⚠️ 以下所有内容总结都来自于 大语言模型的能力,如有错误,仅供参考,谨慎使用
🔴 请注意:千万不要用于严肃的学术场景,只能用于论文阅读前的初筛!
💗 如果您觉得我们的项目对您有帮助 ChatPaperFree ,还请您给我们一些鼓励!⭐️ HuggingFace免费体验
2025-05-24 更新
LiveVLM: Efficient Online Video Understanding via Streaming-Oriented KV Cache and Retrieval
Authors:Zhenyu Ning, Guangda Liu, Qihao Jin, Wenchao Ding, Minyi Guo, Jieru Zhao
Recent developments in Video Large Language Models (Video LLMs) have enabled models to process long video sequences and demonstrate remarkable performance. Nonetheless, studies predominantly focus on offline video question answering, neglecting memory usage and response speed that are essential in various real-world applications, such as Deepseek services, autonomous driving, and robotics. To mitigate these challenges, we propose $\textbf{LiveVLM}$, a training-free framework specifically designed for streaming, online video understanding and real-time interaction. Unlike existing works that process videos only after one question is posed, LiveVLM constructs an innovative streaming-oriented KV cache to process video streams in real-time, retain long-term video details and eliminate redundant KVs, ensuring prompt responses to user queries. For continuous video streams, LiveVLM generates and compresses video key-value tensors (video KVs) to reserve visual information while improving memory efficiency. Furthermore, when a new question is proposed, LiveVLM incorporates an online question-answering process that efficiently fetches both short-term and long-term visual information, while minimizing interference from redundant context. Extensive experiments demonstrate that LiveVLM enables the foundation LLaVA-OneVision model to process 44$\times$ number of frames on the same device, and achieves up to 5$\times$ speedup in response speed compared with SoTA online methods at an input of 256 frames, while maintaining the same or better model performance.
近期视频大型语言模型(Video LLMs)的发展使得模型能够处理长视频序列并展现出卓越的性能。然而,研究主要集中在离线视频问答上,忽视了内存使用和响应速度在深度搜索服务、自动驾驶和机器人等现实应用中的重要性。为了缓解这些挑战,我们提出了LiveVLM,这是一个专为流媒体、在线视频理解和实时交互设计的无训练框架。与现有仅在提出问题后才处理视频的工作不同,LiveVLM构建了一个创新的面向流的KV缓存,以实时处理视频流,保留长期视频细节并消除冗余KV,确保对用户查询的即时响应。对于连续的视频流,LiveVLM生成并压缩视频键值张量(视频KV),以保留视觉信息并提高内存效率。此外,当提出一个新问题时,LiveVLM融入在线问答过程,能够高效提取短期和长期的视觉信息,同时最小化冗余上下文对结果的干扰。大量实验表明,LiveVLM使基础LLaVA-OneVision模型能够在同一设备上处理44倍的帧数,并在输入256帧时,实现了与现有先进在线方法相比最高达5倍的响应速度提升,同时保持相同或更好的模型性能。
论文及项目相关链接
Summary
视频大型语言模型(Video LLM)的最新发展使模型能够处理长视频序列并展现出卓越的性能。然而,研究主要集中在离线视频问答上,忽视了内存使用和响应速度在深度搜索服务、自动驾驶和机器人技术等现实应用中的重要性。为解决这些挑战,提出了无需训练的LiveVLM框架,专为流媒体、在线视频理解和实时交互设计。LiveVLM构建了一个创新的面向流的KV缓存,以实时处理视频流、保留长期视频细节并消除冗余KV,确保对用户查询的即时响应。它通过生成和压缩视频键值张量(video KVs)来提高内存效率,同时为新问题提供在线问答过程,高效获取短期和长期视觉信息,并最小化冗余上下文的干扰。实验表明,LiveVLM使基础LLaVA-OneVision模型的帧处理速度提高了44倍,在输入256帧的情况下,与最新在线方法相比,响应速度提高了5倍,同时保持了相同的或更好的模型性能。
Key Takeaways
- Video LLMs能处理长视频序列,但在现实应用如Deepseek服务中,内存使用和响应速度至关重要。
- LiveVLM是一个面向流的框架,专为在线视频理解和实时交互设计。
- LiveVLM通过构建KV缓存实时处理视频流,保留长期视频细节并消除冗余信息。
- LiveVLM生成和压缩视频键值张量(video KVs),提高内存效率。
- LiveVLM能在新问题提出时提供高效的在线问答过程,结合短期和长期视觉信息。
- 实验显示LiveVLM大幅提高了帧处理速度和响应速度。
点此查看论文截图
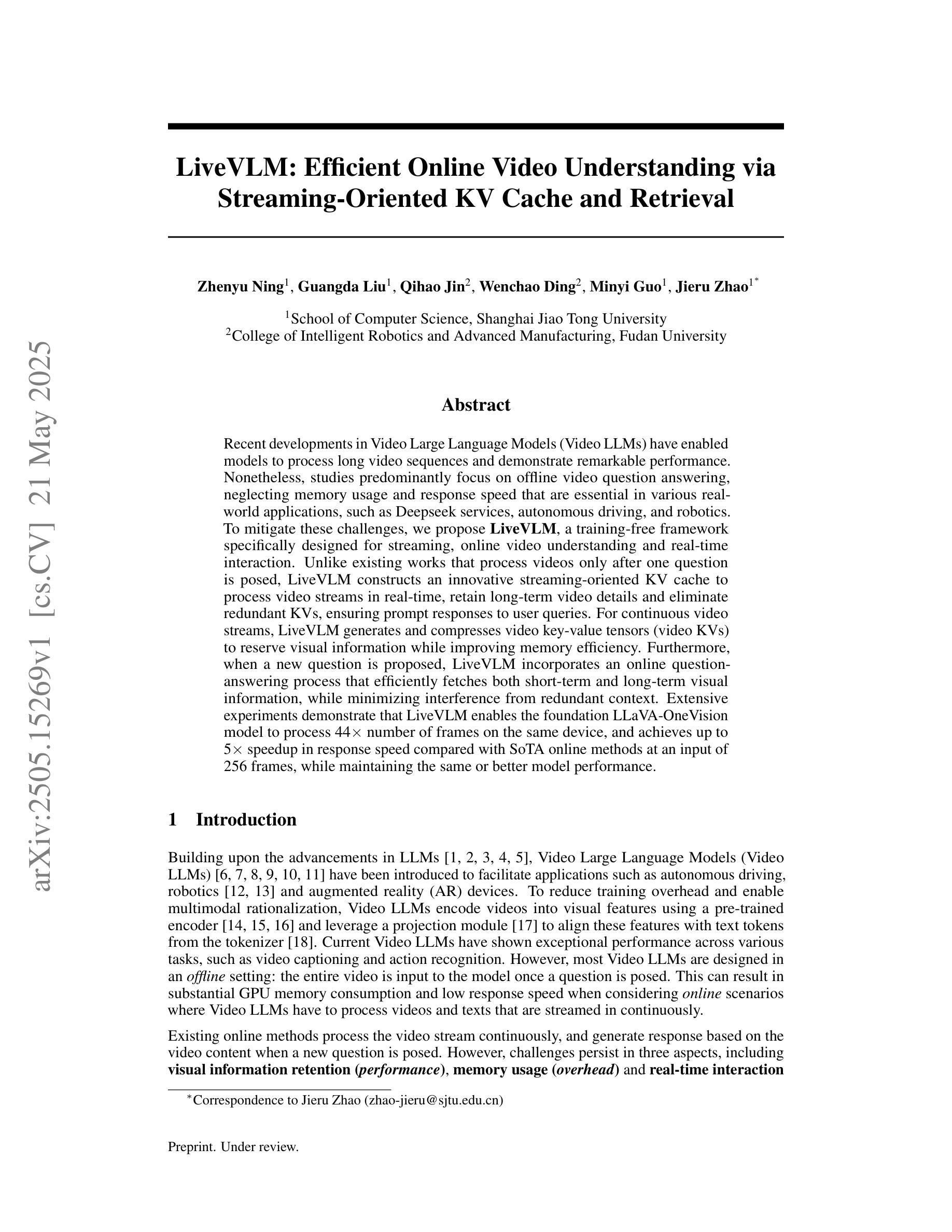
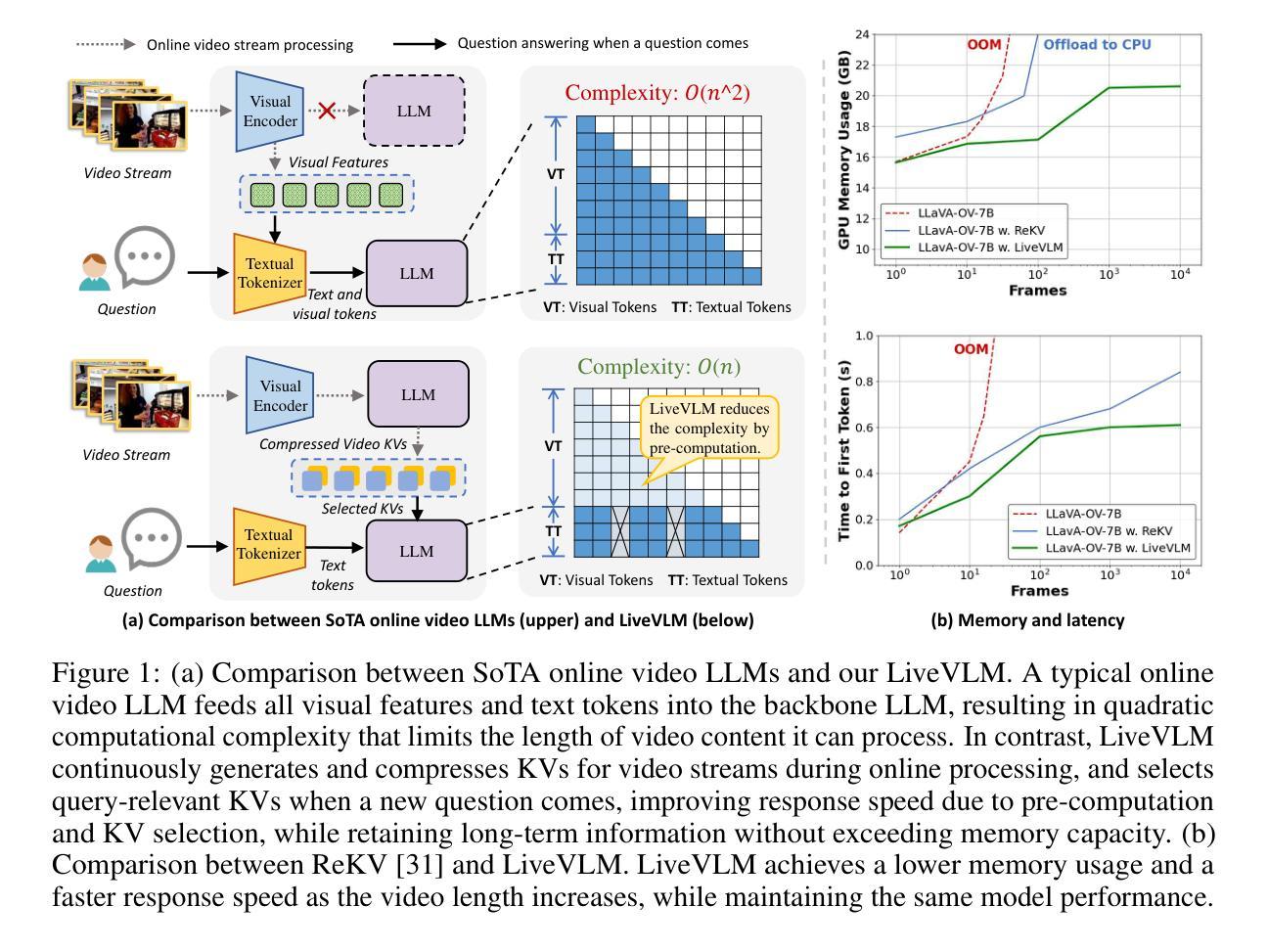
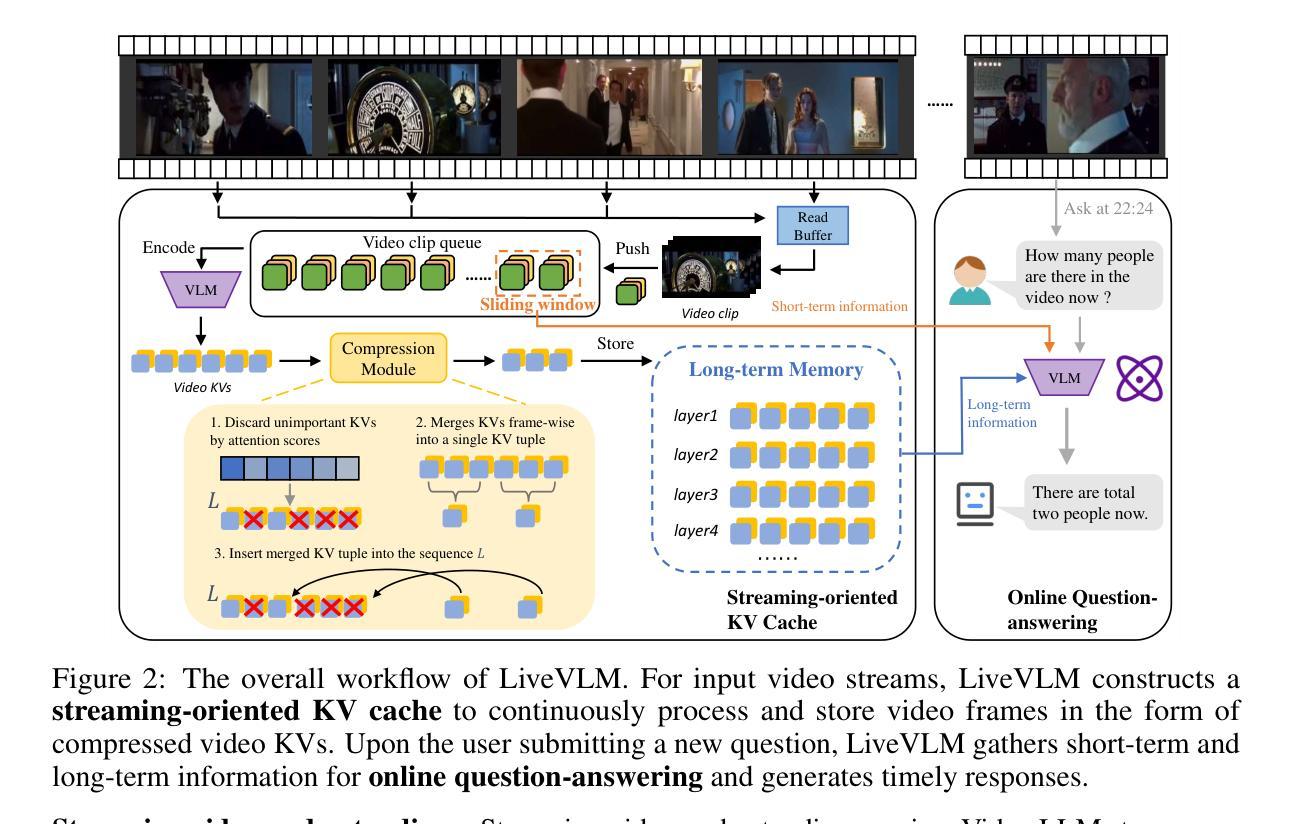
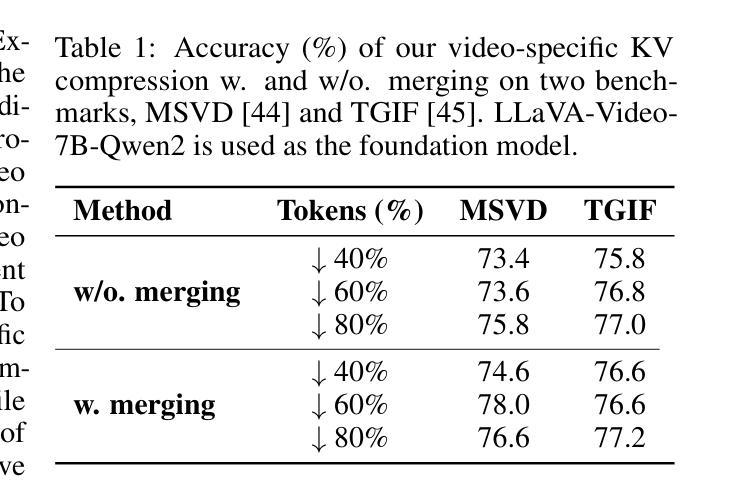
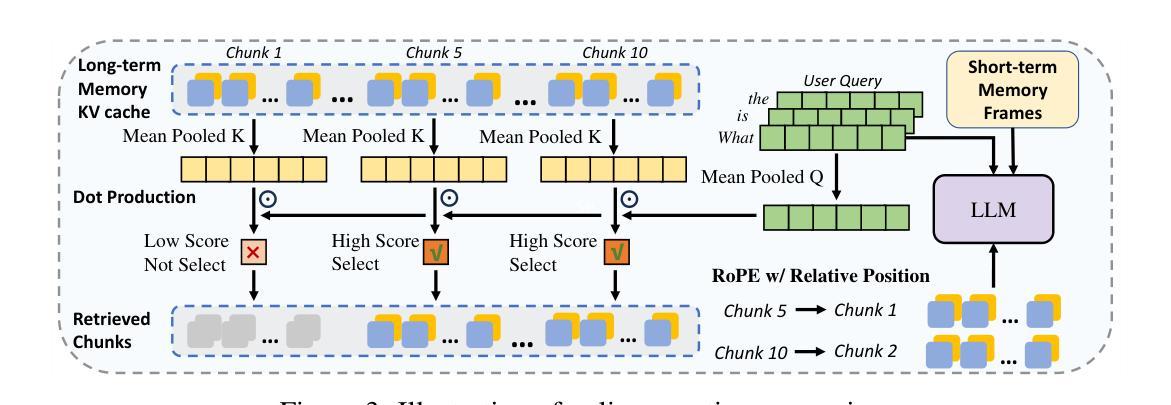