⚠️ 以下所有内容总结都来自于 大语言模型的能力,如有错误,仅供参考,谨慎使用
🔴 请注意:千万不要用于严肃的学术场景,只能用于论文阅读前的初筛!
💗 如果您觉得我们的项目对您有帮助 ChatPaperFree ,还请您给我们一些鼓励!⭐️ HuggingFace免费体验
2025-05-22 更新
Confidence-Regulated Generative Diffusion Models for Reliable AI Agent Migration in Vehicular Metaverses
Authors:Yingkai Kang, Jiawen Kang, Jinbo Wen, Tao Zhang, Zhaohui Yang, Dusit Niyato, Yan Zhang
Vehicular metaverses are an emerging paradigm that merges intelligent transportation systems with virtual spaces, leveraging advanced digital twin and Artificial Intelligence (AI) technologies to seamlessly integrate vehicles, users, and digital environments. In this paradigm, vehicular AI agents are endowed with environment perception, decision-making, and action execution capabilities, enabling real-time processing and analysis of multi-modal data to provide users with customized interactive services. Since vehicular AI agents require substantial resources for real-time decision-making, given vehicle mobility and network dynamics conditions, the AI agents are deployed in RoadSide Units (RSUs) with sufficient resources and dynamically migrated among them. However, AI agent migration requires frequent data exchanges, which may expose vehicular metaverses to potential cyber attacks. To this end, we propose a reliable vehicular AI agent migration framework, achieving reliable dynamic migration and efficient resource scheduling through cooperation between vehicles and RSUs. Additionally, we design a trust evaluation model based on the theory of planned behavior to dynamically quantify the reputation of RSUs, thereby better accommodating the personalized trust preferences of users. We then model the vehicular AI agent migration process as a partially observable markov decision process and develop a Confidence-regulated Generative Diffusion Model (CGDM) to efficiently generate AI agent migration decisions. Numerical results demonstrate that the CGDM algorithm significantly outperforms baseline methods in reducing system latency and enhancing robustness against cyber attacks.
车辆元宇宙是一种新兴范式,它将智能交通系统与虚拟空间相结合,利用先进的数字孪生和人工智能(AI)技术,无缝集成车辆、用户和数字环境。在这个范式中,车载AI代理被赋予环境感知、决策制定和行动执行的能力,能够实时处理和分析多模式数据,为用户提供定制的互动服务。鉴于车载AI代理需要大量资源来进行实时决策,考虑到车辆的移动性和网络动态条件,AI代理被部署在资源充足的路边单元(RSUs)中,并在它们之间进行动态迁移。然而,AI代理迁移需要频繁的数据交换,这可能会使车辆元宇宙面临潜在的网络攻击风险。为此,我们提出了一种可靠的车载AI代理迁移框架,通过车辆和RSUs之间的合作,实现可靠的动态迁移和高效的资源调度。此外,我们基于计划行为理论设计了一个信任评估模型,以动态量化RSUs的声誉,从而更好地满足用户的个性化信任偏好。随后,我们将车载AI代理迁移过程建模为部分可观察的马尔可夫决策过程,并开发了一种信心调节生成扩散模型(CGDM)来有效生成AI代理迁移决策。数值结果表明,CGDM算法在减少系统延迟和提高对网络攻击的鲁棒性方面显著优于基线方法。
论文及项目相关链接
Summary
本文介绍了车辆元宇宙这一新兴概念,它融合了智能交通系统和虚拟空间,利用数字孪生和人工智能(AI)技术无缝集成车辆、用户和数字环境。车辆AI代理被赋予环境感知、决策制定和行动执行的能力,以实时处理和分析多模式数据,为用户提供定制交互服务。文章提出了可靠的车辆AI代理迁移框架和信任评估模型,实现了可靠的动态迁移、高效的资源调度和用户个性化信任偏好的适应。同时,将车辆AI代理迁移过程建模为部分可观测的马尔可夫决策过程,并开发了信心调节生成扩散模型(CGDM)以高效生成AI代理迁移决策。
Key Takeaways
- 车辆元宇宙是智能交通系统与虚拟空间的融合,利用数字孪生和AI技术集成车辆、用户和数字环境。
- 车辆AI代理具有环境感知、决策制定和行动执行能力,能实时处理多模式数据。
- AI代理迁移框架支持动态迁移和高效资源调度。
- 信任评估模型基于计划行为理论,能动态量化路边单元(RSUs)的声誉,适应用户个性化信任偏好。
- 车辆AI代理迁移过程被建模为部分可观测的马尔可夫决策过程。
- 开发了CGDM模型以高效生成AI代理迁移决策。
点此查看论文截图
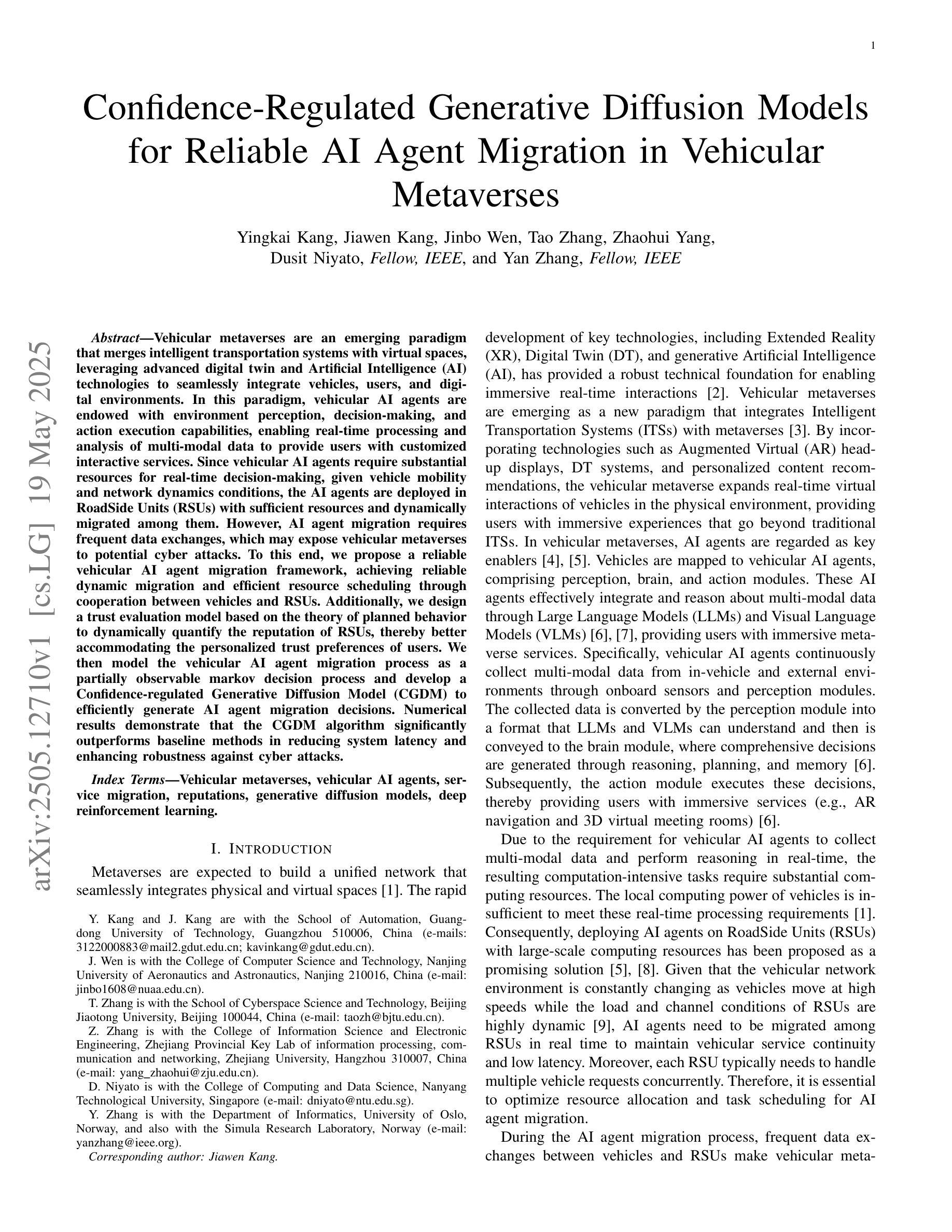
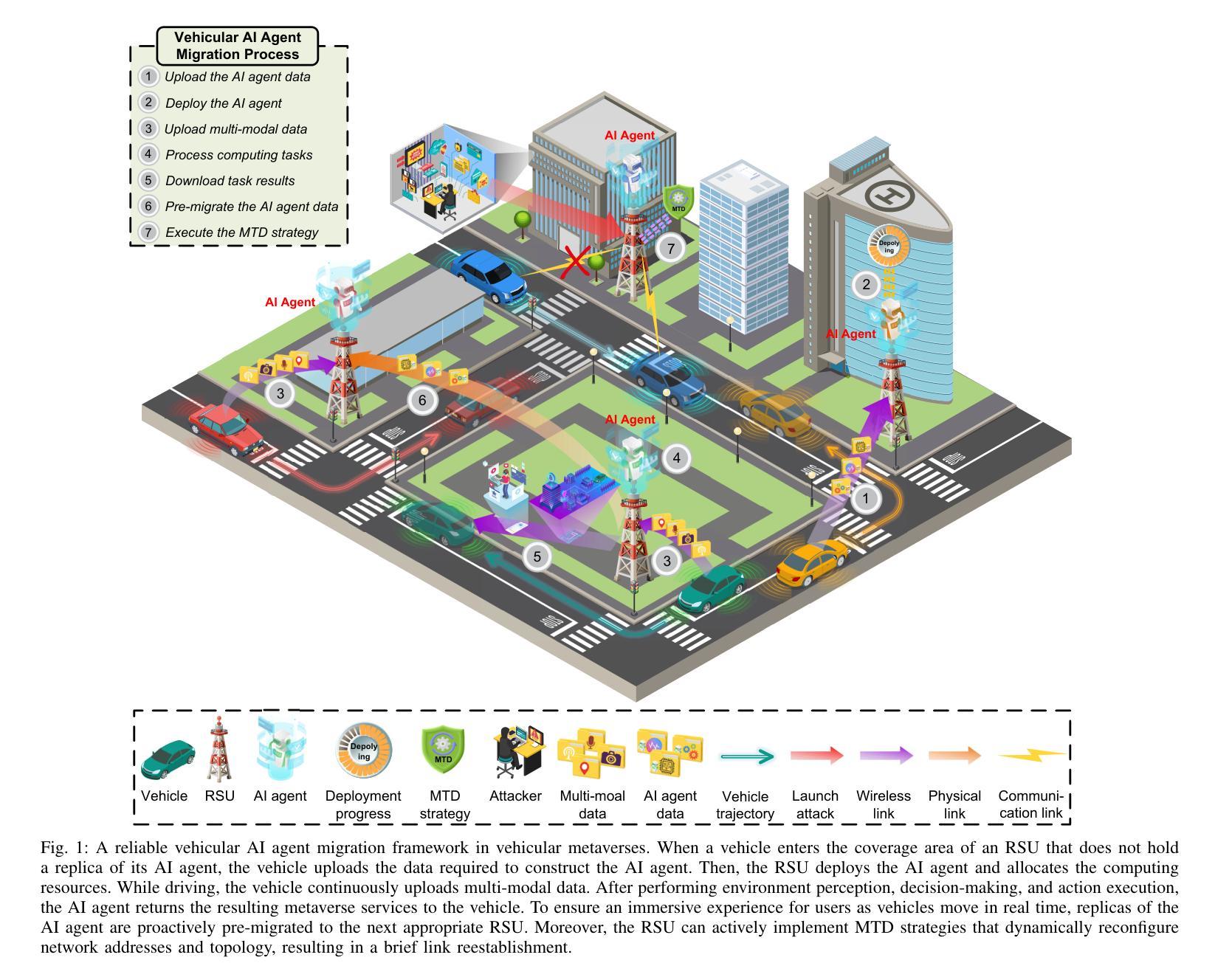
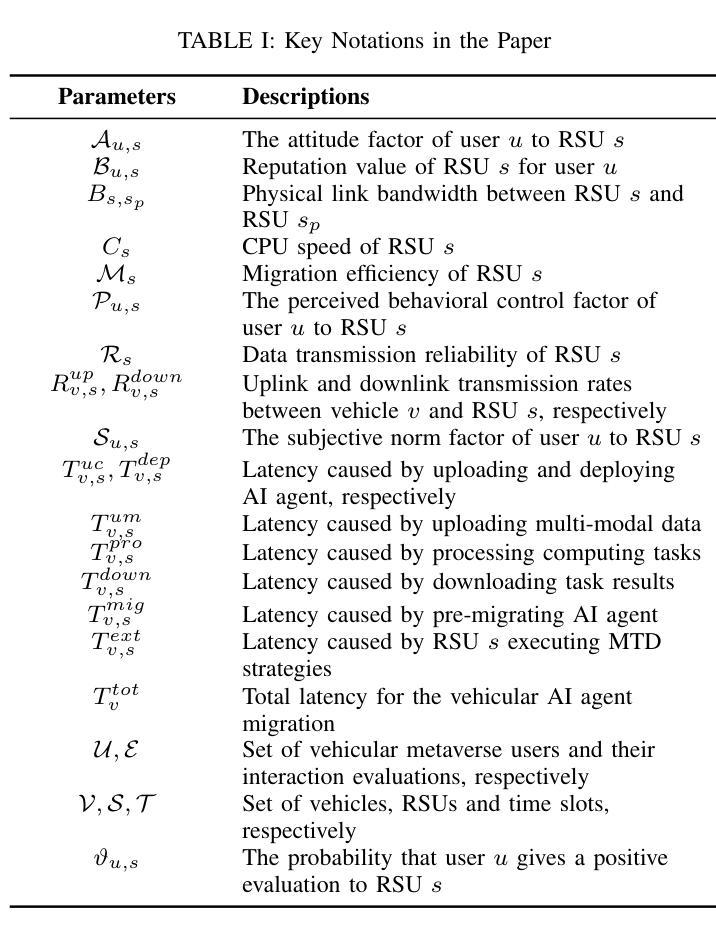