⚠️ 以下所有内容总结都来自于 大语言模型的能力,如有错误,仅供参考,谨慎使用
🔴 请注意:千万不要用于严肃的学术场景,只能用于论文阅读前的初筛!
💗 如果您觉得我们的项目对您有帮助 ChatPaperFree ,还请您给我们一些鼓励!⭐️ HuggingFace免费体验
2025-05-21 更新
Unified Cross-modal Translation of Score Images, Symbolic Music, and Performance Audio
Authors:Jongmin Jung, Dongmin Kim, Sihun Lee, Seola Cho, Hyungjoon Soh, Irmak Bukey, Chris Donahue, Dasaem Jeong
Music exists in various modalities, such as score images, symbolic scores, MIDI, and audio. Translations between each modality are established as core tasks of music information retrieval, such as automatic music transcription (audio-to-MIDI) and optical music recognition (score image to symbolic score). However, most past work on multimodal translation trains specialized models on individual translation tasks. In this paper, we propose a unified approach, where we train a general-purpose model on many translation tasks simultaneously. Two key factors make this unified approach viable: a new large-scale dataset and the tokenization of each modality. Firstly, we propose a new dataset that consists of more than 1,300 hours of paired audio-score image data collected from YouTube videos, which is an order of magnitude larger than any existing music modal translation datasets. Secondly, our unified tokenization framework discretizes score images, audio, MIDI, and MusicXML into a sequence of tokens, enabling a single encoder-decoder Transformer to tackle multiple cross-modal translation as one coherent sequence-to-sequence task. Experimental results confirm that our unified multitask model improves upon single-task baselines in several key areas, notably reducing the symbol error rate for optical music recognition from 24.58% to a state-of-the-art 13.67%, while similarly substantial improvements are observed across the other translation tasks. Notably, our approach achieves the first successful score-image-conditioned audio generation, marking a significant breakthrough in cross-modal music generation.
音乐存在于多种模态中,如乐谱图像、符号乐谱、MIDI和音乐音频。音乐信息检索中的核心任务之一是建立各种模态之间的转换,如自动音乐转录(音频到MIDI)和乐谱识别(乐谱图像到符号乐谱)。然而,过去大多数关于多模态转换的工作都是在各个转换任务上训练专门的模型。在本文中,我们提出了一种通用的方法,该方法可以在多个翻译任务上同时训练一个通用模型。两个关键因素使这种统一的方法可行:一是新的大规模数据集,二是每种模态的标记化。首先,我们提出了一个新的数据集,该数据集包含从YouTube视频收集的超过1300小时的配对音频和乐谱图像数据,其规模比现有的音乐模态转换数据集大一个数量级。其次,我们的统一标记化框架将乐谱图像、音频、MIDI和MusicXML离散成一系列标记,使得单个编码器-解码器Transformer能够将这些多模态转换作为一个连贯的序列到序列任务来解决。实验结果证实,我们的多任务统一模型在几个关键领域优于单任务基线模型,特别是将光学乐谱识别的符号错误率从24.58%降低到最先进的13.67%,而其他翻译任务也观察到了类似的实质性改进。值得注意的是,我们的方法实现了首次成功的乐谱图像条件下的音频生成,标志着跨模态音乐生成方面的一个重大突破。
论文及项目相关链接
PDF Submitted to IEEE Transactions on Audio, Speech and Language Processing (TASLPRO)
Summary
本文提出一种统一的多模态音乐翻译方法,该方法使用大规模数据集和统一的符号化框架,能够同时处理多种跨模态翻译任务。通过训练一个通用模型,实现了音乐模态之间的翻译,如音频转MIDI和乐谱图像转符号乐谱。新方法在多个关键领域优于单任务基准测试,显著降低了光学音乐识别的符号错误率。此外,该方法还实现了首个基于乐谱图像条件的音频生成,是跨模态音乐生成的一大突破。
Key Takeaways
- 提出一种多模态音乐翻译的统一方法,使用统一数据集和符号化框架。
- 引入大规模数据集,包含超过1,300小时的配对音频和乐谱图像数据。
- 通过统一的符号化框架,实现了不同音乐模态的离散化表示。
- 使用单一的编码器-解码器Transformer模型处理多个跨模态翻译任务。
- 与单任务基准测试相比,新方法在多个关键领域有显著改善。
- 在光学音乐识别方面,新方法降低了符号错误率至13.67%,达到最新水平。
点此查看论文截图
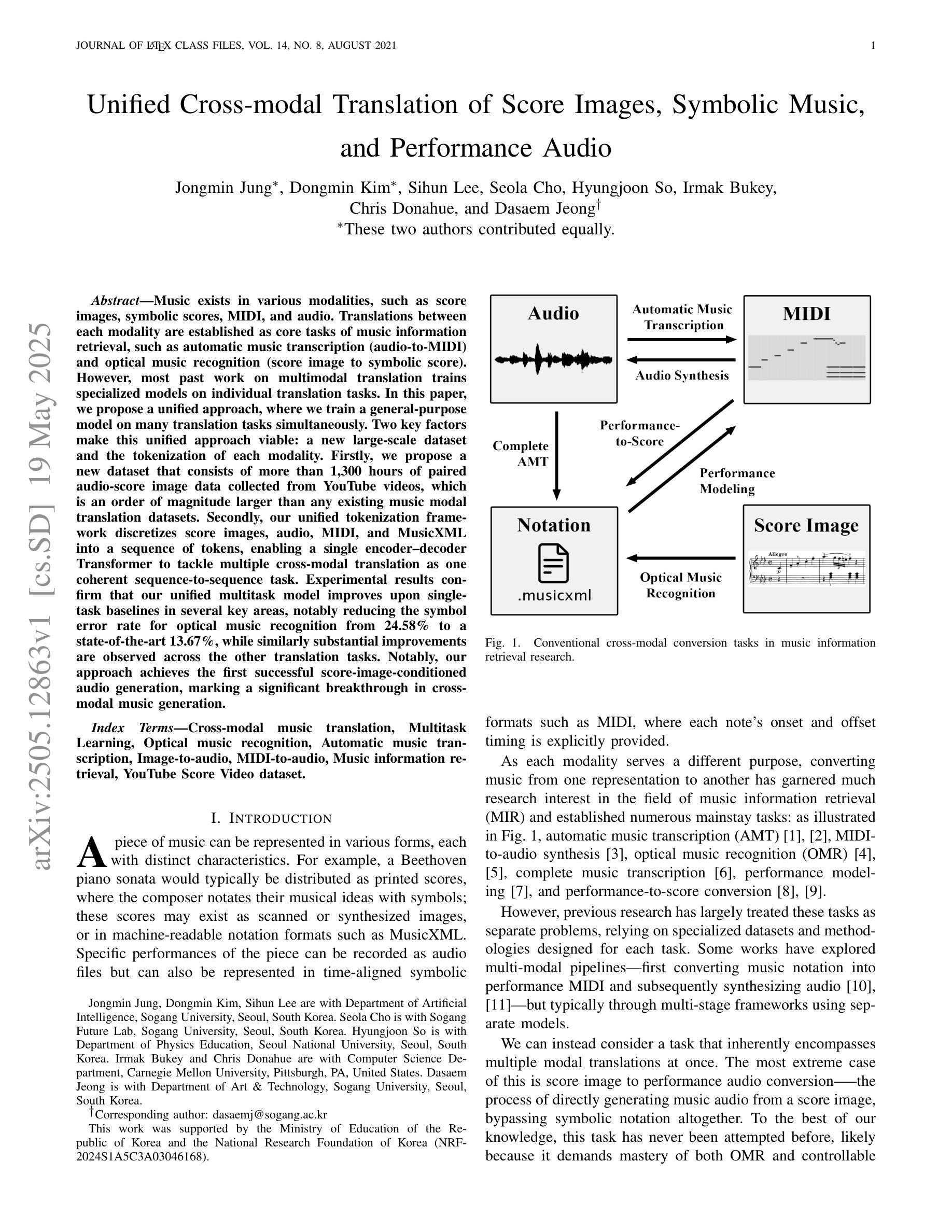
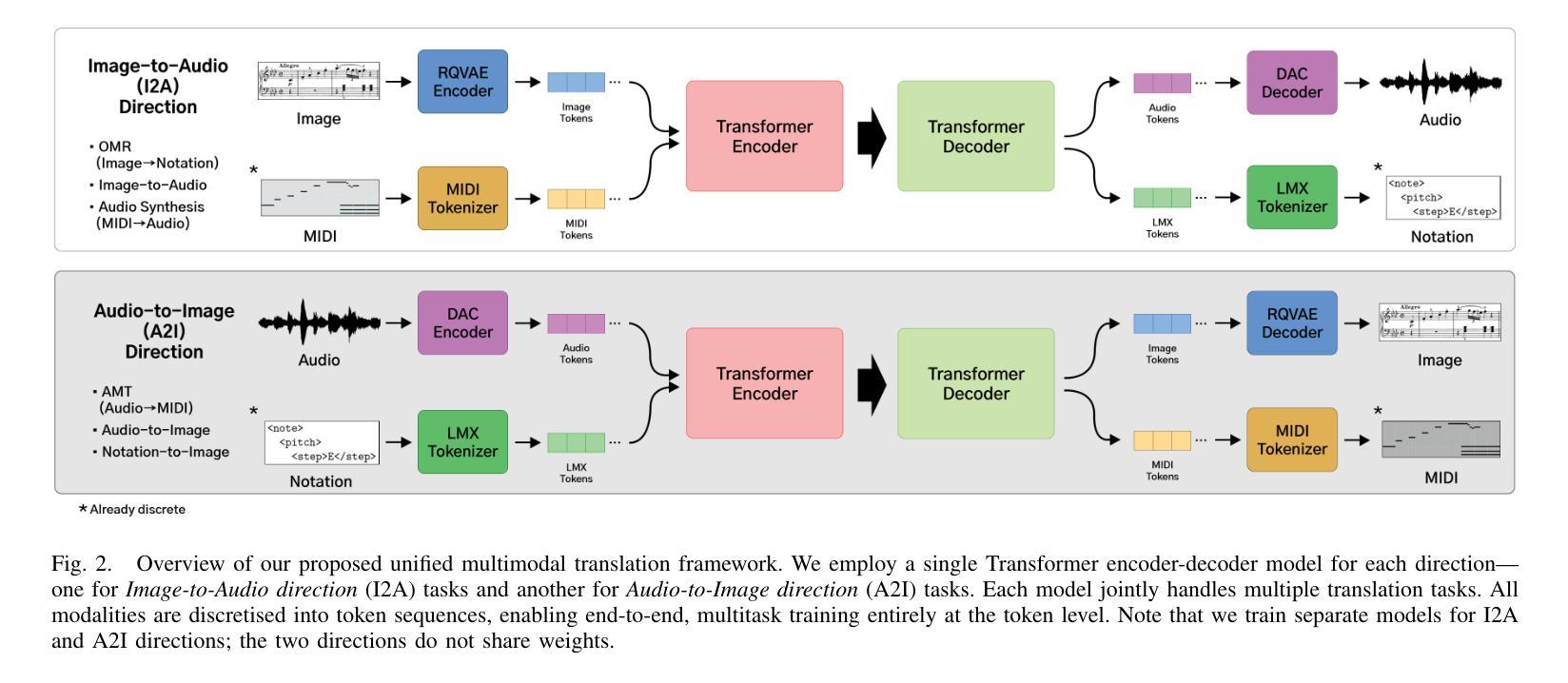
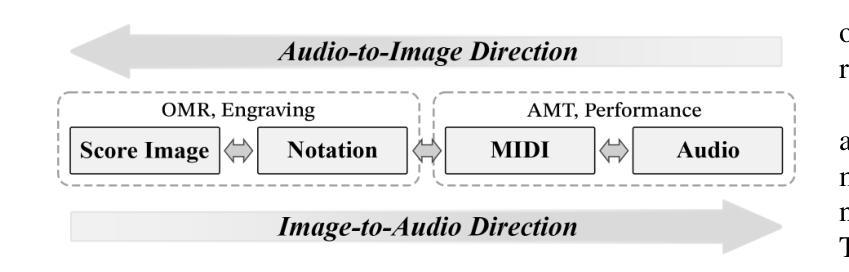
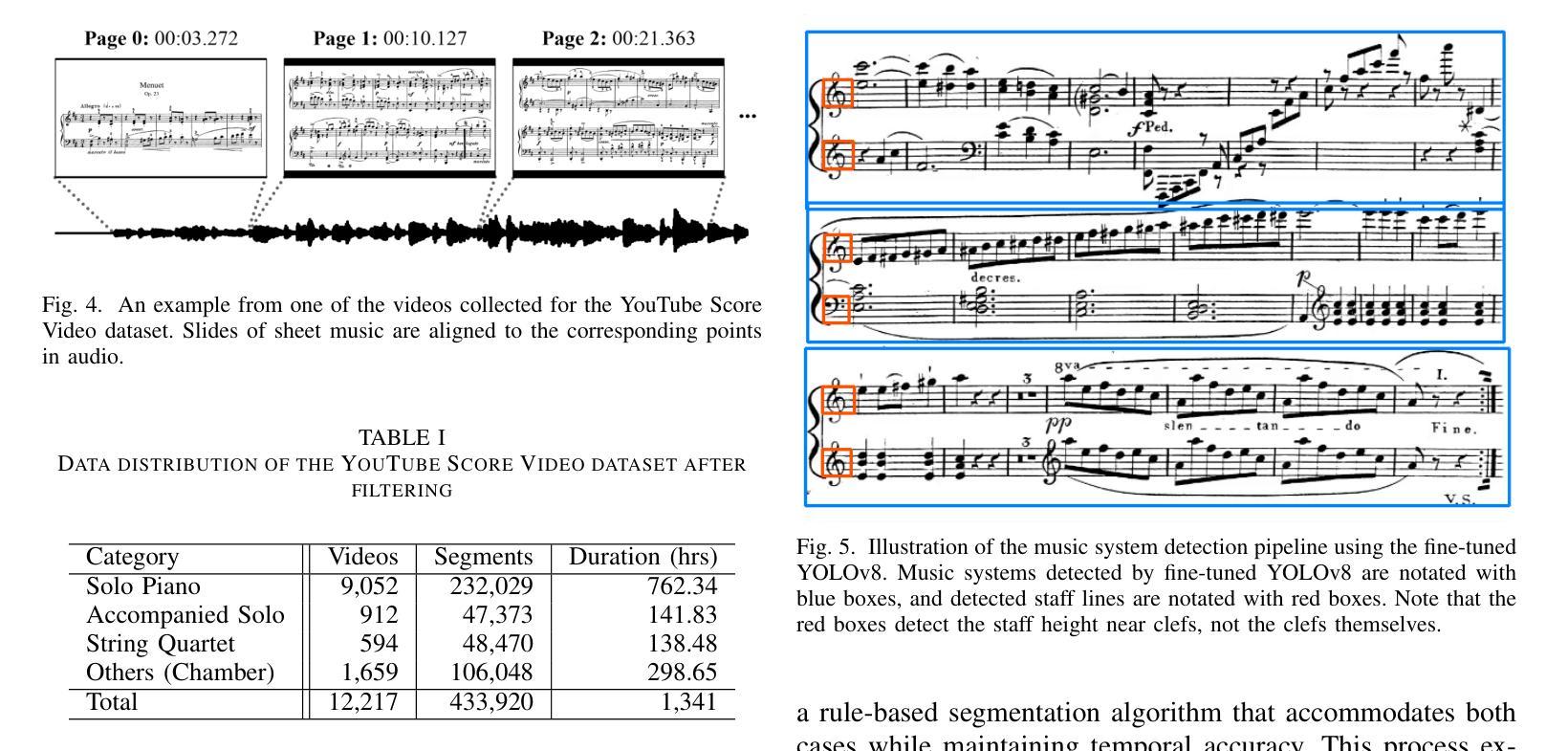
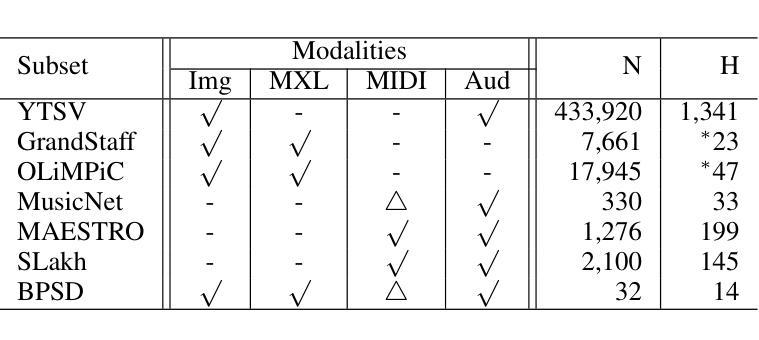