⚠️ 以下所有内容总结都来自于 大语言模型的能力,如有错误,仅供参考,谨慎使用
🔴 请注意:千万不要用于严肃的学术场景,只能用于论文阅读前的初筛!
💗 如果您觉得我们的项目对您有帮助 ChatPaperFree ,还请您给我们一些鼓励!⭐️ HuggingFace免费体验
2025-05-24 更新
Pose-invariant face recognition via feature-space pose frontalization
Authors:Nikolay Stanishev, Yuhang Lu, Touradj Ebrahimi
Pose-invariant face recognition has become a challenging problem for modern AI-based face recognition systems. It aims at matching a profile face captured in the wild with a frontal face registered in a database. Existing methods perform face frontalization via either generative models or learning a pose robust feature representation. In this paper, a new method is presented to perform face frontalization and recognition within the feature space. First, a novel feature space pose frontalization module (FSPFM) is proposed to transform profile images with arbitrary angles into frontal counterparts. Second, a new training paradigm is proposed to maximize the potential of FSPFM and boost its performance. The latter consists of a pre-training and an attention-guided fine-tuning stage. Moreover, extensive experiments have been conducted on five popular face recognition benchmarks. Results show that not only our method outperforms the state-of-the-art in the pose-invariant face recognition task but also maintains superior performance in other standard scenarios.
人脸姿态不变识别已成为现代基于人工智能的人脸识别系统中的一个具有挑战性的问题。它的目标是将在野外捕捉到的侧面人脸与数据库中注册的正脸进行匹配。现有方法通过生成模型或学习对姿态稳健的特征表示来实现人脸正面化。本文提出了一种在特征空间内实现人脸正面化和识别的新方法。首先,提出了一种新型特征空间人脸正面化模块(FSPFM),可将任意角度的侧面图像转换为正面图像。其次,提出了一种新的训练范式,以最大限度地发挥FSPFM的潜力并提高其性能。后者包括预训练阶段和注意力引导微调阶段。此外,已在五个流行的人脸识别基准测试上进行了广泛实验。结果表明,我们的方法不仅在姿态不变人脸识别任务上优于最新技术,而且在其他标准场景中也能保持卓越性能。
论文及项目相关链接
Summary
人脸识别中姿态不变性识别已成为现代人工智能人脸识别系统面临的挑战。该文提出了一种新方法,在特征空间内进行人脸转正和识别。首先提出了特征空间姿态转正模块(FSPFM),可将任意角度的侧面图像转化为正面图像。其次,为最大化FSPFM的潜力并提升其性能,提出了一种新的训练范式,包括预训练和注意力引导微调两个阶段。在五个流行的人脸识别基准测试上的实验结果表明,该方法不仅在姿态不变人脸识别任务上表现优异,而且在其他标准场景下也保持其优势。
Key Takeaways
- 特征空间姿态转正模块(FSPFM)可以将任意角度的侧面人脸图像转化为正面图像。
- 新的训练范式包括预训练和注意力引导微调两个阶段,以最大化FSPFM的潜力。
- 该方法在五个流行的人脸识别基准测试上的实验结果优于现有技术。
- 该方法不仅适用于姿态不变人脸识别任务,也适用于其他标准场景下的人脸识别。
- 现有方法主要通过生成模型或学习姿态稳健的特征表示来进行人脸转正识别,而该方法在特征空间内进行操作。
- FSPFM模块和新的训练范式共同构成了该方法的两大核心。
点此查看论文截图
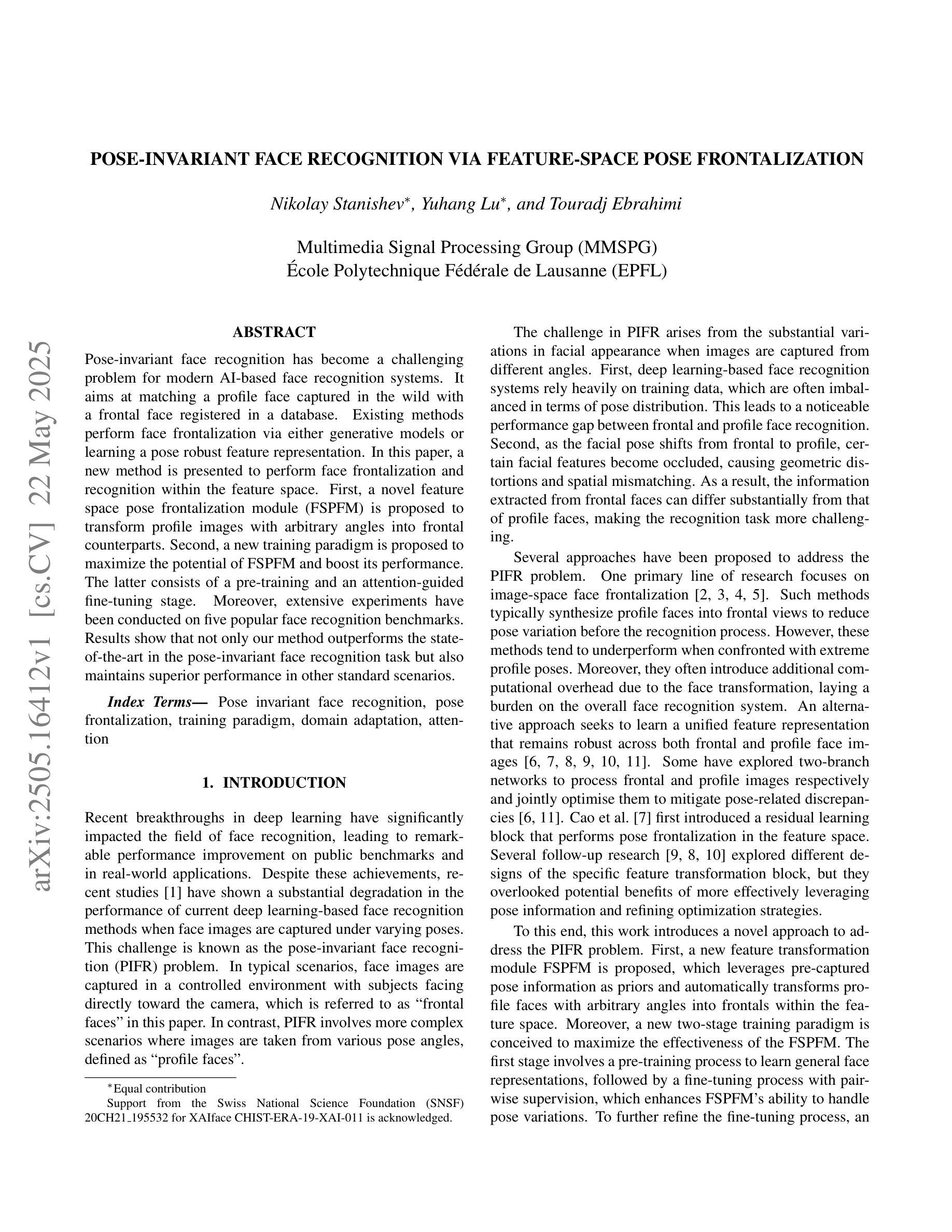
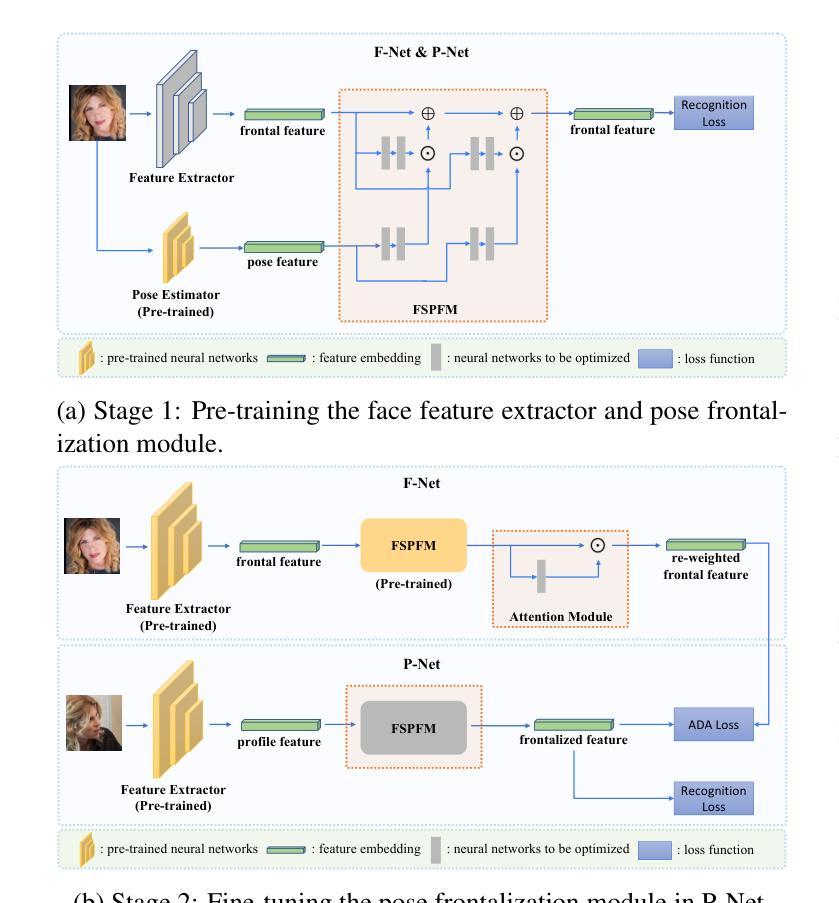
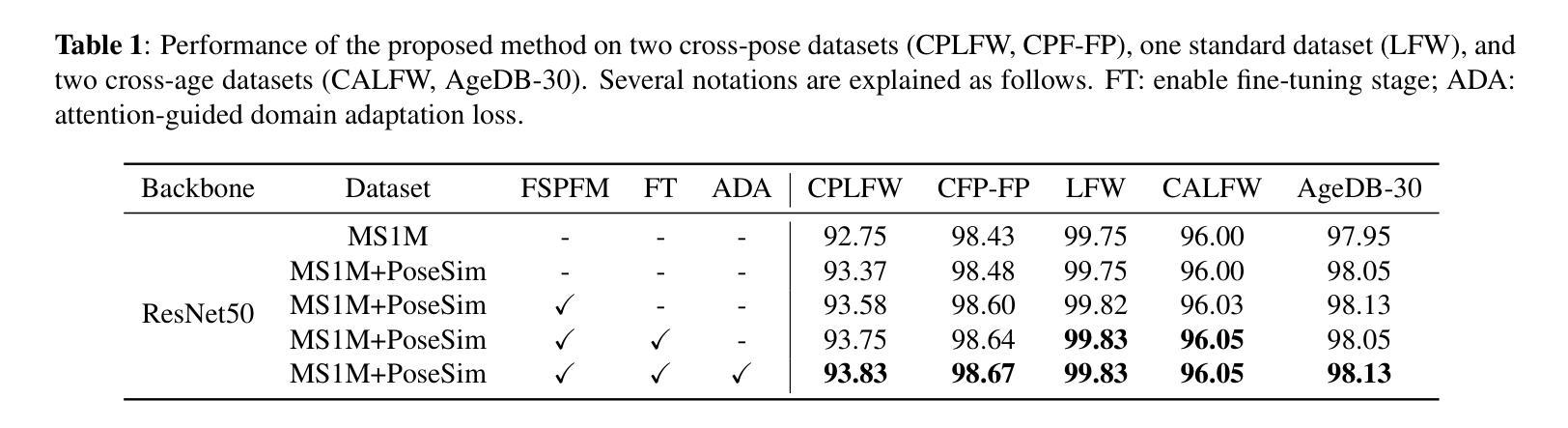
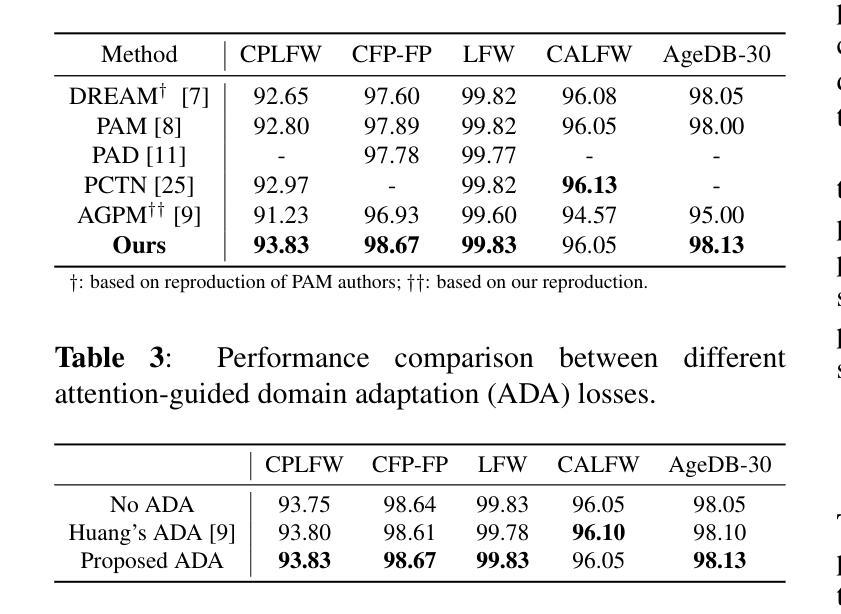
Pura: An Efficient Privacy-Preserving Solution for Face Recognition
Authors:Guotao Xu, Bowen Zhao, Yang Xiao, Yantao Zhong, Liang Zhai, Qingqi Pei
Face recognition is an effective technology for identifying a target person by facial images. However, sensitive facial images raises privacy concerns. Although privacy-preserving face recognition is one of potential solutions, this solution neither fully addresses the privacy concerns nor is efficient enough. To this end, we propose an efficient privacy-preserving solution for face recognition, named Pura, which sufficiently protects facial privacy and supports face recognition over encrypted data efficiently. Specifically, we propose a privacy-preserving and non-interactive architecture for face recognition through the threshold Paillier cryptosystem. Additionally, we carefully design a suite of underlying secure computing protocols to enable efficient operations of face recognition over encrypted data directly. Furthermore, we introduce a parallel computing mechanism to enhance the performance of the proposed secure computing protocols. Privacy analysis demonstrates that Pura fully safeguards personal facial privacy. Experimental evaluations demonstrate that Pura achieves recognition speeds up to 16 times faster than the state-of-the-art.
人脸识别是一种通过面部图像识别目标人物的有效技术。然而,敏感的面部图像会引发隐私担忧。虽然隐私保护人脸识别是潜在解决方案之一,但这种解决方案既不能完全解决隐私担忧,也不够高效。为此,我们提出了一种高效的人脸识别隐私保护解决方案,名为Pura,它充分保护面部隐私,并支持在加密数据上进行高效的人脸识别。具体来说,我们提出了一个基于Paillier门限密码系统的非交互式人脸识别隐私保护架构。此外,我们精心设计了一系列底层的安全计算协议,以实现在加密数据上直接进行高效的人脸识别操作。此外,我们还引入了一种并行计算机制,以提高所提出的安全计算协议的性能。隐私分析表明,Pura能够完全保护个人面部隐私。实验评估表明,Pura的识别速度比最新技术最快可达16倍。
论文及项目相关链接
Summary
人脸识别技术通过面部图像有效识别目标人物,但敏感面部图像引发隐私担忧。为解决这一问题,我们提出了一种高效的人脸识别隐私保护方案——Pura,它既能充分保护面部隐私,又能支持对加密数据的快速人脸识别。通过基于阈值的Paillier密码系统,我们设计了一种非交互式架构,并构建了一系列底层的安全计算协议,确保在加密数据上实现高效的人脸识别操作。此外,引入了并行计算机制提升性能。隐私分析显示Pura能够全面保护个人面部隐私,实验评估显示其识别速度比现有技术最快可达16倍。
Key Takeaways
- 人脸识别技术有效但引发隐私担忧。
- Pura方案旨在解决人脸识别中的隐私保护问题。
- Pura利用基于阈值的Paillier密码系统,设计非交互式架构。
- Pura通过构建安全计算协议,支持在加密数据上高效人脸识别。
- Pura引入并行计算机制以提升性能。
- 隐私分析证实Pura能全面保护个人面部隐私。
点此查看论文截图
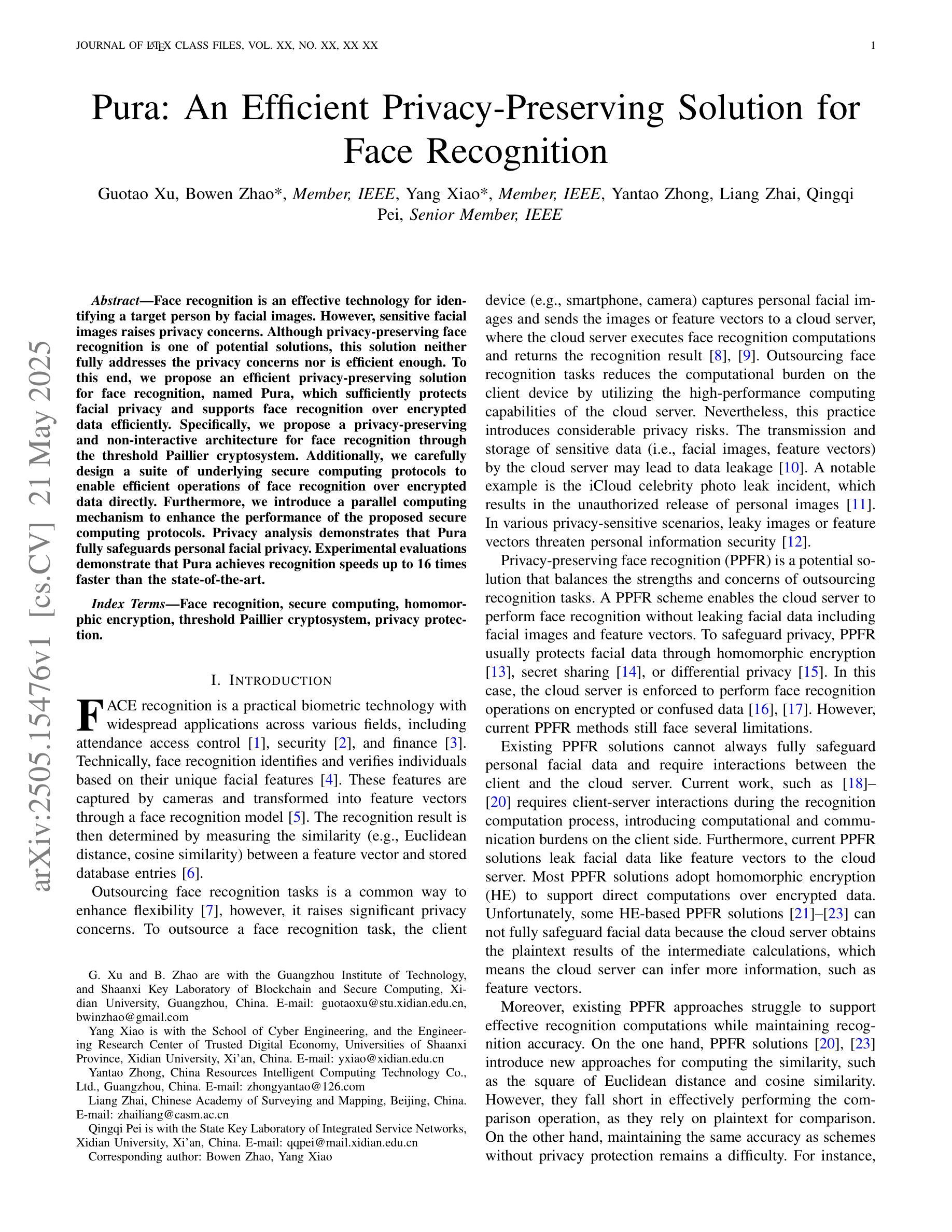
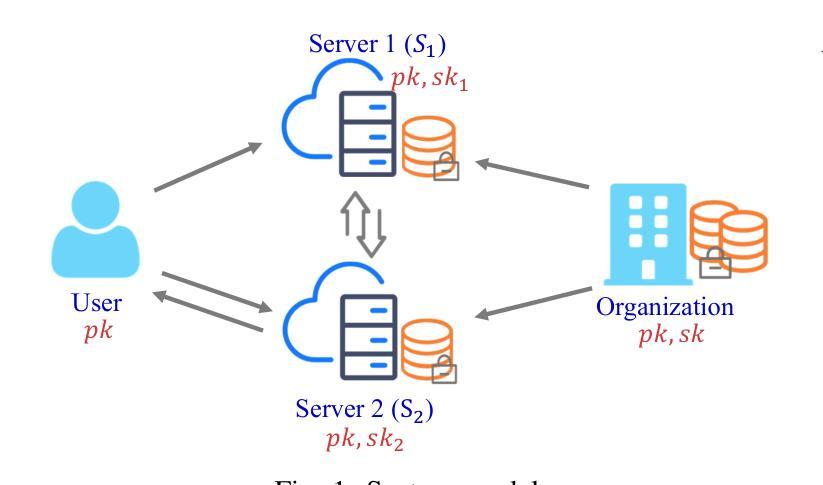
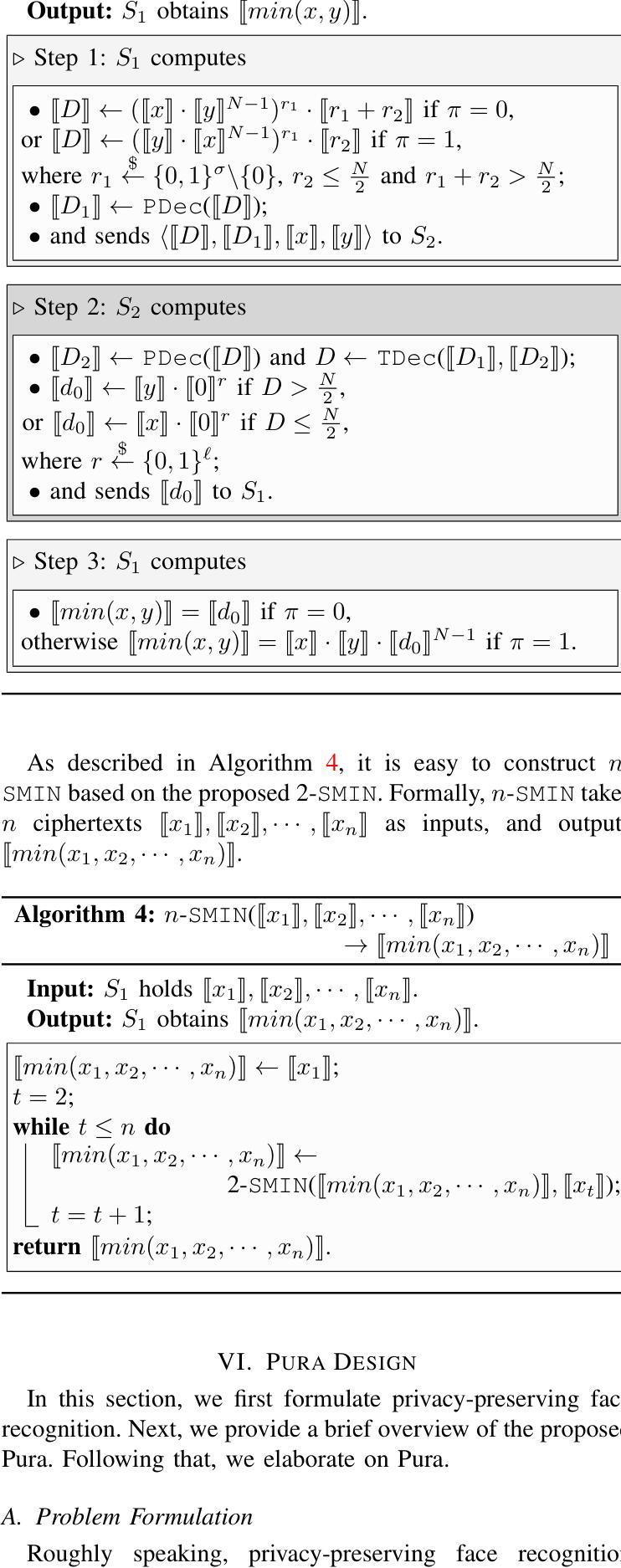
ErasableMask: A Robust and Erasable Privacy Protection Scheme against Black-box Face Recognition Models
Authors:Sipeng Shen, Yunming Zhang, Dengpan Ye, Xiuwen Shi, Long Tang, Haoran Duan, Yueyun Shang, Zhihong Tian
While face recognition (FR) models have brought remarkable convenience in face verification and identification, they also pose substantial privacy risks to the public. Existing facial privacy protection schemes usually adopt adversarial examples to disrupt face verification of FR models. However, these schemes often suffer from weak transferability against black-box FR models and permanently damage the identifiable information that cannot fulfill the requirements of authorized operations such as forensics and authentication. To address these limitations, we propose ErasableMask, a robust and erasable privacy protection scheme against black-box FR models. Specifically, via rethinking the inherent relationship between surrogate FR models, ErasableMask introduces a novel meta-auxiliary attack, which boosts black-box transferability by learning more general features in a stable and balancing optimization strategy. It also offers a perturbation erasion mechanism that supports the erasion of semantic perturbations in protected face without degrading image quality. To further improve performance, ErasableMask employs a curriculum learning strategy to mitigate optimization conflicts between adversarial attack and perturbation erasion. Extensive experiments on the CelebA-HQ and FFHQ datasets demonstrate that ErasableMask achieves the state-of-the-art performance in transferability, achieving over 72% confidence on average in commercial FR systems. Moreover, ErasableMask also exhibits outstanding perturbation erasion performance, achieving over 90% erasion success rate.
人脸识别(FR)模型在面部验证和识别方面带来了极大的便利,但同时也给公众带来了严重的隐私风险。现有的面部隐私保护方案通常采用对抗性样本的方法来破坏人脸识别模型的面部验证。然而,这些方案往往对黑盒人脸识别模型的迁移能力较弱,并且永久破坏了可用于法医学和身份验证等授权操作的识别信息。为了解决这些局限性,我们提出了ErasableMask,这是一种针对黑盒人脸识别模型的稳健且可擦除的隐私保护方案。具体来说,通过重新思考代理人脸识别模型之间的内在关系,ErasableMask引入了一种新型的元辅助攻击,通过稳定且平衡的优化策略学习更通用的特征,提高了黑盒迁移能力。它还提供了一个扰动消除机制,可以在不降低图像质量的情况下,消除受保护面孔的语义扰动。为了进一步改进性能,ErasableMask采用了一种课程学习策略,以缓解对抗性攻击和扰动消除之间的优化冲突。在CelebA-HQ和FFHQ数据集上的大量实验表明,ErasableMask在迁移能力方面达到了最先进的性能,在商用人脸识别系统上的平均置信度超过72%。此外,ErasableMask还具有出色的扰动消除性能,消除成功率超过90%。
论文及项目相关链接
Summary
人脸识别技术带来便利的同时也存在隐私风险。为解决现有面部隐私保护方案对黑箱人脸识别模型转移性的局限性,提出ErableMask方案,该方案采用新型元辅助攻击技术提升模型在黑箱环境下的转移性,并引入扰动消除机制,可在保护面部隐私的同时保持图像质量。通过课程学习策略缓解优化冲突,实现高性能的隐私保护。
Key Takeaways
- 人脸识别技术虽便利但存在隐私风险。
- 现有面部隐私保护方案通常使用对抗性示例来干扰人脸识别模型的验证过程。
- 这些方案在黑箱人脸识别模型中的转移性较弱,且会永久破坏身份信息,无法满足授权操作的要求。
- ErasableMask方案旨在解决上述问题,采用元辅助攻击技术提高在黑箱环境中的转移性。
- ErasableMask引入扰动消除机制,可在保护面部隐私的同时保持图像质量。
- ErasableMask使用课程学习策略缓解优化冲突,实现高效的隐私保护性能。
点此查看论文截图
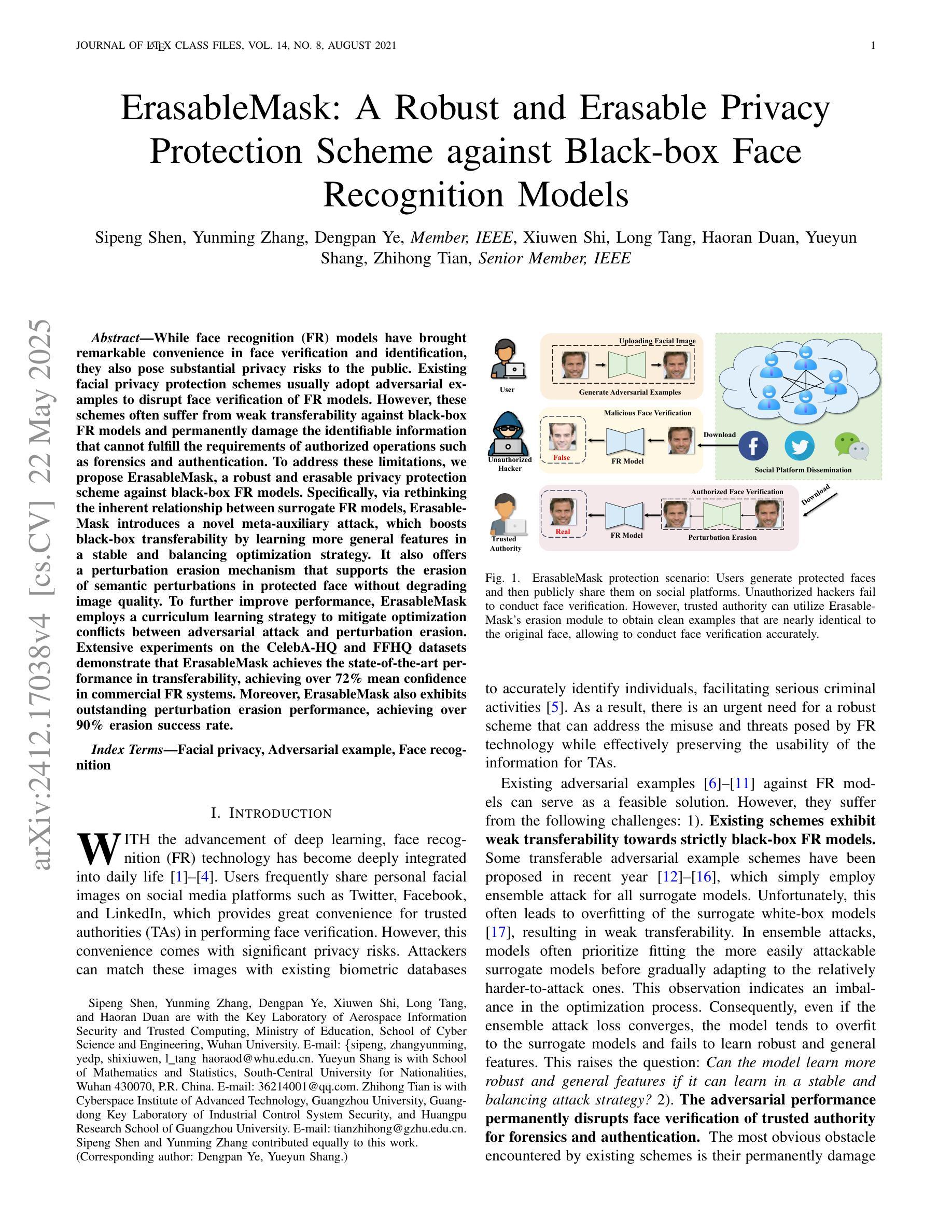
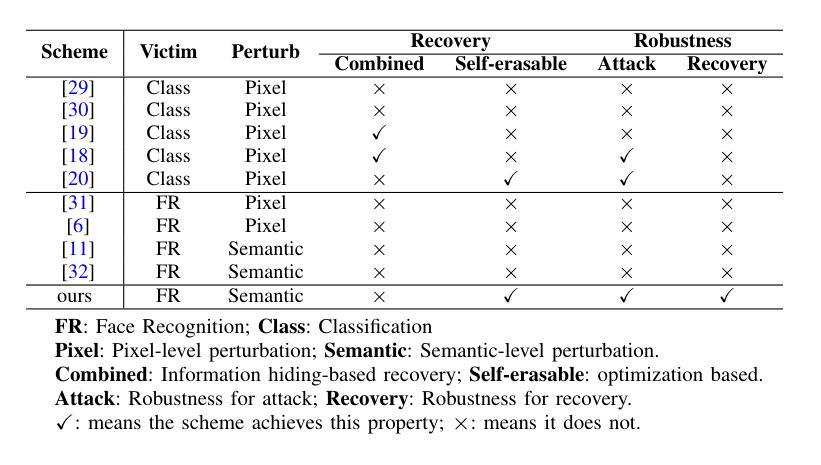
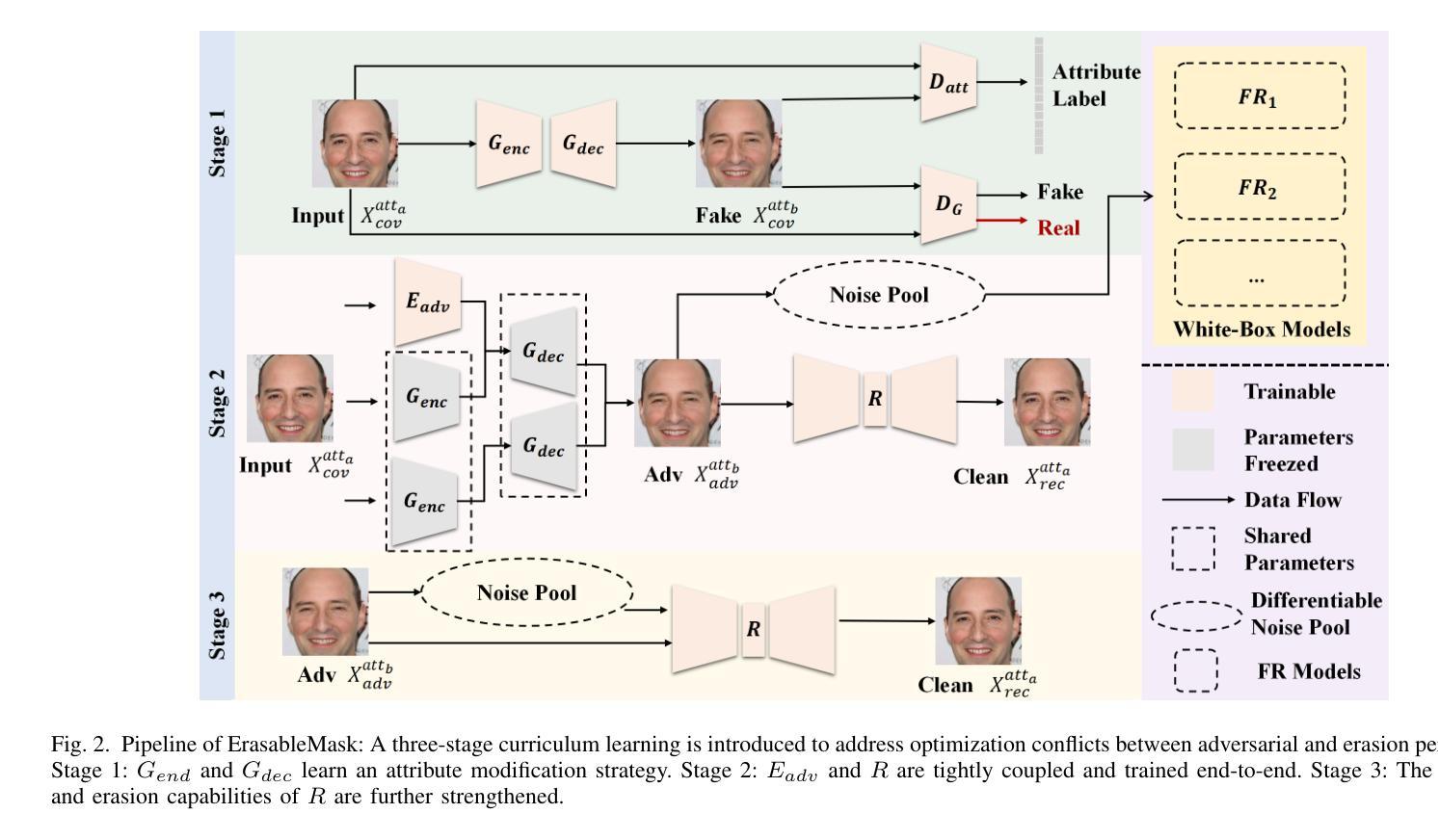
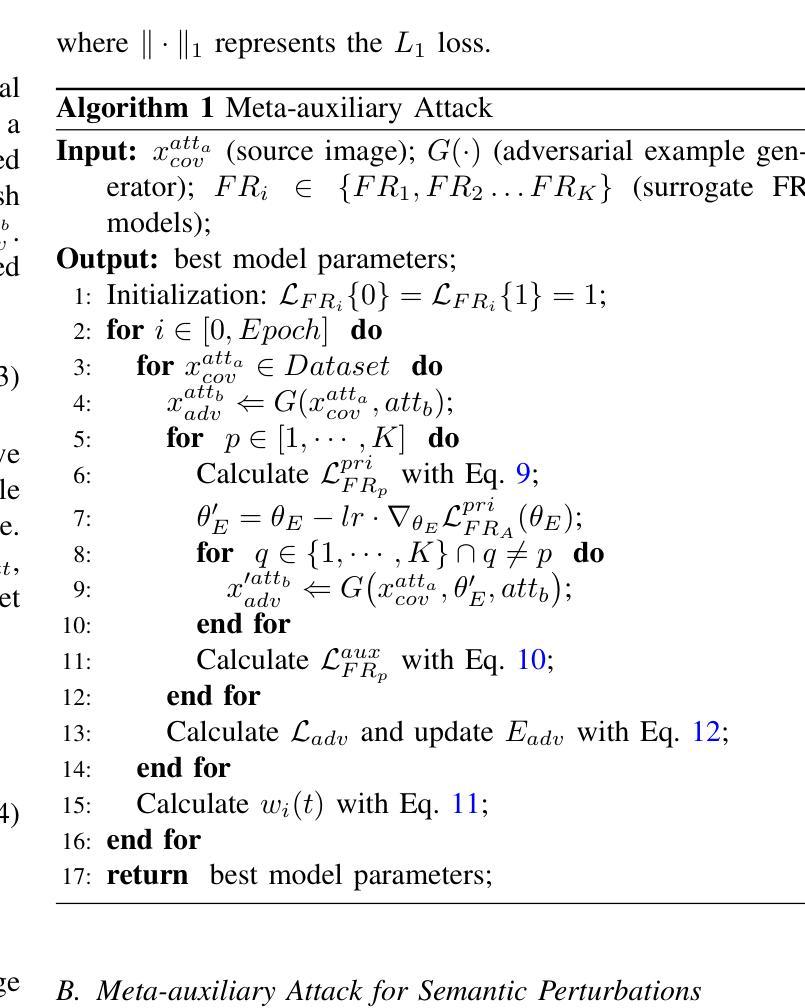
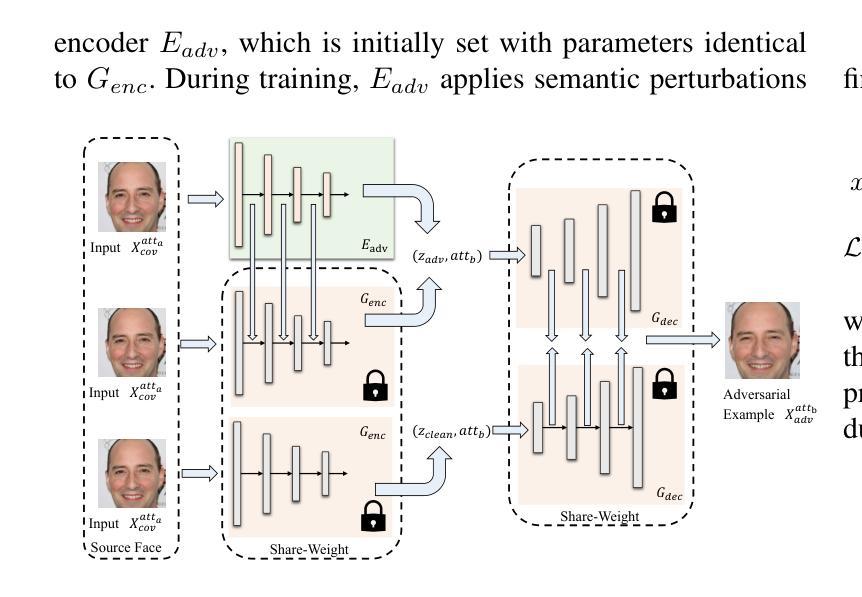
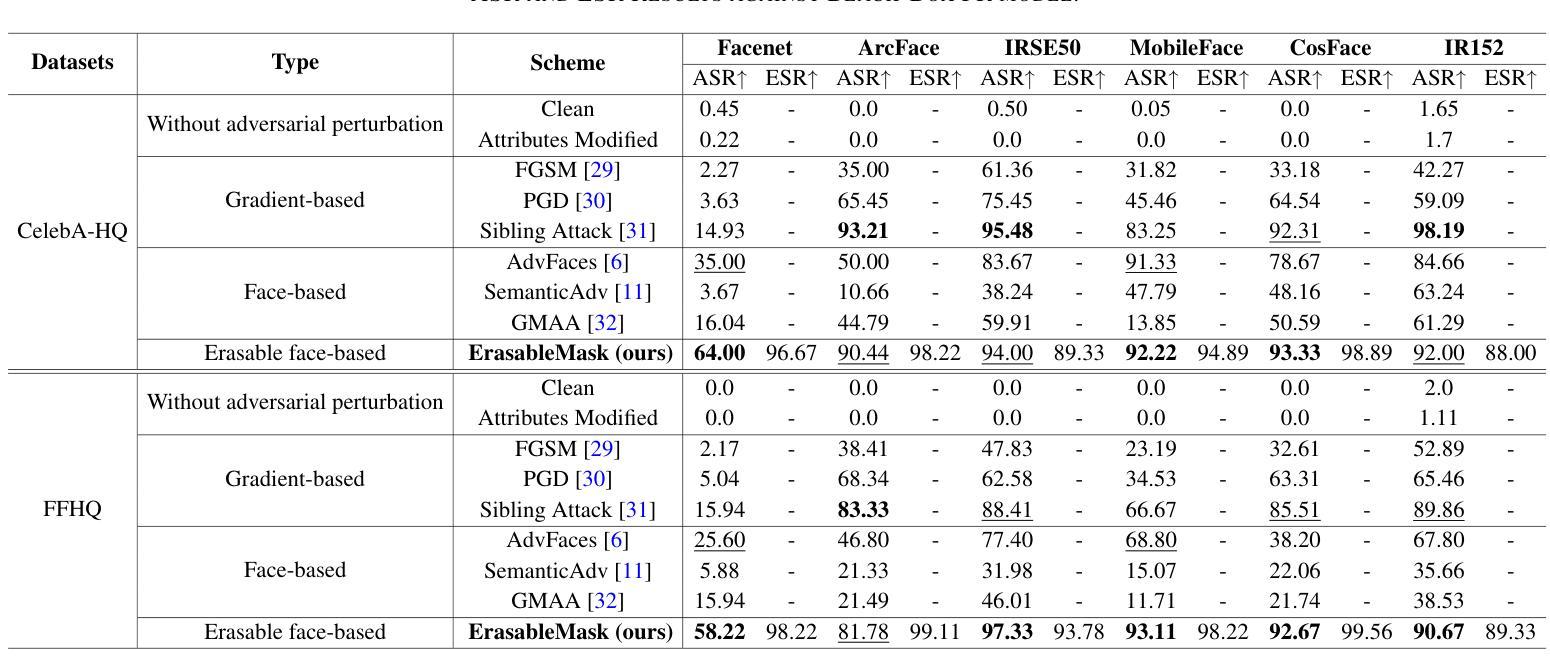