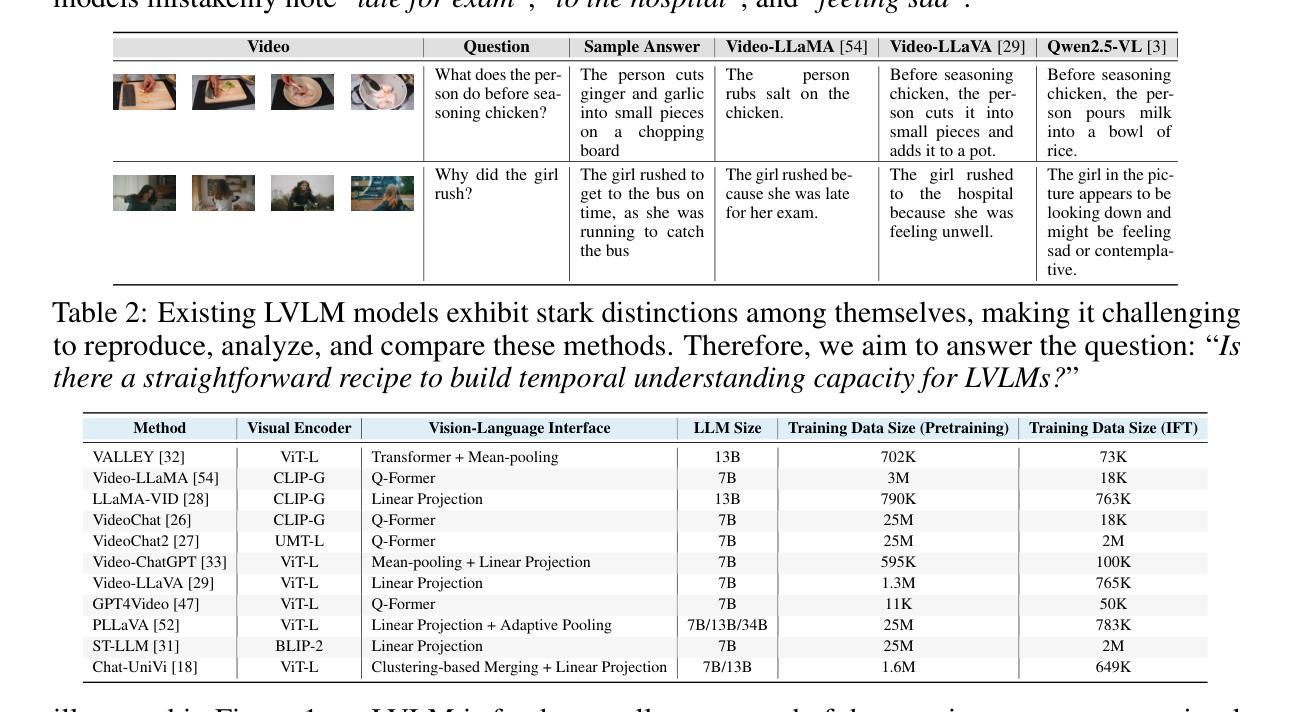
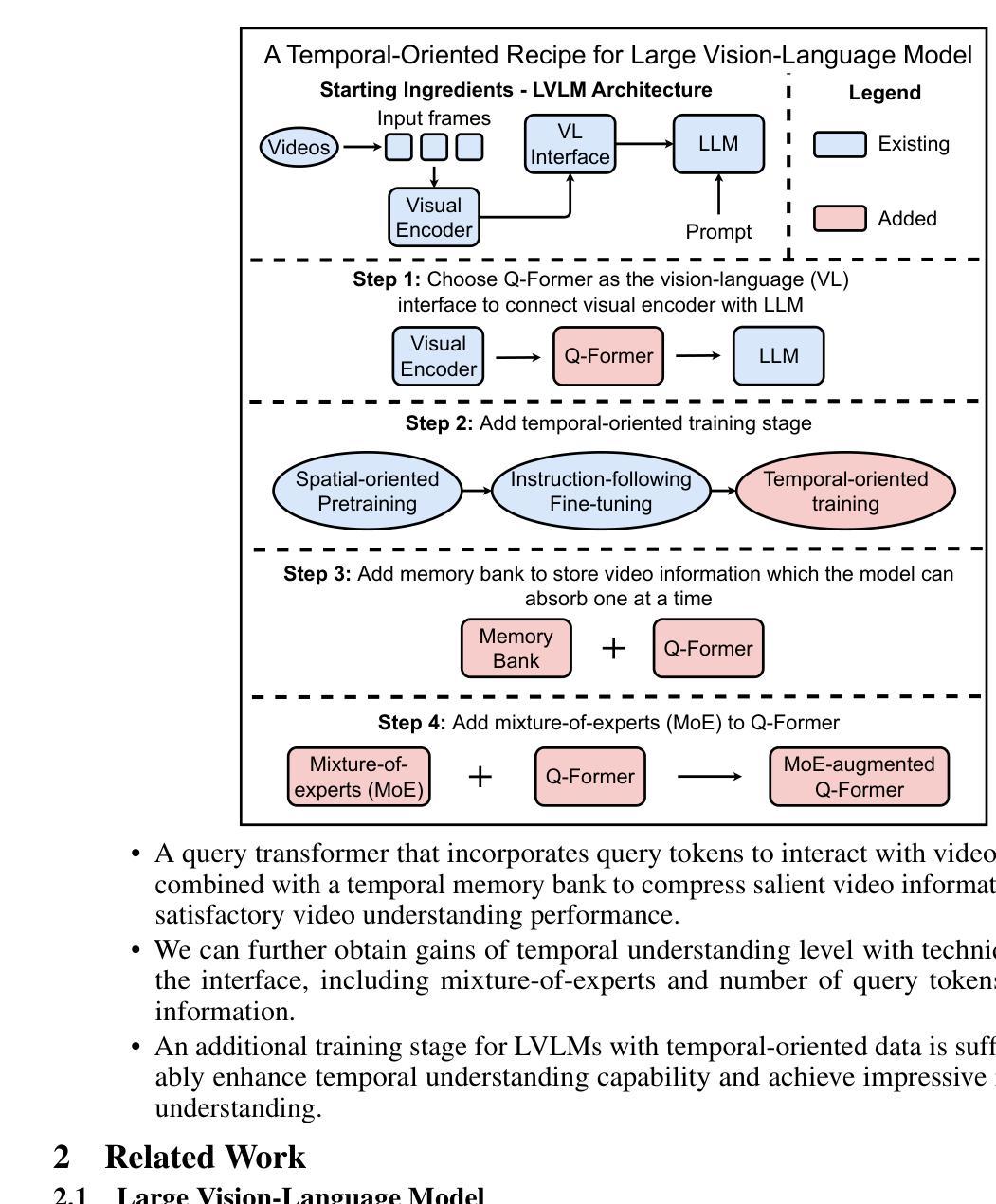
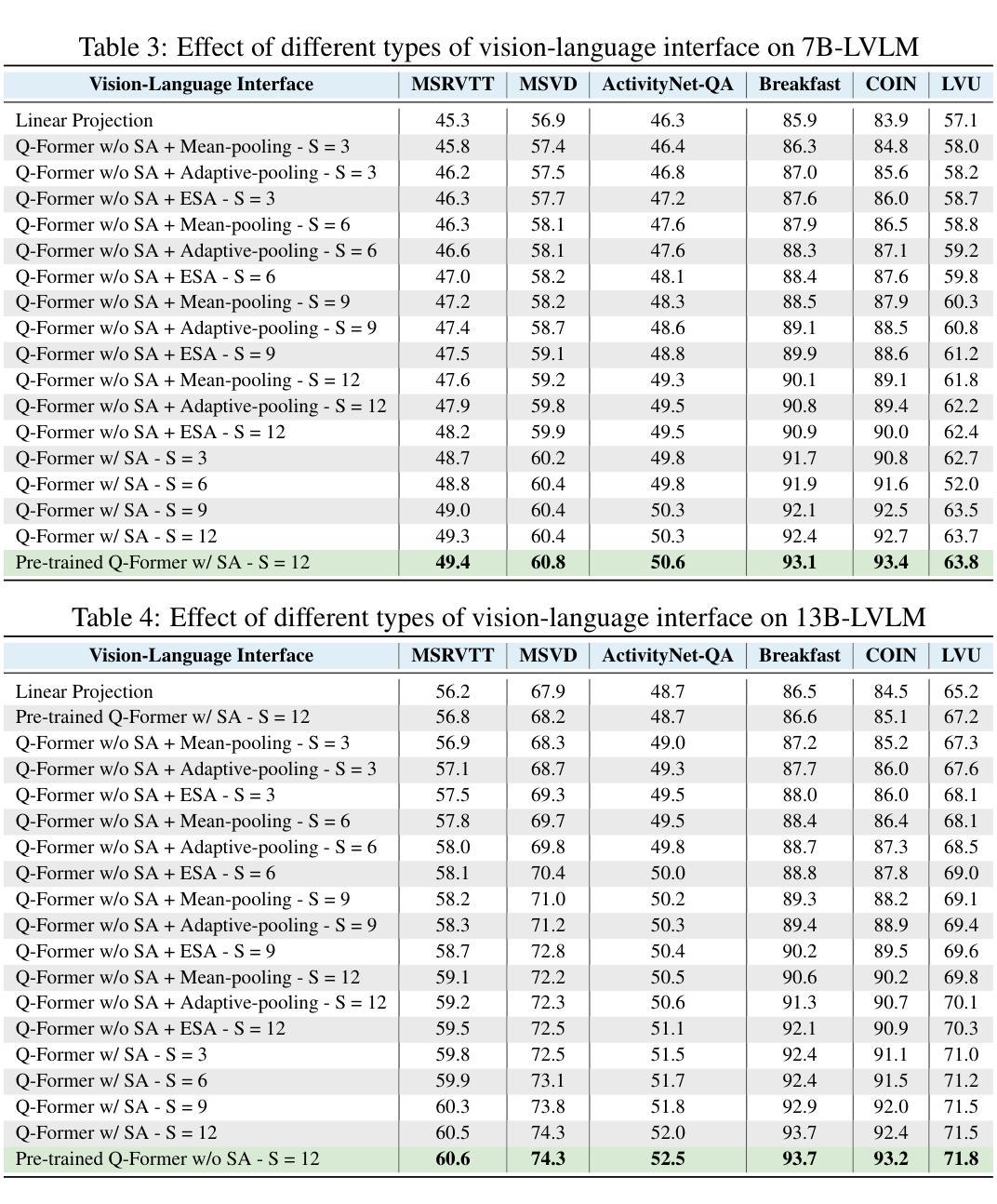
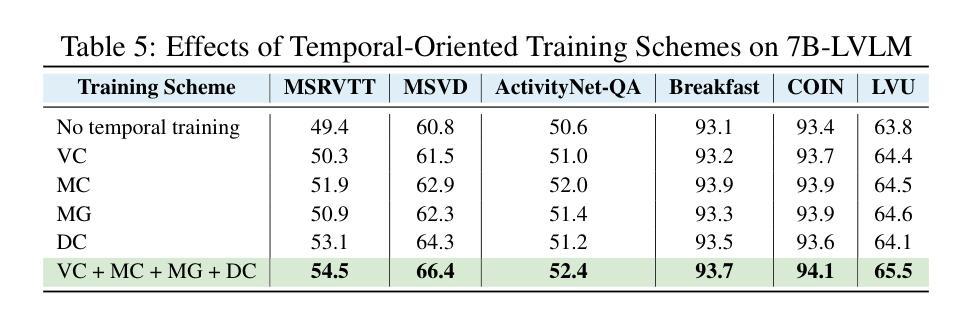
Logic-in-Frames: Dynamic Keyframe Search via Visual Semantic-Logical Verification for Long Video Understanding
Authors:Weiyu Guo, Ziyang Chen, Shaoguang Wang, Jianxiang He, Yijie Xu, Jinhui Ye, Ying Sun, Hui Xiong
Understanding long video content is a complex endeavor that often relies on densely sampled frame captions or end-to-end feature selectors, yet these techniques commonly overlook the logical relationships between textual queries and visual elements. In practice, computational constraints necessitate coarse frame subsampling, a challenge analogous to “finding a needle in a haystack.” To address this issue, we introduce a semantics-driven search framework that reformulates keyframe selection under the paradigm of Visual Semantic-Logical Search. Specifically, we systematically define four fundamental logical dependencies: 1) spatial co-occurrence, 2) temporal proximity, 3) attribute dependency, and 4) causal order. These relations dynamically update frame sampling distributions through an iterative refinement process, enabling context-aware identification of semantically critical frames tailored to specific query requirements. Our method establishes new SOTA performance on the manually annotated benchmark in key-frame selection metrics. Furthermore, when applied to downstream video question-answering tasks, the proposed approach demonstrates the best performance gains over existing methods on LongVideoBench and Video-MME, validating its effectiveness in bridging the logical gap between textual queries and visual-temporal reasoning. The code will be publicly available.
理解长视频内容是一项复杂的任务,通常依赖于密集采样的帧字幕或端到端特征选择器,然而这些技术常常忽略了文本查询和视觉元素之间的逻辑关系。实际上,计算约束需要进行粗略的帧子采样,这类似于“在稻草中寻找针”的挑战。为了解决这个问题,我们引入了一个语义驱动搜索框架,该框架在视觉语义逻辑搜索的范式下重新制定关键帧选择。具体来说,我们系统地定义了四种基本逻辑依赖关系:1)空间共现,2)时间接近性,3)属性依赖,4)因果顺序。这些关系通过迭代优化过程动态更新帧采样分布,使上下文感知的语义关键帧识别能够针对特定查询需求进行定制。我们的方法在关键帧选择指标的手动注释基准测试上建立了最新的最佳性能。此外,当应用于下游视频问答任务时,所提出的方法在LongVideoBench和Video-MME上实现了对现有方法的最佳性能提升,验证了其在弥合文本查询和视觉时间推理之间的逻辑差距方面的有效性。代码将公开可用。
论文及项目相关链接
PDF 32 pages, under review
摘要
视觉内容的长视频理解是一项复杂的任务,常依赖于密集采样帧标注或端到端特征选择器。然而,这些方法常常忽视了文本查询和视觉元素间的逻辑关系。实际应用中,计算限制要求进行粗帧子采样,这一挑战如同“在稻草中寻找针”。为解决这一问题,我们引入了语义驱动搜索框架,在视觉语义逻辑搜索范式下重新构建关键帧选择。具体地,我们系统地定义了四种基本逻辑依赖关系:1)空间共现,2)时间邻近,3)属性依赖和4)因果顺序。这些关系通过迭代优化过程动态更新帧采样分布,实现针对特定查询要求上下文感知的语义关键帧识别。该方法在关键帧选择指标上的手动注释基准测试上建立了最新性能。此外,当应用于下游视频问答任务时,该方法在长视频基准测试和Video-MME上的性能优于现有方法,验证了其在弥合文本查询和视觉时间推理之间的逻辑鸿沟方面的有效性。
要点
- 长视频理解依赖密集帧标注和端到端特征选择器,但忽视了文本查询和视觉元素间的逻辑关系。
- 粗帧子采样是实际应用中的挑战。
- 引入语义驱动搜索框架,在视觉语义逻辑搜索范式下重新构建关键帧选择。
- 定义四种逻辑依赖关系:空间共现、时间邻近、属性依赖和因果顺序。
- 通过迭代优化过程动态更新帧采样分布,实现上下文感知的语义关键帧识别。
- 在关键帧选择指标上表现优越,并且能有效应用于下游视频问答任务。
点此查看论文截图
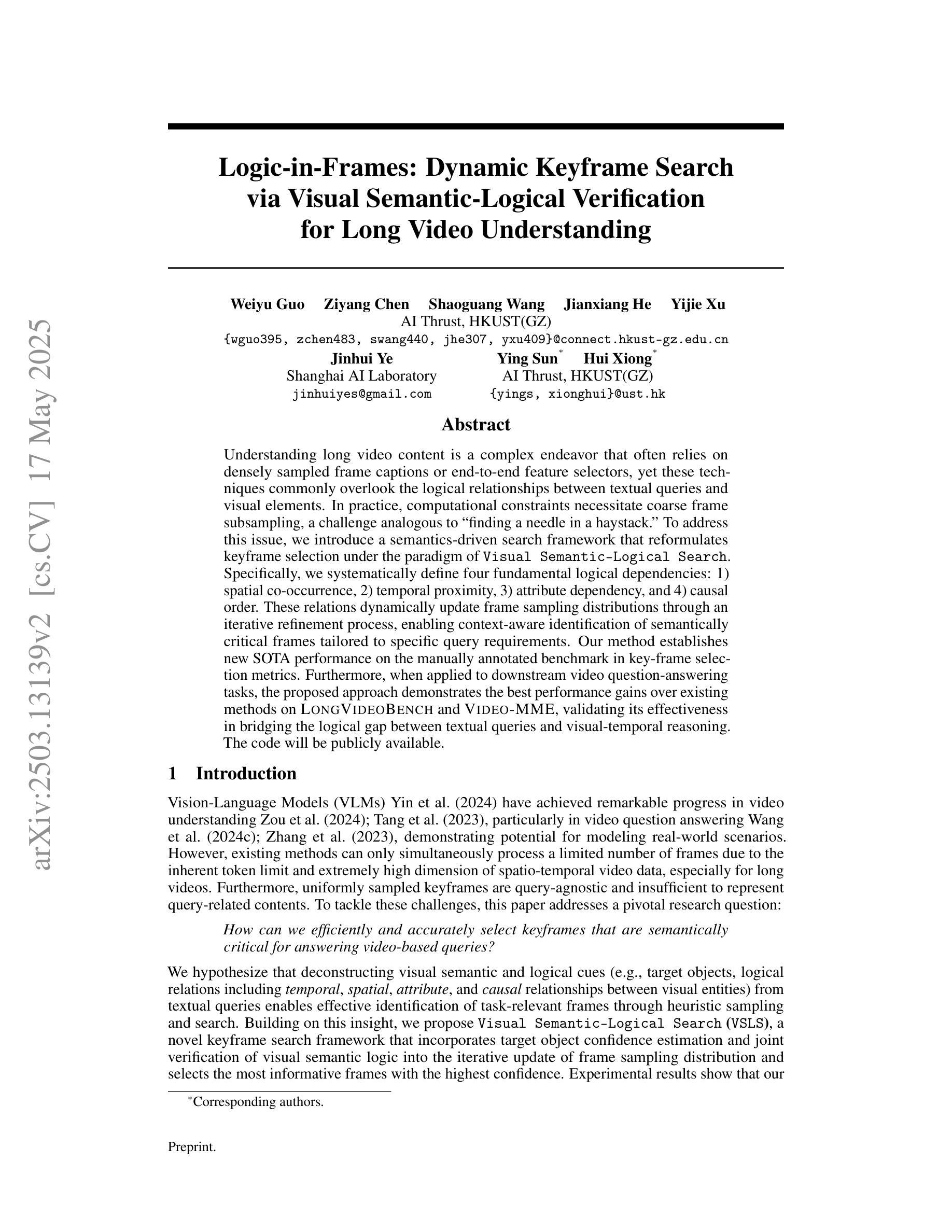
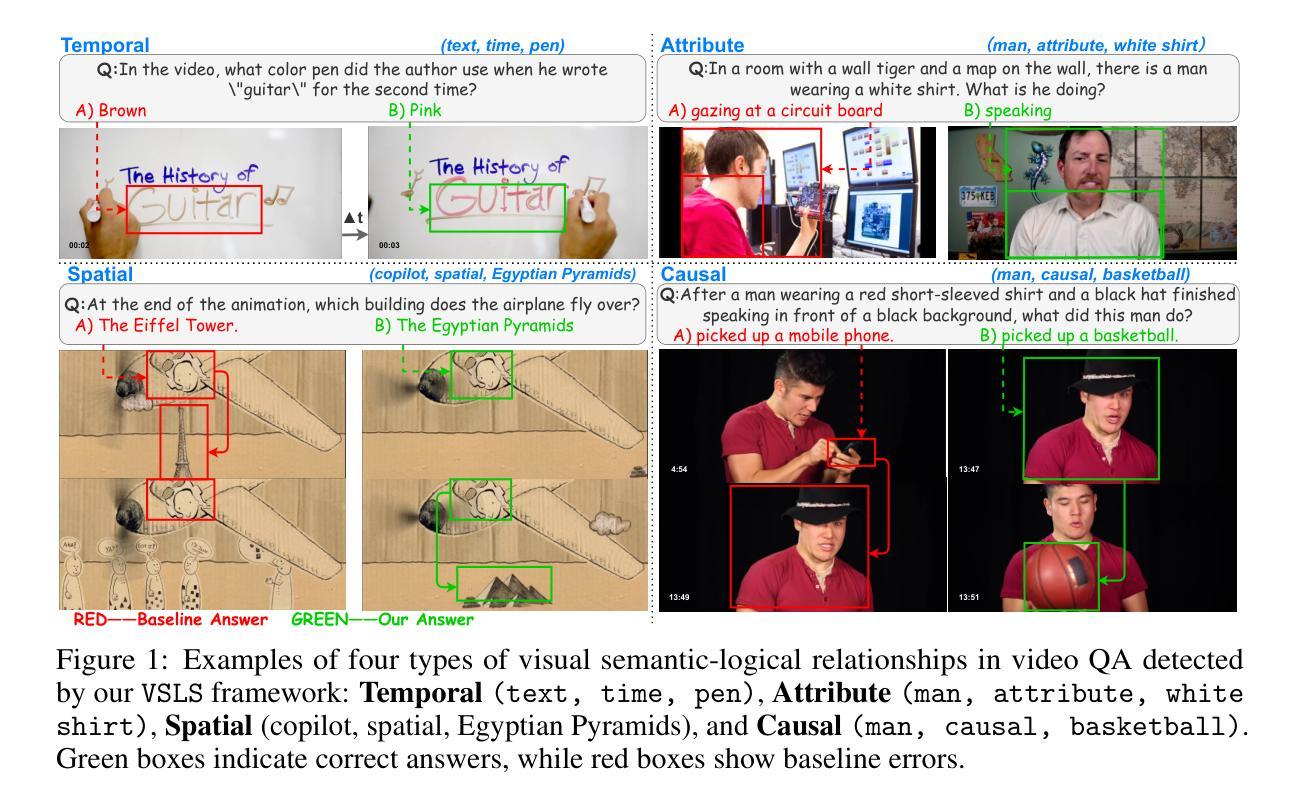
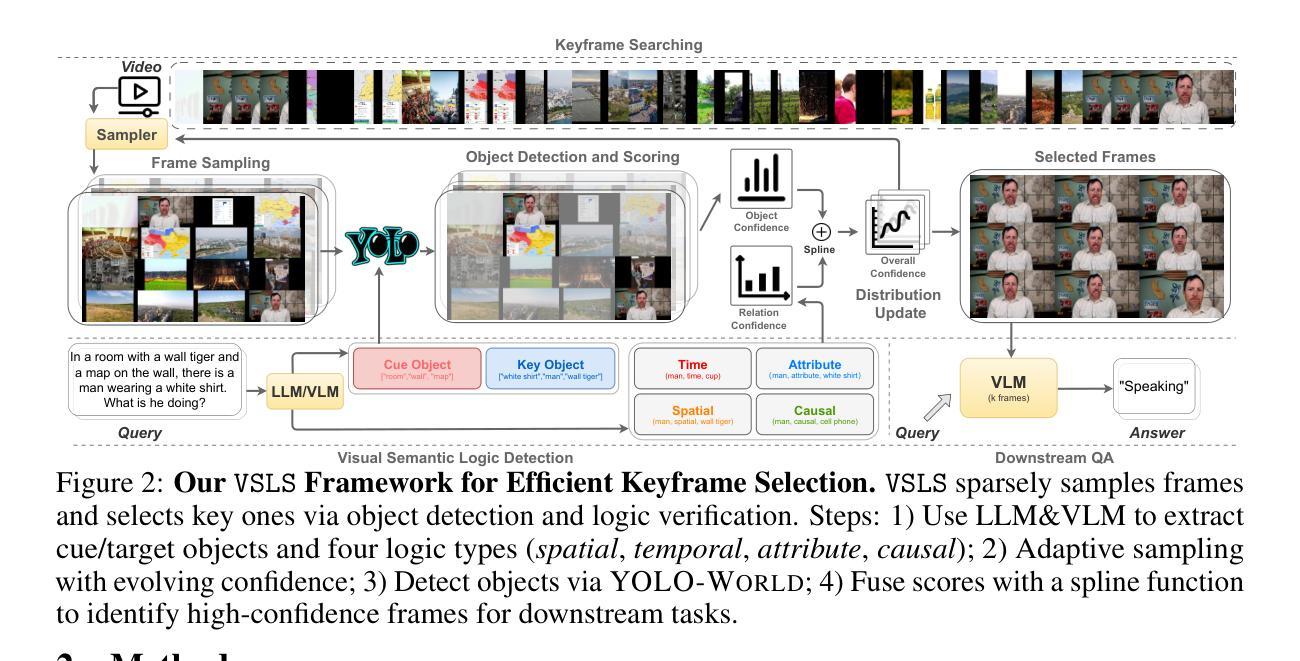
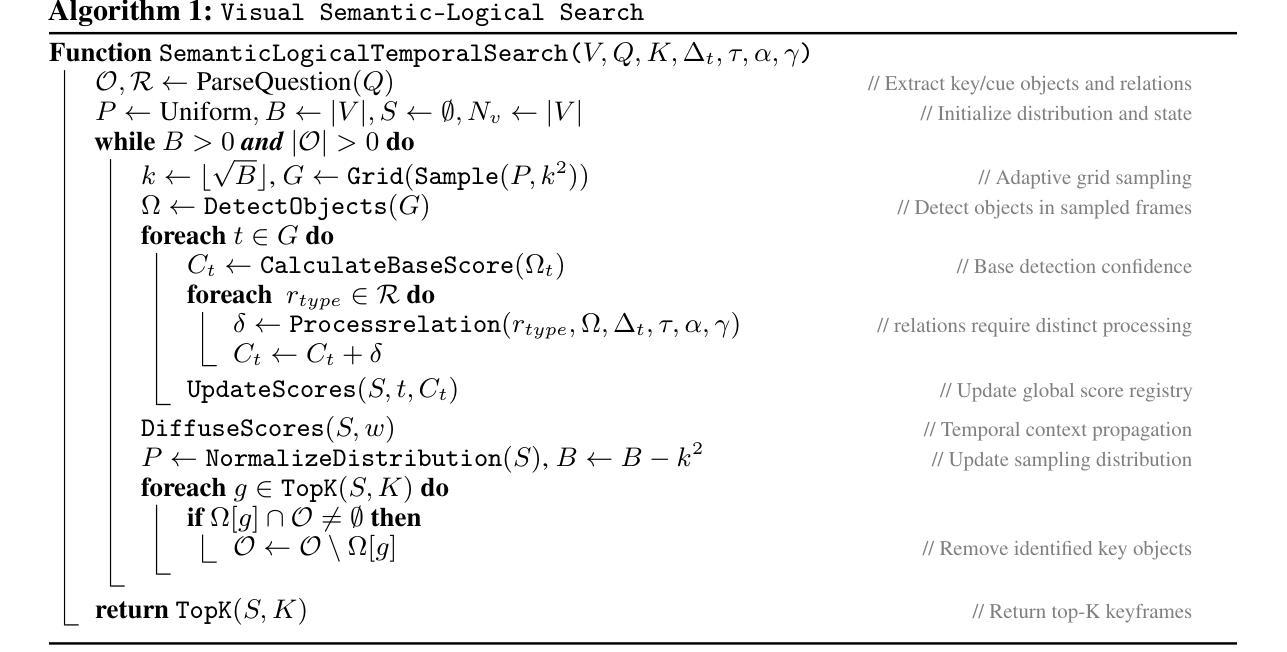
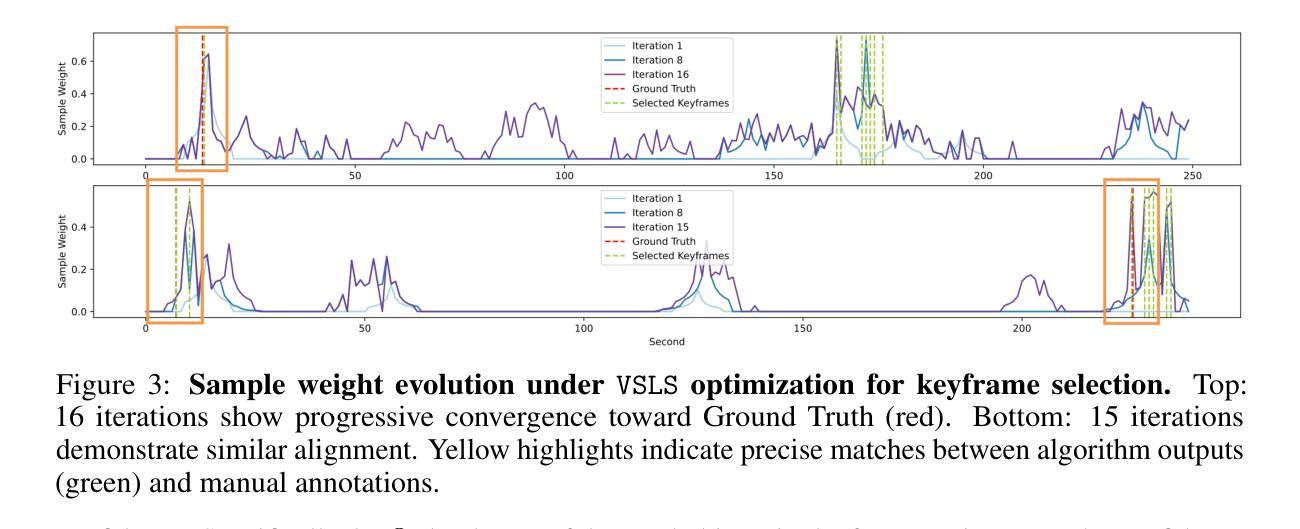