⚠️ 以下所有内容总结都来自于 大语言模型的能力,如有错误,仅供参考,谨慎使用
🔴 请注意:千万不要用于严肃的学术场景,只能用于论文阅读前的初筛!
💗 如果您觉得我们的项目对您有帮助 ChatPaperFree ,还请您给我们一些鼓励!⭐️ HuggingFace免费体验
2025-05-22 更新
Domain Adaptation of VLM for Soccer Video Understanding
Authors:Tiancheng Jiang, Henry Wang, Md Sirajus Salekin, Parmida Atighehchian, Shinan Zhang
Vision Language Models (VLMs) have demonstrated strong performance in multi-modal tasks by effectively aligning visual and textual representations. However, most video understanding VLM research has been domain-agnostic, leaving the understanding of their transfer learning capability to specialized domains under-explored. In this work, we address this by exploring the adaptability of open-source VLMs to specific domains, and focusing on soccer as an initial case study. Our approach uses large-scale soccer datasets and LLM to create instruction-following data, and use them to iteratively fine-tune the general-domain VLM in a curriculum learning fashion (first teaching the model key soccer concepts to then question answering tasks). The final adapted model, trained using a curated dataset of 20k video clips, exhibits significant improvement in soccer-specific tasks compared to the base model, with a 37.5% relative improvement for the visual question-answering task and an accuracy improvement from 11.8% to 63.5% for the downstream soccer action classification task.
视觉语言模型(VLMs)通过有效地对齐视觉和文本表示,在多模态任务中表现出了强大的性能。然而,大多数视频理解VLM研究都是领域无关的,导致对其转移到特定领域的学习能力的研究不足。在这项工作中,我们通过探索开源VLMs对特定领域的适应性来解决这个问题,并以足球作为初步案例研究。我们的方法使用大规模的足球数据集和大型语言模型来创建指令跟随数据,并以课程学习的形式迭代微调通用领域的VLM(首先向模型教授关键的足球概念,然后进行问答任务)。最终模型使用精选的包含2万个视频剪辑的数据集进行训练,相较于基础模型在足球特定任务上表现出了显著的改进。对于视觉问答任务,相对改进率为37.5%;对于下游的足球动作分类任务,准确率从11.8%提高到了63.5%。
论文及项目相关链接
PDF 8 pages, 5 figures, accepted to the 11th IEEE International Workshop on Computer Vision in Sports (CVSports) at CVPR 2025; supplementary appendix included as ancillary PDF
Summary
本文主要研究了在特定领域(足球领域)下,开源的视觉语言模型(VLMs)的适应性问题。研究团队通过使用大规模的足球数据集与大型语言模型(LLM)生成指令数据,以迭代的方式对通用领域的VLM进行微调,首次教授模型关键的足球概念,然后进行问答任务。最终经过训练的模型在足球特定任务上表现出显著改进,视觉问答任务相对改进了37.5%,下游足球动作分类任务的准确率从11.8%提高到63.5%。
Key Takeaways
- VLMs在多模态任务中表现出强大的性能,通过有效对齐视觉和文本表示。
- 大多数视频理解VLM研究是领域无关的,对于其在特定领域的迁移学习能力了解不足。
- 研究聚焦于探索开源VLMs在特定领域(足球)的适应性。
- 使用大规模的足球数据集和大型语言模型(LLM)生成指令数据。
- 采用课程学习的方式,首先教授模型关键的足球概念,然后进行问答任务。
- 最终训练的模型在足球特定任务上表现出显著改进。
点此查看论文截图
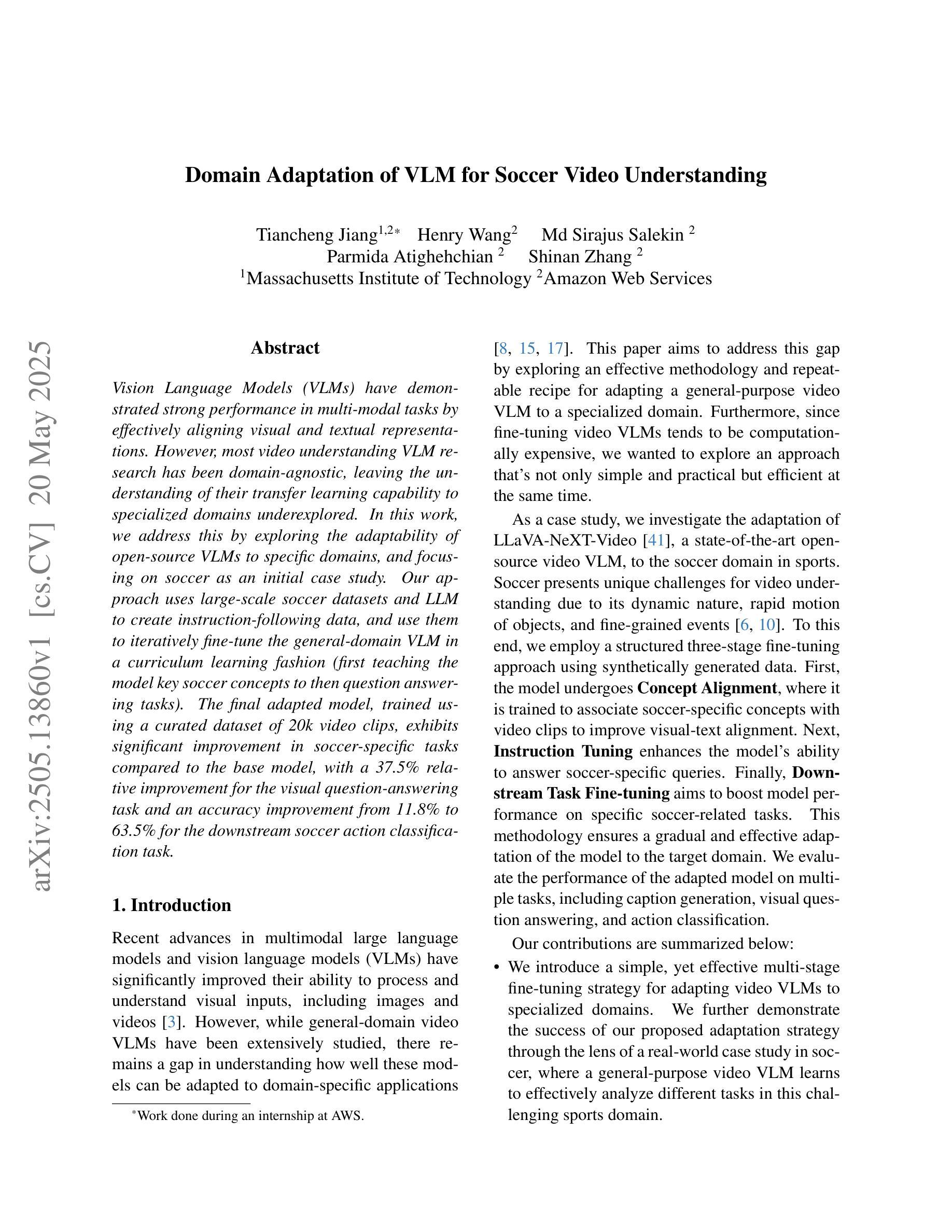
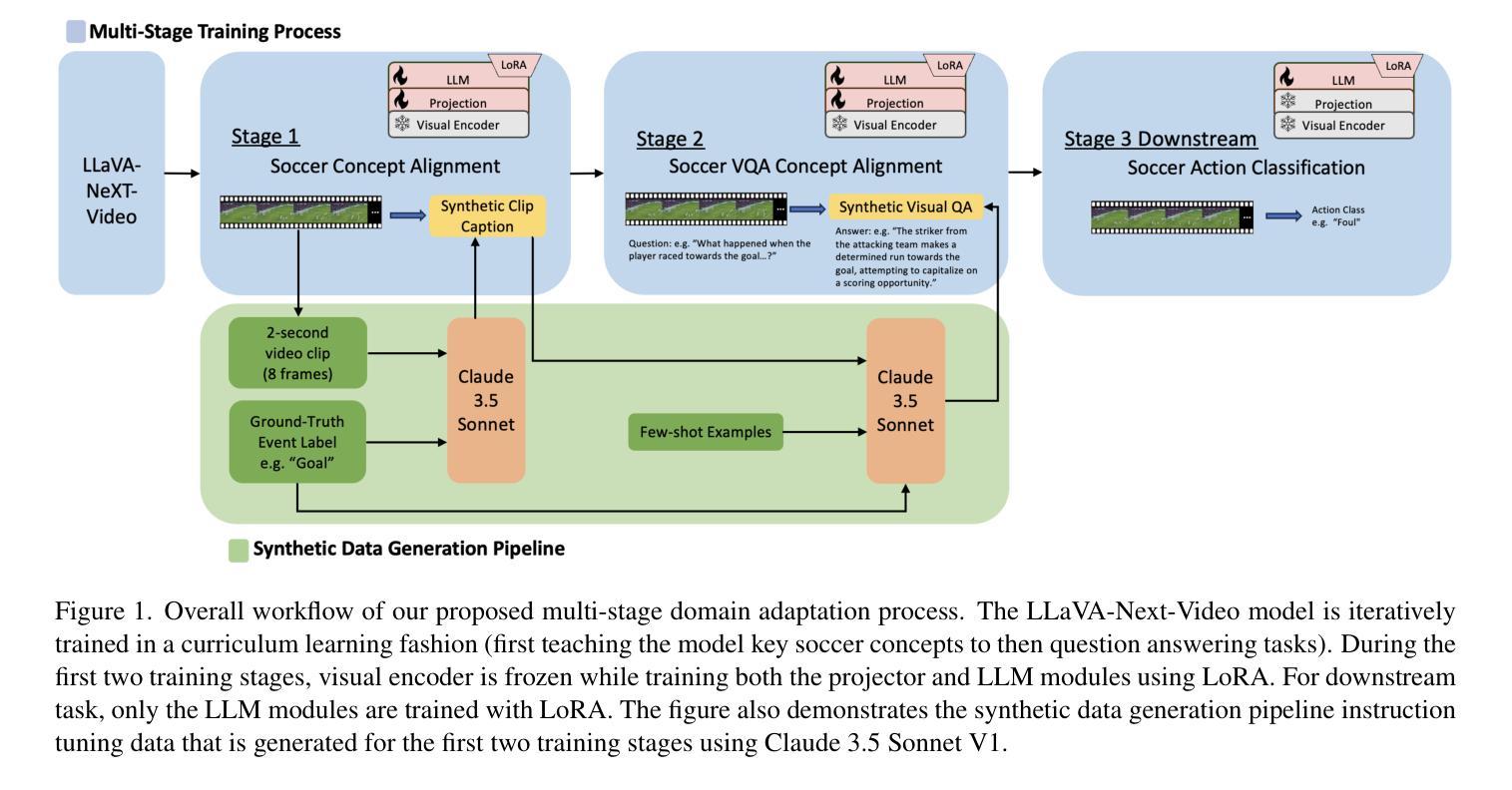
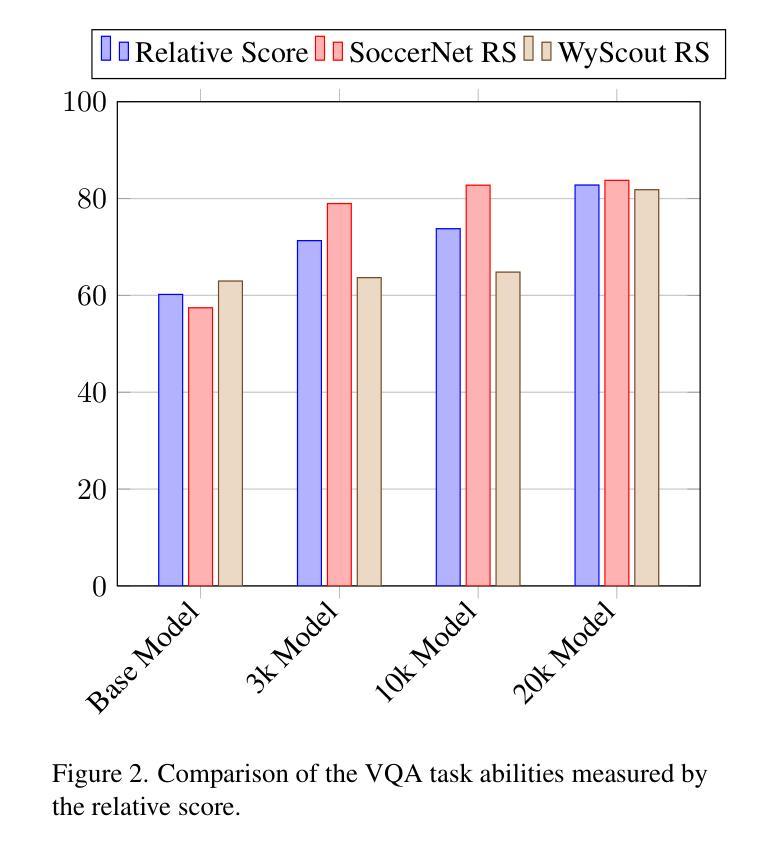
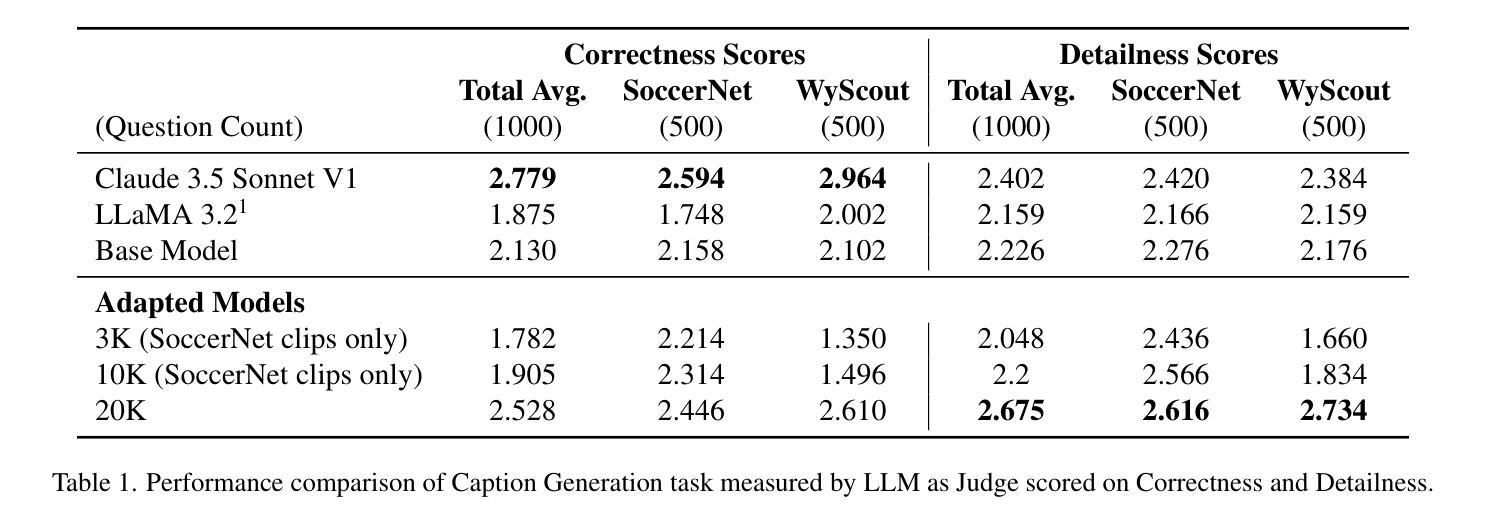